Random projection, margins, kernels and feature selection
《概率论与数理统计》基本名词中英文对照表

《概率论与数理统计》基本名词中英文对照表英文中文Probability theory 概率论mathematical statistics 数理统计deterministic phenomenon 确定性现象random phenomenon 随机现象sample space 样本空间random occurrence 随机事件fundamental event 基本事件certain event 必然事件impossible event 不可能事件random test 随机试验incompatible events 互不相容事件frequency 频率classical probabilistic model 古典概型geometric probability 几何概率conditional probability 条件概率multiplication theorem 乘法定理Bayes's formula 贝叶斯公式Prior probability 先验概率Posterior probability 后验概率Independent events 相互独立事件Bernoulli trials 贝努利试验random variable 随机变量probability distribution 概率分布distribution function 分布函数discrete random variable 离散随机变量distribution law 分布律hypergeometric distribution 超几何分布random sampling model 随机抽样模型binomial distribution 二项分布Poisson distribution 泊松分布geometric distribution 几何分布probability density 概率密度continuous random variable 连续随机变量uniformly distribution 均匀分布exponential distribution 指数分布numerical character 数字特征mathematical expectation 数学期望variance 方差moment 矩central moment 中心矩n-dimensional random variable n-维随机变量two-dimensional random variable 二维离散随机变量joint probability distribution 联合概率分布joint distribution law 联合分布律joint distribution function 联合分布函数boundary distribution law 边缘分布律boundary distribution function 边缘分布函数exponential distribution 二维指数分布continuous random variable 二维连续随机变量joint probability density 联合概率密度boundary probability density 边缘概率密度conditional distribution 条件分布conditional distribution law 条件分布律conditional probability density 条件概率密度covariance 协方差dependency coefficient 相关系数normal distribution 正态分布limit theorem 极限定理standard normal distribution 标准正态分布logarithmic normal distribution 对数正态分布covariance matrix 协方差矩阵central limit theorem 中心极限定理Chebyshev's inequality 切比雪夫不等式Bernoulli's law of large numbers 贝努利大数定律statistics 统计量simple random sample 简单随机样本sample distribution function 样本分布函数sample mean 样本均值sample variance 样本方差sample standard deviation 样本标准差sample covariance 样本协方差sample correlation coefficient 样本相关系数order statistics 顺序统计量sample median 样本中位数sample fractiles 样本极差sampling distribution 抽样分布parameter estimation 参数估计estimator 估计量estimate value 估计值unbiased estimator 无偏估计unbiassedness 无偏性biased error 偏差mean square error 均方误差relative efficient 相对有效性minimum variance 最小方差asymptotic unbiased estimator 渐近无偏估计量uniformly estimator 一致性估计量moment method of estimation 矩法估计maximum likelihood method of estimation 极大似然估计法likelihood function 似然函数maximum likelihood estimator 极大似然估计值interval estimation 区间估计hypothesis testing 假设检验statistical hypothesis 统计假设simple hypothesis 简单假设composite hypothesis 复合假设rejection region 拒绝域acceptance domain 接受域test statistics 检验统计量linear regression analysis 线性回归分析。
gaussianrandomprojection参数

gaussianrandomprojection参数GaussianRandomProjection是一种常用的数据降维方法,它通过高斯随机投射将高维数据投影到低维空间,从而降低了数据的维度,提高了数据的可处理性。
在机器学习和数据分析中,GaussianRandomProjection是一种非常有用的工具,它可以用于特征提取、降维、数据可视化等多个方面。
在使用GaussianRandomProjection时,参数的选择和设置是非常重要的。
下面我们将介绍一些常用的GaussianRandomProjection参数及其含义和选择方法。
**1.随机投影矩阵的维度**GaussianRandomProjection的核心思想是通过高斯随机矩阵将原始数据投影到低维空间。
随机投影矩阵的维度是GaussianRandomProjection的一个重要参数。
一般来说,投影矩阵的维度越高,投影后的数据维数也越高,但可能会引入更多的噪声。
因此,在选择随机投影矩阵的维度时,需要根据具体的应用场景和数据特点进行权衡。
**2.投影矩阵的生成方式**GaussianRandomProjection的另一个重要参数是投影矩阵的生成方式。
一般来说,我们可以使用高斯随机矩阵或混合高斯矩阵来生成投影矩阵。
选择不同的投影矩阵生成方式会对投影后的数据产生不同的影响。
在某些情况下,使用混合高斯矩阵可能会得到更好的效果。
**3.投影次数**GaussianRandomProjection通常需要进行多次投影,以获得更好的降维效果。
投影次数是一个可选的参数,可以根据具体的应用场景和数据特点进行选择。
一般来说,如果数据包含噪声或异常值,可以适当增加投影次数,以提高降维效果和数据的可处理性。
**4.其他参数**除了上述参数外,GaussianRandomProjection还有一些其他的可选参数,如随机种子、投影方式等。
这些参数的具体设置方法也需要根据具体的应用场景和数据特点进行选择。
随机利率和跳-扩散过程下具有随机寿命的未定权益定价
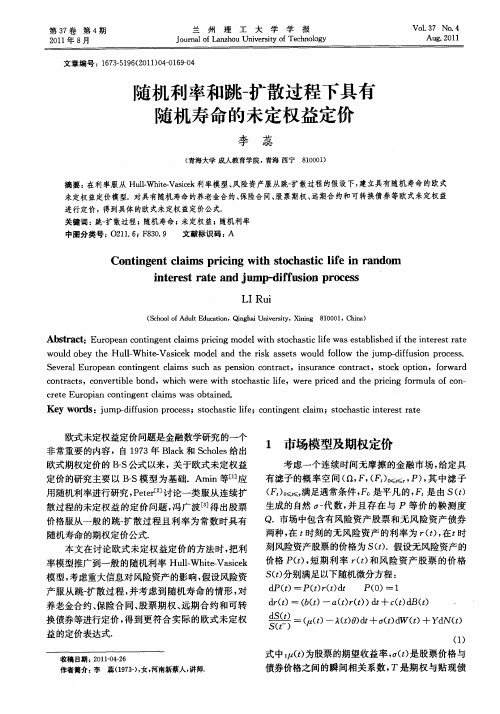
r1 ’
cs £, —E - ( ( —K) r)] ( ()t , P [s + (s= s d
S() 1 ,,) ( )u+ S £,) ( () tu f u d
非常重 要 的 内容 ,自 17 93年 Bak和 S hls给 出 lc eoe
1 市场模型及期权定价
考 虑一 个 连续 时 间无 摩 擦 的金 融 市 场 , 给定 具
欧式期权定价 的 IS } 公式以来, 关于欧式未定权益 定价的研究主要 以 BS 型为基础.A n等[应 -模 mi 1 ]
d () (() n £r £) t c d r t : 6 £ 一 () () d + () B()
:
。
,
( £ ()一 A tO d + () Ⅳ ( ) yd £ () ) t £d f+ N( ) () 1
式 中:( 为股票的期望收益率 ,() ) £是股票价格与 债券价格之间的瞬间相关系数 , T是期权与贴现债
未定权益定价模型.对具有 随机寿命 的养老金合约 、 险合 同、 票期权 、 期合 约和可转换债券 等欧式未定权 益 保 股 远 进行定价 ,得到具体 的欧式未 定权 益定 价公 式. 关键词 :跳一 扩散过程;随机寿命 ; 未定 权益;随机利率
中图分类号 :O 1. 1F 3 . 2 16 8 0 9 文献标识码 : A
作者简介 : 李
蕊 (93)女 , 17一, 河南新蔡人 , 师. 讲
・
10 ・ 7
兰 州 理 工 大 学 学 报
第 3 卷 7
券 的到期 日, 是期权的执行价格, ( ) , £ K S O 一S B() 和 w( 为相互独立 的标准 B o n £ ) rw 运动 , 是 与 N() w( 相互独立 的参数 为 () P i o £ ) 的 o sn过程, s y是 股份每次跳跃 的高度 , 且为随机变量 , № y 表示在
浙大经济学考博真题
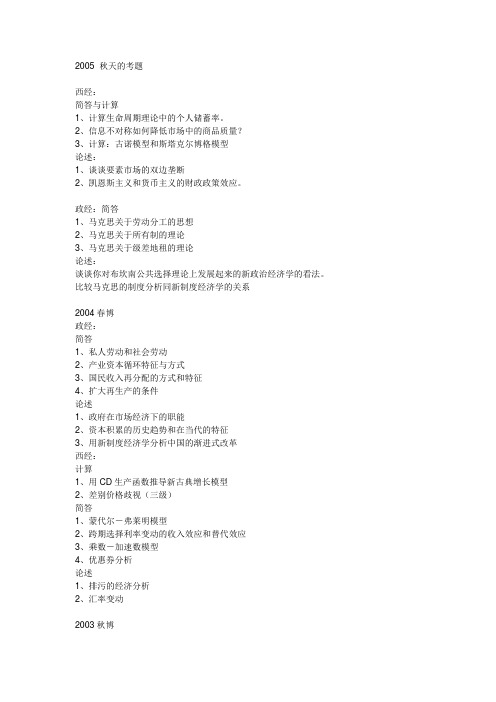
2005 秋天的考题西经:简答与计算1、计算生命周期理论中的个人储蓄率。
2、信息不对称如何降低市场中的商品质量?3、计算:古诺模型和斯塔克尔博格模型论述:1、谈谈要素市场的双边垄断2、凯恩斯主义和货币主义的财政政策效应。
政经:简答1、马克思关于劳动分工的思想2、马克思关于所有制的理论3、马克思关于级差地租的理论论述:谈谈你对布坎南公共选择理论上发展起来的新政治经济学的看法。
比较马克思的制度分析同新制度经济学的关系2004春博政经:简答1、私人劳动和社会劳动2、产业资本循环特征与方式3、国民收入再分配的方式和特征4、扩大再生产的条件论述1、政府在市场经济下的职能2、资本积累的历史趋势和在当代的特征3、用新制度经济学分析中国的渐进式改革西经:计算1、用CD生产函数推导新古典增长模型2、差别价格歧视(三级)简答1、蒙代尔-弗莱明模型2、跨期选择利率变动的收入效应和替代效应3、乘数-加速数模型4、优惠券分析论述1、排污的经济分析2、汇率变动2003秋博政经:简答1、分工理论2、经济增长与制度变迁3、商品经济与市场经济转换4、要素论述1、政经体系的改革2、劳动价值论与边际效用论的比较3、价格双轨制的分析2003春博西经:计算1、消费者均衡2、两部门收入决定简答1、洛论兹曲线和基尼系数2、艾奇沃斯盒3、关税、配额分析4、囚徒困境论述1、资本资产定价模型2、新古典内生增长其他几届政经:1、方法论对经济研究的指导意义2、劳动价值论的当今争论3、对企业性质的理解4、开放对经济转型的作用西经:IS-LM模型增长率的计算收入决定理论拉格朗日乘数效应。
机器学习与深度学习框架考核试卷

C. RMSprop
D.学习率衰减
8.以下哪些技术可以用于改善神经网络训练过程?()
A.梯度消失
B.梯度爆炸
C. Batch Normalization
D.参数共享
9.以下哪些框架支持GPU加速计算?()
A. TensorFlow
B. PyTorch
C. Caffe
D. Theano
10.以下哪些方法可以用于处理不平衡数据集?()
B. LSTM
C. CNN
D. Transformer
17.以下哪些技术可以用于提升神经网络的可解释性?()
A.可视化技术
B. attention机制
C. LIME
D. SHAP
18.以下哪些是深度学习中的预训练方法?()
A.零样本学习
B.迁移学习
C.对抗性学习
D.自监督学习
19.以下哪些是强化学习的主要组成部分?()
7.词嵌入(或词向量)
8.泛化
9.数据增强
10. ROC
四、判断题
1. ×
2. √
3. ×
4. ×
5. √
6. ×
7. ×
8. √
9. ×
10. √
五、主观题(参考)
1.机器学习是利用算法让计算机从数据中学习,深度学习是机器学习的一个分支,使用多层神经网络进行学习。深度学习在实际应用中的例子有:自动驾驶汽车中的图像识别系统。
()
9.在深度学习中,为了防止过拟合,我们可以在训练过程中对输入数据进行______。
()
10.在模型评估中,______曲线可以用来评估分类模型的性能,尤其是对于不平衡数据集。
()
人工智能基础(习题卷9)
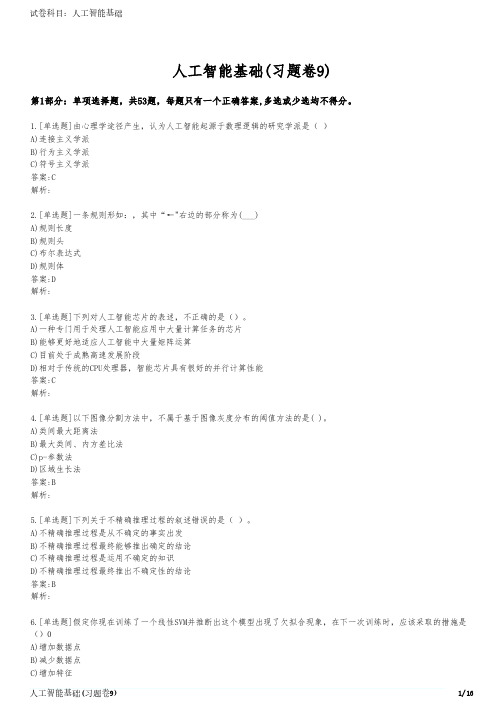
人工智能基础(习题卷9)第1部分:单项选择题,共53题,每题只有一个正确答案,多选或少选均不得分。
1.[单选题]由心理学途径产生,认为人工智能起源于数理逻辑的研究学派是( )A)连接主义学派B)行为主义学派C)符号主义学派答案:C解析:2.[单选题]一条规则形如:,其中“←"右边的部分称为(___)A)规则长度B)规则头C)布尔表达式D)规则体答案:D解析:3.[单选题]下列对人工智能芯片的表述,不正确的是()。
A)一种专门用于处理人工智能应用中大量计算任务的芯片B)能够更好地适应人工智能中大量矩阵运算C)目前处于成熟高速发展阶段D)相对于传统的CPU处理器,智能芯片具有很好的并行计算性能答案:C解析:4.[单选题]以下图像分割方法中,不属于基于图像灰度分布的阈值方法的是( )。
A)类间最大距离法B)最大类间、内方差比法C)p-参数法D)区域生长法答案:B解析:5.[单选题]下列关于不精确推理过程的叙述错误的是( )。
A)不精确推理过程是从不确定的事实出发B)不精确推理过程最终能够推出确定的结论C)不精确推理过程是运用不确定的知识D)不精确推理过程最终推出不确定性的结论答案:B解析:6.[单选题]假定你现在训练了一个线性SVM并推断出这个模型出现了欠拟合现象,在下一次训练时,应该采取的措施是()0A)增加数据点D)减少特征答案:C解析:欠拟合是指模型拟合程度不高,数据距离拟合曲线较远,或指模型没有很好地捕 捉到数据特征,不能够很好地拟合数据。
可通过增加特征解决。
7.[单选题]以下哪一个概念是用来计算复合函数的导数?A)微积分中的链式结构B)硬双曲正切函数C)softplus函数D)劲向基函数答案:A解析:8.[单选题]相互关联的数据资产标准,应确保()。
数据资产标准存在冲突或衔接中断时,后序环节应遵循和适应前序环节的要求,变更相应数据资产标准。
A)连接B)配合C)衔接和匹配D)连接和配合答案:C解析:9.[单选题]固体半导体摄像机所使用的固体摄像元件为( )。
pd莫顿前瞻的财务解释
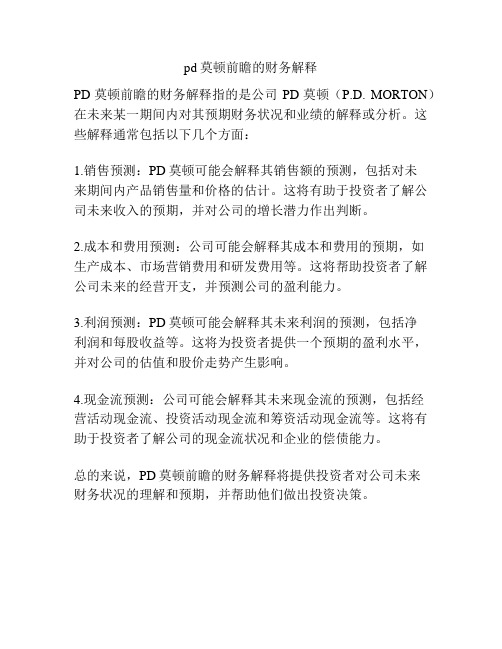
pd莫顿前瞻的财务解释
PD莫顿前瞻的财务解释指的是公司PD莫顿(P.D. MORTON)在未来某一期间内对其预期财务状况和业绩的解释或分析。
这些解释通常包括以下几个方面:
1.销售预测:PD莫顿可能会解释其销售额的预测,包括对未
来期间内产品销售量和价格的估计。
这将有助于投资者了解公司未来收入的预期,并对公司的增长潜力作出判断。
2.成本和费用预测:公司可能会解释其成本和费用的预期,如
生产成本、市场营销费用和研发费用等。
这将帮助投资者了解公司未来的经营开支,并预测公司的盈利能力。
3.利润预测:PD莫顿可能会解释其未来利润的预测,包括净
利润和每股收益等。
这将为投资者提供一个预期的盈利水平,并对公司的估值和股价走势产生影响。
4.现金流预测:公司可能会解释其未来现金流的预测,包括经
营活动现金流、投资活动现金流和筹资活动现金流等。
这将有助于投资者了解公司的现金流状况和企业的偿债能力。
总的来说,PD莫顿前瞻的财务解释将提供投资者对公司未来
财务状况的理解和预期,并帮助他们做出投资决策。
随机森林模型假设条件

随机森林模型假设条件
1 随机森林模型
随机森林模型是一种常用的机器学习技术,用来帮助你预测结果
并对输入变量进行分类或回归。
它是一种集成学习算法,用于解决最
优特征组合/组合筛选问题。
它与单一决策树不同,它将多个决策树组
合起来,以赢得更好的准确性。
简单地说,随机森林模型使用一组决
策树,并反映树出现结果的不同方式,以获得最佳准确性。
2 假设条件
对于随机森林模型而言,假设条件的主要原则是:1)决策树是独
立的;2)所有样本都有等可能地被决策树所采用;3)每个决策树以
它所选择的特征做出决定;4)总体结果基于决策树以投票方式生成;5)不同树之间不能有相同的决定;6)特征值采用均匀分布。
考虑特征值分布特别重要,因为随机森林模型的运行基于决策树
的运行,而决策树正是基于分布的结果,如果特征值分布不均匀,可
能会导致运行结果的出入。
在使用随机森林模型的时候,最好的策略
就是将输入数据进行归一化地处理,以保证特征值的分布是均匀的。
随机森林模型假设条件是此模型正确运行和产生最佳结果的基础。
如果这些条件不被正确遵守,结果可能是不可预测的,或者不可信任的。
因此,在使用随机森林模型之前,最好能确保输入数据的准确性,以及存在正确的假设条件。
- 1、下载文档前请自行甄别文档内容的完整性,平台不提供额外的编辑、内容补充、找答案等附加服务。
- 2、"仅部分预览"的文档,不可在线预览部分如存在完整性等问题,可反馈申请退款(可完整预览的文档不适用该条件!)。
- 3、如文档侵犯您的权益,请联系客服反馈,我们会尽快为您处理(人工客服工作时间:9:00-18:30)。
1
Introduction
Random projection is a technique that has found substantial use in the area of algorithm design (especially approximation algorithms), by allowing one to substantially reduce dimensionality of a problem while still retaining a significant degree of problem structure. In particular, given n points in Euclidean space (of any dimension but which we can think of as Rn ), we can project these points down to a random d-dimensional subspace for d ≪ n, with the following outcomes: 1. If d = ω ( γ12 log n) then Johnson-Lindenstrauss type results (described below) imply that with high probability, relative distances and angles between all pairs of points are approximately preserved up to 1 ± γ . 2. If d = 1 (i.e., we project points onto a random line) we can often still get something useful. Projections of the first type have had a number of uses including fast approximate nearest-neighbor algorithms [IM98,EK00] and approximate clustering algorithms [Sch00] among others. Projections of the second type are often used for “rounding” a semidefinite-programming relaxation, such as for the Max-CUT problem [GW95], and have been used for various graph-layout problems [Vem98]. The purpose of this survey is to describe some ways that this technique can be used (either practically, or for providing insight) in the context of machine
பைடு நூலகம்
We are considering the standard PAC-style setting of supervised learning from i.i.d. data. Specifically, we assume that examples are given to us according to some probability distribution D over an instance space X and labeled by some unknown target function c : X → {−1, +1}. We use P = (D, c) to denote the combined distribution over labeled examples. Given some sample S of labeled training examples (each drawn independently from D and labeled by c), our objective is to come up with a hypothesis h with low true error: that is, we want Prx∼D (h(x) = c(x)) to be low. In the discussion below, by a “learning problem” we mean a distribution P = (D, c) over labeled examples. In the first part of this survey (Sections 2 and 3), we will think of the input space X as Euclidean space, like Rn . In the second part (Section 4), we will discuss kernel functions, in which case one should think of X as just some abstract space, and a kernel function K : X × X → [−1, 1] is then some function that provides a measure of similarity between two input points. Formally, one requires for a legal kernel K that there exist some implicit function φ mapping X into a (possibly very high-dimensional) space, such that K (x, y ) = φ(x) · φ(y ). In fact, one interesting property of some of the results we discuss is that they make sense to apply even if K is just an arbitrary similarity function, and not a “legal” kernel, though the theorems make sense only if such a φ exists. Extensions of this framework to more general similarity functions are given in [BB06]. Definition 1. We say that a set S of labeled examples is linearly separable by margin γ if there exists a unit-length vector w such that:
learning. In particular, random projection can provide a simple way to see why data that is separable by a large margin is easy for learning even if data lies in a high-dimensional space (e.g., because such data can be randomly projected down to a low dimensional space without affecting separability, and therefore it is “really” a low-dimensional problem after all). It can also suggest some especially simple algorithms. In addition, random projection (of various types) can be used to provide an interesting perspective on kernel functions, and also provide a mechanism for converting a kernel function into an explicit feature space. The use of Johnson-Lindenstrauss type results in the context of learning was first proposed by Arriaga and Vempala [AV99], and a number of uses of random projection in learning are discussed in [Vem04]. Experimental work on using random projection has been performed in [FM03,GBN05,Das00]. This survey, in addition to background material, focuses primarily on work in [BB05,BBV04]. Except in a few places (e.g., Theorem 1, Lemma 1) we give only sketches and basic intuition for proofs, leaving the full proofs to the papers cited. 1.1 The setting
Abstract. Random projection is a simple technique that has had a number of applications in algorithm design. In the context of machine learning, it can provide insight into questions such as “why is a learning problem easier if data is separable by a large margin?” and “in what sense is choosing a kernel much like choosing a set of features?” This talk is intended to provide an introduction to random projection and to survey some simple learning algorithms and other applications to learning based on it. I will also discuss how, given a kernel as a black-box function, we can use various forms of random projection to extract an explicit small feature space that captures much of what the kernel is doing. This talk is based in large part on work in [BB05,BBV04] joint with Nina Balcan and Santosh Vempala.