美赛O奖经验分享
当我谈数学建模时我谈些什么——美赛一等奖经验总结
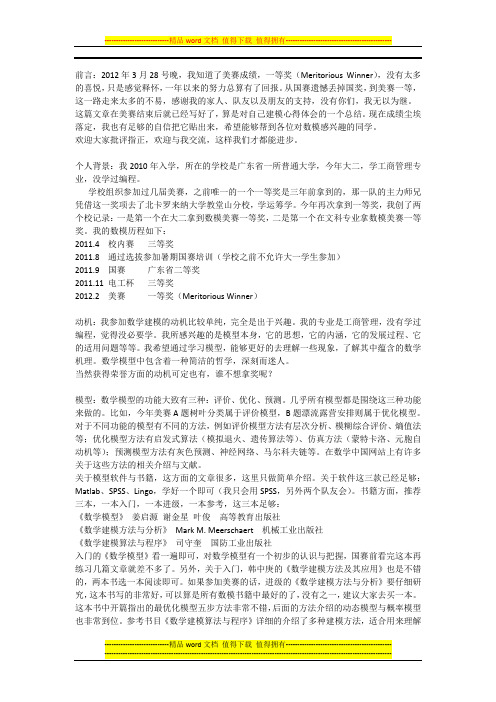
前言:2012年3月28号晚,我知道了美赛成绩,一等奖(Meritorious Winner),没有太多的喜悦,只是感觉释怀,一年以来的努力总算有了回报。
从国赛遗憾丢掉国奖,到美赛一等,这一路走来太多的不易,感谢我的家人、队友以及朋友的支持,没有你们,我无以为继。
这篇文章在美赛结束后就已经写好了,算是对自己建模心得体会的一个总结。
现在成绩尘埃落定,我也有足够的自信把它贴出来,希望能够帮到各位对数模感兴趣的同学。
欢迎大家批评指正,欢迎与我交流,这样我们才都能进步。
个人背景:我2010年入学,所在的学校是广东省一所普通大学,今年大二,学工商管理专业,没学过编程。
学校组织参加过几届美赛,之前唯一的一个一等奖是三年前拿到的,那一队的主力师兄凭借这一奖项去了北卡罗来纳大学教堂山分校,学运筹学。
今年再次拿到一等奖,我创了两个校记录:一是第一个在大二拿到数模美赛一等奖,二是第一个在文科专业拿数模美赛一等奖。
我的数模历程如下:2011.4 校内赛三等奖2011.8 通过选拔参加暑期国赛培训(学校之前不允许大一学生参加)2011.9 国赛广东省二等奖2011.11 电工杯三等奖2012.2 美赛一等奖(Meritorious Winner)动机:我参加数学建模的动机比较单纯,完全是出于兴趣。
我的专业是工商管理,没有学过编程,觉得没必要学。
我所感兴趣的是模型本身,它的思想,它的内涵,它的发展过程、它的适用问题等等。
我希望通过学习模型,能够更好的去理解一些现象,了解其中蕴含的数学机理。
数学模型中包含着一种简洁的哲学,深刻而迷人。
当然获得荣誉方面的动机可定也有,谁不想拿奖呢?模型:数学模型的功能大致有三种:评价、优化、预测。
几乎所有模型都是围绕这三种功能来做的。
比如,今年美赛A题树叶分类属于评价模型,B题漂流露营安排则属于优化模型。
对于不同功能的模型有不同的方法,例如评价模型方法有层次分析、模糊综合评价、熵值法等;优化模型方法有启发式算法(模拟退火、遗传算法等)、仿真方法(蒙特卡洛、元胞自动机等);预测模型方法有灰色预测、神经网络、马尔科夫链等。
历年美赛题目解法
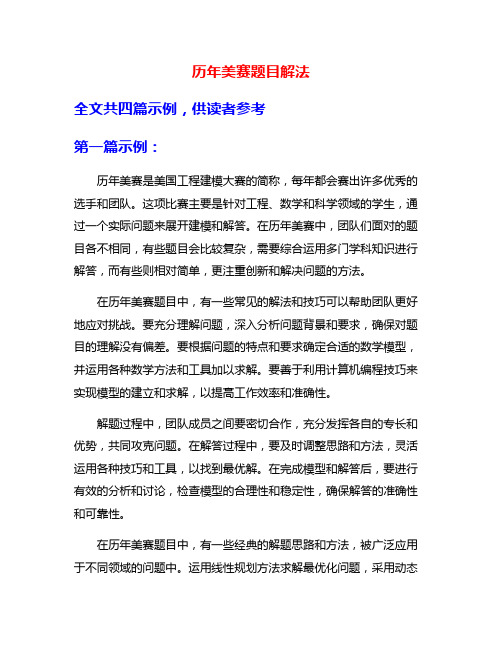
历年美赛题目解法全文共四篇示例,供读者参考第一篇示例:历年美赛是美国工程建模大赛的简称,每年都会赛出许多优秀的选手和团队。
这项比赛主要是针对工程、数学和科学领域的学生,通过一个实际问题来展开建模和解答。
在历年美赛中,团队们面对的题目各不相同,有些题目会比较复杂,需要综合运用多门学科知识进行解答,而有些则相对简单,更注重创新和解决问题的方法。
在历年美赛题目中,有一些常见的解法和技巧可以帮助团队更好地应对挑战。
要充分理解问题,深入分析问题背景和要求,确保对题目的理解没有偏差。
要根据问题的特点和要求确定合适的数学模型,并运用各种数学方法和工具加以求解。
要善于利用计算机编程技巧来实现模型的建立和求解,以提高工作效率和准确性。
解题过程中,团队成员之间要密切合作,充分发挥各自的专长和优势,共同攻克问题。
在解答过程中,要及时调整思路和方法,灵活运用各种技巧和工具,以找到最优解。
在完成模型和解答后,要进行有效的分析和讨论,检查模型的合理性和稳定性,确保解答的准确性和可靠性。
在历年美赛题目中,有一些经典的解题思路和方法,被广泛应用于不同领域的问题中。
运用线性规划方法求解最优化问题,采用动态规划算法处理序列型问题,利用离散事件模拟技术模拟系统行为,通过随机过程分析系统性能等。
团队在解答问题时,可以参考这些经典方法,并根据实际情况进行创新和调整,以获得更好的结果。
在参加历年美赛的过程中,团队可以积累丰富的经验和知识,不断提高解题能力和创新意识。
通过与其他团队的交流和比赛,也能够拓展视野,学习他人的优秀经验和做法。
在解题过程中,要保持耐心和坚持,不断克服困难和挑战,直至最终获得满意的解答。
在历年美赛题目解法中,关键的是全面理解问题,切实分析和建立数学模型,灵活应用各种方法和技巧,团队配合紧密,有效沟通和讨论,并不断实践和改进。
通过不断练习和磨炼,团队可以在历年美赛中取得优异的成绩,展现出自己的才华和实力。
希望各位参赛者能够在历年美赛中不断进步,取得更好的成绩,展现出自己的独特魅力和价值。
美赛Outstanding Winner作者经验分享
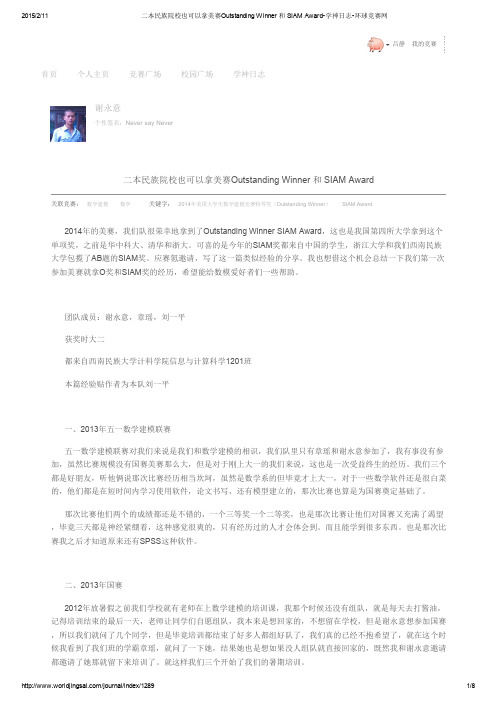
首页个人主页竞赛广场校园广场学神日志谢永意个性签名:Never say Never吕静我的竞赛二本民族院校也可以拿美赛Outstanding Winner 和 SIAM Award关联竞赛: 数学建模 数学 关键字: 2014年美国大学生数学建模竞赛特等奖(Outstanding Winner ) SIAM Award2014年的美赛,我们队很荣幸地拿到了Outstanding Winner SIAM Award ,这也是我国第四所大学拿到这个单项奖,之前是华中科大、清华和浙大。
可喜的是今年的SIAM 奖都来自中国的学生,浙江大学和我们西南民族大学包揽了AB 题的SIAM 奖。
应赛氪邀请,写了这一篇类似经验的分享。
我也想借这个机会总结一下我们第一次参加美赛就拿O 奖和SIAM 奖的经历,希望能给数模爱好者们一些帮助。
团队成员:谢永意,章瑶,刘一平获奖时大二都来自西南民族大学计科学院信息与计算科学1201班本篇经验贴作者为本队刘一平一、2013年五一数学建模联赛五一数学建模联赛对我们来说是我们和数学建模的相识,我们队里只有章瑶和谢永意参加了,我有事没有参加,虽然比赛规模没有国赛美赛那么大,但是对于刚上大一的我们来说,这也是一次受益终生的经历。
我们三个都是好朋友,听他俩说那次比赛经历相当坎坷,虽然是数学系的但毕竟才上大一,对于一些数学软件还是很白菜的,他们都是在短时间内学习使用软件,论文书写,还有模型建立的,那次比赛也算是为国赛奠定基础了。
那次比赛他们两个的成绩都还是不错的,一个三等奖一个二等奖,也是那次比赛让他们对国赛又充满了渴望,毕竟三天都是神经紧绷着,这种感觉很爽的,只有经历过的人才会体会到。
而且能学到很多东西。
也是那次比赛我之后才知道原来还有SPSS 这种软件。
二、2013年国赛2012年放暑假之前我们学校就有老师在上数学建模的培训课,我那个时候还没有组队,就是每天去打酱油,记得培训结束的最后一天,老师让同学们自愿组队,我本来是想回家的,不想留在学校,但是谢永意想参加国赛,所以我们就问了几个同学,但是毕竟培训都结束了好多人都组好队了,我们真的已经不抱希望了,就在这个时候我看到了我们班的学霸章瑶,就问了一下她,结果她也是想如果没人组队就直接回家的,既然我和谢永意邀请都邀请了她那就留下来培训了。
2011美赛C题O奖
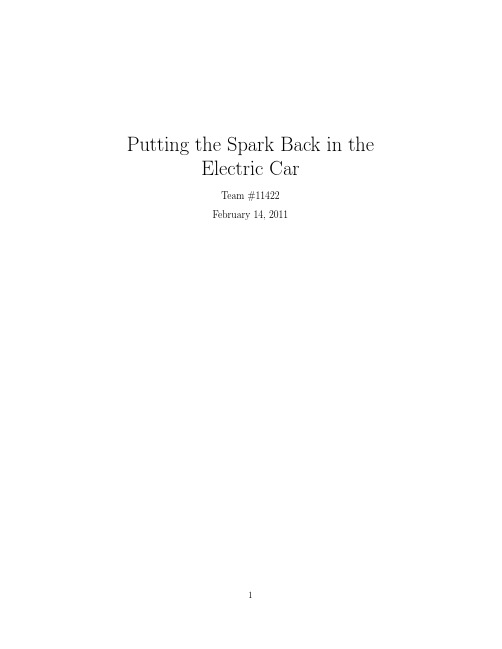
Putting the Spark Back in theElectric CarTeam#11422February14,20111Team#11422Page2of20Contents1Clarification of Problem3 2Plan of Attack3 3Assumptions3 4On Types of Cars4 5Model for Number of Cars4 6Microeconomic Model56.1Global Influence Model (5)6.1.1Strengths&Weaknesses (7)6.2Localized Behavior (8)6.2.1Cellular automata (8)6.3Strengths and Weaknesses (9)7Macroeconomic Model:Meeting the Energy Demand107.1Current Energy Source and Demand (10)7.2Current Pollution rates (11)7.3Quantizing Pollution (11)7.3.1Health (12)7.4Quantizing Cost (12)7.5αParameter (13)7.6Minimizing X:Genetic and Nelder-Mead Methods (14)7.7Using Alpha to Determine Cost,and Vice Versa (14)7.8Example Calculation (16)7.9Fossil Fuels Saved (17)7.10Strengths and Weaknesses (17)8Meshing the Micro and Macro Models18 9Conclusion19Team#11422Page3of201Clarification of ProblemWith the recent introduction of the Nissan Leaf and Chevy Volt to the world carfleet and the fading supply of petroleum,the possibility of electric vehicles replacing standard petroleum cars is increasing.Questions arise concerning the feasibility of such vehicles,specifically regarding the amount of fossil fuels saved through widespread use of electric cars,and the economic feasibility.It is of great concern to auto-manufacturers and environmentalists alike to determine how to cause electric cars to’catch on,’and of equally great concern to govern-ments to determine how to augment the power grid to meet the demand of the electric carfleet.The models proposed within this paper will offer an insight to these problems.2Plan of AttackOur objective is to model the effects of electric vehicles on the environment, public health,and economy.We need to determine which methods would be most effective in causing widespread use of electric vehicles,within a reasonable time rge scale use of electric vehicles would also put an increased strain on the power grid,which would have to be corrected.To determine the most efficient way to do this,we will proceed as follows:1.Create a model for the amount of electric cars at any given point in time.(Micro)2.Create a model that gives a single value to the effect electric cars have onthe environment,health,and economy.(Macro)3.Connect these models so that,by giving setup conditions,we can deter-mine the cost to minimize the pollution values.3AssumptionsDue to the extrapolative nature of our model,and the difficulty in obtaining reliable global information,several assumptions were made in order to complete our model.These simplifying assumptions will be used throughout the paper and could feasibly be replaced with reliable data when it becomes available.•The cost of building more coal,oil,and natural gas plants is negligible to the cost of yearly fossil fuel production.That is to say,energy costs simply rely on production prices from the plants themselves,and not creation of the plants.•We assume100%efficiency of converting fossil fuels to energy for electric-ity.This makes the calculations for energy easier,removing the need to know the electric energy conversion rate for electric generators.•World Governments can control addition of power plants to determine the proportions of energy from each source.This is essential in changing theTeam#11422Page4of20 makeup of the power grid.By being able to change the ratio of the energy sources of our electricity production can we can change the ratio of the pollutants produced for each unit of electrical energy.•Ratios of energy sources into demand sectors for the US in2009is the same as the ratios across the world.This allowed us to generalize the information that we had to the world-wide energy system.•Price increases quadratically as demand increases for fossil fuels.This allows us to extrapolate the past data,allowing us to produce a prediction of the cost of fossil fuels in the future.•Population within the next50years can be modeled with a cubicfit.This allows us to extrapolate the past data as well,ensuring that we know the amount of cars in a given year.•A major factor in choosing which car to buy is what the people around you own.The movie”Who Killed the Electric Car?”suggested that the main reason that electric cars did not become popular was because many people did not know about them or their properties.This assumption is the basis in the models for the spread of electric cars throughout a population.[9]•It is economically and environmentally infeasible to increase current en-ergy contribution to the electric power grid for each power source by more than%25percent.This is to establish upper bounds for the Nelder-Mead methods,and can be replaced with projected maximum contribution for 2060if/when these values become available.4On Types of CarsWe have decided to base our model solely on electric vehicles versus gasoline vehicles,instead of including hybrid vehicles.We have chosen to do this because we are concerned with the widespread usage of electric vehicles.If electric vehicle usage is widespread,then the idea of a hybrid car is useless,since electric cars can be used for most transportation usages and gas cars can be used for any transportation that electric cars cannot do.Hybrid models were created to transition from gas cars to electric cars.However,if we are to consider widespread usage of electric vehicles,hybrids won’t be necessary.It is worth noting that electric vehicles do have limited range,causing some range anxiety. Modern estimates suggest that90%of automobile users do not have needs that exceed the limitations of electric cars,however,so the range anxiety will only affect10%of the population[9].5Model for Number of CarsIn order to model the change towards electric cars and its impact on the environment,we need a model for the number of cars in the future.We found an estimated134motor vehicles per1000people in the top130developed countries. From this,an estimate of120vehicles per1000people in the world can be made.Team#11422Page5of20 Using this and population data,we can expect to have C cars in t years after 1950based on the following equation[10]:C(t)=.002t(2.55·109+3.91·107t+1.1·106t2−9.38·103t3)We decided upon a cubicfit to model the population because itfitted popu-lation data very well.However,thisfit will only accurately model population data until2060,due to the cubic nature of the function.We have a multiplier of .002t because the number of cars per capita will increase over time,as data has shown[1].We are going to let E equal the proportion of cars which are electric. The following2equations give the number of electric and gasoline vehicles over time in terms of E.E(t)=E·C(t)G(t)=(1−E)·C(t)In our microeconomic model,we examine how E will change50years in the future based on an initial proportion of electric cars.This will allow us to see what must be done to make electric vehicle usage widespread.For our macroeconomic model,we let E=.9since we wish to examine the effects of widespread electric vehicle usage.6Microeconomic ModelThrough examination of how individuals react to electric car usage we can model the change from petroleum vehicles to electric vehicles.Our small-scale model needs to be based on the likelihood that individuals will switch to electric vehicles.We propose two models.Both of these models require a government subsidy for electric cars in order to”jumpstart”their production,and explosion in popularity.Thefirst model is based on coupled differential equations for how one might expect the number of electric vehicles and the number of gasoline vehicles will change over a continuous time interval.The second model is a 2D cellular automata simulation to model the local influence as well as global influence of the number of electric vehicles,over a discrete time interval.6.1Global Influence ModelThis model assumes that individuals are influenced by the global proportion of people who have electric cars.An individual who is going to buy a new car or replace a broken down gas car will buy an electric car with a probability equal to the proportion of people who have electric cars.This is because the more people who have electric cars,the more likely an individual is to hear about electric cars and be persuaded to switch to an electric car.This allows us to define the following coupled differential equations where BDE and BDG is the probability that an electric and gas car will break down during one year.Since gas cars last for about8years and electric cars last for about20years,we letBDE=120and BDG=18[4].E (t)=E(t)E(t)+G(t)·(C (t)+BDG·G(t))−BDE·E(t)·1−E(t)E(t)+G(t)Team#11422Page6of20G (t)=1−E(t)E(t)+G(t)·(C (t)+BDE·E(t))−BDG·G(t)·E(t)E(t)+G(t)Since our C(t)and is only valid forfifty years in the future,solving these equations outright is unnecessary.We use Euler’s Method to approximate E(t) and G(t).To do this,we need two points,one for E(t)and one for G(t).Since t is measured in years after1950,we let G(60)=C(60).We cannot let E(60)=0 since the only way for the number of gas cars to grow is from the probabilityE(t) C(t).This model requires that a certain number of electric cars be seeded intothe population to jump start the growth of electric cars.In order to seed these cars,the government could pay the difference in cost between an electric vehicle and an average gas car to give people an incentive to buy an electric car.By spending this money to encourage people to use electric cars,the government would save money later by spending less money for fossil fuels,such as oil.We will examine how this works after we have built our macroeconomic model.To determine the seeding cost,we assume that the government will pay the differ-ence between the cost of an electric vehicle and a gasoline vehicle.We decided this cost per car would be$41,000−$28,400=$12,600.The following graphs demonstrate the rate at which the proportion of electric vehicles grows(with seeding values of.05and.3)and the following table summarizes this data with varying proportions of seeding in2011.Team#11422Page7of20Seed Proportion Seeding Cost E in20600.011.0327·10110.2670.055.16348·10110.6670.11.0327·10120.8150.151.54854·10120.8770.22.06514·10120.9120.252.58174·10120.9330.33.09834·10120.948The more money spent on jumpstarting electric vehicles,the larger E will be50years in the future.In order to determine which proportion of seeded cars would be most profitable in the future,we would need to know the make up of the power grid,which we determine in our macroeconomic model.We will connect this model to the macroeconomic model later.6.1.1Strengths&WeaknessesA strength of this model is that it allows the government to see what would need to be done in order for people to want to buy electric cars.By basing this model on the proportion of people who have electric cars,this model can realistically model an individual’s likelihood of switching to an electric car.A weakness in this model is that seeding only occurs in one year,instead of a range of years.Another weakness of this model is that it does not include locality,which misses out on what seems to be a crucial point in the rise of electric vehicles.Another weakness of this model is it does not consider current sources of energy.Currently,electric cars are not better for the environment because the largest source of electrical energy is coal;this will be considered and changed in the macroeconomic model.Team#11422Page8of206.2Localized BehaviorThe previous model assumes that the total percentage of electric cars influences the chance of a single person purchasing one.However,a person is affected by the people closest to them in addition to the global behavior.This is why we decided to model the spread of electric cars using2-dimensional cellular automata.First,we decided that a cell’s percentage to pick either electric or gas is based on the8cells that are adjacent,known as the Moore Neighborhood. The influence from locality is converted into a chance of buying an electric car based on the number of your neighbors who are electric cars(N)according to the following equation:P(if electric stay electric)=18·(.1N+.1)P(if gas become electric)=120·(.1N+.1)Global influence is also considered with this model,and is incorporated with what we call the”snowball constant.”The differential equations given above can be applied to cellular automata rules by setting C (t)=0,since the number ofcells is constant,which allows us to substitute E(t)E(t)+G(t)with E p t,the proportionof electric vehicles.Ultimately we can rewrite our differential equations as:E pn+1(t)−E pn(t)=3E p(t)40·(1−E p(t))G pn+1(t)−G pn(t)=3E p(t)40·(E p(t)−1)To consider both the local and globalized behavior(L and W),we can simply weight these with the relative importance of localized behavior(due to the snowball effect)with that of global behavior.Exact values of snowball constants will have to be determined through real world observations,and will likely vary throughout the population.For our data,we used a snowball constant k=4, assuming that localized behavior is responsible for80%of buying patterns.Our final proportion looks like this:P=k·L+W 1+kThe amount of electric cars that are placed initially is changed in order to model the seeding program that the government has put in place.The output of the model gives the percentage of electric cars out of the total population of cars.This percentage can be traced from year to year to give you the effect of governmental electric car seeding,both in thefinal percent as well as the year when government seeding no longer plays a role in the percentage of cars.6.2.1Cellular automataInitial seeding is important as there is no point to seeding more cars if fewer cars will get you to your goal percentage of electric cars on the road by a certain year.By using both the global and the local model,we determined that thefinal percentage of cars that are electric,for a given number ofTeam#11422Page9of20 seeding,is less in the local model than in the global model,meaning that these local interactions seem to slow down the distribution of cars.Thefinal state of one simulation and a chart of the proportion of electric vehicles versus timeare shown below:6.3Strengths and WeaknessesThe benefits of a cellular automata model are many:this model differs from all others in this report int that there is no population increase,which means that this model is independent of theflawed population model,and is free from allflaws that come with that.This means that this model can more accurately model years after1960.Modifications can be made to increase the chances of buying an electric car as time goes on,due to improvements in technology and the decrease in electric car cost.Furthermore,the effects of localized behav-ior are well documented,and completely overlooked with differential equation models.The effects of these localized behavior can be combined with the differ-ential equation model with the snowball constant–an option unavailable to theTeam#11422Page10of20 differential equation model.This localized model is not without it’s weakness. Because of thefinite number of cells,it is difficult to incorporate growth of the population(of total cars)into the CA model.Much of our values for proba-bilities rely on rough probabilities and assumptions of the snowball constant. These can be adjusted on a product-by-product,or even a cell-by-cell basis,but it complicates the model greatly.We also could have overlooked crucial values in our probability models percentages needed to factor into the lifetime cost of a car,relative usability values like the average range an electric car can go without recharging,and more qualitative values like sticker shock.7Macroeconomic Model:Meeting the Energy DemandWhereas our microeconomic model focused on the necessary parameters to en-sure a large number of electric cars in the future,our macroeconomic model focuses on the changes that need to be made to accommodate the increased demand of electricity.It is important to consider both the costs required to produce these new amounts of electric energy,and the”hidden”costs of pollu-tion.Without considering these”hidden”costs,our model would simply gener-ate the cheapest solution to the increased power demand,which could possibly just trade one fossil fuel(gasoline)for another(coal,etc.).Thus,we’ve gener-ated an equation to determine the cost associated with the increased electricity demand,depending upon how much of each energy source we utilize,and a variable parameter equating pollution to cost.7.1Current Energy Source and DemandData from the EIA has shown that38.075quadrillion BTU was used for elec-tricity in2009,producing11.159trillion kWh[7].The breakdown of energy sources contributing to this statistic is summarized in the following chart.Since it is assumed that this breakdown is roughly equal for all highly-developed coun-tries,the countries who will have the largest number of electric cars,and thus increased demand for electricity.[7]Team#11422Page11of20 The most recent electric cars from Li-ion Motors Corp have a range of120 miles and require8hours of charging from a110V source[4].This means that 52.8kWh are needed for a full charge,or.44kWh per mile of travel.This translates to1501.3BTU needed a mile in an electric vehicle.The average pas-senger car can travel31miles on a gallon of gasoline.Since a gallon of gasoline contains roughly116,090BTU,the average gas car runs on3744.84BTU per mile[1].This is more than twice as much energy required by electric cars,so switching to electric cars decreases the amount of energy required worldwide for transportation purposes,but also requires a switch from the100%gasoline power source for gas cars,to the medley of power sources used for electricity.7.2Current Pollution ratesBurning fossil fuels creates pollutants that damage the environment,increasing acid rain,respiratory illness,and photochemical ing current energy source quantities,the pounds per mile of use of electric and gas cars is sum-marized in the following table.Though nuclear power sources have not gained widespread popularity due to social fears of nuclear accidents and the relative cost of creating a network of reactors,their carbon footprints are negligible.It also goes without saying that the footprint of renewable energy sources are also negligible.Pollutant Electric Car Gasoline Car Difference Carbon Dioxide0.1839693020.614153760.430184458Carbon Monoxide0.0001611950.00012358−3.76149·10−5Nitrogen Oxides0.0003609130.0016776880.001316776Sulfur Dioxide0.0018842520.004201710.002317459Particulates0.0019805450.000314567-0.001665978Mercury1.16351·10−82.62139·10−81.45788·10−8 From our equation of the number of cars in terms of years since1950,C(t),and the proportion of those which are electric E,we create the following equations for the pounds of pollutant reduced each year.Pollutant Pounds SavedCarbon Dioxide0.430·12,200·E·C(t)Carbon Monoxide−3.76·10−5·12,200·E·C(t)Nitrogen Oxides0.00132·12,200·E·C(t)Sulfur Dioxide0.00232·12,200·E·C(t)Particulates-0.00167·12,200·E·C(t)Mercury1.46·10−8·12,200·E·C(t)7.3Quantizing PollutionThe pollution value is a metric that determines how good for the environment having a certain percentage of electric cars are.Thefirst value that determines this metric is the amount of pollution that is being saved in pounds per year. The second value is the percent pollutant decrease,which describes how muchTeam#11422Page12of20 control of that pollutant is had.For example,if you could either cut50%of the total carbon monoxide emissions or25%of the total carbon dioxide emis-sions,that50%decrease is weighted heavier,regardless of the actual pounds of emissions you are eliminating.A third,hypothetical,value would be the bad-ness of each pollutant.Since not all pollutants damage the environment and peoples health as much as others,this badness relates to the degree to which the current yearly amounts of pollutants are damaging the environment.In the trials we ran,we assumed that the total yearly emissions of every pollutant were equally bad,so each badness value was set at1.Given the set of pollutants, {CO2,CO,NO x,SO2,P articulate},where the subscripts,G,E,and T corre-spond to the amount emitted from gas cars,the amount saved by electric cars, and total emissions,respectively,our pollution can be defined as follows:P ollution=pollutantsjj G+E·j Ej T7.3.1HealthIn examining the effects of pollution,we should also consider the effects on health.This is incorporated in our Pollution function because if we can only change a small percent of the quantity of a pollutant,it will have a smaller effect on health,whereas if we can change a larger percent of the quantity of a pollutant,it will have a larger effect on health.However,some pollutants may be more damaging to the environment than others,meaning that eliminating 50%of one pollutant would not be equivalent to eliminating50%of another. By analyzing data concerning the effects of the pollutants on health and the environment,a badness factor could be determined by which each pollution percentage change could be multiplied with.By minimizing X,which is a func-tion for cost and pollution,we will also be minimizing the effects.However in this model,we assumed that the badness factor,or the relative damage each pollutant causes to the environment and health,of each pollutant is the same.7.4Quantizing CostOnline sources can be used to estimate the small-scale cost of each BTU of each power source,in addition to the current production in the US[3].Bereft of data of the maximum production limits of each power source,it can be assumed that it would be economically infeasible to increase the current production limits for each power source to electricity by a factor greater than25%.This can be modified if more accurate statistics were obtained.Since widespread use of electric cars will require a major revamping of the power grid,demand will rise dramatically,potentially with no increase in supply.The prices of commodities increase with their scarcity,as seen by supply and demand curves.Again lacking data of supply and demand curves for power sources,we’ll be forced to make several assumptions.Given our maximum production limits,m,and our current production limits(defined to be0here)and prices,i,we can define the price of a commoditiy to be ten times it’s current cost when we reach maximum production.We’ll also define the price to be2.5times current cost when weTeam#11422Page13of20 are halfway between current and maximum production ing these data points,we can set up a quadraticfit to model the price p(L)of one quadrillion BTU’s of a particular energy source per quadrillion BTU(L)more than current production as:p(L)=i−3i·Lm+12i·L2mto determine the total cost to increase production from current values to pro-duction l,we can simply integrate from0to l:P tot(l)=l0P(l)dl=l−3i·l22m+4i·l3m2So total cost for all power sources is equivalent to:Cost=P ower Sourcesjl j−3i j·l2j2m j+4i j·l3jm2jThe current production,cost,and maximum values are shown in the following table,where all productions are in QBTU,and cost in dollars per QBTU[3].Power Source Current Production Max Production Current Cost Petroleum0.383.4793.63∗1010 Natural Gas 6.8948.61751.47∗1010 Coal18.38422.988.7∗109 Renewable Energy 4.213 5.2662.2∗1010 Nuclear Energy8.42610.53255.9∗109 7.5αParameterWith cost and pollution both quantized,we can define an objective function asX=Cost+α·P ollutionWhere the number of electric cars,and hence their energy demand,is held constant.X is dependent on the number of quadrillion BTU’s we add to each power source and the alpha value,because cost is dependent on the power sources,and pollution is dependent on both power sources andα.Since wewant to minimize both cost and pollution,our goal is to minimize X.Theαvalue simply serves as a constant defining how much the government values costto environment.For example,ifα=0,damage to the environment is not takeninto effect and minimizing X is simply minimizing Cost.In and of itself,αis a relative value,as the relationship between it and pollution is very messy (again,dependent on all power sources).However,given a maximum amountof allowable pollutants,anαcan be determined.Possible values forαand their meaning will be discussed further in this report.Team#11422Page14of207.6Minimizing X:Genetic and Nelder-Mead Methods With X being a function of six variables(five power sources,and alpha),there are several methods that we can use search for global minimum,subject to the constraints that each power source is never to decrease from current pro-duction standards(under the assumption that removal of production facilities is both costly and creates largescale unemployment),and is never to exceed previously defined maximum production standards.However,the nonlinear of nature eliminates the possibility of linear algebra techniques.Instead,we’ll rely heavily upon a Nelder-Mead iterative search technique and a genetic algorithm to define global minima.Though both of our techniques warrant equivalent so-lutions,we found that the Nelder-Mead search was much more computationally efficient,so the genetic methods were ruled out.Thus,we run a minimiza-tion of X=Cost+α·P ollution subject to the following constraints,with variables{P etr,Nat,Coal,Ren,Nuc}defining the amount of qBTU’s added to the power grid for petroleum,natural gas,coal,renewable energy and nuclear power,respectively:Minimize X=Cost+α·P ollution Subject toP etr+Nat+Coal+Ren+Nuc≤T otal Energy DemandCurrent P roduction≤P etr≤Current P roduction·1.25With thefinal constraint repeated for all power sources.7.7Using Alpha to Determine Cost,and Vice Versa Sinceαsimply refers to the amount to which we care about the environment, something that is difficult to assign a concrete value to,we’ve allowed forαto vary.By iterating the Nelder-Mead optimization for a range ofαvalues,we can generate plots of each of the Power sources versus alpha.In plainspeak,that is to say that by choosing someαvalue(e.g.,we care x much about damage to the environment),we can locate the values of each power source qBTU by simply reading the graph.Since Cost is simply a function of the power sources and is monotonically increasing withα,we can generate a graph showing cost versus alpha,by simply repeating the above procedure,and then calculating the cost from the values of each power source,plotting this to a particular alpha value. Shown below are graphs of’cost Vs.α’and’Power Sources Vs.α’with90%of the motorfleet being electric cars,50years from today:Team#11422Page15of20These graphs allow us to offer some insight into the behavior of the relation-ship betweenα(how much we care about the environment)and how we should augment the power grid.As is intuitive,a high reliance on coal and natural gas are necessary withα=0.Nuclear power seems constantly limited by our maxi-mum production value,suggesting that nuclear power,if production levels could be raised high enough,could be utilized in generating a low-cost,low-footprint power grid.Also intuitive is the monotonic behavior of the cost vsαgraph. The piecewise behavior is likely a result of certain’feasible pockets’within the polytope scanned with the Nelder-Mead method.。
参加美国大学生数学建模竞赛 - 知乎
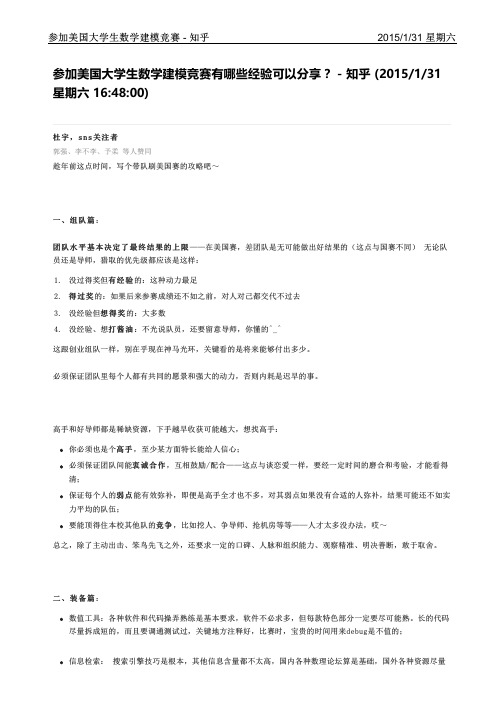
5.制胜点
纵观整篇论文,没完成的模型(论文引用)不少,编程总共就两个,一个人口增长,一个层次分析。但是我们有条 理的解决了这个问题,并且排版相当美观,图表制作的非常好看。
我觉得美赛并不是考你有多少数学知识,或者编程多么牛逼,或者队员背景多么生猛。而是你们是否能够好好合 作,理清解决问题的思路,并有条理的写出来。你不一定要数学好编程好,也不需要有庞大的知识储备,而是要会 尽可能的找到你要的资料和数据,尽可能全面的思考问题,在可选范围内,挑你能做好的去做。网上资料那么多, 只是看你会不会发掘利用。
比赛报名:提前准备好visa或master card,名字和地址不要写错;
作息:要看各队情况了,原则是保证效率、不打乱节奏。前期都很亢奋,但如果打乱节奏可能导致后面疲劳期 时效率过低,其实美国赛截止时间并不是很严格,前期利用好亢奋期和每天的高效率时间的话,到了疲劳期还 能继续坚持下去,否则就是给你再多时间都无法持续下去。对那些想尝试达芬奇睡眠法的同学,建议先在之前 比赛和练习时充分适应,避免临时改变作息方式,打乱节奏,降低效率; 引用:如果copy了整段的原始论文,一定要注明来源——07年就出过outstanding奖因为引用的问题被收回的 事。这是原则问题,千万注意! 邮寄论文:提前联系邮局/快递,确认好邮局每天邮寄时间,以倒推截止时间,事实上这么多时间,很少有人 能用满——这给了慢热队伍一个优势,之前练习也应先关注深度和质量,再考虑速度和效率;之前比赛的时 候,交完论文的几天别闲着,继续魔鬼训练——对做到极致的模型再完善深化,对论文结论再推广演绎,甚至
必须保证团队里每个人都有共同的愿景和强大的动力,否则内耗是迟早的事。
高手和好导师都是稀缺资源,下手越早收获可能越大,想找高手:
你必须也是个高手 ,至少某方面特长能给人信心; 必须保证团队间能衷诚合作 ,互相鼓励/配合——这点与谈恋爱一样,要经一定时间的磨合和考验,才能看得 清; 保证每个人的弱点 能有效弥补,即便是高手全才也不多,对其弱点如果没有合适的人弥补,结果可能还不如实 力平均的队伍; 要能顶得住本校其他队的竞争 ,比如挖人、争导师、抢机房等等——人才太多没办法,哎~
数学建模美赛须知
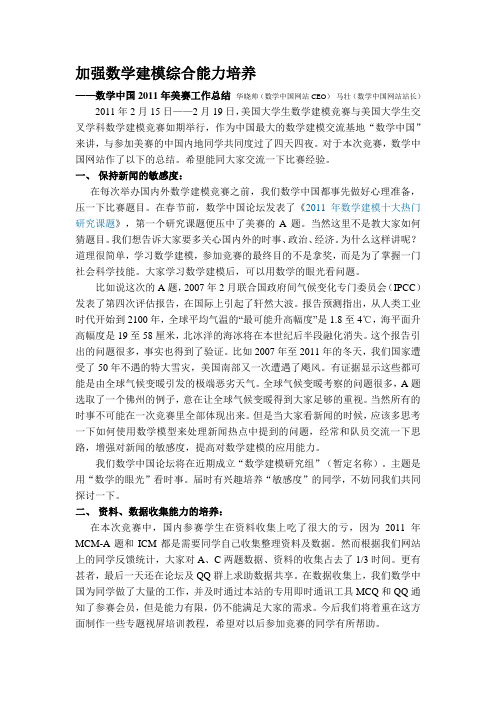
加强数学建模综合能力培养——数学中国2011年美赛工作总结华晓帅(数学中国网站CEO)马壮(数学中国网站站长)2011年2月15日——2月19日,美国大学生数学建模竞赛与美国大学生交叉学科数学建模竞赛如期举行,作为中国最大的数学建模交流基地“数学中国”来讲,与参加美赛的中国内地同学共同度过了四天四夜。
对于本次竞赛,数学中国网站作了以下的总结。
希望能同大家交流一下比赛经验。
一、保持新闻的敏感度:在每次举办国内外数学建模竞赛之前,我们数学中国都事先做好心理准备,压一下比赛题目。
在春节前,数学中国论坛发表了《2011年数学建模十大热门研究课题》,第一个研究课题便压中了美赛的A题。
当然这里不是教大家如何猜题目。
我们想告诉大家要多关心国内外的时事、政治、经济。
为什么这样讲呢?道理很简单,学习数学建模,参加竞赛的最终目的不是拿奖,而是为了掌握一门社会科学技能。
大家学习数学建模后,可以用数学的眼光看问题。
比如说这次的A题,2007年2月联合国政府间气候变化专门委员会(IPCC)发表了第四次评估报告,在国际上引起了轩然大波。
报告预测指出,从人类工业时代开始到2100年,全球平均气温的“最可能升高幅度”是1.8至4℃,海平面升高幅度是19至58厘米,北冰洋的海冰将在本世纪后半段融化消失。
这个报告引出的问题很多,事实也得到了验证。
比如2007年至2011年的冬天,我们国家遭受了50年不遇的特大雪灾,美国南部又一次遭遇了飓风。
有证据显示这些都可能是由全球气候变暖引发的极端恶劣天气。
全球气候变暖考察的问题很多,A题选取了一个佛州的例子,意在让全球气候变暖得到大家足够的重视。
当然所有的时事不可能在一次竞赛里全部体现出来。
但是当大家看新闻的时候,应该多思考一下如何使用数学模型来处理新闻热点中提到的问题,经常和队员交流一下思路,增强对新闻的敏感度,提高对数学建模的应用能力。
我们数学中国论坛将在近期成立“数学建模研究组”(暂定名称)。
美赛写作材料模板及参赛经验分享
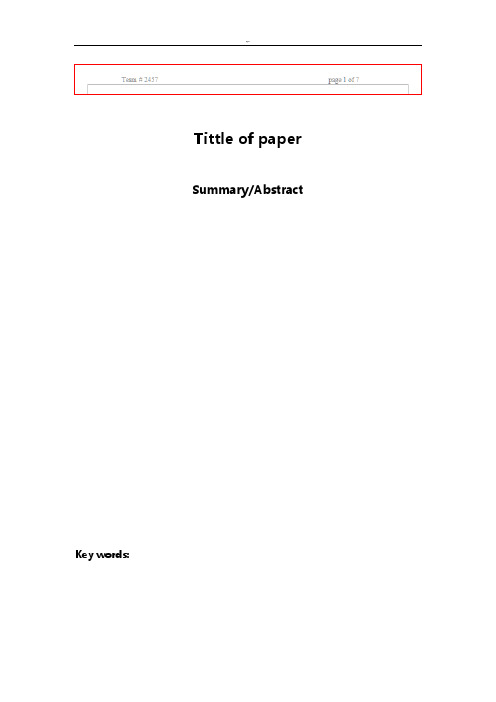
Tittle of paperSummary/Abstract Key words:I.Introduction(引言)Organ transplantation is a preferable treatment for the most serious forms of end-stage diseases. In recent years, advances in medical science and technology have made solid organ transplantation an increasingly successful and common medical procedure, a literal ''second chance at life". Not only does it offer the best hope for complete rehabilitation, but it has also proved to be the most cost-effective of all treatment options, including dialysis. Consequently, more and more people are benefiting from organ transplants and their survival rates are steadily improving. The surgical techniques involved have been mastered for half a century and are now considered as routine. The two main sources of kidneys for transplantation are deceased-donor kidneys and live-donations from family and friends. However, unfortunately, there is a considerable shortage of donor organs, compared to demands. As a matter of fact, efficient matching and allocation of organs donated has become an exigent problem.The United Network for Organ Sharing (UNOS), as the operator of the Organ Procurement and Transplantation Network (OPTN), is responsible for transplant organ distribution in the United States. UNOS oversees the allocation of many different types of transplants, including liver, kidney, pancreas, heart, lung, and cornea.Focusing on kidney transplantation, based on UNOS Kidney Allocation Model, we develop a mathematical model for US transplant networks. First, incomingorgans are matched with waiting candidates by medical institutions considering the factors as ABO blood compatibility, the degree of recipient major HLA mismatch in order to obtain a matching degree applied on the allocation part. After that, from the patients’perspective, on the basis of linear regression, priority weight is defined by pondering age, disease severity, time on waiting, PRA level, and region. Applying this mechanism of ranking, we realize MWBM (Maximum Weight Bipartite-graph Matching) and SMGS (Stable Matching based on Gale-Shapley algorithm). MWBM focuses on the optimal assignment of donors following the algorithm of bipartite-graph maximum weight matching; SMGS emphasizes the process of exchanges in order to obtain the stable exchanges between donors and candidates on the waiting list.II.The Description of Problem(问题重述)III.Basic Assumptions●The level of mismatch is only relative to the number of antigens.●The data and information are accurately registered according to the medicalmeasures●The data and information are refreshed in time according to the status of thepatients●No differences in the quality of the donor kidneys●The quality of the donor kidney is constantIV.Definitions and Notations●Kidney transplantation: A kidney transplant is a surgical procedure to implant ahealthy kidney into a patient with kidney failure.●Prioritization●MD: Matching Degree●PW: Prioritization weight●MWSM: Maximum Weight Bipartite Matching●SMGS: Stable Matching based on Gale-Shapley algorithm或V.ModelsThrough the investigation of US transplantation network, we draw a general picture of the mechanism. With reference to some resources available on the website of UNOS, a flow chart (Figure 1) is developed showing the procedure of the network.Currently, the initial waiting list is composed of patients who are waiting for a kidney or combined kidney-pancreas transplant. For the first time, the patients arerequested to show the correct and scientific information to the US kidney transplant network which is needed for donor-recipient matching, the ranking of patients on the waiting list, and determining the outcome of those transplanted. The patients’waiting lists are composed of initial patients, historical patients and unsuccessful recipient after transplantation. Historical patients refer to registered patients whose status have changed and have an influence on the procedure. A patient is taken off the waiting list when a graft is offered and accepted by that patient or the patient is dead while waiting for a transplant. Unsuccessful recipients refer to the patients who have a bad result of transplantation calling for transplantation again, as it is so-called relistFigure 1. A schematic depicting the steps occurring in the transplantation networks......Table 1.Survival rate involving HLA mismatchVI.Conclusions.Our model for the optimal allocation of the donor organs is established by three modules, procurement of MD and PW, optimal assignment by MWBM model and Stable Matching of Gale-Shapley algorithm. The model has offered a convincing procedure of the allocation with the ……VII.Strengths and weaknesses(模型优缺点)Strengths●……Weaknesses●VIII.References注意文献的积累,不要等到文章写完再去重新寻找文献。
数学建模美赛经验
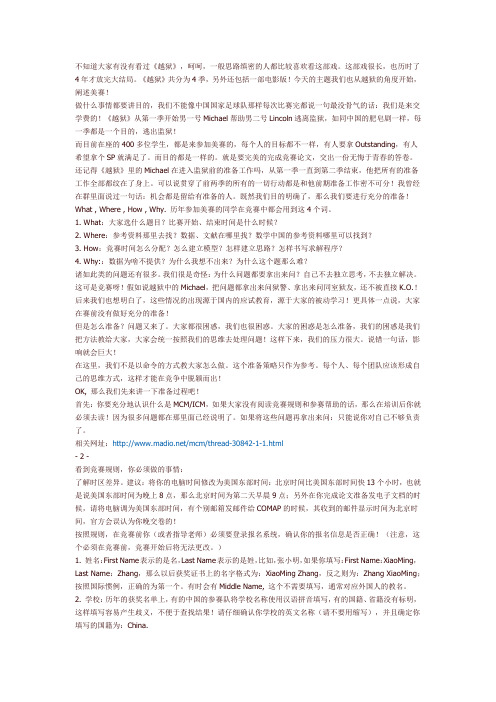
不知道大家有没有看过《越狱》,呵呵,一般思路缜密的人都比较喜欢看这部戏。
这部戏很长,也历时了4年才放完大结局。
《越狱》共分为4季,另外还包括一部电影版!今天的主题我们也从越狱的角度开始,阐述美赛!做什么事情都要讲目的,我们不能像中国国家足球队那样每次比赛完都说一句最没骨气的话:我们是来交学费的!《越狱》从第一季开始男一号Michael帮助男二号Lincoln逃离监狱,如同中国的肥皂剧一样,每一季都是一个目的,逃出监狱!而目前在座的400多位学生,都是来参加美赛的,每个人的目标都不一样,有人要拿Outstanding,有人希望拿个SP就满足了。
而目的都是一样的。
就是要完美的完成竞赛论文,交出一份无悔于青春的答卷。
还记得《越狱》里的Michael在进入监狱前的准备工作吗,从第一季一直到第二季结束,他把所有的准备工作全部都纹在了身上。
可以说贯穿了前两季的所有的一切行动都是和他前期准备工作密不可分!我曾经在群里面说过一句话:机会都是留给有准备的人。
既然我们目的明确了,那么我们要进行充分的准备!What , Where , How , Why. 历年参加美赛的同学在竞赛中都会用到这4个词。
1. What:大家选什么题目?比赛开始、结束时间是什么时候?2. Where:参考资料那里去找?数据、文献在哪里找?数学中国的参考资料哪里可以找到?3. How:竞赛时间怎么分配?怎么建立模型?怎样建立思路?怎样书写求解程序?4. Why::数据为啥不提供?为什么我想不出来?为什么这个题那么难?诸如此类的问题还有很多。
我们很是奇怪:为什么问题都要拿出来问?自己不去独立思考,不去独立解决。
这可是竞赛呀!假如说越狱中的Michael,把问题都拿出来问狱警、拿出来问同室狱友,还不被直接K.O.!后来我们也想明白了,这些情况的出现源于国内的应试教育,源于大家的被动学习!更具体一点说,大家在赛前没有做好充分的准备!但是怎么准备?问题又来了。
- 1、下载文档前请自行甄别文档内容的完整性,平台不提供额外的编辑、内容补充、找答案等附加服务。
- 2、"仅部分预览"的文档,不可在线预览部分如存在完整性等问题,可反馈申请退款(可完整预览的文档不适用该条件!)。
- 3、如文档侵犯您的权益,请联系客服反馈,我们会尽快为您处理(人工客服工作时间:9:00-18:30)。
美赛O奖经验分享
主讲人:熊风华中科技大学电信11级
我与美赛
熊风华中科技大学电子信息与通信学院1102班
参加过两次美赛
2013年美赛(ICM) C题一等奖(Meritorious)
2014年美赛(MCM) B题特等奖(Outstanding)
(B题唯一一篇入选官方杂志umap的论文)比赛负责的部分:建模、算法、编程
准备和大家分享的内容1.APMCM B题评讲
2.个人对美赛的理解
3.对最后11天准备美赛的一些建议
4.Q & A
B 题:如何评价微信公众号微信是腾讯公司推出的是一款跨平
台的通讯工具。
微信公众平
台,是用户利用公众账号进行自媒体活动,简单来说就是进行一对多的媒体性的行为活动,如商家通过申请公众微信服务号通过二次开发,如对接微信会员云营销系统展示商家微官网、微会员、微推送、微支付、微活动,微报名、微分享、微名片等,已经形成了一种主流的线上线下微信互动营销方式。
值得注意的是, 微信每天允许公号向受众群发1 条消息, 而只有极少数公号会每天都把这一次群发用掉。
大多数公号都是基于自身话题领域,根据自己的内容定位,或推送他们认为值得受众关注的重要新闻, 或推送受众可能更容易感兴趣的趣味性内容。
微信公众平台包括不同领域的各种账号,分析这些平台运营是否有效,是否能够在微时代更好地运用自己成为了各大企业思考的问题。
请建立数学模型完成以下问题:
1、查找相关资料分析微信公众帐号的领域,建立模型,预测不同领域的公众帐号数量的增长趋势。
2、请建立数学模型分析不同领域的微信帐号运营是否有效,说明你的数学模型的优缺点。
3、假如给你一个“数学建模”的微信帐号,你该如何运营,给出方案并预测关注量增长趋势。
问题分析
1. 问题一:预测问题预测常用的模型:灰色预测、
时间序列、回归与拟合
2. 问题二:评价问题评价常用的模型:模糊综合评价、
层次分析法(AHP)、
3. 问题三:发散+ 预测
言之成理即可
大家用到的模型基本都是比较合适的,但普通都存在一些问题
举个例子:
摘要没有实
际的内容
一篇好的摘要要讲清楚的东西:
(1)建立了什么模型
(2)用了什么算法来求解
(3)得到了什么结果/结论(最好有数字)
(4)对模型进行了什么分析(比如敏感性分析),得到了什么结论
注意:
(1)摘要里最好不要出现公式
(2)摘要里不要有语法错误
(3)摘要尽量简洁
我的观点:摘要实在实在是太重要了!
(1)在哪里都可以马虎,但在摘要的地方不能马虎!!!(2)一篇摘要不好的文章,最多只有SP(三等奖)!
(3)一篇摘要好的文章,最少也有H(二等奖)
(4)对摘要的态度一定要非常非常重视。
(我们队每次写摘要都是三个人一起,会花接近两个小时)
问题二:模型分析普遍做得不够好
模型分析是我们队的杀手锏,并且我也认为模型分析这个部分最能够区分新手和老手。
一篇完整的论文:模型建立、模型求解、模型结果、模型分析
前三个部分大家往往都比较重视,但模型分析这个部分却被很多参赛者所忽视。
新手的思路:当求解好结果之后,长吁一口气,感觉大功已告成。
老手的思路:得到结果后,不会松懈,会继续分析模型,力求做得更深。
问题二:模型分析普遍做得不够好
大家的论文在模型分析这一块出现的问题
大部分人:干脆没有模型分析这个部分
少部分人:做了模型分析这个部分,但只是定性,不是定量
极少部分人:定量地做了模型分析这个部分,但不够深入给大家的建议:在正式比赛中,一定要做定量地去做模型分析;一定要在这一
块画几个图。
(即使什么思路也没有,至少你们可以做敏感性分析)
为什么模型分析这么重要?
(1)能够拉开差距对于有些题目,模型建立和模型求解的方法相对固定,大家都是这样,难以拉开差距。
而模型分析部分则可以各显神通
(2)能够做出深度即使是最基本、最常见的敏感性分析,只要能够从多个角度去做,也能够做出深度。
(3)容易展现亮点这一块往往会得到很多效果不错的图,能够得出一些很有意思的结论。
如何拿到Successful Participants(三等奖)?
成功提交论文即可。
2014年数据:
MCM(A、B题)6755支队伍,15支unsuccessful participants
ICM(C题)1028支队伍,0支unsuccessful participants 从概率上来看,unsuccessful participants比O奖还稀有。
如何拿到Honorable Mentions(二等奖)?
摘要+ 排版即可。
美赛二等奖及其以上的比
例占40%-50%
第一轮筛选一般只看摘要,摘要不过关就会被淘汰到SP那一档
,只要过关就至少是H
评委在这一轮不会读你的正文,最多扫几眼。
因此对于正文来说,排版好很重要。
如何拿到Meritorious Winners(一等奖)?
论文完整,没有硬伤(建模、求解、结果、分析)
有个别亮点(比如图表做得漂亮,用了很漂亮的算法等等)
2014年数据:
MCM(A、B题)6755支队伍,656支M (9%)
ICM(C题)1028支队伍,131支M(13%)
M的比例大概在10%左右,有一定难度,但大多数人都是可以争取的。
如何拿到Finalist 或者Outstanding ?
(1)论文完整,没有硬伤,细节处也处理得较好(2)有较大的亮点,有多个亮点
(3)运气比较好
2014年数据:
MCM(A、B题)6755支队伍,12支F,13
支O ICM(C题)1028支队伍,5支F,6支O
不管是F还是O,每道题都只有个位数的获奖者。
Finalist和Outstanding的区别?
经过层层选拔之后,MCM最后会剩下20多篇论文。
其中大约
一半会被评为Outstanding(特等奖),剩下的则是Finalist (特等奖入围奖)。
个人观点:到最后阶段的论文都非常优秀,这时候比谁更厉害主要就是看亮点以及运气(例如评委的口味等等)。
如何获更高的奖?
我的心得:不要逃避麻烦的问题,考虑得更全面更有深度。
新手都习惯于逃避麻烦的问题:
(1)比如数据不好找,就随便找几个简单的数据,甚至都不标明来源。
(2)比如在建模时,有些因素不好考虑就完全放弃
(3)比如觉得模型分析做起来太麻烦,就随便简单地做一下………… 所有你怠慢的地方,竞争对手都可能在那些地方做出亮点。
不要怕麻烦,精益求精,追求完美!
最后11天,我们可以干啥?
我认为必须做的事情:
(1)如果到现在还没做过美赛模拟赛的同学,抽4天时间做一次完整的模拟赛。
模拟赛要点:
一定要当作正式比赛来做。
题目最好选择以前的真题,但注意比赛期间不要查看当年的获奖论文,查资料时也不要找那一年之后的论文。
赛后要仔细分析自己队伍的缺陷与不足;与当年O奖论文对照,看看自己的论文与获奖论文的区别。
(2)准备好论文的模版。
论文排版、一些图表的制作
最后11天,我们可以干啥?
我认为必须做的事情
(3)基本的编程(matlab)
至少要会用matlab做基本的数据处理
至少要会用matlab画各种图
(4)基本的英语表达能力
✓一些常见模型、算法的英文翻译、一些常用的句式✓至少要能保证写好摘要
(5)精读几篇以前的优秀论文,认真揣摩
最后11天,我们可以干啥?
我觉得可以做的事情:
(1)再熟悉一遍常见的模型和算法,准备好相应的求解程序(2)熟悉可能用到的软件工具
(3)准备更详细的英语表达方法
原则:能在赛前做好的工作就不要拖到比赛中,比赛中的时间是很宝贵的!
特别推荐
我讲的这些都只是老生常谈,更详细具体的经验请参见校苑数模上的精华帖。
我的美赛特等奖论文:
/thread-
9542-1-1.html;
我的经验分享《两年数模路》:
/thread-9550-1-1.html。
特别推荐赵松学长的《美赛如何获奖》
/thread-10962-1-6.html。
Q & A
谢谢!。