An Analytic Potential Model for Symmetric and Asymmetric DG MOSFETs
层状双金属氢氧化物微观结构与性质的理论研究进展
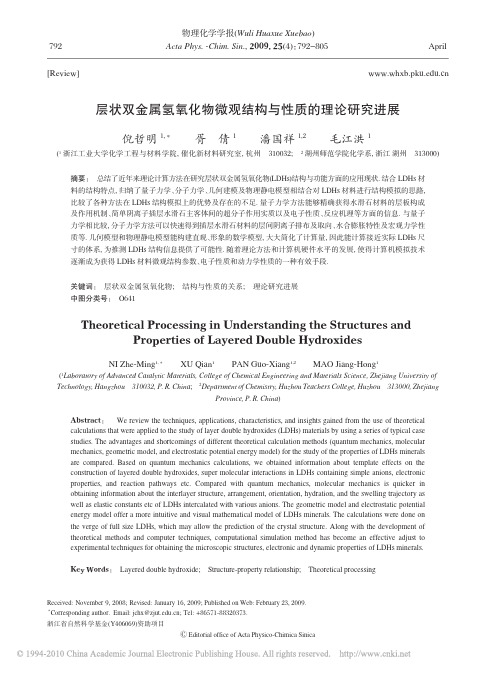
层状双金属氢氧化物微观结构与性质的理论研究进展倪哲明1,*胥倩1潘国祥1,2毛江洪1(1浙江工业大学化学工程与材料学院,催化新材料研究室,杭州310032;2湖州师范学院化学系,浙江湖州313000)摘要:总结了近年来理论计算方法在研究层状双金属氢氧化物(LDHs)结构与功能方面的应用现状.结合LDHs 材料的结构特点,归纳了量子力学、分子力学、几何建模及物理静电模型相结合对LDHs 材料进行结构模拟的思路,比较了各种方法在LDHs 结构模拟上的优势及存在的不足.量子力学方法能够精确获得水滑石材料的层板构成及作用机制、简单阴离子插层水滑石主客体间的超分子作用实质以及电子性质、反应机理等方面的信息.与量子力学相比较,分子力学方法可以快速得到插层水滑石材料的层间阴离子排布及取向、水合膨胀特性及宏观力学性质等.几何模型和物理静电模型能构建直观、形象的数学模型,大大简化了计算量,因此能计算接近实际LDHs 尺寸的体系,为推测LDHs 结构信息提供了可能性.随着理论方法和计算机硬件水平的发展,使得计算机模拟技术逐渐成为获得LDHs 材料微观结构参数、电子性质和动力学性质的一种有效手段.关键词:层状双金属氢氧化物;结构与性质的关系;理论研究进展中图分类号:O641Theoretical Processing in Understanding the Structures and Properties of Layered Double HydroxidesNI Zhe -Ming 1,*XU Qian 1PAN Guo -Xiang 1,2MAO Jiang -Hong 1(1Laboratory of Advanced Catalytic Materials,College of Chemical Engineering and Materials Science,Zhejiang University of Technology,Hangzhou 310032,P.R.China ;2Department of Chemistry,Huzhou Teachers College,Huzhou 313000,ZhejiangProvince,P.R.China )Abstract :We review the techniques,applications,characteristics,and insights gained from the use of theoretical calculations that were applied to the study of layer double hydroxides (LDHs)materials by using a series of typical case studies.The advantages and shortcomings of different theoretical calculation methods (quantum mechanics,molecular mechanics,geometric model,and electrostatic potential energy model)for the study of the properties of LDHs minerals are compared.Based on quantum mechanics calculations,we obtained information about template effects on the construction of layered double hydroxides,super molecular interactions in LDHs containing simple anions,electronic properties,and reaction pathways pared with quantum mechanics,molecular mechanics is quicker in obtaining information about the interlayer structure,arrangement,orientation,hydration,and the swelling trajectory as well as elastic constants etc of LDHs intercalated with various anions.The geometric model and electrostatic potential energy model offer a more intuitive and visual mathematical model of LDHs minerals.The calculations were done on the verge of full size LDHs,which may allow the prediction of the crystal structure.Along with the development of theoretical methods and computer techniques,computational simulation method has become an effective adjust to experimental techniques for obtaining the microscopic structures,electronic and dynamic properties of LDHs minerals.Key Words :Layered double hydroxide;Structure -property relationship;Theoretical processing[Review]物理化学学报(Wuli Huaxue Xuebao )Acta Phys.-Chim.Sin .,2009,25(4):792-805Received:November 9,2008;Revised:January 16,2009;Published on Web:February 23,2009.*Corresponding author.Email:jchx@;Tel:+86571-88320373.浙江省自然科学基金(Y406069)资助项目鬁Editorial office of Acta Physico -Chimica SinicaApril792No.4倪哲明等:层状双金属氢氧化物微观结构与性质的理论研究进展层状双金属氢氧化物(layer double hydroxides,简称LDHs)是一类重要的新型无机功能材料,可作为制备无机-无机、无机-有机纳米复合材料的母体[1].因其具有特殊的层状结构及物理化学性质,在催化[2-5]、离子交换和吸附[6-8]、医药[9-13]等诸多领域具有广阔的应用前景.目前,粉末X射线衍射(XRD)、红外(IR)、热重差热分析(TG-DTA)、核磁共振(NMR)、X射线光电子能谱技术(XPS)、透射电镜(TEM)、中子衍射等实验手段已被应用于LDHs微观结构研究中,并取得了一些重要的结构数据[14-17].比如,LDHs微观结构研究中最为重要的XRD表征技术,能够得到合成材料是否具有层状构型,以及层间距、层板中金属离子的平均间距等重要结构参数[14].将插层阴离子的尺寸与层间距大小相关联,能推测阴离子在层间大致的排布情况(比如单层垂直、双层垂直交替、倾斜交替等)[14].IR、TG-DTA、NMR、中子衍射技术可作为辅助手段来佐证XRD推测所得LDHs微观结构的正确性[15-17].然而,对于复杂阴离子插层LDHs体系,其层间阴离子的排布形态确认仍存在困难,层间结构水分子的排布信息,从实验手段进行表征更是无法得知的.LDHs单晶合成非常有限(迄今为止只合成了2、3个LDHs单晶[1,18]),因而要从实验角度精确探求LDHs微观结构还存在相当大的困难.同时,要探求LDHs层板内部金属离子与羟基之间、主体层板与客体阴离子及水分子之间的超分子作用实质,从实验角度也是很难解决的.近年来,理论模拟计算作为获得LDHs材料微观结构信息和动力学性质的一种有效手段,为LDHs材料的分子设计提供了理论指导.本文总结了近年来理论计算方法在LDHs材料结构与功能方面的研究进展.结合LDHs 材料的结构特点,归纳了量子力学、分子力学、几何建模及物理静电模型相结合对LDHs材料进行结构模拟的思路,比较了各种方法在LDHs结构模拟上的优势及存在的不足.1层状LDHs材料微观结构与功能的关系LDHs是一类具有主体氢氧化物层板、客体阴离子柱撑的无机功能材料,其结构与水镁石结构类似[19,20].LDHs由水滑石(Mg6Al2(OH)16CO3·4H2O)经层板金属阳离子与层间阴离子调变而形成,其结构如图1所示,组成通式为[M II1-x M III x(OH)2]x+(A n-)x/n·m H2O,其中M II和M III分别代表二价和三价金属离子;x 是摩尔比n(M3+)/(n(M2+)+n(M3+));A n-是层间阴离子;m为层间结构水分子数目.LDHs独特的层状构型和结构的可调变性决定了其功能的多样性和应用的广泛性,研究LDHs材料微观结构与功能间的关系,必须对材料的精细结构取得足够的了解.LDHs材料的功能特性按照其微观和亚微观结构(三级结构:主体氢氧化物的层板、层间客体阴离子、结构水分子)进行划分,大致可分为以下三个方面:(1)层板有序结构主要由金属离子与羟基通过配位键形成,主体层板金属离子具有同晶可取代性.LDHs层状材料经合适温度焙烧,能够形成具有高表面积、高分散性、酸碱性可调的复合氧化物.焙烧产物中某些过渡金属经还原,便可形成性能优良的纳米金属负载型催化剂.由于LDHs层板金属离子具有可调变性(如Mg2+、Zn2+、Co2+、Ni2+、Cu2+和Al3+、Fe3+、Cr3+等),所以LDHs的焙烧/还原衍生物在催化、吸附等领域得到广泛应用.(2)层间客体阴离子具有可组装性.LDHs具有客体阴离子可插层性,主体层板与层间客体阴离子间存在着静电和氢键等分子间作用力,很多功能性阴离子能通过这些分子间相互作用而被引入LDHs 层间,形成一类新型LDHs基复合材料.比如:(a)杂多、同多酸阴离子、金属配离子;(b)羧酸、磺酸类有机阴离子;(c)布洛芬、萘普生等医药分子及草甘膦等农药分子;(d)氨基酸、DNA、核苷酸、ATP、酶等生物分子.这些分子/离子与LDHs组装后,能应用于催化、PVC改性剂、药物缓释、无机/生物复合材料、紫外阻隔材料、油田化工品、环境修复等领域.(3)层间水合膨胀及剥离特性.实验证明层间水分子具有稳定LDHs结构的作用,层间水分子与层板及阴离子间主要以氢键作用相结合,并能在一定温度下可逆脱除/吸收,所以在湿度调节方面得图1LDHs材料的结构示意图Fig.1Scheme of microstructure for LDHs793Acta Phys.-Chim.Sin.,2009Vol.25到应用.其他有机中性分子通过克服主客体间超分子作用力被引入LDHs层间,能使LDHs材料层间距膨胀乃至剥离,形成纳米LDHs层片,应用于LDHs/高分子复合材料的合成中.根据Lehn对超分子化学的阐述[21],LDHs主体层板形成、层板中M2+(M3+)-OH-(H2O)作用机制、层板与层间阴离子及水分子间相互作用都可纳入超分子化学研究范畴.从超分子化学角度,使用实验表征与理论模拟计算方法研究LDHs层板内部金属离子与羟基之间、层板与客体阴离子及水分子之间的相互作用,可取得LDHs材料结构调变与其功能间的关系.LDHs超分子结构与功能的基础性研究工作的进展,将有助于LDHs功能的强化以及应用领域的拓展.2理论方法研究LDHs材料的结构与性质近年来,理论模拟计算作为有效的研究方法在LDHs材料的微观结构、力学、热学、电磁学等性能的研究中卓有成效.其中,计算机模拟研究根据模拟尺度、理论依据、研究性质的不同,可分为量子力学(quantum mechanics,QM)和分子力学方法(molecular mechanics,MM)两大类,它被认为是本世纪以来除理论分析和实验观察之外的第三种科学研究手段,称之为“计算机实验”[22].2.1量子力学方法量子力学方法主要包括:从头算法(ab initio method)、分子轨道半经验计算法(semi-empirical molecular orbital method)和密度泛函理论(density functional theory,DFT)[22,23].它以分子中电子的非定域化(delocalization)为基础,电子的行为以其波函数表示.根据海森堡(Heisenberg)的测不准原理,量子力学仅能计算区间内电子出现的概率,其概率正比于波函数绝对值的平方.通过求解由核和电子组成的多电子体系的薛定谔方程,从而获得LDHs材料结构与功能方面的信息[22-24].它建立在原子核与核外电子作用基础上,计算精度高.量子力学方法适用于简单的分子或电子数量较少的体系,能够精确计算分子的性质、结构、构象、偶极矩、电离能、电子亲和力、电子密度等,以及了解分子间相互作用的电子性质.2.1.1从头算法从头算法利用变分原理(variation principle),将系统电子的波函数展开为原子轨道波函数的组合,而原子轨道的波函数又为一些特定的数学函数(如高斯函数)的组合[22,23].这种方法虽然精确,却甚为缓慢,所能计算的系统亦极为有限,通常不超过100个原子.目前,该方法主要应用于LDHs材料精确结构参数[25]、金属离子同晶取代对层板结构的影响[26]、酸碱性等物化性质的影响[26]、催化反应机理、催化剂活性[26]以及电子相关性质(态密度、能带结构)[26]方面的计算.Masini等[25]运用ab initio Car-Parrinello模拟了水镁石(brucite)表面的羟基化和脱羟基作用反应,在获得水镁石(0001),(1100)和(1000)晶面结构特性的同时,通过比较水镁石表面和内部的脱羟基反应所需的能量,指出水镁石分解生成MgO和H2O的反应首先在材料表面发生.Trave等[26]计算了含有不同层板阳离子和层间阴离子的LDHs体系的晶格参数、自由度等结构信息,探讨了层板Al3+的排列方式和Mg/Al摩尔比两个参数对Mg/Al-LDHs结构的影响,当R(M II/M III 摩尔比)约为3时,体系的结合能最低,而层间通道高度最大,建立了结构参数与化学组成和结合能之间的关系.另一方面,通过对态密度等电子性质的研究,发现层间阴离子的性质对化合物的电子性质有很大的影响.与LDHs-Cl体系相比,LDHs-OH体系最高占据轨道(HOMO)-最低空轨道(LUMO)间的禁带宽度较小,且层间的LUMO轨道主要由层间的阴离子提供(如图2),导致了LDHs-OH具有更强的接受电子的能力,所以LDHs-OH较LDHs-Cl在羟醛缩合碱催化反应中具有更高的碱催化活性.李蕾等[27]在制备表征磺基水杨酸、4-羟基-3-甲氧基肉桂酸和2-羟基-4-甲氧基二苯甲酮-5-磺酸等紫外吸收剂插层锌铝水滑石的基础上,运用(a)(b)图2(a)LDHs-Cl和(b)LDHs-OH的HOMO和LUMO轨道分布图[26]Fig.2Spatial distributions of the HOMO and LUMO for(a)LDHs-Cl and(b)LDHs-OH [26]794No.4倪哲明等:层状双金属氢氧化物微观结构与性质的理论研究进展Gaussian98[28]软件中的ab initio分子轨道法(HF/6-31G)计算了LDHs层间有机紫外吸收剂的分子结构和电荷分布,提出了合理的客体阴离子在主体层间的排列方式,并分析了其结构与光化学行为的关系.结果表明,层板间的限域空间有利于主客体间的静电和氢键相互作用,插层产物的紫外吸收范围和能力显著增强,是一类具有潜在应用价值的无机-有机超分子复合结构的紫外吸收材料.2.1.2分子轨道半经验方法分子轨道半经验方法在从头算法的基础上,多引用了一些实验值为参数,求解Hartree-Fock-Roothaan 方程,以取代计算真正的积分项[22,23].采用此方法计算LDHs材料时,相关的结构参数如键长、键角等,往往不是通过几何优化获得,而是来源于实验文献.目前常用的分子轨道半经验方法有休克尔方法(EHMO),全略微分重叠(CNDO),间略微分重叠(INDO),改进的间略微分重叠(MINDO),忽略双原子微分重叠(MNDO),以及在忽略双原子微分重叠(MNDO)基础上发展起来的AM1方法和PM3方法等[22,23].与从头算法相比,利用半经验分子轨道方法可计算较大的分子,但需以大量的实验数据为基础.Pu等[29,30]采用MNDO/d、PM3法优化了不同尺寸水滑石层板结构,优化所得的晶体团簇模型呈六边形结构,且层板的Mg/Al摩尔比为可变参数,但当层板直径变得比较大时,Mg/Al摩尔比的极限值为3.在插层反应过程中,层板的边缘较容易吸附阳离子,而层板中心则易接受阴离子.在此基础上,他们采用B3PW91方法在Lanl2dz基组水平上进一步研究了Mg/Al摩尔比为3的水滑石层板结构[31],通过将结构参数和模拟得到的XRD图谱分别与实验观测结果相比较,确定了水滑石层板符合空间群R3m(166),沿第三维方向有序堆积.李蕾等[32]采用以含原子对排斥的EHMO法ASED(atom superposition and electron delocalization) -MO法为基础的结构自动优化EHTOPT程序,优化计算了Mg6Al2(OH)16X·H2O主体层板与不同简单客体阴离子稳定结构的能量变化、成键状况及电荷转移情况,揭示了LDHs层板与层间阴离子间存在静电吸引、氢键等非共价键弱相互作用.其中氢键作用为主,且强弱与阴离子电荷分布、空间排布方式密切相关,层间阴离子电荷分布对层板酸碱性变化也有影响.2.1.3密度泛函理论密度泛函理论是一种研究多电子体系电子结构的量子力学方法,即为非常精确的量子计算方法.在Kohn-Sham DFT的框架中,最难处理的多体问题(由于处在一个外部静电势中的电子相互作用而产生的)被简化成了一个没有相互作用的电子在有效势场中运动的问题[23].密度泛函理论是目前多种领域中电子结构计算的领先方法,但是用它来恰当地描述分子间相互作用,特别是范德华力(van der Waals),或者计算半导体的能隙还存在一定的困难.目前该方法主要被应用于研究LDHs材料层板金属离子同晶取代对层板结构的影响[31]、层板内部金属离子与羟基之间配位作用[33]、简单阴离子插层LDHs材料的微观结构性质和主客体间超分子作用实质[34-36]、成键规律、热稳定性、酸碱性等物化性质、离子交换性能、催化性能[32-37]、反应机理[38,39]等相关领域的研究.Wei等[33]采用混合密度泛函B3LYP方法对构建LDHs时产生的模板效应进行了研究.根据计算所得的结构畸变角θ的不同,可将层板金属离子分为三类(如图3):类型I(canonical structure,θ:0°-1°),类型II(slightly distorted structure,θ:1°-10°)和类型III(heavily distorted structure,θ>10°).计算了与LDHs 结构相关的键长、O—M—O键弯曲角、键能、价电图3[M(H2O)6]n+的三种几何优化结构[33]Fig.3Three types of optimized structures of the[M(H2O)6]n +[33]795Acta Phys.-Chim.Sin.,2009Vol.25子构型、配位场以及Jahn-Teller效应和自然键轨道(NBO).指出同晶取代Mg2+进入LDHs层板的金属阳离子,其与氧原子形成的八面体六配位的畸变角较离子半径对LDHs的层板结构有着更大的影响,且影响程度为类型I>类型II>类型III.倪哲明等[34,35]建立了LDHs与卤素阴离子(F-、Cl-)的单层簇模型,运用Gaussian03[40]程序,采用混合密度泛函B3LYP方法,在6-31G(d)基组水平上进行结构优化和频率分析,然后分别用6-31G(d)和6-311++G(d,p)计算主客体相互作用能,分析了LDHs 主体层板与卤素阴离子的超分子作用(静电作用和氢键作用),并对F-、Cl-超分子作用的强弱进行了比较.然后又建立了LDHs与CO2-3、H2O的双层簇模型,采用B3LYP/6-31G(d)//B3LYP/3-21G方法计算类水滑石(LDHs-CO3-n H2O)的结构与能量,探讨了主客体间的超分子作用,并对LDHs层间存在的三种不同类型氢键的强度进行了比较.在此基础上,还构建LDHs-Cl-n H2O周期性模型(如图4),采用了CASTEP[41]优化计算其微观结构,从结构参数、Mulliken电荷布居、态密度(DOS)、能量等角度研究层间Cl-和不同数目水分子的分布形态以及与LDHs层间的超分子作用[36].随着水分子数的增加,层间距逐渐增大后趋于平衡.水合过程中氢键作用比静电作用更占优势,layer-water型氢键要略强于anion-water型氢键.当n=1、2时,Cl-与水分子所在平面以平行层板的方式存在于LDHs层间,并且与两层板的距离基本相等;当n=3、4时,Cl-与水分子则以偏向某一层的方式随机地存在于LDHs 层板间,并且得出LDHs-Cl的水合具有饱和量.Anderson等[37]在实验合成sulfonato-salen-M III (M=Mn,Fe,Co)配合物插层Zn/Al-LDHs的基础上,在分子氧和常温、常压条件下,测试其对生成环己烯和二聚环戊二烯的环氧化反应的催化性能.采用混合密度泛函B3LYP方法,计算了sulfonato-salen-M III (M=Mn,Fe,Co)的结构参数(如图5),发现不同插层产物的层间通道高度和催化行为是有区别的,中心金属离子的性质较大地修饰了催化活性位附近的化学环境,从而导致了不同配合物的催化活性不同,催化性能大小为LDH-[Fe(Cl)(salen)]<LDH-[Co(Cl) (salen)]<LDH-[Mn(Cl)(salen)].Greenwell等[38]采用平面波密度泛函理论(PW-DFT)研究了镁铝水滑石催化叔丁醇酯交换反应的机理.通过计算反应机理中涉及的过渡态,指出Choudary等提出的机理(如图6(a))中催化剂再生的步骤无法实现.通过一系列计算模拟,提出层间水的图4LDHs-Cl-n H2O(n=1-4)主客体作用模型[36]Fig.4Host-guest interaction models of LDHs-Cl-n H2O(n=1-4)[36]图5Sulfonato-salen-M III配合物的几何结构[37]Fig.5Computed structures of sulfonato-salen-M III [37] 796No.4倪哲明等:层状双金属氢氧化物微观结构与性质的理论研究进展存在是催化剂再生的必要条件(如图6(b)),叔丁基阳离子在层间不可能单独存在,活性部分叔丁醇的羟基—OH与LDHs层板间存在着比较强的相互作用.LDHs层板的亲水性与t-Bu-LDH的层间区域的疏水性,导致有机物分子的极化基团排列于LDHs 表面,增强了材料的催化活性,促进整个催化反应的进行.Wei等[39]采用混合密度泛函B3PW91方法在6-31G(d,p)基组水平上,对手性药物左旋多巴(L-dopa)插层前后的外消旋现象进行了研究.对反应中涉及的过渡态的模拟结果表明,插层前左旋多巴单体手性碳上的H会迁移至羧基,构成烯醇式的活性中间体,从而外消旋化.插层后,左旋多巴的羧基会和LDHs层板发生强的主客体相互作用(相互作用能为-1100kJ·mol-1),而无法成为质子接受体,外消旋化得到了抑制.从而证明了LDHs材料是一种很好的用来贮存和运载手性药物的载体.2.2分子力学方法分子力学方法起源于1970年左右,是依据经典力学(classical mechanics)的计算方法.此种方法主要依据Born-Oppenheimer近似原理,计算中将电子的运动忽略,而将系统的能量视为原子核位置的函数[23,24].以原子间相互作用势为基础,主要依据分子的力场计算分子的各种特性.该方法主要包括:能量最小化(energy minimization)、蒙持卡罗计算法(Monte Carlo method,MC)和分子动力学模拟(molecular dynamics simulation,MD).分子力场中的关于LDHs材料的结构参数通常可经由量子力学计算或实验方法得到.与量子力学相比较,此方法可以快速地得到分子的各种性质,但无法得到有关体系电子性质方面的结果.分子力学方法常被用于药物、团簇体、生化大分子的研究、复杂的有机阴离子(药物、氨基酸、DNA)插层LDHs复合材料超分子结构的优化计算.2.2.1能量最小化法能量最小化方法是将最初的结构进行模型修饰(model refinement)的过程,藉由分子力学的能量最小化来修正不利的非共价碰触及达到理想的键结合和能量最低的构型.分子力学方法主要计算包括键长、键角、二面角、静电作用力和范德华作用力等位能参数,它的能量计算公式:E total=E stretching+E bending+E dihedral+E out-of-plane+E cross terms+E van der Waals+E coulombic在分子力学方法中常用于能量最小化的力场有CHARMM[42],AMBER[43],CVFF[44],CFF91[45]或GROMOS[46]等.Fogg等[47]采用GULP程序(general utility lattice program)计算了Li/Al-LDHs-Cl、Li/Al-LDHs-Br和Li/ Al-LDHs-NO3体系的最优结构.模拟结果表明,能量最低时所得的结构与实验表征的结果相一致,能够较好地解释NO-3在Li/Al-LDHs-NO3层板间的无规律排列现象.这种计算方法同时也被认为是研究Li/ Al-LDHs-CO3、Li/Al-LDHs-SO4和Li/Al-LDHs-C2O4等体系层间间距和层间客体排布取向的有效手段. 2.2.2蒙特卡罗计算法蒙特卡罗计算法借由系统中质点(原子或分子)的随机运动,结合统计力学的概率分配原理,以得到体系的统计及热力学资料.此方法多用以研究复杂体系及金属的结构及其相变性质.蒙特卡罗计算法的弱点在于只能计算统计的平均值,不能追踪势能变化的路径,无法得到系统的动态信息[24].此计算所依据的随机运动并不适合物理学的运动原理,与其他的非量子计算方法相较亦非特别经济快速.因此,自分子动力学计算逐渐盛行后,蒙特卡罗计算方(a)Choudary et al.′s proposed mechanism(b)Greenwell et al.′s proposed mechanism图6两种可能的酯交换反应机理[38]Fig.6Two possible reaction mechanism for the transesterification reaction [38]797Acta Phys.-Chim.Sin.,2009Vol.25法已较少为人所采用.Kirkpatrick等[48]采用NMR与Monte Carlo模拟相结合的方法,观察模拟了SO2-3、SeO2-4、PO2-4、HPO3-4、MoO2-4、ClO-4、SeO2-3、CO2-3、F-、Cl-、Br-、I-、OH-和NO-3阴离子进入Li/Al和Mg/Al-LDHs后与层间水相互影响可能发生的三种膨胀情况,并分析了相对湿度(RH)对该体系膨胀行为的影响.类型I,明显膨胀行为,膨胀尺度为0.15-0.30nm,通过XRD和NMR 测定发现,层间水从单层排列转变为双层排列,与吸附水等温曲线相一致.类型II,轻微膨胀行为,膨胀尺度<0.05nm,伴随着大量的层间水交换行为;层间水在层间只呈单层排列,且随着RH的增加,层间阴离子会经历一个动力学无序化阶段.类型III,基本不产生膨胀行为,膨胀尺度为0-0.02nm,只有微量的层间水交换行为;由于层间客体的紧密排列,只有极少量的水分子被吸收进入层间.此外,由于阴离子的体积较小,使其和层板羟基之间存在着强烈的静电作用和氢键作用,导致RH对环境结构和阴离子的动态特征几乎没有影响.2.2.3分子动力学模拟分子动力学模拟是应用力场及根据牛顿运动力学原理所发展的计算方法[23],在物理学、化学、生物学和材料科学等许多领域中得到广泛地应用.与蒙特卡罗计算方法比较,分子动力学模拟时系统中粒子的运动有正确的物理依据.优点是精准性高,可同时获得系统的动态与热力学统计资料,并可广泛地适用于各种系统及各类特性的探讨.分子动力学模拟方法本身亦有一定的限制,由于此计算需要引用数理积分方法,因此仅能研究系统短时间范围内的运动,而无法模拟一些时间较长的运动问题[24].MD方法主要用于软性层状粘土材料的模拟,在可变层间空间条件下,计算LDHs层间客体(简单阴离子、有机大分子、生物分子)的排布方式及取向[49-55]、层间阴离子与主体层板的成键情况、吸附、水合膨胀特性模拟[50-59]以及氢键结构分析[57-60],预测化学反应的活性及产物分析[55],LDHs材料XRD图谱模拟计算[61,62],药物分子在层板间的存在形式、结合情况[63-65]、热效应[62]等方面的计算模拟研究.Ma等[50]对包含水分子和不同客体阴离子(CO2-3、SO2-4、OH-、F-、Cl-、Br-、NO-3)的α-Ni(OH)2(1)、β-Ni(OH)2 (2)和Ni/Al-LDHs(3)三个体系的结构进行了分子动力学模拟,发现对于α-Ni(OH)2和Ni/Al-LDHs体系,当层间阴离子为F-、Cl-、Br-、OH-、NO-3、CO2-3时,层间水分子的堆积模式为紧密堆积(如图7(a));当层间阴离子为SO2-4时,层间水分子的排列方式相对松散(如图7(b));且对于Ni/Al-LDHs-SO2-4体系,由于层间含水量较高,水分子在层间为双层排布(如图7(c)).同时,对β-Ni(OH)2体系水合能以及无水β-Ni(OH)2的相对结合能进行了有关计算,为不同阴离子在离子交换反应的相对亲和力提供了理论依据,并指出库仑引力是影响势能的主要因素,层间阴离子的净电荷和范德华半径以及层间水的含量都对结合能有显著的影响,尤其当层间为有机阴离子时,效果更为显著.Lombardo等[62]首次以实验XRD和MD相结合的方法应用于获取热处理过程中低晶状[Zn0.65Al0.35 (OH)2]Cl0.35·0.35H2O(I)和[Zn0.65Al0.35(OH)2](CO3)0.175·0.69H2O(II)的结构、动力学等性质.他们工作的亮点是MD模拟所得到的XRD谱图是通过计算一系列晶胞参数不同的结构模型拟合得到的,而不是传图7层间水分子的堆积方式[50]Fig.7Schematic to show possible packing styles of interlayer water molecules [50] 798。
潜在类别模型在试题分析中的应用
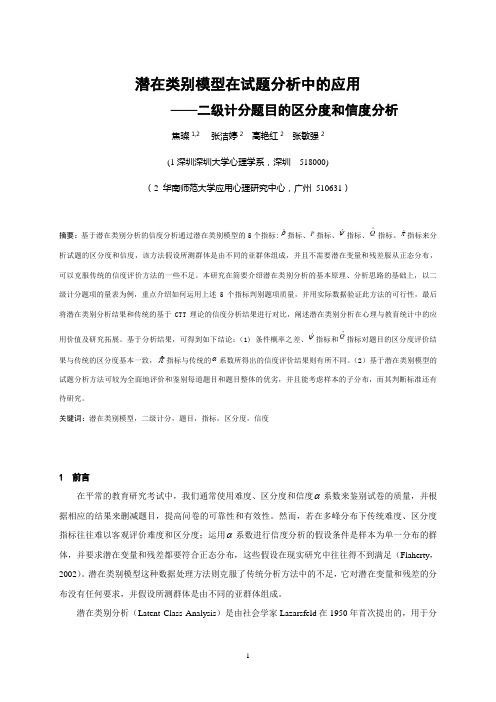
潜在类别模型在试题分析中的应用——二级计分题目的区分度和信度分析焦璨1,2 张洁婷2高艳红2张敏强2(1深圳深圳大学心理学系,深圳518000)(2 华南师范大学应用心理研究中心,广州510631)摘要:基于潜在类别分析的信度分析通过潜在类别模型的5个指标:ρˆ指标、P指标、ψˆ指标、Qˆ指标、πˆ指标来分析试题的区分度和信度,该方法假设所测群体是由不同的亚群体组成,并且不需要潜在变量和残差服从正态分布,可以克服传统的信度评价方法的一些不足。
本研究在简要介绍潜在类别分析的基本原理、分析思路的基础上,以二级计分题项的量表为例,重点介绍如何运用上述5个指标判别题项质量,并用实际数据验证此方法的可行性,最后将潜在类别分析结果和传统的基于CTT理论的信度分析结果进行对比,阐述潜在类别分析在心理与教育统计中的应用价值及研究拓展。
基于分析结果,可得到如下结论:(1) 条件概率之差、ˆψ指标和ˆQ指标对题目的区分度评价结果与传统的区分度基本一致,ˆπ指标与传统的α系数所得出的信度评价结果则有所不同。
(2)基于潜在类别模型的试题分析方法可较为全面地评价和鉴别每道题目和题目整体的优劣,并且能考虑样本的子分布,而其判断标准还有待研究。
关键词:潜在类别模型,二级计分,题目,指标,区分度,信度1 前言在平常的教育研究考试中,我们通常使用难度、区分度和信度α系数来鉴别试卷的质量,并根据相应的结果来删减题目,提高问卷的可靠性和有效性。
然而,若在多峰分布下传统难度、区分度指标往往难以客观评价难度和区分度;运用α系数进行信度分析的假设条件是样本为单一分布的群体,并要求潜在变量和残差都要符合正态分布,这些假设在现实研究中往往得不到满足(Flaherty,2002)。
潜在类别模型这种数据处理方法则克服了传统分析方法中的不足,它对潜在变量和残差的分布没有任何要求,并假设所测群体是由不同的亚群体组成。
潜在类别分析(Latent Class Analysis)是由社会学家Lazarsfeld在1950年首次提出的,用于分析态度量表中潜在变量的一种方法(Lazarsfeld & Henry,1986)。
Analytical model

An Effective Potential for Composite Operators

1. Introduction
It is well known that the effective potential for elementary fields is gauge dependent[1]. The effective potential can be used in the studies of spontaneous symmetry break, inflationary cosmology and many other problems. It is important to examine if the gauge dependence of the effective potential causes the physical quantities to be gauge dependent. Nielsen discovered an important identity on the gauge-dependence of the effective potential[2]. With the Nielsen identity and its variations, many physical quantities can be proved to be gauge independent. The gauge dependence of the effective potential arises because the elementary fields are not invariant under gauge transformation. This suggests that one might obtain an explicitly gauge-independent result by defining an effective potential for a gauge-invariant composite operator[3]. We will examine this issue in this paper. To find the effective potential U (σ ) for a composite operator ϕ2 [4], one introduces a source coupled to this operator L → L − Jϕ2 . (1.1)
Summary

A Computational Model for Periodic Pattern Perception Based on Crystallographic GroupsPI:Yanxi LIU(yanxi@)Robotics Institute,Carnegie Mellon UniversityNSF research grant#IIS-0099597.Funding period:2001-2004URL:/afs//user/yanxi/www/symmetry.htmlSummaryThe objective of this project is to build a computational model based on crystallographic groups to perceive real-world near-periodic patterns.Symmetry or near-symmetry is a pervasive phenomena in both natural and man-made environments.A computational model for symmetry is particularly pertinent to robotics and computer vision research where we are studying how a man-made intelligent being can perceive and interact with the chaotic real world in the most effective manner.Being able to reason about symmetries is thefirst step towards minimizing redundancy and capturing the essential degrees of freedom of a problem,and can often lead to drastic reductions in computation.Our computational model for periodic pattern perception is based on the solution to Hilbert’s18th problem:there is afinite number of symmetry groups for all possible periodic patterns in each-dimensional space.When there are seven frieze groups,and when there are17wallpaper groups.Based on these mathematical proofs we are able to construct a computationally tractable framework to automatically classify all types of periodic patterns.Being able to tolerate departures from perfect symmetry reflects one’s level of sophistication in perception.To this end, we study the combination of group theory with statistical learning theory and geometric model selection in various applications where near-periodic patterns dominate(see projects below and Figure1). Symmetry Group Classification for Real World Near-periodic Patterns(Euclidean version)We develop a set of computer algorithms that“understand”a given periodic pattern by automaticallyfinding its underlying lattice,identifying its symmetry group and extracting its representative ing a novel idea of regions-of-dominance,defined as the largest circle centered on the candidate peak such that no higher peaks are contained in the circle.We are able to extract underlying lattices from given patterns with imper-fections caused by lighting and surface defects(bottom-right of Figure1).Two versions of symmetry group classification algorithms are implemented and tested.One is robust to patterns with moderate amount of pixel noise and outliers.A second group classification algorithm uses the idea of treating symmetry groups as a continuous feature such that a distance is defined for each near-periodic pattern to their corresponding regular patterns.A geometric AIC model selection algorithm is applied to give all symmetry groups a fair chance to be selected as the classified result.We have tested both algorithms on a large number of different types of patterns including man-made,bee-made periodic patterns and wallpaper,frieze spatiotemporal pat-terns obtained from human and animal gait videos.The computational model also includes a set of functions that are able to identify the representative motifs of a given periodic pattern by locating the highest order of rotational symmetry centers andfinding their orbits.These automatically selected motifs exhibit the same local symmetries within a single tile as the global pattern,and conform well with human perception. Potential Symmetry and Skewed Symmetry Groups(affine version)Under viewing angle variations(with an affine camera model),the symmetry group of a periodic pattern going through affine deformation varies.We introduce the term skewed symmetry groups and provide a complete theoretical treatment for2D wallpaper groups under affine transformations.For thefirst time, a given periodic pattern can be classified not simply by its Euclidean symmetry group but by its highest skewed symmetry group,which indicates the inherent symmetry potential of a pattern uniquely.In addition, a concise wallpaper group migration map(17by17)is constructed that separates the17affinely deformed wallpaper groups into small,distinct orbits.The practical value of this result includes a novel indexing and retrieval scheme for regular patterns,and a maximal-symmetry-based method for estimating shape and orientation from texture under unknown views(top-right of Figure1).1Spatiotemporal Gait Pattern Analysis using wallpaper and frieze groupsWe studied two spatiotemporal image representations of gait.Thefirst is a wallpaper pattern composed of the correlation scores between all pairs of video frames from a gait ing our symmetry group classification algorithm,the gait patterns of a running dog and a walking human are classified into different symmetry groups,and respectively.This result reinforces that human gait is more bilaterally symmetric than a dog’s running gait,and thus symmetry of the gait patterns is useful for subject distinction when shape cues are hard to extract.The second type of spatiotemporal representation of gaits is a frieze pattern that is formed by collapsing video sequences of body silhouette images into a set of snapshots along the time axis.Here we used frieze groups of the patterns and statistical pattern similarity analysis to determine the orientation of the human gaits,and carried out human identification of CMU ROBO dataset under the same view(top-left of Figure1).Near-regular Texture Synthesis by capturing departures from regularityMotivated by the low structuralfidelity for near-regular textures in current texture synthesis algorithms, we extended our computational model for repeated pattern perception to an alternative texture synthesis method for near-regular texture.We view such textures as statistical departures from regular patterns and argue that a thorough understanding of their structures in terms of their translation symmetries can enhance existing methods of texture synthesis.We demonstrate a faithful preservation of the regularity as well as the randomness in several near-regular texture synthesis results that the state of the art texture synthesis algorithms are having problems with(bottom-left Figure1).In addition to texture replacement,under the theme of deformable textures we are exploring the departures of a regular texture from its regularity along different axes including geometry,color,intensity and lighting conditions.References[1]Y.Liu and R.T.Collins.Skewed Symmetry Groups.In Proceedings of IEEE Computer Society Con-ference on Computer Vision and Pattern Recognition(CVPR’01),pages872,879,Kauai,HI,December 2001.IEEE Computer Society Press.(/pubs/pubsample applications3。
To transfer or not to transfer
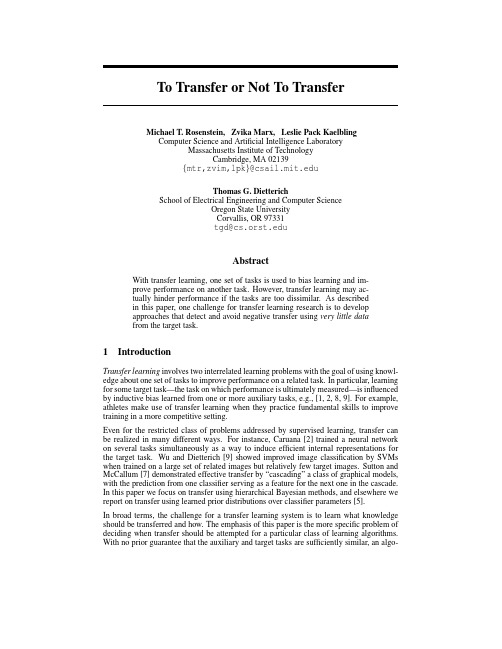
To Transfer or Not To TransferMichael T.Rosenstein,Zvika Marx,Leslie Pack KaelblingComputer Science and Artificial Intelligence LaboratoryMassachusetts Institute of TechnologyCambridge,MA02139{mtr,zvim,lpk}@Thomas G.DietterichSchool of Electrical Engineering and Computer ScienceOregon State UniversityCorvallis,OR97331tgd@AbstractWith transfer learning,one set of tasks is used to bias learning and im-prove performance on another task.However,transfer learning may ac-tually hinder performance if the tasks are too dissimilar.As describedin this paper,one challenge for transfer learning research is to developapproaches that detect and avoid negative transfer using very little datafrom the target task.1IntroductionTransfer learning involves two interrelated learning problems with the goal of using knowl-edge about one set of tasks to improve performance on a related task.In particular,learning for some target task—the task on which performance is ultimately measured—is influenced by inductive bias learned from one or more auxiliary tasks,e.g.,[1,2,8,9].For example, athletes make use of transfer learning when they practice fundamental skills to improve training in a more competitive setting.Even for the restricted class of problems addressed by supervised learning,transfer can be realized in many different ways.For instance,Caruana[2]trained a neural network on several tasks simultaneously as a way to induce efficient internal representations for the target task.Wu and Dietterich[9]showed improved image classification by SVMs when trained on a large set of related images but relatively few target images.Sutton and McCallum[7]demonstrated effective transfer by“cascading”a class of graphical models, with the prediction from one classifier serving as a feature for the next one in the cascade. In this paper we focus on transfer using hierarchical Bayesian methods,and elsewhere we report on transfer using learned prior distributions over classifier parameters[5].In broad terms,the challenge for a transfer learning system is to learn what knowledge should be transferred and how.The emphasis of this paper is the more specific problem of deciding when transfer should be attempted for a particular class of learning algorithms. With no prior guarantee that the auxiliary and target tasks are sufficiently similar,an algo-rithm must use the available data to guide transfer learning.We are particularly interested in the situation where an algorithm must detect,perhaps implicitly,that the inductive bias learned from the auxiliary tasks will actually hurt performance on the target task.In the next section,we describe a“transfer-aware”version of the naive Bayes classification algorithm.We then illustrate that the benefits of transfer learning depend,not surprisingly, on the similarity of the auxiliary and target tasks.The key challenge is to identify harmful transfer with very few training examples from the target task.With larger amounts of “target”data,the need for auxiliary training becomes diminished and transfer learning becomes unnecessary.2Hierarchical Naive BayesThe standard naive Bayes algorithm—which we callflat naive Bayes in this paper—has proven to be effective for learning classifiers in non-transfer settings[3].Theflat naive Bayes algorithm constructs a separate probabilistic model for each output class,under the “naive”assumption that each feature has an independent impact on the probability of the class.We chose naive Bayes not only for its effectiveness but also for its relative sim-plicity,which facilitates analysis of our hierarchical version of the algorithm.Hierarchical Bayesian models,in turn,are well suited for transfer learning because they effectively combine data from multiple sources,e.g.,[4].To simplify our presentation we assume that just two tasks,A and B,provide sources of data,although the methods extend easily to multiple A data sources.Theflat version of naive Bayes merges all the data without distinction,whereas the hierarchical version con-structs two ordinary naive Bayes models that are coupled together.LetθA i andθB i denote the i-th parameter in the two models.Transfer is achieved by encouragingθA i andθB i to have similar values during learning.This is implemented by assuming thatθA i andθB i are both drawn from a common hyperprior distribution,P i,that is designed to have unknown mean but small variance.Consequently,at the start of learning,the values ofθA i andθB i are unknown,but they are constrained to be similar.As with any Bayesian learning method,learning consists of computing posterior distribu-tions for all of the parameters in the two models,including the hyperprior parameters.The overall model can“decide”that two parameters are very similar(by decreasing the variance of the hyperprior)or that two other parameters are very different(by increasing the vari-ance of the hyperprior).To compute the posterior distributions,we developed an extension of the“slice sampling”method introduced by Neal[6].3ExperimentsWe tested the hierarchical naive Bayes algorithm on data from a meeting acceptance task. For this task,the goal is to learn to predict whether a person will accept an invitation to a meeting given information about(a)the current state of the person’s calendar,(b)the person’s roles and relationships to other people and projects in his or her world,and(c)a description of the meeting request including time,place,topic,importance,and expected duration.Twenty-one individuals participated in the experiment:eight from a military exercise and 13from an academic setting.Each individual supplied between99and400labeled ex-amples(3966total examples).Each example was represented as a15-dimensional feature vector that captured relational information about the inviter,the proposed meeting,and any conflicting meetings.The features were designed with the meeting acceptance task in mind but were not tailored to the algorithms studied.For each experiment,a single person was08162432Amount of Task B Training (# instances)T a s k B P e r f o r m a n c e (% c o r r e c t )Figure 1:Effects of B training set size on performance of the hierarchical naive Bayes al-gorithm for three cases:no transfer (“B-only”)and transfer between similar and dissimilar individuals.In each case,the same person served as the B data source.Filled circles de-note statistically significant differences (p <0.05)between the corresponding transfer and B-only conditions.chosen as the target (B )data source;100of his or her examples were set aside as a holdout test set,and from the remaining examples either 2,4,8,16,or 32were used for training.These training and test sets were disjoint and stratified by class.All of the examples from one or more other individuals served as the auxiliary (A )data source.Figure 1illustrates the performance of the hierarchical naive Bayes algorithm for a single B data source and two representative A data sources.Also shown is the performance for the standard algorithm that ignores the auxiliary data (denoted “B-only”in the figure).Transfer learning has a clear advantage over the B-only approach when the A and B data sources are similar,but the effect is reversed when A and B are too dissimilar.Figure 2a demonstrates that the hierarchical naive Bayes algorithm almost always performs at least as well as flat naive Bayes,which simply merges all the available data.Figure 2b shows the more interesting comparison between the hierarchical and B-only algorithms.The hierarchical algorithm performs well,although the large gray regions depict the many pairs of dissimilar individuals that lead to negative transfer.This effect diminishes—along with the positive transfer effect—as the amount of B training data increases.We also ob-served qualitatively similar results using a transfer-aware version of the logistic regression classification algorithm [5].4ConclusionsOur experiments with the meeting acceptance task demonstrate that transfer learning often helps,but can also hurt performance if the sources of data are too dissimilar.The hierar-chical naive Bayes algorithm was designed to avoid negative transfer,and indeed it does so quite well compared to the flat pared to the standard B-only approach,however,there is still room for improvement.As part of ongoing work we are exploring the use of clustering techniques,e.g.,[8],to represent more explicitly that some sources of data may be better candidates for transfer than others.Amount of Task B Training (#instances)F r a c t i o n o f P e r s o n P a i r sAmount of Task B Training (#instances)F r a c t i o n o f P e r s o n P a i r s(a)(b)Figure 2:Effects of B training set size on performance of the hierarchical naive Bayes al-gorithm versus (a)flat naive Bayes and (b)training with no auxiliary data.Shown are the fraction of tested A-B pairs with a statistically significant transfer effect (p <0.05).Black and gray respectively denote positive and negative transfer,and white indicates no statis-tically significant difference.Performance scores were quantified using the log odds of making the correct prediction.AcknowledgmentsThis material is based upon work supported by the Defense Advanced Research Projects Agency (DARPA),through the Department of the Interior,NBC,Acquisition Services Division,under Con-tract No.NBCHD030010.Any opinions,findings,and conclusions or recommendations expressed in this material are those of the authors and do not necessarily reflect the views of DARPA.References[1]J.Baxter.A model of inductive bias learning.Journal of Artificial Intelligence Research ,12:149–198,2000.[2]R.Caruana.Multitask learning.Machine Learning ,28(1):41–70,1997.[3]P.Domingos and M.Pazzani.On the optimality of the simple bayesian classifier under zero-one loss.Machine Learning ,29(2–3):103–130,1997.[4] A.Gelman,J.B.Carlin,H.S.Stern,and D.B.Rubin.Bayesian Data Analysis,Second Edition .Chapman and Hall/CRC,Boca Raton,FL,2004.[5]Z.Marx,M.T.Rosenstein,L.P.Kaelbling,and T.G.Dietterich.Transfer learning with an ensemble of background tasks.Submitted to this workshop.[6]R.Neal.Slice sampling.Annals of Statistics ,31(3):705–767,2003.[7] C.Sutton and position of conditional random fields for transfer learning.In Proceedings of the Human Language Technologies /Emprical Methods in Natural Language Processing Conference (HLT/EMNLP),2005.[8]S.Thrun and J.O’Sullivan.Discovering structure in multiple learning tasks:the TC algorithm.In L.Saitta,editor,Proceedings of the Thirteenth International Conference on Machine Learning ,pages 489–497.Morgan Kaufmann,1996.[9]P.Wu and T.G.Dietterich.Improving SVM accuracy by training on auxiliary data sources.In Proceedings of the Twenty-First International Conference on Machine Learning ,pages 871–878.Morgan Kaufmann,2004.。
my model的英语作文
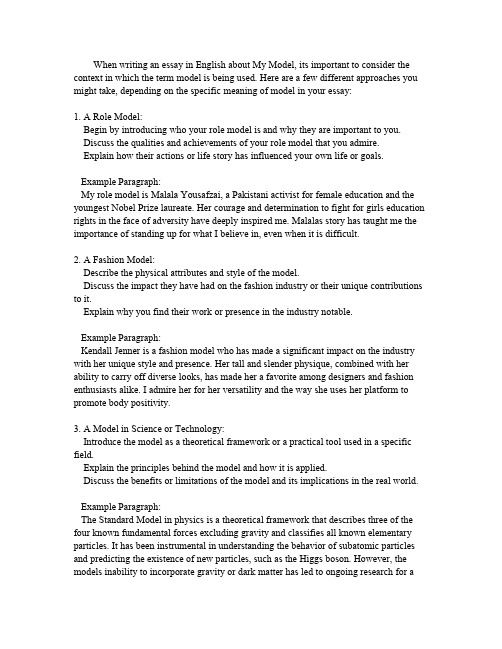
When writing an essay in English about My Model,its important to consider the context in which the term model is being used.Here are a few different approaches you might take,depending on the specific meaning of model in your essay:1.A Role Model:Begin by introducing who your role model is and why they are important to you. Discuss the qualities and achievements of your role model that you admire. Explain how their actions or life story has influenced your own life or goals.Example Paragraph:My role model is Malala Yousafzai,a Pakistani activist for female education and the youngest Nobel Prize laureate.Her courage and determination to fight for girls education rights in the face of adversity have deeply inspired me.Malalas story has taught me the importance of standing up for what I believe in,even when it is difficult.2.A Fashion Model:Describe the physical attributes and style of the model.Discuss the impact they have had on the fashion industry or their unique contributions to it.Explain why you find their work or presence in the industry notable.Example Paragraph:Kendall Jenner is a fashion model who has made a significant impact on the industry with her unique style and presence.Her tall and slender physique,combined with her ability to carry off diverse looks,has made her a favorite among designers and fashion enthusiasts alike.I admire her for her versatility and the way she uses her platform to promote body positivity.3.A Model in Science or Technology:Introduce the model as a theoretical framework or a practical tool used in a specific field.Explain the principles behind the model and how it is applied.Discuss the benefits or limitations of the model and its implications in the real world.Example Paragraph:The Standard Model in physics is a theoretical framework that describes three of the four known fundamental forces excluding gravity and classifies all known elementary particles.It has been instrumental in understanding the behavior of subatomic particles and predicting the existence of new particles,such as the Higgs boson.However,the models inability to incorporate gravity or dark matter has led to ongoing research for amore comprehensive theory.4.A Model in Business or Economics:Introduce the business or economic model and its purpose.Explain how the model works and the strategies it employs.Discuss the success or challenges associated with the model and its potential for future growth.Example Paragraph:The subscriptionbased business model has become increasingly popular in recent years, particularly in the software panies like Adobe have transitioned from selling packaged software to offering services on a subscription basis,allowing for continuous revenue streams and a more predictable income.This model has been successful in fostering customer loyalty and providing a steady income,although it requires ongoing innovation to maintain customer interest.5.A Model in Art or Design:Describe the aesthetic or functional qualities of the model.Discuss the creative process or design principles that inform the model.Explain the cultural or historical significance of the model and its influence on contemporary art or design.Example Paragraph:The Eames Lounge Chair,designed by Charles and Ray Eames,is a model of modern furniture that has become an icon of midcentury design.Its elegant form,made from molded plywood and leather,exemplifies the designers commitment to blending comfort with aesthetics.The chairs timeless appeal has made it a staple in both residential and commercial settings,influencing countless furniture designs that followed. Remember to structure your essay with a clear introduction,body paragraphs that develop your points,and a conclusion that summarizes your main e specific examples and evidence to support your claims,and ensure your writing is clear,concise, and engaging.。
ASDL Intro to XRF

The shells are labelled from the nucleus outward
K shell - 2 electrons L shell - 8 electrons M shell - 18 electrons N shell - 32 electrons
This work is licensed under the Creative Commons Attribution-ShareAlike 3.0 Unported License
WHAT IS XRF?
a. X-ray Fluorescence Spectrometry
a. An elemental analysis technique
THE ELECTROMAGNETIC SPECTRUM
How does light affect molecules and atoms?
D.C. Harris, Quantitative Chemical Analysis, 7th Ed., Freeman, NY, 2007.
X-RAY INTERACTIONS WITH MATTER
“OWNERSHIP” OF XRF WITHIN ACADEMIA
• Although XRF is a physical phenomena involving the interaction of X-rays with matter, most of the applications of XRF are in areas outside of physics (chemistry, environmental sciences, food and product quality monitoring, etc.) • Although XRF requires specialized knowledge in chemistry (spectral interpretation, calibration, sample prep, etc.), it is not even mentioned in 99% of undergraduate chemistry programs in the U.S. • These materials will hopefully encourage wider dissemination and use of XRF in undergraduate chemistry and biochemistry programs and demonstrate its potential as a means for teaching concepts such as spectroscopy, sampling, qualitative and quantitative analysis, and elemental composition in
- 1、下载文档前请自行甄别文档内容的完整性,平台不提供额外的编辑、内容补充、找答案等附加服务。
- 2、"仅部分预览"的文档,不可在线预览部分如存在完整性等问题,可反馈申请退款(可完整预览的文档不适用该条件!)。
- 3、如文档侵犯您的权益,请联系客服反馈,我们会尽快为您处理(人工客服工作时间:9:00-18:30)。
Abstract—This paper presents an analytic potential model for long-channel symmetric and asymmetric double-gate (DG) MOSFETs. The model is derived rigorously from the exact solution to Poisson’s and current continuity equation without the charge-sheet approximation. By preserving the proper physics, volume inversion in the subthreshold region is well accounted for in the model. The resulting analytic expressions of the drain–current, terminal charges, and capacitances for longchannel DG MOSFETs are continuous in all operation regions, i.e., linear, saturation, and subthreshold, making it suitable for compact modeling. As no fitting parameters are invoked throughout the derivation, the model is physical and predictive. All parameter formulas are validated by two-dimensional numerical simulations with excellent agreement. The model has been implemented in Simulation Program with Integrated Circuit Emphasis version 3 (SPICE3), and the feasibility is demonstrated by the transient analysis of sample CMOS circuits. Index Terms—Analytic potential model, compact model, double-gate (DG) MOSFET.
and above threshold regions [5], which will potentially cause convergence problems. Another model, although continuous and analytic, is based on the approximate solution to onedimensional (1-D) Poisson’s and current continuity equations and requires some fitting parameters [6]. In this paper, we rigorously solve Poisson’s equation to obtain analytic potential expression and, further, derive the implicit drain–current model based on current continuity equation. We then proceed to develop charge and capacitance models. This methodology is applied to the derivation of both symmetric DG (SDG) and asymmetric DG (ADG) MOSFETs. As the model involves implicit functions, iterations are required to solve the equations. It is shown that the results of the analytic model exhibit excellent agreement with two-dimensional (2-D) numerical simulation values, and yet, the expressions are continuous in all operation regions. Sections II and III are devoted to the development of SDG and ADG MOSFETs, respectively. Finally, the implementation in SPICE3 is discussed, and the transient analysis of sample circuit is demonstrated in Section IV.
IEEE TRANSACTIONS ON ELECTRON DEVICES, VOL. 53, NO. 5, MAY 2006
1161
An Analytic Potential Model for Symmetric and Asymmetric DG MOSFETs
Huaxin Lu, Student Member, IEEE, and Yuan Taur, Fellow, IEEE
C
II. ANALYTIC POTENTIAL MODEL FOR SDG MOSFETs A. Current and Transconductance Model for SDG MOSFETs In our previous paper [7], we proposed an analytic drain–current model for SDG MOSFETs. The model continuously covers all operation regions and is in excellent agreement with 2-D numerical simulation results without any ad hoc fitting parameters. These features enable the model to be an ideal kernel of SDG MOSFET modeling. In this section, we first briefly review the drain–current model. Then, starting from the drain–current expression, we further carry on the derivation to develop the intrinsic charge and capacitance models of SDG MOSFETs, which are required to perform transient analysis in circuit simulation. Consider an undoped (or lightly doped) SDG MOSFET shown schematically in Fig. 1. Poisson’s equation along a vertical cut perpendicular to the Si film (Fig. 2) takes the following form with only the mobile charge (electrons) term:
I. INTRODUCTION OMPACT models of MOSFETs have evolved from piecewise models to unified models due to the numerical divergence problems in circuit simulation caused by discontinuous derivatives of MOSFET models [1], [2]. For bulk MOSFETs, Pao-Sah’s double integral based on gradual-channel approximation (GCA), although valid in all operation regions, cannot be carried out analytically due to the presence of both depletion and mobile charges in the integral [3]. This necessitates the charge-sheet approximation that would lead to further simplification to obtain a piecewise explicit current expression. However, charge-sheet-based models cannot properly describe volume inversion, a unique characteristic of double-gate (DG) MOSFETs in the subthreshold region [4]. Contrary to bulk MOSFETs, depletion charges in DG MOSFETs are negligible because the silicon film is undoped (or lightly doped). Thus, only the mobile charge term needs to be included in Poisson’s equation. Therefore, the exact solutions to Poisson’s and current continuity equations based on GCA can be derived without the charge-sheet approximation. Several drain–current models for DG MOSFETs have been proposed previously. One model that allows two gates independently driven adopts a smooth function to join the subthreshold