Dynamically Constructed Bayesian Networks for Modeling Interests and Knowledge
浙江大学研究生《人工智能引论》课件
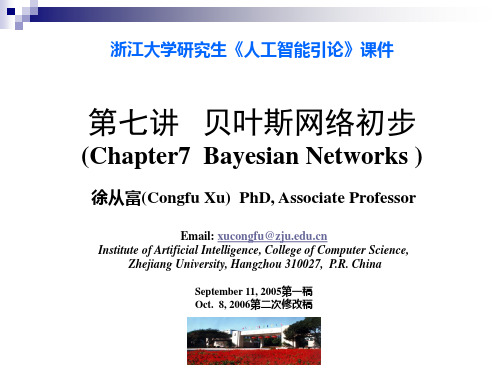
因此,P(B|j, m) = α <0.00059224, 0.0014919> ≈ <0.284, 0.716> 即在John和Mary都打电话的条件下,出现盗贼的 概率约为28%。
【课后习题1】
国家政策 (C) )
P(C) 0.50
C
P(U) 0.95 0.01 U P(B) 0.30 0.01
D. 独立和条件独立
Cavity Weather
Toothache
Catch
Weather和其它3个变量相互独立 给定Cavity后,Toothache和Catch条件独立
E. 贝叶斯网络示例
Burglary
P(B) 0.001 P(E)
Earthquake
B E t f t f P(A) 0.95 0.94 0.29 0.001
P(~b) 0.999 P(e) 0.002 P(a|~b,e) 0.29
P(~e)
+
0.998 P(a|~b,~e) 0.001 P(j|a) 0.90 P(m|a) 0.70 P(j|~a) 0.05 P(m|~a) 0.01
+
+
P(~a|~b,e) 0.71 P(j|~a) 0.05 P(m|~a) 0.01
B. 贝叶斯网络的定义
是一个有向无环图(DAG) 随机变量集组成网络节点,变量可离散或连续 一个连接节点对的有向边或箭头集合 每 节 点 Xi 都 有 一 个 条 件 概 率 分 布 表 : P(Xi|Parents(Xi)),量化其父节点对该节点的影响
C. 贝叶斯网络的别名
信念网(Belief Network) 概率网络(Probability Network) 因果网络(Causal Network) 知识图(Knowledge Map) 图模型(Graphical Model)或概率图模型(PGM) 决策网络(Decision Network) 影响图(Influence Diagram)
基于贝叶斯Noisy-or Gate网络对潜艇航行隐蔽性评估
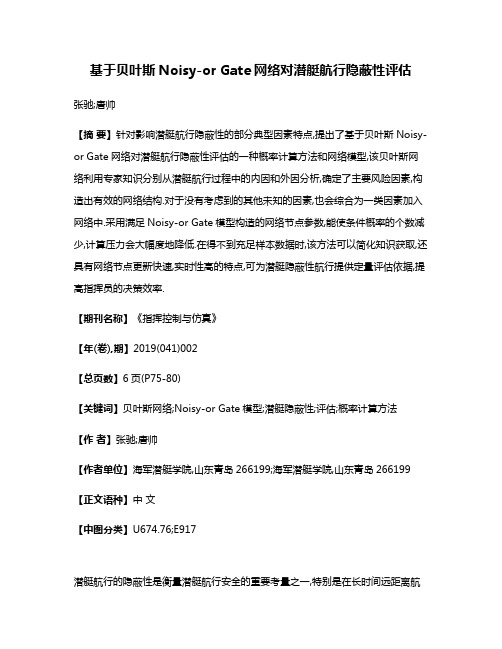
基于贝叶斯Noisy-or Gate网络对潜艇航行隐蔽性评估张驰;唐帅【摘要】针对影响潜艇航行隐蔽性的部分典型因素特点,提出了基于贝叶斯Noisy-or Gate网络对潜艇航行隐蔽性评估的一种概率计算方法和网络模型,该贝叶斯网络利用专家知识分别从潜艇航行过程中的内因和外因分析,确定了主要风险因素,构造出有效的网络结构.对于没有考虑到的其他未知的因素,也会综合为一类因素加入网络中.采用满足Noisy-or Gate模型构造的网络节点参数,能使条件概率的个数减少,计算压力会大幅度地降低.在得不到充足样本数据时,该方法可以简化知识获取,还具有网络节点更新快速,实时性高的特点,可为潜艇隐蔽性航行提供定量评估依据,提高指挥员的决策效率.【期刊名称】《指挥控制与仿真》【年(卷),期】2019(041)002【总页数】6页(P75-80)【关键词】贝叶斯网络;Noisy-or Gate模型;潜艇隐蔽性;评估;概率计算方法【作者】张驰;唐帅【作者单位】海军潜艇学院,山东青岛266199;海军潜艇学院,山东青岛266199【正文语种】中文【中图分类】U674.76;E917潜艇航行的隐蔽性是衡量潜艇航行安全的重要考量之一,特别是在长时间远距离航行的情况下,关键环节较多,需要考虑的因素也很多,有多种因素决定着潜艇是否隐蔽安全。
指挥员通常是根据本艇态势,搜集的情报信息加上个人经验去推测潜艇航行时的安全程度,但是没有相关辅助手段去定量支撑指挥员的推断。
根据周围因素判断潜艇隐蔽性评估用到的信息是在有限、不完整的信息背景下进行的,贝叶斯网络具有在不确定推理问题中进行学习和推理的能力,可以很好地将先验知识和多源数据融合进行推理。
贝叶斯网络的快速推理功能,已成为人工智能中解决不确定性推理的重要研究方向,也成为不同行业中数据分析、故障诊断、目标识别的一种有效工具[1]。
Pearl将贝叶斯推理进行网络化表达以来,提出的一些算法对不确定性问题进行很好的解决。
基于动态贝叶斯网络的社交圈归属匹配模型

第2 8卷 第 l 2期
20 0 8年 1 2月
文 章 编号 :0 1— 0 1 2 0 )2—30 0 10 9 8 (0 8 1 12— 3
计 算机 应 用
C mp trAp l ain o u e pi t s c o
V0 . 1 28 No. 2 1 De .2 0 c 08
t, col Eet n sadI omai e 1Sho l r i n n r t n co c f o U i rt S ag a 08 4 hn nv sy h nh i 10 C in ei % 2
2 N t n l n i r ga dTcnl yCn rfHg . ai a E g i n e o g et i o  ̄e n h o eo h
m 。c C m u n ,TnjB ac,S ag a 2 10 ,C i ) n o p t g og rn i i h h nh i 0 8g h a n
Ab ta t n od r1 ep an w cin n出esca ew r n igteb s thn iceo ca o sr c:I re h l e l t o e i o il tokf dn e t n i h mae igcrl fs ilcmmu iainfr o nc t o o
动态信任关系建模和管理技术研究

49.Gerck E Toward Real-World Models of Trust 1998
20.Chen R.W.Yeager Poblano:A Distributed Trust Model for Peer-to-Peer Networks.Sun Microsystems 2000
21.Winsborough W H.N Li Towards Practical Automated Trust Negotiation 2002
11.A.Abdul-Rahman.S.Halles A distributed trust model 1997
12.A.Abdul-Rahman.S.Hailes Using recommendations for managing trust in distributed systems 1997
34.F.Cornelli.E.Damiani.S.C.Vimercati.S.Paraboschi,P.Samarati A reputation-based approach for choosing reliable resources in peer-to-peer networks 2002
●由于PeerTrust和SecDWTrust都采用了社群信任查询的方法,因此在可信节点占大多数的情况下可以避免虚假的推荐,在~定程度上减轻了联合作弊的危害。而DwTrust 仪依赖直接经验进行判断,在缺乏赢接交互历史的情况下,无法识别联合欺骗行为。
意图识别与语义槽填充的双向关联模型
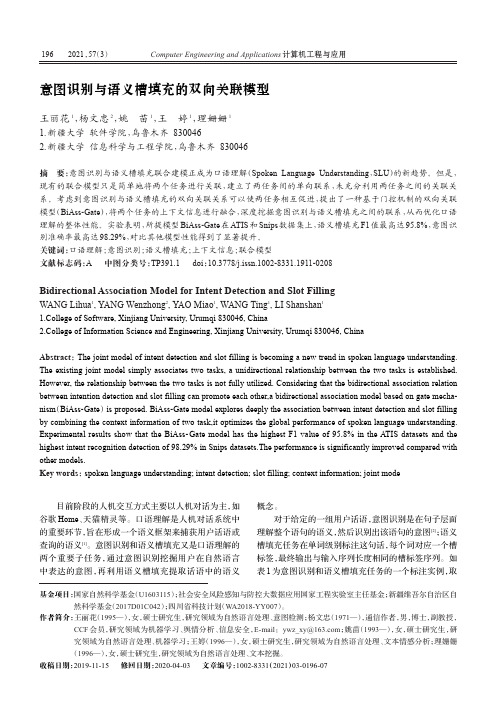
目前阶段的人机交互方式主要以人机对话为主,如谷歌Home、天猫精灵等。
口语理解是人机对话系统中的重要环节,旨在形成一个语义框架来捕获用户话语或查询的语义[1]。
意图识别和语义槽填充又是口语理解的两个重要子任务,通过意图识别挖掘用户在自然语言中表达的意图,再利用语义槽填充提取话语中的语义概念。
对于给定的一组用户话语,意图识别是在句子层面理解整个语句的语义,然后识别出该语句的意图[2];语义槽填充任务在单词级别标注这句话,每个词对应一个槽标签,最终输出与输入序列长度相同的槽标签序列。
如表1为意图识别和语义槽填充任务的一个标注实例,取意图识别与语义槽填充的双向关联模型王丽花1,杨文忠2,姚苗1,王婷1,理姗姗11.新疆大学软件学院,乌鲁木齐8300462.新疆大学信息科学与工程学院,乌鲁木齐830046摘要:意图识别与语义槽填充联合建模正成为口语理解(Spoken Language Understanding,SLU)的新趋势。
但是,现有的联合模型只是简单地将两个任务进行关联,建立了两任务间的单向联系,未充分利用两任务之间的关联关系。
考虑到意图识别与语义槽填充的双向关联关系可以使两任务相互促进,提出了一种基于门控机制的双向关联模型(BiAss-Gate),将两个任务的上下文信息进行融合,深度挖掘意图识别与语义槽填充之间的联系,从而优化口语理解的整体性能。
实验表明,所提模型BiAss-Gate在ATIS和Snips数据集上,语义槽填充F1值最高达95.8%,意图识别准确率最高达98.29%,对比其他模型性能得到了显著提升。
关键词:口语理解;意图识别;语义槽填充;上下文信息;联合模型文献标志码:A中图分类号:TP391.1doi:10.3778/j.issn.1002-8331.1911-0208Bidirectional Association Model for Intent Detection and Slot FillingWANG Lihua1,YANG Wenzhong2,YAO Miao1,WANG Ting1,LI Shanshan11.College of Software,Xinjiang University,Urumqi830046,China2.College of Information Science and Engineering,Xinjiang University,Urumqi830046,ChinaAbstract:The joint model of intent detection and slot filling is becoming a new trend in spoken language understanding. The existing joint model simply associates two tasks,a unidirectional relationship between the two tasks is established. However,the relationship between the two tasks is not fully utilized.Considering that the bidirectional association relation between intention detection and slot filling can promote each other,a bidirectional association model based on gate mecha-nism(BiAss-Gate)is proposed.BiAss-Gate model explores deeply the association between intent detection and slot filling by combining the context information of two task,it optimizes the global performance of spoken language understanding. Experimental results show that the BiAss-Gate model has the highest F1value of95.8%in the ATIS datasets and the highest intent recognition detection of98.29%in Snips datasets.The performance is significantly improved compared with other models.Key words:spoken language understanding;intent detection;slot filling;context information;joint mode基金项目:国家自然科学基金(U1603115);社会安全风险感知与防控大数据应用国家工程实验室主任基金;新疆维吾尔自治区自然科学基金(2017D01C042);四川省科技计划(WA2018-YY007)。
参数自适应调整的稀疏贝叶斯重构算法
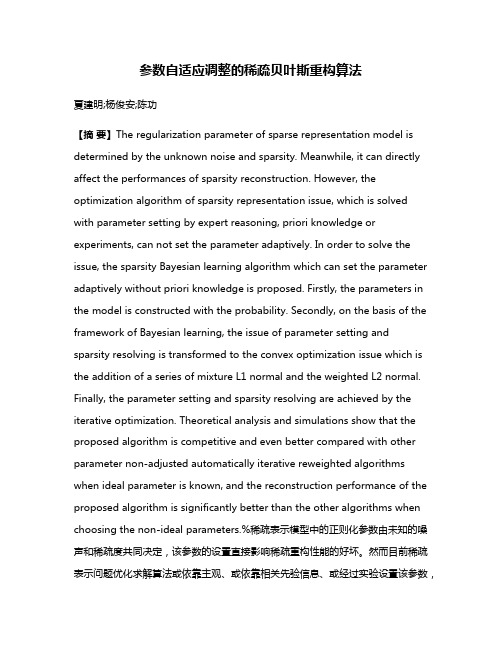
参数自适应调整的稀疏贝叶斯重构算法夏建明;杨俊安;陈功【摘要】The regularization parameter of sparse representation model is determined by the unknown noise and sparsity. Meanwhile, it can directly affect the performances of sparsity reconstruction. However, the optimization algorithm of sparsity representation issue, which is solvedwith parameter setting by expert reasoning, priori knowledge or experiments, can not set the parameter adaptively. In order to solve the issue, the sparsity Bayesian learning algorithm which can set the parameter adaptively without priori knowledge is proposed. Firstly, the parameters in the model is constructed with the probability. Secondly, on the basis of the framework of Bayesian learning, the issue of parameter setting and sparsity resolving is transformed to the convex optimization issue which is the addition of a series of mixture L1 normal and the weighted L2 normal. Finally, the parameter setting and sparsity resolving are achieved by the iterative optimization. Theoretical analysis and simulations show that the proposed algorithm is competitive and even better compared with other parameter non-adjusted automatically iterative reweighted algorithms when ideal parameter is known, and the reconstruction performance of the proposed algorithm is significantly better than the other algorithms when choosing the non-ideal parameters.%稀疏表示模型中的正则化参数由未知的噪声和稀疏度共同决定,该参数的设置直接影响稀疏重构性能的好坏。
基于动态贝叶斯网络的入侵意图识别方法

V0 .7 13
.
计
算
机
工
程
2 1 年 4月 0 1
Ap i 01 rl 2 1
NO8 .
Co p e g ne rn m utrEn i e ig
安全 技术 ・
文章编号:l 0_48 01 8 02— 3 0 _32( l0— 1 0 0_ 2 ) 7
p o e s ti p p r r snsa t s nitnin rc g io to ae nDy a cBa e inNe r sD ) Di c dAc ci Grp ( G) r c s, hs a e e e t n i r i ne t o n t n me db s do n mi p nuo o e i h y s t k ( BN . r t y l a h GA a wo ee c
[ ywod ]D nmi B ysa t ok( N)it s nitnin Di c d yl ahGA ; rbblt esnn Ke rs y a c a einNew rsDB ;n ui e t ; r t ciGrp ( G)po aisirao ig r o n o e e Ac c ic
a t c Ex e i n sr fe tt e c a gel w f n r s o n e t n i e a t c r c s , n r v st e f a i i t n a i iy t k. p rme t e c h h n a l a o t u i n i t n i n t t k p o e s a d p o e h e sb l y a d v l t . i o h a i d
DOI 1 .9 9 . s .0 0 3 2 0 .80 3 : 03 6 /i n1 0 —4 82 1 0 .4 js 1
基于贝叶斯-斯坦科尔伯格博弈的DNN动态防御方法

第38卷第3期 计算机应用与软件Vol 38No.32021年3月 ComputerApplicationsandSoftwareMar.2021基于贝叶斯 斯坦科尔伯格博弈的DNN动态防御方法王 芳1 周湘贞2,31(重庆商务职业学院 重庆401331)2(北京航空航天大学计算机学院 北京100191)3(郑州升达经贸管理学院信息工程系 河南郑州451191)收稿日期:2019-07-12。
国家自然科学基金面上项目(61672077);2018年度河南省重点研发与推广专项支持项目(182102110277)。
王芳,副教授,主研领域:计算机网络技术。
周湘贞,副教授。
摘 要 基于梯度攻击对图像进行修改,可造成基于神经网络的分类技术的精确度降低10%左右,针对这一问题,提出利用网络空间领域里移动目标防御思想来增加神经网络对抗该类攻击的鲁棒性。
定义整体网络集的“区别免疫”概念,将网络中防御方和用户之间的交互模拟为一个重复贝叶斯 斯坦科尔伯格博弈过程。
基于此从该组网络集中挑选出一个受训练的网络对输入图像进行分类。
该防御方法能减少MNIST数据库中受干扰图像的分类错误,同时对于正常的测试图像保持较高的分类精度。
该方法可以与现有的防御机制结合使用,确保神经网络安全性。
关键词 贝叶斯 斯坦科尔伯格博弈 移动目标防御 深度神经网络中图分类号 TP309.2 文献标志码 A DOI:10.3969/j.issn.1000 386x.2021.03.021DNNDYNAMICDEFENSEMETHODBASEDONBAYESIANSTACKELBERGGAMEWangFang1 ZhouXiangzhen2,31(ChongqingBusinessVocationalCollege,Chongqing401331,China)2(SchoolofComputerScienceandEngineering,BeihangUniversity,Beijing100191,China)3(DepartmentofInformationEngineering,ZhengzhouShengdaUniversityofEconomics,BusinessandManagement,Zhengzhou451191,Henan,China)Abstract Gradient basedattackcanreducetheaccuracyofclassificationtechnologybasedonneuralnetworkbyabout10%bymodifyingtheimage.Tosolvetheproblem,theideaofmovingtargetdefenseincyberspaceisusedtoenhancetherobustnessofneuralnetworkagainstsuchattacks.Theconceptof“differentialimmunity”ofthewholenetworksetwasdefined,andtheinteractionbetweenthedefenderandtheuserinthenetworkwassimulatedasarepeatedBayesianStackelberggamesprocess.Basedonthisprocess,atrainednetworkwasselectedfromthissetofnetworkstoclassifytheinputimages.ThedefensemethodcanreducetheclassificationerrorsofinterferedimagesinMNISTdatabase,andmaintainhighclassificationaccuracyfornormaltestimages.Themethodcanalsobecombinedwithexistingdefensemechanismtoensurethesecurityofneuralnetworks.Keywords BayesianStackelberggame Movingtargetdefense Deepneuralnetwork0 引 言当前基于深度神经网络(DeepNeuralNetworks,DNN)的图像分类系统被应用于多种重要场所,如支票上手写数字的识别[1]、自动监督中物品的分类、自动驾驶领域[2]等。
- 1、下载文档前请自行甄别文档内容的完整性,平台不提供额外的编辑、内容补充、找答案等附加服务。
- 2、"仅部分预览"的文档,不可在线预览部分如存在完整性等问题,可反馈申请退款(可完整预览的文档不适用该条件!)。
- 3、如文档侵犯您的权益,请联系客服反馈,我们会尽快为您处理(人工客服工作时间:9:00-18:30)。
Proceedings of the Fourth International Conference on User Modeling,Hyannis MA,August1994.
Dynamically Constructed Bayesian Networks
for Modeling Interests and Knowledge
Anthony Jameson and Ralph Sch¨a fer
Department of Computer Science,University of Saarbr¨u cken
P.O.Box151150,D-66041Saarbr¨u cken,Germany
jameson,ralph@cs.uni-sb.de
Inferring a user’s underlying interests and knowledge on
the basis of observable behavior is a complex task involving
a great deal of uncertainty.We demonstrate the utility of an
approach that combines the theory of intuitive psychomet-
rics(Jameson1992)with the techniques of Bayesian belief
networks(Pearl1991).
Simple Interpretation and Prediction
Intuitive psychometrics is a normative theory of how a per-
son predicts or interprets the(internal or observable)
responses of another person in terms of’s interests or
knowledgeability.To start with a simple example,suppose
that is trying to sell a car to and wants to predict how
the statement that the car has air conditioning would affect
’s evaluation of it on the dimension“comfort”.may
have impressions of two variables:(1)the level of interest
that this specific has in comfort and(2)the importance
for people in general of the implications of air conditioning
with respect to comfort.Such an impression can be seen as
a probability distribution that associates a probability with
each possible level of interest(or importance).can predict
’s expected evaluation shift if assumes that it depends
probabilisticallyon thefirst two variables(e.g.,that the most
likely evaluation shift is determined by their product).If
laterfinds out what’s evaluation shift actually was,
can interpret this information,using Bayes’Rule to derive
updated impressions of both variables.
Complications in Dialog Situations
These basic schemata have proven useful as standards of
comparison for human inferences in laboratory experiments.
In any realistic dialog situation,however,their application
encounters a number of complications.Some of these arise,
for example,when the dialog system P RACMA(Jameson et
al.1994)models in the car-selling situation mentioned
above.
First,several dimensions can be relevant to the evalua-
tion of a single fact about the car(e.g.,both“comfort”and。