Automatic Detection of Interaction Groups
统计学专业名词中英对照

统计学专业名词中英对照Lane某y我大学毕业已经多年,这些年来,越发感到外刊的重要性。
读懂外刊要有不错的英语功底,同时,还需要掌握一定的专业词汇。
掌握足够的专业词汇,在国内外期刊的阅读和写作中会游刃有余。
abcia横坐标abencerate缺勤率Abolutedeviation绝对离差Abolutenumber绝对数abolutevalue绝对值Abolutereidual绝对残差accidenterror偶然误差Accelerationarray加速度立体阵Accelerationinanarbitrarydirection任意方向上的加速度Accelerationnormal法向加速度Accelerationpacedimenion加速度空间的维数Accelerationtangential切向加速度Accelerationvector加速度向量Acceptablehypothei可接受假设Accumulation累积Accumulatedfrequency累积频数Accuracy准确度Actualfrequency实际频数Adaptiveetimator自适应估计量Addition相加Additiontheorem加法定理AdditiveNoie加性噪声Additivity可加性Adjutedrate调整率Adjutedvalue校正值Admiibleerror容许误差Aggregation聚集性Alphafactoringα因子法Alternativehypothei备择假设Amonggroup组间Amount总量ANOVA(analyiofvariance)方差分析ANOVAModel方差分析模型ANOVAtableandeta分组计算方差分析Arcing弧/弧旋Arcinetranformation反正弦变换Area区域图Areaunderthecurve曲线面积AREG评估从一个时间点到下一个时间点回归相关时的误差ARIMA季节和非季节性单变量模型的极大似然估计Arithmeticgridpaper算术格纸Arithmeticmean算术平均数Arithmeticweightedmean加权算术均数Arrheniurelation艾恩尼斯关系Aeingfit拟合的评估Aociativelaw结合律Aumedmean假定均数Aymmetricditribution非对称分布Autocorrelationofreidual残差的自相关Average平均数Averageconfidenceintervallength平均置信区间长度averagedeviation平均差Averagegrowthrate平均增长率BBarchart/graph条形图Baeperiod基期Baye'theoremBaye定理Bell-hapedcurve钟形曲线Bernoulliditribution伯努力分布Bet-trimetimator最好切尾估计量Bia偏性Biometric生物统计学Binarylogiticregreion二元逻辑斯蒂回归Binomialditribution二项分布Biquare双平方BivariateCorrelate二变量相关Bivariatenormalditribution双变量正态分布Bivariatenormalpopulation双变量正态总体Biweightinterval双权区间BiweightM-etimator双权M估计量Block区组/配伍组Canonicalcorrelation典型相关Caption纵标目Cartogram统计图Caefatalityrate病死率Cae-controltudy病例对照研究Categoricalvariable分类变量Catenary悬链线Cauchyditribution柯西分布Caue-and-effectrelationhip因果关系Cell单元Cenoring终检cenu普查Centerofymmetry对称中心Centeringandcaling中心化和定标Centraltendency集中趋势Centralvalue中心值CHAID-χ2AutomaticInteractionDetector卡方自动交互检测Chance 机遇Chanceerror随机误差Chancevariable随机变量Characteriticequation特征方程Characteriticroot特征根Characteriticvector特征向量Chebhevcriterionoffit拟合的切比雪夫准则Chernoffface切尔诺夫脸谱图Claifiedvariable分类变量Cluteranalyi聚类分析Cluterampling 整群抽样Code代码Codeddata编码数据Coding编码Coefficientofmultiplecorrelation多重相关系数Coefficientofpartialcorrelation偏相关系数Columneffect列效应Columnfactor列因素Conditionale某pectation条件期望Conditionallikelihood条件似然Conditionalprobability条件概率Conditionallylinear依条件线性Confidenceinterval置信区间Confidencelevel可信水平,置信水平Confidencelimit置信限Confidencelowerlimit置信下限Confidenceupperlimit置信上限ConfirmatoryFactorAnalyi验证性因子分析Confirmatoryreearch 证实性实验研究Confoundingfactor混杂因素Conjoint联合分析Conitency相合性Conitencycheck一致性检验Conitentaymptoticallynormaletimate相合渐近正态估计Conitentetimate相合估计Contituentratio构成比,结构相对数Contrainednonlinearregreion受约束非线性回归Contraint约束Contaminatedditribution污染分布ContaminatedGauian污染高斯分布Contaminatednormalditribution污染正态分布Contamination污染Contaminationmodel污染模型Continuity连续性Contingencytable 列联表Contour边界线Contributionrate贡献率Control对照质量控制图Controlgroup对照组Controllede某periment对照实验Conventionaldepth常规深度Convolution卷积Coordinate坐标Correctedfactor校正因子Correctedmean校正均值Correctioncoefficient校正系数Correctionforcontinuity连续性校正Correctionforgrouping归组校正Correctionnumber校正数Correctionvalue校正值Correctne正确性Equalun-clanumber相等次级组含量Equallylikely等可能Equationoflinearregreion线性回归方程Equivariance同变性Error误差/错误Errorofetimate估计误差Errorofreplication重复误差ErrortypeI 第一类错误ErrortypeII第二类错误Etimand被估量Etimatederrormeanquare估计误差均方Etimatederrorumofquare估计误差平方和Euclideanditance欧式距离Event事件E某ceptionaldatapoint异常数据点E某pectationplane期望平面E某pectationurface期望曲面E某pectedvalue期望值E某periment 实验E某perimentdeign实验设计E某perimenterror实验误差E某perimentalgroup实验组E某perimentalampling试验抽样E某perimentalunit试验单位E某plainedvariance(已说明方差)E某planatoryvariable说明变量E某ploratorydataanalyi探索性数据分析E某ploreSummarize探索-摘要E某ponentialcurve指数曲线E某ponentialgrowth指数式增长E某SMOOTH指数平滑方法E某tendedfit扩充拟合E某traparameter附加参数E某trapolation外推法E某tremeobervation末端观测值E某treme极端值/极值FFditributionF分布FtetF检验Factor因素/因子Factoranalyi因子分析FactorAnalyi因子分析Factorcore因子得分Factorial阶乘Factorialdeign析因试验设计Falenegative假阴性Falenegativeerror假阴性错误Familyofditribution分布族Familyofetimator估计量族Fanning扇面Fatalityrate病死率Fieldinvetigation现场调查Fieldurvey现场调查Finitepopulation有限总体Finite-ample有限样本Firtderivative一阶导数Forecat预测Fourfoldtable四格表Fourth四分点Fractionblow左侧比率Fractionalerror相对误差Frequency频率Freguencyditribution频数分布Frequencypolygon频数多边图Frontierpoint界限点Functionrelationhip泛函关系GGammaditribution伽玛分布Gauincrement高斯增量Gauianditribution高斯分布/正态分布Gau-Newtonincrement高斯-牛顿增量Generalcenu全面普查Generalizedleatquare综合最小平方法GENLOG(Generalizedlinermodel)广义线性模型Geometricmean几何平均数Gini'meandifference基尼均差GLM(Generallinermodel)通用线性模型Goodneoffit拟和优度/配合度Gradientofdeterminant行列式的梯度Graeco-Latinquare希腊拉丁方Grandmean总均值Groerror重大错误Half-life半衰期HampelM-etimator汉佩尔M估计量Happentance偶然事件Harmonicmean调和均数Hazardfunction风险均数Hazardrate风险率Heading标目Heavy-tailedditribution重尾分布Heianarray海森立体阵Heterogeneity不同质Heterogeneityofvariance方差不齐Hierarchicalclaification组内分组Hierarchicalcluteringmethod系统聚类法High-leveragepoint高杠杆率点High-Low低区域图HigherOrderInteractionEffect,高阶交互作用HILOGLINEAR多维列联表的层次对数线性模型Hinge折叶点Hitogram直方图Hitoricalcohorttudy历史性队列研究Hole空洞HOMALS多重响应分析Homogeneityofvariance方差齐性Homogeneitytet齐性检验HuberM-etimator休伯M估计量Hyperbola双曲线Hypotheiteting假设检验Hypotheticalunivere假设总体IImagefactoring多元回归法Impoibleevent不可能事件Independence独立性Independentvariable自变量Inde某指标/指数Indirecttandardization间接标准化法Individual个体Inferenceband推断带Infinitepopulation无限总体Infinitelygreat无穷大Infinitelymall无穷小Influencecurve影响曲线Informationcapacity信息容量Initialcondition初始条件Initialetimate初始估计值Initiallevel最初水平Interaction交互作用Interactionterm交互作用项Intercept截距Interpolation内插法Interquartilerange四分位距Intervaletimation区间估计Intervalofequalprobability等概率区间Intriniccurvature固有曲率Invariance不变性Inverematri某逆矩阵Invereprobability逆概率Invereinetranformation反正弦变换Iteration迭代JJacobiandeterminant雅可比行列式Jointditributionfunction分布函数Jointprobability联合概率Jointprobabilityditribution联合概率分布KK-MeanCluter逐步聚类分析Kmeanmethod逐步聚类法Kaplan-Meier 评估事件的时间长度Kaplan-MerierchartKaplan-Merier图Kendall'rankcorrelationKendall等级相关Kinetic动力学Kolmogorov-Smirnovetet柯尔莫哥洛夫-斯米尔诺夫检验KrukalandWallitetKrukal及Walli检验/多样本的秩和检验/H检验Kurtoi峰度LLackoffit失拟Ladderofpower幂阶梯Lag滞后Largeample大样本Largeampletet大样本检验Latinquare拉丁方Latinquaredeign拉丁方设计Leakage泄漏Leatfavorableconfiguration最不利构形Leatfavorableditribution最不利分布Leatignificantdifference最小显著差法Leatquaremethod最小二乘法LeatSquaredCriterion,最小二乘方准则Leat-abolute-reidualetimate最小绝对残差估计Leat-abolute-reidualfit最小绝对残差拟合Leat-abolute-reidualline最小绝对残差线Legend图例L-etimatorL估计量L-etimatoroflocation位置L估计量L-etimatorofcale尺度L估计量Level水平LeveageCorrection,杠杆率校正Lifee某pectance预期期望寿命Lifetable寿命表Lifetablemethod生命表法Light-tailedditribution轻尾分布Likelihoodfunction似然函数Likelihoodratio似然比linegraph 线图Linearcorrelation直线相关Linearequation线性方程Linearprogramming线性规划Linearregreion直线回归LinearRegreion 线性回归Lineartrend线性趋势Loading载荷Locationandcaleequivariance位置尺度同变性Locationequivariance位置同变性Locationinvariance位置不变性Locationcalefamily位置尺度族Logranktet时序检验Logarithmiccurve 对数曲线Logarithmicnormalditribution对数正态分布Logarithmiccale对数尺度Logarithmictranformation对数变换Logiccheck逻辑检查Logiticditribution逻辑斯特分布LogittranformationLogit转换Outlier极端值OVERALS多组变量的非线性正规相关Overhoot迭代过度PPaireddeign配对设计Pairedample配对样本Pairwielope成对斜率Parabola抛物线Paralleltet平行试验Parameter参数Parametrictatitic参数统计Parametrictet参数检验Pareto直条构成线图(佩尔托图)Partialcorrelation偏相关Partialregreion偏回归Partialorting偏排序Partialreidual偏残差Pattern模式PCA(主成分分析)Pearoncurve皮尔逊曲线Peeling退层Percentbargraph百分条形图Percentage百分比Percentile百分位数Percentilecurve百分位曲线Periodicity周期性Permutation排列P-etimatorP估计量Piegraph 构成图饼图Pitmanetimator皮特曼估计量Pivot枢轴量Planar平坦Planaraumption平面的假设PLANCARDS生成试验的计划卡PLS(偏最小二乘法)Pointetimation点估计Poionditribution泊松分布Polihing 平滑Polledtandarddeviation合并标准差Polledvariance合并方差Polygon多边图Polynomial多项式Polynomialcurve多项式曲线Population总体Probabilitydenity概率密度Productmoment乘积矩/协方差Profiletrace截面迹图Proportion比/构成比Proportionallocationintratifiedrandomampling按比例分层随机抽样Proportionate成比例Proportionateub-clanumber成比例次级组含量Propectivetudy前瞻性调查Pro某imitie亲近性PeudoFtet近似F检验Peudomodel近似模型Peudoigma伪标准差Purpoiveampling有目的抽样QQuantile-quantileplot分位数-分位数图/Q-Q图Quantitativeanalyi定量分析Quartile四分位数QuickCluter快速聚类RRadi某ort基数排序Randomallocation随机化分组Randomblockdeign随机区组设计Randomevent随机事件Randomization随机化Range极差/全距Rankcorrelation等级相关Reciprocaltranformation倒数变换Recording记录Redecendingetimator回降估计量Reducingdimenion降维Re-e某preion重新表达Referenceet标准组Regionofacceptance接受域Regreioncoefficient回归系数Regreionumofquare回归平方和Rejectionpoint拒绝点Relativediperion相对离散度Relativenumber 相对数Reliability可靠性Reparametrization重新设置参数Replication重复ReportSummarie 报告摘要Reidualumofquare剩余平方和reidualvariance(剩余方差)Reitance耐抗性Reitantline耐抗线Reitanttechnique耐抗技术R-etimatoroflocation位置R估计量R-etimatorofcale尺度R估计量Retropectivetudy回顾性调查Ridgetrace岭迹RiditanalyiRidit分析Rotation旋转Rounding舍入Row行Roweffect行效应Rowfactor行因素R某CtableR某C表SSample样本Sampleregreioncoefficient样本回归系数Sampleize样本量Sampletandarddeviation样本标准差Samplingerror抽样误差SAS(Statiticalanalyiytem)SAS统计软件包Scale尺度/量表Scatterdiagram散点图Schematicplot示意图/简图Scoretet计分检验Screening筛检SEASON季节分析Secondderivative二阶导数SEM(Structuralequationmodeling)结构化方程模型Semi-logarithmicgraph半对数图Semi-logarithmicpaper半对数格纸Senitivitycurve敏感度曲线Sequentialanalyi贯序分析Sequence普通序列图Sequentialdataet顺序数据集Sequentialdeign贯序设计Sequentialmethod贯序法Sequentialtet贯序检验法Serialtet系列试验Short-cutmethod简捷法SigmoidcurveS形曲线Signfunction正负号函数Signtet符号检验Signedrank符号秩SignificantLevel显著水平Significancetet显著性检验Significantfigure有效数字Simplecluterampling简单整群抽样Simplecorrelation简单相关Simplerandomampling简单随机抽样Simpleregreion简单回归impletable简单表Sineetimator正弦估计量Single-valuedetimate单值估计Singularmatri某奇异矩阵Skewedditribution偏斜分布Skewne 偏度Slahditribution斜线分布Slope斜率Spearmanrankcorrelation斯皮尔曼等级相关Specificfactor特殊因子Specificfactorvariance特殊因子方差Spectra频谱Sphericalditribution球型正态分布Spread展布SPSS(Statiticalpackagefortheocialcience)Spurioucorrelation 假性相关Squareroottranformation平方根变换Stabilizingvariance稳定方差Standarddeviation标准差Standarderror标准误Standarderrorofdifference差别的标准误Standarderrorofetimate 标准估计误差Standarderrorofrate率的标准误Standardnormalditribution标准正态分布Standardization标准化Startingvalue起始值Statitic统计量Statiticalcontrol统计控制Statiticalgraph统计图Statiticalinference统计推断SPSS统计软件包Statiticaltable统计表Steepetdecent最速下降法Stemandleafdiplay茎叶图Stepfactor步长因子Stepwieregreion逐步回归Storage存Strata层(复数)Stratifiedampling分层抽样Stratifiedampling分层抽样Strength 强度Stringency严密性Structuralrelationhip结构关系Studentizedreidual学生化残差/t化残差Sub-clanumber次级组含量Subdividing分割Sufficienttatitic充分统计量Sumofproduct积和Sumofquare离差平方和Sumofquareaboutregreion回归平方和Sumofquarebetweengroup组间平方和Sumofquareofpartialregreion偏回归平方和Sureevent必然事件Survey调查。
一种机器人未知环境下动态目标跟踪交互多模滤波算法
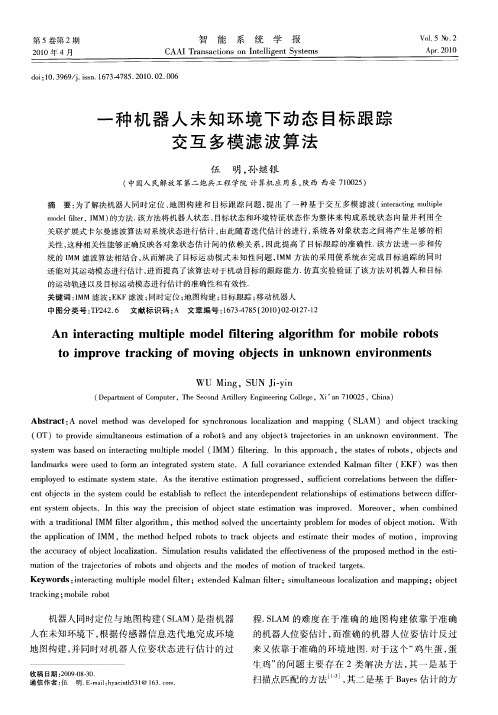
m dlie, MM) oe ftr I l 的方 法. 该方法将机器人状态 、 目标状 态和环境 特征状态 作为整体 来构成 系统状态 向量并 利用全 关联扩展式卡尔曼滤波算法对 系统状态进行估计 , 由此随着迭代估计 的进行 , 系统 各对象状 态之间将产 生足够 的相 关性 , 这种相关性 能够正确反 映各 对象状态估 计 问的依赖关 系 , 因此提 高了 目标 跟踪 的准确性 . 该方 法进 一步和传
An i t r c i g m u tp e m o e le i g a g r t m o o ie r b t n e a tn li l d lf t r n l o ih i f r m b l o o s
t r v r c ig o vn bet i n n wn e vr n ns oi o eta kn f mp mo ig ojcs n u k o n io me t
关键 词 : I 滤波 ;K MM E F滤 波 ; 同时 定 位 ; 图构 建 ; 地 目标 跟 踪 ; 动机 器人 移 中 图分 类 号 : P4 . 文 献标 识码 : 文 章 编 号 :6 3 75 2 1 )20 2 — T 226 A 17 - 8 (0 0 0 -t71 4 2
W U Mi g UN j—i n .S i n y
( eat n o o ue, h eo dA tlr E gnei o ee X ’n 10 5, hn ) D pr met f mpt T eScn rl y nier gC lg , ia 0 2 C ia C r ie n l 7
第 5卷第 2期
21 0 0年 4月 智能系统
学
报
VoI5 № . . 2
CAAITr n a to n I tli e tS se a s cinso n elg n y t ms
人工智能辅助语音识别的关键技术

人工智能辅助语音识别的关键技术人工智能(Artificial Intelligence,简称AI)的快速发展已经渗透到我们生活的各个方面。
语音识别作为人机交互的重要手段之一,受到了广泛的关注和应用。
人工智能技术的不断进步,为语音识别带来了革命性的突破。
本文将探讨人工智能辅助语音识别的关键技术以及其在现实生活中的应用。
一、声学模型声学模型是语音识别中的重要组成部分,用于将声音信号转换为文字。
传统的声学模型基于高斯混合模型(Gaussian Mixture Model,简称GMM),但其对于复杂声音的处理效果有限。
近年来,随着深度学习(Deep Learning)技术的发展,逐渐出现了基于深度神经网络(Deep Neural Network,简称DNN)的声学模型,如深度神经网络隐马尔可夫模型(Deep Neural Network Hidden Markov Model,简称DNN-HMM)。
DNN-HMM模型利用深度神经网络对声音信号进行特征提取和建模,大大提高了声学模型的性能和准确度。
通过多层次的神经网络结构,DNN-HMM模型能够学习到更复杂的语音特征表征,从而提高语音识别的效果。
此外,还有基于循环神经网络(Recurrent Neural Network,简称RNN)的声学模型,如长短时记忆网络(Long Short-Term Memory,简称LSTM),能够更好地处理时序信息,提高语音识别的鲁棒性。
二、语言模型语言模型是语音识别中另一个重要的组成部分,用于对识别结果进行语言上下文的判断和调整。
传统的语言模型主要基于统计的方法,如n-gram模型。
但这种方法需要对大量的语料库进行统计分析,且容易受到语料库大小和稀疏性的限制。
随着深度学习的发展,基于神经网络的语言模型逐渐兴起。
其中,循环神经网络(RNN)在语言模型中的应用得到了广泛的关注。
RNN 能够通过学习上下文之间的依赖关系,更好地捕捉到长距离的语言依赖关系,从而提高语音识别的准确度和流畅度。
北京理工大学科技成果——一种基于自适应采样的实时视觉跟踪系统

北京理工大学科技成果——一种基于自适应采样的
实时视觉跟踪系统
成果简介
视觉跟踪是计算机视觉领域中备受关注的一个研究热点。
它在智能交通、人机交互、视频监控和军事等领域有着广泛的应用。
在实际应用中,视觉跟踪方法通常需要处理一些复杂的目标运动如运动突变和几何外观急剧变化等情况,传统的跟踪技术无法满足跟踪要求。
我们提出基于自适应MCMC采样的方法,很好地解决了这一难题,为实际应用中复杂运动目标的跟踪提供了保障。
项目来源自主研发
技术领域计算机应用技术,人工智能与模式识别。
应用范围交通、广场、车站、会场等视频监控中人,车辆等目标的实时跟踪。
现状特点本系统的跟踪准确率>95%;在使用现有性能个人计算机的条件下,可以做到每秒30帧的实时跟踪,采用嵌入式技术固化跟踪算法可以做到复杂目标运动的实时跟踪。
所在阶段跟踪算法已在一些应用系统中试用,跟踪效果良好。
成果知识产权独立知识产权。
成果转让方式技术合作
市场状况及效益分析目前视频监控的基础设施已经普及使用到各种公共场所,特别是“十二五”期间国家实施的天网计划将进一步推动这些基础设施的普及,使得本项目减少了大量的基础实施投入。
另外由于具有广泛的应用前景,市场效益可观。
发生镜头切换情况的行人跟踪,传统跟踪方法此时将会跟踪失败。
语音识别中的关键词检测技术研究
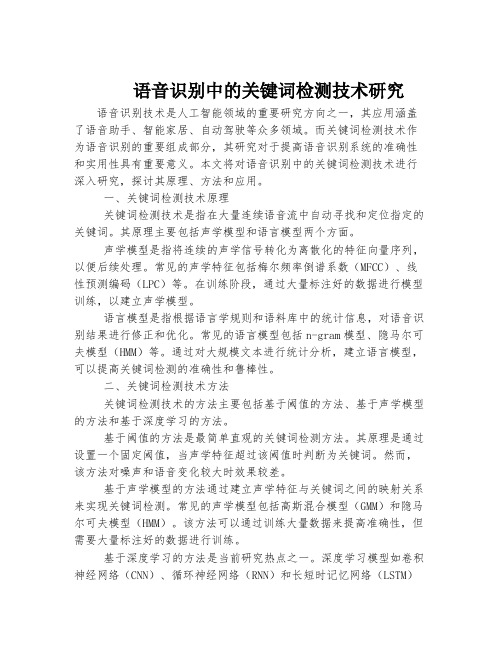
语音识别中的关键词检测技术研究语音识别技术是人工智能领域的重要研究方向之一,其应用涵盖了语音助手、智能家居、自动驾驶等众多领域。
而关键词检测技术作为语音识别的重要组成部分,其研究对于提高语音识别系统的准确性和实用性具有重要意义。
本文将对语音识别中的关键词检测技术进行深入研究,探讨其原理、方法和应用。
一、关键词检测技术原理关键词检测技术是指在大量连续语音流中自动寻找和定位指定的关键词。
其原理主要包括声学模型和语言模型两个方面。
声学模型是指将连续的声学信号转化为离散化的特征向量序列,以便后续处理。
常见的声学特征包括梅尔频率倒谱系数(MFCC)、线性预测编码(LPC)等。
在训练阶段,通过大量标注好的数据进行模型训练,以建立声学模型。
语言模型是指根据语言学规则和语料库中的统计信息,对语音识别结果进行修正和优化。
常见的语言模型包括n-gram模型、隐马尔可夫模型(HMM)等。
通过对大规模文本进行统计分析,建立语言模型,可以提高关键词检测的准确性和鲁棒性。
二、关键词检测技术方法关键词检测技术的方法主要包括基于阈值的方法、基于声学模型的方法和基于深度学习的方法。
基于阈值的方法是最简单直观的关键词检测方法。
其原理是通过设置一个固定阈值,当声学特征超过该阈值时判断为关键词。
然而,该方法对噪声和语音变化较大时效果较差。
基于声学模型的方法通过建立声学特征与关键词之间的映射关系来实现关键词检测。
常见的声学模型包括高斯混合模型(GMM)和隐马尔可夫模型(HMM)。
该方法可以通过训练大量数据来提高准确性,但需要大量标注好的数据进行训练。
基于深度学习的方法是当前研究热点之一。
深度学习模型如卷积神经网络(CNN)、循环神经网络(RNN)和长短时记忆网络(LSTM)等可以自动学习特征表示,从而提高关键词检测的准确性和鲁棒性。
该方法需要大量的训练数据,但相对于传统方法,其效果更好。
三、关键词检测技术应用关键词检测技术在语音识别领域有着广泛的应用。
人机交互知识:人机交互中的自然语言生成和识别
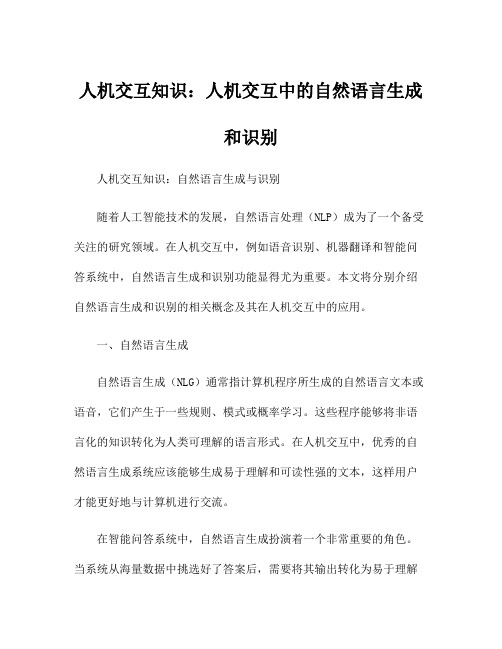
人机交互知识:人机交互中的自然语言生成和识别人机交互知识:自然语言生成与识别随着人工智能技术的发展,自然语言处理(NLP)成为了一个备受关注的研究领域。
在人机交互中,例如语音识别、机器翻译和智能问答系统中,自然语言生成和识别功能显得尤为重要。
本文将分别介绍自然语言生成和识别的相关概念及其在人机交互中的应用。
一、自然语言生成自然语言生成(NLG)通常指计算机程序所生成的自然语言文本或语音,它们产生于一些规则、模式或概率学习。
这些程序能够将非语言化的知识转化为人类可理解的语言形式。
在人机交互中,优秀的自然语言生成系统应该能够生成易于理解和可读性强的文本,这样用户才能更好地与计算机进行交流。
在智能问答系统中,自然语言生成扮演着一个非常重要的角色。
当系统从海量数据中挑选好了答案后,需要将其输出转化为易于理解的自然语言文本。
另外,在自然语言对话系统中,当计算机无法理解用户输入时,需要生成一些提示性的语句,用于引导用户再次输入。
此时自然语言生成系统的作用同样非常重要。
二、自然语言识别自然语言识别(NLI)通常指计算机程序识别和理解人类自然语言的能力。
这些程序能够较准确地解析文本、识别意图、实现查询等任务。
在人机交互中,自然语言识别系统扮演的作用非常重要,因为它是机器能够有效地理解和响应用户输入的前提。
自然语言识别领域的一个重要研究方向是情感分析。
情感分析是指在文本中自动识别情感以及评价,这对情感交互应用非常重要。
例如,在智能客服系统中,当用户表达了情感,自然语言识别系统便能准确理解并相应地进行回复。
三、自然语言生成和识别的应用自然语言生成和识别在人机交互中应用广泛。
例如,在语音识别领域,大多数语音助理(如Siri、Alexa和小爱同学等)都采用了自然语言识别技术,使得用户能够通过语音指令与智能设备进行交互。
另外,在智能问答系统及机器翻译领域,自然语言生成技术发挥着非常重要的作用。
自然语言识别也广泛应用在自然语言处理中的各个领域。
基于深度学习的多模态人体行为识别技术研究

基于深度学习的多模态人体行为识别技术研究在人工智能领域中,多模态人体行为识别技术是一项关键性的研究领域。
通过深度学习模型的引入,多模态人体行为识别技术正在取得令人鼓舞的进展。
本文将探讨基于深度学习的多模态人体行为识别技术的研究进展、应用领域以及挑战。
首先,我们需要明确什么是多模态人体行为识别。
在现实生活中,我们通过多个感官(如视觉、听觉、触觉等)来感知和理解他人的行为。
多模态人体行为识别技术旨在通过结合多种感知方式,如视频、声音、动作等,来准确地识别和理解人体的行为。
深度学习技术在多模态人体行为识别中的应用已经取得了显著的性能提升。
深度学习模型具有较强的表达能力和特征提取能力,能够从原始数据中学习到更高级别的抽象特征。
这一特性使得深度学习模型在多模态数据融合和行为识别方面具有优势。
在多模态人体行为识别中,最常用的深度学习模型之一是卷积神经网络(Convolutional Neural Network,CNN)。
卷积神经网络在图像处理方面具有卓越的性能,可以自动学习和提取图像特征。
通过将卷积神经网络与其他感知模态数据进行融合,可以更准确地识别人体行为。
另一个被广泛应用于多模态人体行为识别的深度学习模型是循环神经网络(Recurrent Neural Network,RNN)。
循环神经网络具有记忆能力,可以处理时序数据,如音频和视频。
通过利用循环神经网络的时间依赖性,可以更好地建模和识别人体的动作序列。
近年来,深度学习模型的不断发展使得多模态人体行为识别在许多应用领域取得了突破。
其中一个应用领域是安防监控。
通过多模态人体行为识别技术,可以实时监测并识别可疑行为,从而提高安全性和防范犯罪。
另外,多模态人体行为识别还可以应用于智能家居、健康监测等领域,为人们的生活带来便利和舒适。
然而,多模态人体行为识别技术仍面临一些挑战。
首先,多模态数据的采集和融合是一个复杂的问题。
不同感知模态的数据可能存在不同的时间和空间维度,如何有效地融合这些数据仍是一个待解决的问题。
基于神经网络的多特征轻度认知功能障碍检测模型
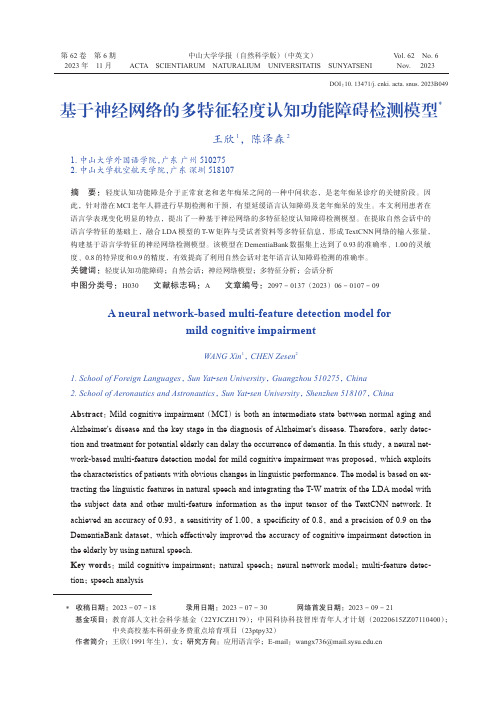
第 62 卷第 6 期2023 年11 月Vol.62 No.6Nov.2023中山大学学报(自然科学版)(中英文)ACTA SCIENTIARUM NATURALIUM UNIVERSITATIS SUNYATSENI基于神经网络的多特征轻度认知功能障碍检测模型*王欣1,陈泽森21. 中山大学外国语学院,广东广州 5102752. 中山大学航空航天学院,广东深圳 518107摘要:轻度认知功能障是介于正常衰老和老年痴呆之间的一种中间状态,是老年痴呆诊疗的关键阶段。
因此,针对潜在MCI老年人群进行早期检测和干预,有望延缓语言认知障碍及老年痴呆的发生。
本文利用患者在语言学表现变化明显的特点,提出了一种基于神经网络的多特征轻度认知障碍检测模型。
在提取自然会话中的语言学特征的基础上,融合LDA模型的T-W矩阵与受试者资料等多特征信息,形成TextCNN网络的输入张量,构建基于语言学特征的神经网络检测模型。
该模型在DementiaBank数据集上达到了0.93的准确率、1.00的灵敏度、0.8的特异度和0.9的精度,有效提高了利用自然会话对老年语言认知障碍检测的准确率。
关键词:轻度认知功能障碍;自然会话;神经网络模型;多特征分析;会话分析中图分类号:H030 文献标志码:A 文章编号:2097 - 0137(2023)06 - 0107 - 09A neural network-based multi-feature detection model formild cognitive impairmentWANG Xin1, CHEN Zesen21. School of Foreign Languages, Sun Yat-sen University, Guangzhou 510275, China2. School of Aeronautics and Astronautics, Sun Yat-sen University, Shenzhen 518107, ChinaAbstract:Mild cognitive impairment (MCI) is both an intermediate state between normal aging and Alzheimer's disease and the key stage in the diagnosis of Alzheimer's disease. Therefore, early detec‐tion and treatment for potential elderly can delay the occurrence of dementia. In this study, a neural net‐work-based multi-feature detection model for mild cognitive impairment was proposed, which exploits the characteristics of patients with obvious changes in linguistic performance. The model is based on ex‐tracting the linguistic features in natural speech and integrating the T-W matrix of the LDA model with the subject data and other multi-feature information as the input tensor of the TextCNN network. It achieved an accuracy of 0.93, a sensitivity of 1.00, a specificity of 0.8, and a precision of 0.9 on the DementiaBank dataset, which effectively improved the accuracy of cognitive impairment detection in the elderly by using natural speech.Key words:mild cognitive impairment; natural speech; neural network model; multi-feature detec‐tion; speech analysisDOI:10.13471/ki.acta.snus.2023B049*收稿日期:2023 − 07 − 18 录用日期:2023 − 07 − 30 网络首发日期:2023 − 09 − 21基金项目:教育部人文社会科学基金(22YJCZH179);中国科协科技智库青年人才计划(20220615ZZ07110400);中央高校基本科研业务费重点培育项目(23ptpy32)作者简介:王欣(1991年生),女;研究方向:应用语言学;E-mail:******************第 62 卷中山大学学报(自然科学版)(中英文)轻度认知障碍(MCI,mild cognitive impair‐ment)是一种神经系统慢性退行性疾病,也是阿尔茨海默病(AD,Alzheimer's disease)的早期关键阶段。
- 1、下载文档前请自行甄别文档内容的完整性,平台不提供额外的编辑、内容补充、找答案等附加服务。
- 2、"仅部分预览"的文档,不可在线预览部分如存在完整性等问题,可反馈申请退款(可完整预览的文档不适用该条件!)。
- 3、如文档侵犯您的权益,请联系客服反馈,我们会尽快为您处理(人工客服工作时间:9:00-18:30)。
Automatic Detection of Interaction GroupsOliver Brdiczka, Jérôme Maisonnasse, Patrick ReignierLaboratoire GRAVIRINRIA Rhône-Alpes655 Av. de l’Europe38330 Montbonnot, France.{brdiczka, maisonnasse, reignier}@inrialpes.frABSTRACTThis paper addresses the problem of detecting interaction groups in an intelligent environment. To understand human activity, we need to identify human actors and their interpersonal links. An interaction group can be seen as basic entity, within which individuals collaborate in order to achieve a common goal. In this regard, the dynamic change of interaction group configuration, i.e. the split and merge of interaction groups, can be seen as indicator of new activities. Our approach takes speech activity detection of individuals forming interaction groups as input. A classical HMM-based approach learning different HMM for the different group configurations did not produce promising results. We propose an approach for detecting interaction group configurations based on the assumption that conversational turn taking is synchronized inside groups. The proposed detector is based on one HMM constructed upon conversational hypotheses. The approach shows good results and thus confirms our conversational hypotheses.Categories and Subject DescriptorsI.2.10 [ARTIFICIAL INTELLIGENCE]: Vision and Scene Understanding - Perceptual reasoning.General TermsAlgorithms, Human Factors, Experimentation, Performance. KeywordsClustering interaction groups, Hidden Markov Model, speech detection, conversational analysis, intelligent environment, ubiquitous computing.1.INTRODUCTIONUbiquitous computing enables computer systems to sense and to respond to human activity. Human actors need to be identified in order to perceive correctly their activity. In intelligent environments more and more devices are capable of perceiving user activity and offering services to the user. Offering services means to supply a system reaction or an interaction at the most appropriate moment, aligned with the activity of the users. Addressing the right user at the correct moment is essential. Thus we need to detect potential users and their connection while doing an activity.The identification of the current group configuration of the users is necessary to analyze activity. In a physical environment, several individuals can form one group working on the same task, or they can split into subgroups doing independent tasks in parallel. The dynamics of group configuration, i.e. the split and merge of small interaction groups, allows us to perceive the appearing of new activities. We assume that a change in group configuration is strongly linked to a change in activity, at least to an interruption of the current activity. The fusion of several independent small groups is seen as important information for detecting a change of the current activity, on a local or global level. For example, people attending a seminar tend to form small groups discussing different topics before the seminar starts. When the lecturer arrives, these small groups merge and form a big group listing to the lecture. In this example, the fusion of several small groups to one big group can be used to detect the beginning of a seminar. In the same manner, the split of the big group into several small groups can indicate a pause or the end of the lecture. The change in group configuration is thus a strong indicator of new activities as well as of activities that are linked to a particular group configuration (for example a lecture).In this paper, we propose a method for the dynamic detection of small group configurations based on Hidden Markov Models. The method relies on speech activity detection as sensor information for interacting individuals. We focus thus on verbal interaction, which further implies a minimum size of two individuals for one group (assuming that isolated individuals do not speak). The method has been tested in experiments recording meetings of 4 individuals.2.PREVIOUS AND RELATED WORKThe recognition of human activity based on speech events is often used in the context of group analysis. In general, the group and its members are defined in advance. The objective is then to use frequency and duration of speech contributions to recognize particular key actions executed by group members [11] or to analyse the type of meeting in a global manner [3]. However, the detection of dependencies between individuals and their membership in one or several groups is not considered. AnalysingPermission to make digital or hard copies of all or part of this work for personal or classroom use is granted without fee provided that copies are not made or distributed for profit or commercial advantage and that copies bear this notice and the full citation on the first page. To copy otherwise, or republish, to post on servers or to redistribute to lists, requires prior specific permission and/or a fee.ICMI’05, October 4–6, 2005, Trento, Italy.Copyright 2005 ACM 1-59593-028-0/05/0010...$5.00.large amounts of data from recordings of interactions enables the reconstruction of social networks for a number of individuals [4]. The detection and analysis of conversations is then necessary. The automatic detection of conversations using mutual information [1], in order to determine who speaks and when, needs an important duration of each conversation.In this paper, we want to develop a real-time detector for interaction groups. This detector should be robust and as general as possible. The objective is to define the limits combining several individuals for doing the same intended activity. This activity is in most cases the main activity [8], provided that verbal interaction needs a certain level of attention. Note that the activity of a group of individuals can, in particular moments, attract the attention of other individuals, which means a short-term collective focusing on one activity [10]. Goffman calls this focusing “participation framework” [9]. Our objective is to visualize this dynamics in order to enable intelligent environments to use this information for the recognition of activity.Conversational analysis [13] and social psychology pointed out a certain number of important points concerning interpersonal interaction. Verbal interactions within a group are regulated [7], intentional and composed of successive conversations aiming at acting on a common ground [5] [6]. These statements will allow us to formulate conversational hypotheses as significant criteria for the detection of interaction groups.3.APPROACHWe present an approach based on Hidden Markov Models [12]. A Hidden Markov Model is a stochastic process where the evolution is managed by states. The series of states constitute a Markov chain which is not directly observable. This chain is “hidden”. Each state of the model generates an observation. Only the observations are visible. The objective is to derive the state sequence and its probability, given a particular sequence of observations. Hidden Markov Models have been used with success in speech recognition [14], sign language recognition [15], hand-writing gesture recognition [16] and many other domains. We use a HMM approach due to the dynamics of group split and merge as well as the noisy character of speech activity detection data.3.1Speech Activity DetectionThe observations used as input for the HMM are generated from speech activity data. An automatic speech detector [17] parses multi-channel audio input and detects which individual stops and starts speaking. The observations of the Hidden Markov Model are a discretization of speech activity events sent by this detector. One observation is a vector containing a binary value (speaking, not speaking) for each individual that is recorded. This vector is transformed to a 1-dimensional discrete code used as input for the HMM (see Table 1). The automatic speech detector has a sampling rate of 62.5 Hz, which corresponds to the generation of an observation vector every 16 milliseconds.Table 1. Observations of a Hidden Markov Model for ameeting of 4 individualsSpeech ActivityObservationNumber A B C D0 0 0 0 01 0 0 0 12 0 0 1 03 0 0 1 14 0 1 0 05 0 1 0 16 0 1 1 07 0 1 1 18 1 0 0 09 1 0 0 110 1 0 1 011 1 0 1 112 1 1 0 013 1 1 0 114 1 1 1 015 1 1 1 13.2Classification Using HMMsA first approach is to classify different group configurations using several HMMs. Each a priori group configuration class is associated with a Hidden Markov Model. The classification system is composed of n HMMs, where n corresponds to the number of possible group configurations for the recorded individuals. During a training stage, HMM parameters are estimated from a data set. This set is composed of several example concurrencies for each group configuration class. To classify, the probability that an unknown observation sequence was produced by the Hidden Markov Model is calculated using the forward-backward procedure [12]. The HMM with the highest probability for the given observation sequence determines the current group configuration.The number of states of the HMMs for the different group configurations is unknown a priori and needs to be fixed before training stage. K-means algorithm [12] is used for an initial training of the parameters of the HMMs, while Baum-Welch re-estimation formulas [12] are used for further training. Both algorithms are run with a fixed number of states for the HMM to train. To determine the optimal number of states for the HMMs, we varied the number of fixed states for the training. We evaluated the HMMs using audio recordings of two meetings of four individuals. Four HMMs for the four possible group configurations have been trained with different numbers of states. We did a cross-validation by training HMMs with the group configurations of the first meeting and classifying the group configurations of the second meeting and vice versa. Table 2 and 3 show the results.Table 2. Correct classification of group configurations ofMeeting 1 and Meeting 2 with HMMs trained on group configurations of Meeting 1 (training set: Meeting 1, test set:Meeting 2).States 1 2 4 8 Meeting 1 0.72 0.29 0.27 0.29 Meeting 2 0.51 0.23 0.23 0.19Table 3. Correct Classification of group configurations of Meeting 1 and Meeting 2 with HMMs trained on group configurations of Meeting 2 (training set: Meeting 2, test set:Meeting 1).States 1 2 4 8 Meeting 1 0.49 0.40 0.27 0.29 Meeting 2 0.77 0.35 0.26 0.21Classification results of this approach are not very promising. In particular, the optimal state number for both training sets when classifying test and training seems to be one state. This indicates that the sequential structure of the observations, i.e. who speaks after whom, is not discriminating for the different groupconfigurations. It is rather the distribution of the different observations, i.e. the number of interruptions, monologues of different participants, that seem to discriminate a group configuration. This is also due to the fact that we want to focus on short-term group configurations. In our meeting recordings, the duration of group configurations was between two and three minutes, which may not be sufficient for training and recognition of sequential speech patterns.3.3HMM based on Conversational HypothesesThe second approach is to construct a Hidden Markov Model based on conversational hypotheses. These conversational hypotheses are translated to probability distributions of the different observations generated by the states of the HMM. These states correspond to the different group configurations. We can use the Viterbi algorithm [12] to calculate the most probable state sequence matching a given observation sequence, i.e. a given sequence of speech detection. This state sequence corresponds to the sequence of group configurations that have been recognized for the given observations. In the following, we will detail the conversional hypotheses as well as the construction of the Hidden Markov Model.3.3.1Conversational HypothesesThis approach is based on basic conversational hypotheses. When two individuals are speaking at the same time, it is unlikely that they are in the same group. If two individuals do not speak, we can not decide their group membership. Finally, if one of the two individuals is speaking, it is likely that they are in the same group. We benefit from the regulation of speech activity, taking into account that verbal interaction are ordered within each group and disordered between different groups. As explained in Section 1, we assume that a group consists of at least two individuals.Figure 1. States of a HMM describing possible group configurations for a meeting of 4 individuals.3.3.2Construction of Hidden Markov ModelThe construction of the Hidden Markov Model relies on the estimation of different probability distributions for the observations depending on the group configuration. Each possible group configuration is represented by a state of the HMM. And each state has a probability distribution for the observations associated. This probability distribution is based on the conversational hypotheses. We assume that the probability that two individuals of the same group are speaking at the same time is low, while this probability is high when two individuals are not in the same group. When all individuals form one big group, the probability that one single individual speaks is high, while the probability of several individuals speaking in parallel is low. The transition probabilities between the states are set to a very low level in comparison to the probabilities to stay in the same state of the HMM. This is necessary to stabilize the detection of state changes as the frequency of incoming observations (speech activity events) is very high in comparison to the dynamics of group changes (16ms compared to circa 30 seconds). We assume hence that group changes occur in reasonable delays. Figure 1 shows the states of a HMM for 4 individuals.To detect the group configurations in real-time, we apply the Viterbi algorithm to the flow of arriving observations. Viterbi calculates the most probable state sequence that generated the observations. This state sequence corresponds to the sequence of detected group configurations. We calculate the state sequence for a window of the last 5000 arriving observation, which corresponds to an observationwindow of 80 seconds. The state calculated for the last observation represents the actual group configuration. The Viterbi algorithm is recalculated every 10 observations, which corresponds to a displacement of the window of 10 observations and an estimation of the group configuration every 160 milliseconds.4.EVALUATION AND RESULTSIn this section, we describe the evaluation of the approaches. We recorded the interactions of 4 individuals during 3 experiments. The number and order of group configurations, i.e. who will speak with whom, was fixed in advance for the experiments. TheLow transition probabilityexact timestamps and durations of the group configurations were, however, not predefined and changed spontaneously. The individuals were free to move and to discuss any topic.Figure 2. Picture of an example configuration of 2independent groups of 2 individuals.The speech of each individual was recorded using a lapel microphone. The speech activity detector [17] is executed on the audio channels of the different lapel microphones. We admit the use of lapel microphones in order to minimize correlation errors of speech activity of different individuals, i.e. speech of individual A is detected as speech of individual B. Figure 2 shows a picture of a configuration of 2 independent groups of 2 individuals during the experiments.Table 4. Confusion matrix for the 3 experiments Group Conf. (ABCD) (AB)(CD) (AC)(BD) (AD)(CB) (ABCD) 0.87535 0.02737 0.06245 0.03460(AB)(CD) 0.08535 0.86707 0.045490.00207 (AC)(BD) 0.21531 0.01057 0.77411 0.0 (AD)(BC) 0.039260.036290.079510.844924.1 ResultsTable 4 shows the confusion matrix for the 3 experiments. This matrix indicates for each group configuration the correct and wrong detections. The lines of the matrix contain the detection results, while the columns contain the expected response. We obtain a total recognition rate for the group configurations of 84.8 %. Figures 3, 4 and 5 give an overview of the detection of group configurations over time. The lines of the chart correspond to different group configurations. The continuous line indicates the correct group configuration expected as detection result.Figure 3. Group configuration detection during Experiment 1(duration: 9 min. 22 sec.).Figure 4. Group configuration detection during Experiment 2(duration: 15 min. 16 sec.).Figure 5. Group configuration detection during Experiment 3(duration: 16 min. 19 sec.).The results are encouraging and tend to validate the conversational hypotheses to distinguish interaction groups. The Viterbi algorithm executed on long observation sequences (like the observation window) is quite robust to wrong detections of the speech activity detector. However, a minimum number of correct speech activity detections is necessary, as the method relies on the information of who speaks at which moment. The use of lapel microphones made it possible to limit wrong detections as these microphones are attached to a particular person (and thus should only detect his/her speech).5. CONCLUSIONWe proposed a real-time detector for configurations of interaction groups. This detector is based on a HMM constructed upon conversational hypotheses. The input of the detector is a speech activity vector containing the information which individual is speaking or not. The synchronization of speech contributions within a group enables the detection of possible group configurations by a HMM built upon conversational hypotheses. The obtained results are encouraging, in particular as the group detection is exclusively based on speech activity, in presence of wrong speech activity detections.The integration of further information into the model will be an important aspect of future research. Speech activity detection is not sufficient to disambiguate all situations, in particular to detect isolated individuals. Further information like head orientation and interpersonal distances seem to be good indicators [2]. Thus a multimodal approach needs to be envisaged.Further audio recordings need to be done in order to validate and refine the conversational hypotheses. A big amount of representative conversational data will enable learning and adjustment of the probabilities of the HMM, which may improve its general performance. In addition, enough conversational data representing a specific context may permit to adapt the detector toa particular context to improve its performance in this context.6.REFERENCES[1]Basu S. Conversational Scene Analysis. Ph.D. Thesis. MITDepartment of EECS. September, 2002.[2]Beattie, G. The regulation of speaker turns in face-to-faceconversation;some implications for conversation in sound-only communication channels, Semiotica, 34, 55-70, 1981 [3]Burger, S., MacLaren, V., and Yu, H., The ISL MeetingCorpus: The Impact of Meeting Type on Speech Style, Proc.of ICSLP 2002, Denver, CO, USA, 2002.[4]Choudhury, T. and Pentland, A.,Characterizing SocialInteractions Using the Sociometer, Proceedings of NAACOS 2004, June 2004[5]Clark H. Using Language. Cambridge University Press,1996.[6]Clark H., Schaefer E., F. Contributing to Discourse,Cognitive Science 13, 259-294, 1989.[7]Duncan, S., Some signals and rules for taking speaking turnsin conversation, Journal of Personality and SocialPsychology, 23(2), 283-293, 1972.[8]Goffman, E. Frame analysis. Harper Row, New York, 1974. [9]Goffman, E. Footing. Forms of Talk, 124-159, University ofPennsylvania Press, Philadelphia, 1981.[10]Joseph, I., Attention distribuée, attention focalisée. Lesprotocoles de la coopération au PCC de la ligne A du RER.Sociologie du Travail, 4/94, 563-587, 1994.[11]McCowan, I., Gatica-Perez, D., Bengio, S., Lathoud, G.,Barnard, M., and Zhang, D.,Automatic Analysis ofMultimodal Group Actions in Meetings, IEEE Transactionson Pattern Analysis and Machine Intelligence, 2004. [12]Rabiner, L. A tutorial on Hidden Markov Models andselected applications in speech recognition, Proc. IEEE77(2):257-286, 1989.[13]Sacks, H., Schegloff, E., and Jefferson, G. A simplestsystematics for the organization of turn-taking forconversation. Language, 50, 696-735, 1974.[14]Huang, X.D., Ariki, Y., and Jack, M.A. Hidden MarkovModels for Speech Recognition. Edinburgh University Press, 1990.[15]Starner, T.E. Visual Recognition of American Sign LanguageUsing Hidden Markov Model. PhD Thesis, MIT, MediaLaboratory, Perceptual Computing Section, 1995.[16]Martin, J., and Durand, J.-B. Automatic HandwritingGestures Recognition Using Hidden Markov Models. FG2000: 403-409.[17]Vaufreydaz, D., IST-2000-28323 FAME: Facilitating Agentfor Multi-Cultural Exchange (WP4), European Commission project IST-2000-28323 October 2001.。