On the Generalization Capability of Multi-Layered Networks in the Extraction of Speech Prop
驳论文作文
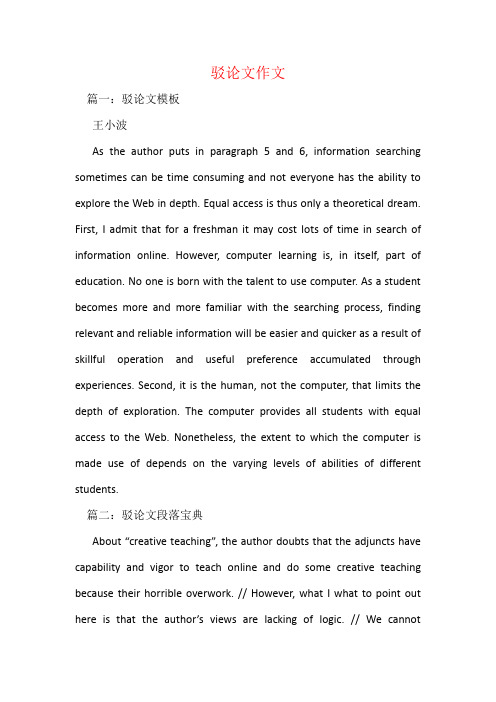
驳论文作文篇一:驳论文模板王小波As the author puts in paragraph 5 and 6, information searching sometimes can be time consuming and not everyone has the ability to explore the Web in depth. Equal access is thus only a theoretical dream. First, I admit that for a freshman it may cost lots of time in search of information online. However, computer learning is, in itself, part of education. No one is born with the talent to use computer. As a student becomes more and more familiar with the searching process, finding relevant and reliable information will be easier and quicker as a result of skillful operation and useful preference accumulated through experiences. Second, it is the human, not the computer, that limits the depth of exploration. The computer provides all students with equal access to the Web. Nonetheless, the extent to which the computer is made use of depends on the varying levels of abilities of different students.篇二:驳论文段落宝典About “creative teaching”, the author doubts that the adjuncts have capability and vigor to teach online and do some creative teaching because their horrible overwork. // However, what I what to point out here is that the author’s views are lacking of logic. // We cannotdefinitely say that adjuncts who have heavy teaching load are unlikely to be doing any “creative teaching”. adjuncts, who mainly focus on teaching and usually acquire rich classroom experience, are in a better position to know how to pass on knowledge efficiently and creatively. // In fact, almost all students highly praise the creativity and vigor demonstrated by teachers in the New Oriental, a well-known private language training school in China, where teachers usually have pretty heavy workloads.篇三:作文驳论文的常犯错误Fallacies of DistractionFalse Dilemma: two choices are given when in fact there are three optionsFrom Ignorance: because something is not known to be true, it is assumed to be falseSlippery Slope: a series of increasingly unacceptable consequences is drawnComplex Question: two uelated points are conjoined as a single propositionAppeals to Motives in Place of SupportAppeal to Force: the reader is persuaded to agree by forceAppeal to Pity: the reader is persuaded to agree by sympathyConsequences: the reader is warned of unacceptable consequences Prejudicial Language: value or moral goodness is attached tobelieving the authorPopularity: a proposition is argued to be true because it is widely held to be trueChanging the SubjectAttacking the Person:(1) the persons character is attacked(2) the persons circumstances are noted(3) the person does not practise what is preachedAppeal to Authority:(1) the authority is not an expert in the field(2) experts in the field disagree(3) the authority was joking, drunk, or in some other way not being seriousAnonymous Authority: the authority in question is not namedStyle Over Substance: the manner in which an argument (or arguer) is presented is felt to affect the truth of the conclusionInductive FallaciesHasty Generalization: the sample is too small to support an inductive generalization about a populationUepresentative Sample: the sample is uepresentative of the sample as a wholeFalse Analogy: the two objects or events being compared arerelevantly dissimilarSlothful Induction: the conclusion of a strong inductive argument is denied despite the evidence to thecontraryFallacy of Exclusion: evidence which would change the outcome of an inductive argument is excluded from considerationFallacies Involving Statistical SyllogismsAccident: a generalization is applied when circumstances suggest that there should be an exceptionConverse Accident : an exception is applied in circumstances where a generalization should applyCausal FallaciesPost Hoc: because one thing follows another, it is held to cause the otherJoint effect: one thing is held to cause another when in fact they are both the jointeffects of an underlying causeInsignificant: one thing is held to cause another, and it does, but it is insignificant compared to other causes of the effectWrong Direction: the direction between cause and effect is reversed Complex Cause: the cause identified is only a part of the entire cause of the effectMissing the PointBegging the Question: the truth of the conclusion is assumed by the premisesIrrelevant Conclusion: an argument in defense of one conclusion instead proves a different conclusionStraw Man: the author attacks an argument different from (and weaker than) the oppositions best argumentFallacies of AmbiguityEquivocation: the same term is used with two different meaningsAmphiboly: the structure of a sentence allows two different interpretationsAccent: the emphasis on a word or phrase suggests a meaning contrary to what the sentence actually saysCategory ErrorsComposition: because the attributes of the parts of a whole have a certain property, it is argued that the whole has that property Division: because the whole has a certain property, it is argued that the parts have that property Non SequiturAffirming the Consequent: any argument of the form: If A then B, B, therefore ADenying the Antecedent: any argument of the form: If A then B, Not A, thus Not BInconsistency: asserting that contrary or contradictory statements are both trueSyllogistic ErrorsFallacy of Four Terms: a syllogism has four termsUndistributed Middle: two separate categories are said to be connected because they share a common propertyIllicit Major: the predicate of the conclusion talks about all of something, but the premises only mention some cases of the term in the predicateIllicit Minor: the subject of the conclusion talks about all of something, but the premises only mention some cases of the term in the subjectFallacy of Exclusive Premises: a syllogism has two negative premises Fallacy of Drawing an Affirmative Conclusion From a Negative Premise: as the name impliesExistential Fallacy: a particular conclusion is drawn from universal premisesFallacies of ExplanationSubverted Support (The phenomenon being explained doesnt exist) Non-support (Evidence for the phenomenon being explained is biased)Untestability (The theory which explains cannot be tested)Limited Scope (The theory which explains can only explain one thing) Limited Depth (The theory which explains does not appeal to underlying causes)Fallacies of DefinitionToo Broad (The definition includes items which should not be included)Too Narrow (The definition does not include all the items which shouls be included)Failure to Elucidate (The definition is more difficult to understand than the word orconcept being defined)Circular Definition (The definition includes the term being defined as a part of the definition)Conflicting Conditions (The definition is self-contradictory)References。
点云边缘检测算法
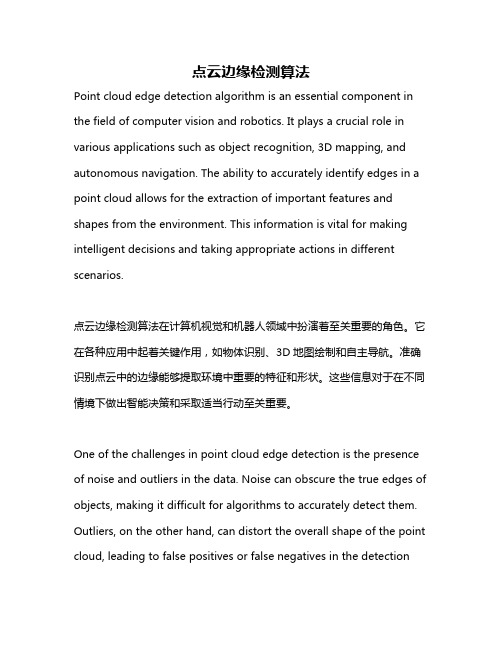
点云边缘检测算法Point cloud edge detection algorithm is an essential component in the field of computer vision and robotics. It plays a crucial role in various applications such as object recognition, 3D mapping, and autonomous navigation. The ability to accurately identify edges in a point cloud allows for the extraction of important features and shapes from the environment. This information is vital for making intelligent decisions and taking appropriate actions in different scenarios.点云边缘检测算法在计算机视觉和机器人领域中扮演着至关重要的角色。
它在各种应用中起着关键作用,如物体识别、3D地图绘制和自主导航。
准确识别点云中的边缘能够提取环境中重要的特征和形状。
这些信息对于在不同情境下做出智能决策和采取适当行动至关重要。
One of the challenges in point cloud edge detection is the presence of noise and outliers in the data. Noise can obscure the true edges of objects, making it difficult for algorithms to accurately detect them. Outliers, on the other hand, can distort the overall shape of the point cloud, leading to false positives or false negatives in the detectionprocess. Dealing with noise and outliers is a critical aspect of developing robust edge detection algorithms that can perform well in real-world scenarios.点云边缘检测中的一个挑战是数据中存在噪声和异常值。
人工通用智能概述_英文_
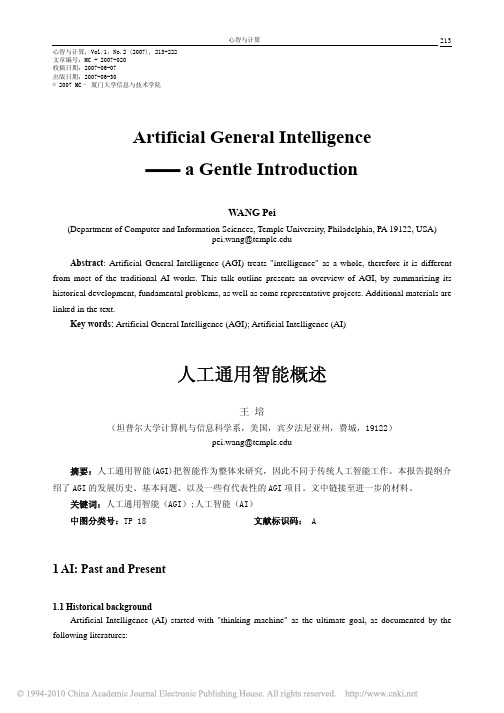
213
Artificial General Intelligence a Gentle Introduction
2.2 Fundamental AI/AGI questions At the top level, theoretical questions every AI (AGI) researcher needs to answer include: (1) What is AI, accurately specified? (2) Is it possible to build the AI as specified? (3) If AI is possible, what is the most efficient way to achieve it? (4) Even if we know how to achieve AI, should we really do it? Most AI (AGI) researchers answer "Yes" to the 2nd and 4th questions, though some outside people say "No" to one of them. In the following we will compare the different answers to the 1st and 3rd questions, that is, on the research goal and technical strategy of AI, respectively.
特征选择方法综述
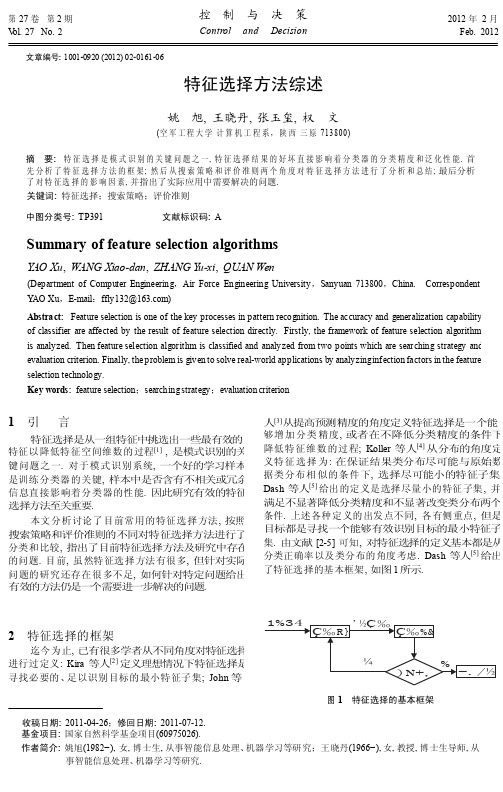
控 制 与 决 策第 27 卷 第 2 期 V ol. 27 No. 22012 年 2 月Feb. 2012Control andDecision文章编号: 1001-0920 (2012) 02-0161-06特征选择方法综述姚 旭, 王晓丹, 张玉玺, 权 文(空军工程大学 计算机工程系,陕西 三原 713800)摘 要: 特征选择是模式识别的关键问题之一, 特征选择结果的好坏直接影响着分类器的分类精度和泛化性能. 首 先分析了特征选择方法的框架; 然后从搜索策略和评价准则两个角度对特征选择方法进行了分析和总结; 最后分析 了对特征选择的影响因素, 并指出了实际应用中需要解决的问题. 关键词: 特征选择;搜索策略;评价准则 中图分类号: TP391文献标识码: ASummary of feature selection algorithmsYAO Xu , WANG Xiao-dan , ZHANG Y u-xi , QUAN Wen(Department of Computer Engineering ,Air Force Engineering University ,Sanyuan 713800,China. Correspondent: Y AO Xu ,E-mail :***************)Abstract: Feature selection is one of the key processes in pattern recognition. The accuracy and generalization capability of classifier are affected by the result of feature selection directly. Firstly, the framework of feature selection algorithm is analyzed. Then feature selection algorithm is classified and analyzed from two points which are searching strategy and evaluation criterion. Finally, the problem is given to solve real-world applications by analyzing infection factors in the feature selection technology.Key words: feature selection ;searching strategy ;evaluation criterion引言1 人[3] 从提高预测精度的角度定义特征选择是一个能 够增加分类精度, 或者在不降低分类精度的条件下 降低特征维数的过程; Koller 等人[4] 从分布的角度定 义特征选择为: 在保证结果类分布尽可能与原始数 据类分布相似的条件下, 选择尽可能小的特征子集; Dash 等人[5] 给出的定义是选择尽量小的特征子集, 并 满足不显著降低分类精度和不显著改变类分布两个 条件. 上述各种定义的出发点不同, 各有侧重点, 但是 目标都是寻找一个能够有效识别目标的最小特征子 集. 由文献 [2-5] 可知, 对特征选择的定义基本都是从 分类正确率以及类分布的角度考虑. Dash 等人[5] 给出 了特征选择的基本框架, 如图 1 所示.特征选择是从一组特征中挑选出一些最有效的 特征以降低特征空间维数的过程[1] , 是模式识别的关 键问题之一. 对于模式识别系统, 一个好的学习样本 是训练分类器的关键, 样本中是否含有不相关或冗余 信息直接影响着分类器的性能. 因此研究有效的特征 选择方法至关重要.本文分析讨论了目前常用的特征选择方法, 按照 搜索策略和评价准则的不同对特征选择方法进行了 分类和比较, 指出了目前特征选择方法及研究中存在 的问题. 目前, 虽然特征选择方法有很多, 但针对实际 问题的研究还存在很多不足, 如何针对特定问题给出 有效的方法仍是一个需要进一步解决的问题.特征选择的框架迄今为止, 已有很多学者从不同角度对特征选择进行过定义: Kira 等人[2] 定义理想情况下特征选择是 寻找必要的、足以识别目标的最小特征子集; John 等 2 图 1 特征选择的基本框架收稿日期: 2011-04-26;修回日期: 2011-07-12.基金项目: 国家自然科学基金项目(60975026).作者简介: 姚旭(1982−), 女, 博士生, 从事智能信息处理、机器学习等研究;王晓丹(1966−), 女, 教授, 博士生导师, 从事智能信息处理、机器学习等研究.由于子集搜索是一个比较费时的步骤, Y u 等 人[6]基于相关和冗余分析, 给出了另一种特征选择框 架, 避免了子集搜索, 可以高效快速地寻找最优子集. 框架如图 2 所示.间远远小于 (2N ).存在的问题: 具有较高的不确定性, 只有当总循 环次数较大时, 才可能找到较好的结果. 在随机搜索 策略中, 可能需对一些参数进行设置, 参数选择的合 适与否对最终结果的好坏起着很大的作用. 因此, 参 数选择是一个关键步骤.3.3 采用启发式搜索策略的特征选择方法这类特征选择方法主要有: 单独最优特征组合, 序列前向选择方法 (SFS), 广义序列前向选择方法 (GSFS), 序列后向选择方法 (SBS), 广义序列后向选择 方法 (GSBS), 增 l 去 选择方法, 广义增 l 去 选择方 法, 浮动搜索方法. 这类方法易于实现且快速, 它的搜 索空间是 (N 2 ). 一般认为采用浮动广义后向选择方 法 (FGSBS) 是较为有利于实际应用的一种特征选择 搜索策略, 它既考虑到特征之间的统计相关性, 又用 浮动方法保证算法运行的快速稳定性[13] . 存在的问 题是: 启发式搜索策略虽然效率高, 但是它以牺牲全 局最优为代价.每种搜索策略都有各自的优缺点, 在实际应用过 程中, 可以根据具体环境和准则函数来寻找一个最佳 的平衡点. 例如, 如果特征数较少, 可采用全局最优搜 索策略; 若不要求全局最优, 但要求计算速度快, 则可 采用启发式策略; 若需要高性能的子集, 而不介意计 算时间, 则可采用随机搜索策略.图 2 改进的特征选择框架从特征选择的基本框架可以看出, 特征选择方法中有 4 个基本步骤: 候选特征子集的生成 (搜索策 略)、评价准则、停止准则和验证方法[7-8] . 目前对特征 选择方法的研究主要集中于搜索策略和评价准则, 因 而, 本文从搜索策略和评价准则两个角度对特征选择 方法进行分类.基于搜索策略划分特征选择方法基本的搜索策略按照特征子集的形成过程可分 为以下 3 种: 全局最优、随机搜索和启发式搜索[9] . 一 个具体的搜索算法会采用两种或多种基本搜索策略, 例如遗传算法是一种随机搜索算法, 同时也是一种启 发式搜索算法. 下面对 3 种基本的搜索策略进行分析 比较.3.1 采用全局最优搜索策略的特征选择方法 迄今为止, 唯一得到最优结果的搜索方法是分支 定界法[10] . 这种算法能保证在事先确定优化特征子 集中特征数目的情况下, 找到相对于所设计的可分 性判据而言的最优子集. 它的搜索空间是 (2N ) (其 中 N 为特征的维数). 存在的问题: 很难确定优化特征 子集的数目; 满足单调性的可分性判据难以设计; 处 理高维多类问题时, 算法的时间复杂度较高. 因此, 虽 然全局最优搜索策略能得到最优解, 但因为诸多因素 限制, 无法被广泛应用.3.2 采用随机搜索策略的特征选择方法在计算过程中把特征选择问题与模拟退火算 法、禁忌搜索算法、遗传算法等, 或者仅仅是一个随 机重采样[11-12] 过程结合起来, 以概率推理和采样过程 作为算法的基础, 基于对分类估计的有效性, 在算法 运行中对每个特征赋予一定的权重; 然后根据用户所 定义的或自适应的阈值来对特征重要性进行评价. 当 特征所对应的权重超出了这个阈值, 它便被选中作为 重要的特征来训练分类器. Relief 系列算法即是一种 典型的根据权重选择特征的随机搜索方法, 它能有效 地去掉无关特征, 但不能去除冗余, 而且只能用于两 类分类. 随机方法可以细分为完全随机方法和概率随 机方法两种. 虽然搜索空间仍为 (2N ), 但是可以通 过设置最大迭代次数限制搜索空间小于 (2N ). 例如 遗传算法, 由于采用了启发式搜索策略, 它的搜索空3 基于评价准则划分特征选择方法特征选择方法依据是否独立于后续的学习算 法, 可分为过滤式 (Filter) 和封装式 (Wrapper)[14] 两种. Filter 与后续学习算法无关, 一般直接利用所有训练 数据的统计性能评估特征, 速度快, 但评估与后续学 习算法的性能偏差较大. Wrapper 利用后续学习算法 的训练准确率评估特征子集, 偏差小, 计算量大, 不适 合大数据集. 下面分别对 Filter 和 Wrapper 方法进行 分析.4.1 过滤式 (Filter) 评价策略的特征选择方法Filter 特征选择方法一般使用评价准则来增强特 征与类的相关性, 削减特征之间的相关性. 可将评价 函数分成 4 类[5] : 距离度量、信息度量、依赖性度量以 及一致性度量.4.1.1 距离度量 距离度量通常也认为是分离性、差异性或者辨4 识能力的度量. 最为常用的一些重要距离测度 有[1] 欧氏距离、 阶 Minkowski 测度、Chebychev 距离、平 方距离等. 两类分类问题中, 对于特征 X 和 Y , 如果 由 X 引起的两类条件概率差异性大于 Y , 则 X 优于 Y . 因为特征选择的目的是找到使两类尽可能分离的姚 旭 等: 特征选择方法综述 第2 期 163特征. 如果差异性为 0, 则 X 与 Y 是不可区分的. 算法 Relief [2] 及其变种 ReliefF [15] , 分支定界 和 BFF [16] 等都 是基于距离度量的. 准则函数要求满足单调性, 也可 通过引进近似单调的概念放松单调性的标准. 蔡哲元 等人[17] 提出了基于核空间的距离度量, 有效地提高了 小样本与线性不可分数据集上的特征选择能力. 4.1.2 信息度量信息度量通常采用信息增益 (IG) 或互信息 (MI) 衡量. 信息增益定义为先验不确定性与期望的后验不 确定性之间的差异, 它能有效地选出关键特征, 剔除 无关特征[18] . 互信息描述的是两个随机变量之间相 互依存关系的强弱. 信息度量函数 (f ) 在 Filter 特征 选择方法中起着重要的作用. 尽管 (f ) 有多种不同 形式, 但是目的是相同的, 即使得所选择的特征子集 与类别的相关性最大, 子集中特征之间的相关性最小. 刘华文[19] 给出了一种泛化的信息标准, 准则如下:互信息的评价准则, 具体函数如下:1 ∑(f ) = (C ; f ) −(; f ), (4)∣∣s ∈S 其中 ∣∣ 表示已选特征的个数. 该算法的思想就是最 大化特征子集和类别的相关性, 同时最小化特征之间 的冗余. Peng 用这种方法将多变量联合概率密度估计 问题转化为多重二变量概率密度估计, 解决了一大难 题. Ding 等人[23] 还给出了此算法的一种变种形式, 将 准则函数中的减法改为除法, 即(C ; f )(f ) = .(5)1 ∑ s ∈S (; f )∣∣4) FCBF (fast correlation-based filter)[6] 是基于相 互关系度量给出的一种算法. 对于线性随机变量, 用 相关系数分析特征与类别、特征间的相互关系. 对于 非线性随机变量, 采用对称不确定性 (SU) 来度量. 对 于两个非线性随机变量 X 和 Y , 它们的相互关系可表 示为(f ) = α ⋅ (, , ) − . (1) [ (X ∣Y )]其中: C 为类别, f 为候选特征, 为已选择的特征, 函数 (, , ) 为 , , 之间的信息量; α 为调控系数,δ 为惩罚因子. 下面就此信息标准的泛化形式与几个 现有选择算法中的信息度量标准之间的关系进行讨 论:1) BIF (best individual feature)[20] 是一种最简单最 直接的特征选择方法. 它的评价函数为B (, Y ) = 2 .(6) (X ) + (Y ) 其中: (X ) 与 (Y ) 为信息熵, (X ∣Y ) 为信息增益. 该算法的基本思想是根据所定义的 C - 相关 (特征与类别的相互关系) 和 - 相关 (特征之间的相互关 系), 从原始特征集合中去除 C - 相关值小于给定阈值 的特征, 再对剩余的特征进行冗余分析.5) CMIM (conditional mutual information maxi-mization). 有些特征选择方法利用条件互信息来评价特征的重要性程度, 即在已知已选特征集 的情况下通过候选特征 f 与类别 C 的依赖程度来确定 f 的重要性, 其中条件互信息 (C ; f ∣) 值越大, f 能提供的新信息越多. 因为 (C ; f ∣) 计算费用较高, 且样本的多维性导致了其估值不准确, Fleuret [24] 在提出的条件互信息最大化选择算法 CMIM 中采取一种变 通的方式, 即使用单个已选特征 来代替整个已选子集 以估算 (C ; f ∣), 其中 是使 (C ; f ∣) 值最大的 已选特征. CMIM 的评价函数为(2) (f ) = (C ; f ),其中 ( ) 为互信息, (C ; f ) 为类别 C 与候选特征 f 之间的互信息. 它的基本思想是对于每一个候选特征 f 计算评价函数 (f ), 并按评价函数值降序排列, 取 前 k 个作为所选择的特征子集. 这种方法简单快速, 尤其适合于高维数据. 但是它没有考虑到所选特征间 的相关性, 会带来较大的冗余.2) MIFS (mutual information feature selection) 为 基于上述算法的缺点, 由 Battiti [21] 给出的一种使用候 选特征 f 与单个已选特征 相关性对 f 进行惩罚的方 法, 其评价函数为(f ) = arg min (C ; f∣).(7) s ∈S(f ) = (C ; f ) − β ∑(;(3)除以上几种信息度量和算法外, 针对存在的问 题, 研究者们提出了新的评价函数和算法. Kwak 等 人[25] 指出 MIFS 算法中评价函数 ( ) 的惩罚因子并 不能准确地表达冗余程度的增长量, 给出了 MIFS- U (MIFS-uncertainty) 算法; 与 MIFS 算法类似, MIFS- U 算法中参数 β 的取值将影响选择算法的性能. 为 了解决该问题, Novovicova 等人[26] 提出了 MIFS-U 的 一种改进算法 mMIFS-U (modified version of MIFS-U), 算法中将 f 与 中单个已选特征相关程度最大的 作 为它们之间的冗余程度; 为了解决对称不确定性可能s ∈S其中 β 为调节系数, 当 β ∈ [0.5, 1] 时, 算法性能较好. 3) mRMR (minimal-redundancy and maximal-relevance) [22] 方法. 从理论上分析了 mRMR 等价于 最大依赖性, 并分析了三者的关系. 基于最大依赖性, 可通过计算不同特征子集与类别的互信息来选取最 优子集. 但是, 在高维空间中, 估计多维概率密度是一 个难点. 另一个缺点是计算速度非常慢. 所以本文从 与其等价的最小冗余和最大相关出发, 给出一种基于提供一些错误或不确定信息, Qu 等人[27] 利用决策依赖相关性来精确度量特征f与间的依赖程度, 提出了DDC (decision dependent correlation) 算法. 它们的思想都是一致的, 只是评价函数的表达形式不同. 刘华文[19] 还提出了一种基于动态互信息的特征选择方法. 随着已选特征数的增加, 类别的不确定性也逐渐降低, 无法识别的样本数也越来越少. 因此, 已识别的样本会给特征带来干扰信息, 可采用动态互信息作为评价标准, 在特征选择过程中不断地删除已识别的样本, 使得评价标准在未识别样本上动态估值.基于信息的度量是近年来的一个研究热点, 出现了大量基于信息熵的特征选择方法, 如文献[28-31] 等. 因为信息熵理论不要求假定数据分布是已知的, 能够以量化的形式度量特征间的不确定程度, 并且能有效地度量特征间的非线性关系. 因此, 信息度量被广泛应用, 并且也通过试验证明了其性能[32-34] . 以上基于信息度量的评价准则虽然形式不同, 但是核心思想都是找出与类别相关性最大的特征子集, 并且该子集中特征之间的相关性最小. 设计体现这一思想的函数是至关重要的.4.1.3 依赖性度量有许多统计相关系数, 如Pearson 相关系数、概率误差、Fisher 分数、线性可判定分析、最小平方回归误差[35] 、平方关联系数[36] 、-test 和F-Statistic 等被用来表达特征相对于类别可分离性间的重要性程度. 例如, Ding[23] 和Peng[22] 在mRMR 中处理连续特征时, 分别使用F-Statistic 和Pearson 相关系数度量特征与类别和已选特征间的相关性程度. Hall[37] 给出一种既考虑了特征的类区分能力, 同时又考虑特征间冗余性的相关性度量标准. Zhang 等人[38] 使用成对约束即must-link 约束和cannot-link 约束计算特征的权重, 其中must-link 约束表示两个样本离得很近, 而cannot-link 表示样本间离得足够远.在依赖性度量中, Hilbert-Schmidt 依赖性准则(HSIC) 可作为一个评价准则度量特征与类别的相关性. 核心思想是一个好的特征应该最大化这个相关性. 特征选择问题可以看成组合最优化问题性准则用不一致率来度量, 它不是最大化类的可分离性, 而是试图保留原始特征的辨识能力, 即找到与全集有同样区分类别能力的最小子集. 它具有单调、快速、去除冗余和不相关特征、处理噪声等优点, 能获得一个较小的特征子集. 但其对噪声数据敏感, 且只适合离散特征. 典型算法有Focus[41] , LVF[42] 等. 文献[43-44] 给出了基于不一致度量的算法.上面分析了Filter 方法中的一些准则函数, 选择合适的准则函数将会得到较好的分类结果. 但Filter 方法也存在很多问题: 它并不能保证选择出一个优化特征子集, 尤其是当特征和分类器息息相关时. 因而, 即使能找到一个满足条件的优化子集, 它的规模也会比较庞大, 会包含一些明显的噪声特征. 但是它的一个明显优势在于可以很快地排除很大数量的非关键性的噪声特征, 缩小优化特征子集搜索的规模, 计算效率高, 通用性好, 可用作特征的预筛选器.4.2 封装式(Wrapper) 评价策略的特征选择方法除了上述4 种准则, 分类错误率也是一种衡量所选特征子集优劣的度量标准. Wrapper 模型将特征选择算法作为学习算法的一个组成部分, 并且直接使用分类性能作为特征重要性程度的评价标准. 它的依据是选择子集最终被用于构造分类模型. 因此, 若在构造分类模型时, 直接采用那些能取得较高分类性能的特征即可, 从而获得一个分类性能较高的分类模型. 该方法在速度上要比Filter 方法慢, 但是它所选择的优化特征子集的规模相对要小得多, 非常有利于关键特征的辨识; 同时它的准确率比较高, 但泛化能力比较差, 时间复杂度较高. 目前此类方法是特征选择研究领域的热点, 相关文献也很多. 例如, Hsu 等人[45] 用决策树来进行特征选择, 采用遗传算法来寻找使得决策树分类错误率最小的一组特征子集. Chiang 等人[46] 将Fisher 判别分析与遗传算法相结合, 用来在化工故障过程中辨识关键变量, 取得了不错的效果. Guyon 等人[47] 使用支持向量机的分类性能衡量特征的重要性程度, 并最终构造一个分类性能较高的分类器. Krzysztof [48] 提出了一种基于相互关系的双重策略的Wrapper 特征选择方法. 叶吉祥等人[49] 提出了一种快速的Wrapper 特征选择方法FFSR(fast feature subset ranking), 以特征子集作为评价单位, 以子集收敛能力作为评价标准. 戴平等人[50] 利用SVM 线性核与多项式核函数的特性, 结合二进制PSO 方法, 提出了一种基于SVM 的快速特征选择方法.综上所述, Filter 和Wrapper 特征选择方法各有优缺点. 将启发式搜索策略和分类器性能评价准则相结合来评价所选的特征, 相对于使用随机搜索策略的方法, 节约了不少时间. Filter 和Wrapper 是两种(8)= arg max (), s.t.∣∣⩽.⊆F其中: 为所选特征个数的上限, 为特征集合,为已选特征的集合, () 为评价准则. 从式(8) 中可知需要解决两个问题: 一是评价准则() 的选择; 二是算法的选择. 文献[39-40] 是HSIC 准则的具体应用.4.1.4 一致性度量给定两个样本, 若他们特征值均相同, 但所属类别不同, 则称它们是不一致的; 否则, 是一致的. 一致姚 旭 等: 特征选择方法综述第2 期 165互补的模式, 两者可以结合. 混合特征选择过程一般 由两个阶段组成, 首先使用 Filter 方法初步剔除大部 分无关或噪声特征, 只保留少量特征, 从而有效地减 小后续搜索过程的规模. 第 2 阶段将剩余的特征连 同样本数据作为输入参数传递给 Wrapper 选择方法, 以进一步优化选择重要的特征. 例如, 文献 [51] 采用 混合模型选择特征子集, 先使用互信息度量标准和 bootstrap 技术获取前 k 个重要的特征, 然后再使用支 持向量机构造分类器.292.Manoranjan Dash, Huan Liu. Feature selection forclassification[J]. Intelligent Data Analysis, 1997, 1(3): 131-156.Lei Y u, Huan Liu. Efficient feature selection via analysisof relevance and redundancy[J]. J of Machine Learnin gResearch, 2004, 5(1): 1205-1224.Liu H, Motoda H. Feature selection for knowledgediscovery and data mining[M]. Boston: Kluwer AcademicPublishers, 1998.Molina L C, Llu´ıs Belanche, A` ngela Nebot. Feature [5] [6] [7] 结论5 [8] 本文首先分析了特征选择的框架, 然后从两个角度对特征选择方法进行分类: 一个是搜索策略, 一个 是评价准则. 特征选择方法从研究之初到现在, 已经 有了很多成熟的方法, 但是, 研究过程中也存在很多 问题. 例如: 如何解决高维特征选择问题; 如何设计小 样本问题的特征选择方法; 如何针对不同问题设计特 定的特征选择方法; 研究针对新数据类型的特征选 择方法等. 影响特征选择方法的因素主要有数据类 型、样本数量. 针对两类还是多类问题, 特征选择方 法的选择也有不同. 例如 Koll-Saha [4] 和 Focus 等人[41] 受限于连续型特征; 分支定界, BFF [16] 和 MDLM(min description length method)[52] 等 不 支 持 布 尔 型 特 征;Relief 系 列 算 法, DTM(decision tree method)[53]和 PRESET [54] 都适合于大数据集; Focus 等人[41] 适用于 小样本; 在度量标准的选择中, 只有一致性度量仅适 用于离散型数据等等.尽管特征选择方法已经有很多, 但针对解决实 际问题的方法还存在很多不足, 如何针对特定问题 给出有效的方法仍是一个需要进一步解决的问题. 将 Filter 方法和 Wrapper 方法两者结合, 根据特定的环境 选择所需要的度量准则和分类器是一个值得研究的 方向.selection algorithms: A survey and experimentalevaluation[R]. Barcelona:Catalunya, 2002.Universitat Politecnicade[9] Sun Z H, George Bebis, Ronald Miller. Object detectionusing feature subset selection[J]. Pattern Recognition, 2004, 37(11): 2165-2176.Narendra P M, Fukunaga K. A branch and bound algorithmfor feature selection[J]. IEEE Trans on Computers, 1977, 26(9): 917-922.Tsymbal A, Seppo P, David W P. Ensemble featureselection with the simple Bayesian classification[J].Information Fusion, 2003, 4(2): 87-100.Wu B L, Tom A, David F, et al. Comparison of statisticalmethods for classification of ovarian cancer using massspectrometry data[J]. Bioinformatics, 2003, 19(13): 1636- 1643.Furlanello C, Serafini M, Merler S, et al. An acceleratedprocedure for recursive feature ranking on microarraydata[J]. Neural Networks, 2003, 16(4): 641-648.Langley P. Selection of relevant features in machinelearning[C]. Proc of the AAAI Fall Symposium on Relevance. New Orleans, 1994: 1-5. [10] [11] [12] [13] [14] [15] Kononenko I. Estimation attributes:Analysis andextensions of RELIEF[C]. Proc of the 1994 European Conf on Machine Learning. New Brunswick, 1994: 171-182.Xu L, Y an P, Chang T. Best first strategy for featureselection[C]. Proc of 9th Int Conf on Pattern Recognition.Rome, 1988: 706-708.蔡哲元, 余建国, 李先鹏, 等. 基于核空间距离测度的特征选择[J]. 模式识别与人工智能, 2010, 23(2): 235-240.(Cai Z Y , Y u J G, Li X P, et al. Feature selection algorithm based on kernel distance measure[J]. Pattern Recognition and Artificial Intelligence, 2010, 23(2): 235-240.) 徐燕, 李锦涛, 王斌, 等. 基于区分类别能力的高性能特 征选择方法[J]. 软件学报, 2008, 19(1): 82-89.(Xu Y , Li J T, Wang B, et al. A category resolve power- based feature selection method[J]. J of Software, 2008, 19(1): 82-89.)参考文献(References )边肇祺, 张学工. 模式识别[M]. 第 2 版. 北京: 清华大学出版社, 2000.(Bian Z Q, Zhang X G. Pattern recognition[M]. 2nd ed. Beijing: Tsinghua University Publisher, 2000.)Kira K, Rendell L A . The feature selection problem:Traditional methods and a new algorithm[C]. Proc of the9th National Conf on Artificial Intelligence. Menlo Park, 1992: 129-134.John G H, Kohavi R, Pfleger K. Irrelevant features and thesubset selection problem[C]. Proc of the 11th Int Conf onMachine Learning. New Brunswick, 1994: 121-129. Koller D, Sahami M. Toward optimal feature selection[C].Proc of Int Conf on Machine Learning. Bari, 1996: 284-[1] [16] [17] [2] [3] [18][4]刘华文. 基于信息熵的特征选择算法研究[D]. 长春: 吉林大学, 2010.(Liu Hua-wen. A study on feature selection algorithm using 孟洋, 赵方. 基于信息熵理论的动态规划特征选取算法[J]. 计算机工程与设计, 2010, 31(17): 3879-3881.(Meng Y , Zhao F. Feature selection algorithm based on dynamic programming and comentropy[J]. Computer Engineering and Design, 2010, 31(17): 3879-3881.) Forman G. An extensive empirical study of feature selection metrics for text classification[J]. J of MachineLearning Research, 2003, 3(11): 1289-1305.Liu H, Liu L, Zhang H. Feature selection using mutualinformation: An experimental study[C]. Proc of the 10thPacific Rim Int Conf on Artificial Intelligence. Las V egas, 2008: 235-246.Hua J, Waibhav D T, Edward R D. Performance of feature-selection methods in the classification of high-dimensiondata[J]. Pattern Recognition, 2009, 42(7): 409-424.Mitra P, Murthy C A, Sankar K P. Unsupervised featureselection using feature similarity[J]. IEEE Trans on PatternAnalysis and Machine Intelligence, 2002, 24(3): 301-312.Wei H-L, Billings S A. Feature subset selection and rankin gfor data dim ensionality reduction[J]. IEEE Trans on PatternAnalysis and Machine Intelligence, 2007, 29(1): 162-166.Hall M A. Correlation-based feature subset selection formachine learning[M]. Hamilton: University of Waikato,1999.Zhang D, Chen S, Zhou Z-H. Constraint score: A new filtermethod for feature selection with pairwise constraints[J].Pattern Recognition, 2008, 41(5): 1440-1451.Le Song, Alex Smola, Arthur Gretton, et al. Supervisedfeature selection via dependence estimation[C]. Proc of the24th Int Conf on Machine Learning. Corvallis, 2007: 245- 252.Gustavo Camps-V alls, Joris Mooij, Bernhard Scholkopf.Remote sensing feature selection by kernel dependencemeasures[J]. IEEE Geoscience and Remote Sensin gLetters, 2010, 7(3): 587-591.Almuallim H, Dietterich T G. Learning with manyirrelevant features[C]. Proc of 9th National Conf onArtificial Intelligence. Menlo Park, 1992: 547-552.Liu H, Setiono R. A probabilistic approach to featureselection –A filter solution[C]. Proc of Int Conf on MachineLearning. Bari, 1996: 319-327.Manoranjan Dash, Huan Liu. Consistency-based search infeature selection[J]. Artificial Intelligence, 2003, 151(16):155-176.Huan Liu, Hiroshi Motoda, Manoranjan Dash. Amonotonic measure for optimal feature selection[M].Machine Learning: ECML-98, Lecture Notes in ComputerScience, 1998: 101-106.(下转第192页)[19] [31] information entropy[D]. Changchun: 2010.)Jain A K, Robert P W, Mao J C. Jilin University, [20] Statistical pattern[32] recognition: A review[J]. IEEE Trans on Pattern Analysis and Machine Intelligence, 2000, 22(1): 4-37.Battiti R. Using mutual information for selecting featuresin supervised neural net learning[J]. IEEE Trans on Neural Networks, 1994, 5(4): 537-550.Hanchuan Peng, Fuhui Long, Chris Ding. Feature selectionbased on mutual information: Criteria of max-dependency, max-relevance, and min-redundancy[J]. IEEE Trans on Pattern Analysis and Machine Intelligence, 2005, 27(8): 1226-1238.Ding C, Peng H. Minimum redundancy feature selectionfrom microarray gene expression data[J]. J of Bioinformatics and Computational Biology, 2005, 3(2): 185-205.Francois Fleuret. Fast binary feature selection withconditional mutual information[J]. J of Machine Learnin g Research, 2004, 5(10): 1531-1555.Kwak N, Choi C-H. Input feature selection by mutualinformation based on Parzen window[J]. IEEE Trans on Pattern Analysis and Machine Intelligence, 2002, 24(12): 1667-1671.Novovicova J, Petr S, Michal H, et al. Conditional mutualinformation based feature selection for classification task[C]. Proc of the 12th Iberoamericann Congress on Pattern Recognition. V alparaiso, 2007: 417-426.Qu G, Hariri S, Y ousif M. A new dependency andcorrelation analysis for features[J]. IEEE Trans onKnowledge and Data Engineering, 2005, 17(9): 1199- 1207.赵军阳, 张志利. 基于模糊粗糙集信息熵的蚁群特征选择方法[J]. 计算机应用, 2009, 29(1): 109-111.(Zhao J Y , Zhang Z L. Ant colony feature selection based on fuzzy rough set information entropy[J]. J of Computer Applications, 2009, 29(1): 109-111.)赵军阳, 张志利. 基于最大互信息最大相关熵的特征选 择方法[J]. 计算机应用研究, 2009, 26(1): 233-235.(Zhao J Y , Zhang Z L. Feature subset selection based on maxmutual information and max correlation entropy[J]. Application Research of Computers, 2009, 26(1): 233- 235.)渠小洁. 一种基于条件熵的特征选择算法[J]. 太原科技大学学报, 2010, 31(5): 413-416.(Qu X J. An algorithm of feature selection based on conditional entropy[J]. J of Taiyuan University of Science and Technology, 2010, 31(5): 413-416.)[21] [33] [22] [34] [35] [23] [36] [24] [37] [25] [38] [26] [39] [27] [40] [28] [41] [42] [29] [43] [44] [30]。
management动态能力与战略方案管理
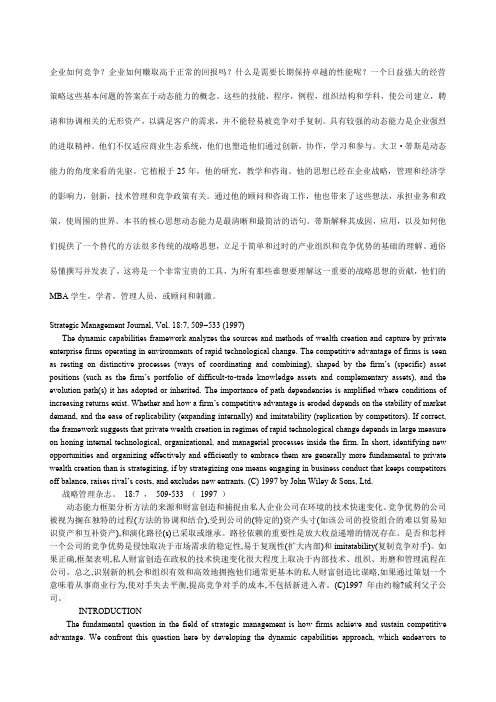
企业如何竞争?企业如何赚取高于正常的回报吗?什么是需要长期保持卓越的性能呢?一个日益强大的经营策略这些基本问题的答案在于动态能力的概念。
这些的技能,程序,例程,组织结构和学科,使公司建立,聘请和协调相关的无形资产,以满足客户的需求,并不能轻易被竞争对手复制。
具有较强的动态能力是企业强烈的进取精神。
他们不仅适应商业生态系统,他们也塑造他们通过创新,协作,学习和参与。
大卫·蒂斯是动态能力的角度来看的先驱。
它植根于25年,他的研究,教学和咨询。
他的思想已经在企业战略,管理和经济学的影响力,创新,技术管理和竞争政策有关。
通过他的顾问和咨询工作,他也带来了这些想法,承担业务和政策,使周围的世界。
本书的核心思想动态能力是最清晰和最简洁的语句。
蒂斯解释其成因,应用,以及如何他们提供了一个替代的方法很多传统的战略思想,立足于简单和过时的产业组织和竞争优势的基础的理解。
通俗易懂撰写并发表了,这将是一个非常宝贵的工具,为所有那些谁想要理解这一重要的战略思想的贡献,他们的MBA学生,学者,管理人员,或顾问和刺激。
Strategic Management Journal, Vol. 18:7, 509–533 (1997)The dynamic capabilities framework analyzes the sources and methods of wealth creation and capture by private enterprise firms operating in environments of rapid technological change. The competitive advantage of firms is seen as resting on distinctive processes (ways of coordinating and combining), shaped by the firm’s (specific) asset positions (such as the firm’s portfolio of difficult-to-trade knowledge assets and complementary assets), and the evolution path(s) it has adopted or inherited. The importance of path dependencies is amplified where conditions of increasing returns exist. Whether and how a firm’s competitive advantage is eroded depends on the stability of market demand, and the ease of replicability (expanding internally) and imitatability (replication by competitors). If correct, the framework suggests that private wealth creation in regimes of rapid technological change depends in large measure on honing internal technological, organizational, and managerial processes inside the firm. In short, identifying new opportunities and organizing effectively and efficiently to embrace them are generally more fundamental to private wealth creation than is strategizing, if by strategizing one means engaging in business conduct that keeps competitors off balance, raises rival’s costs, and excludes new entrants. (C) 1997 by John Wiley & Sons, Ltd.战略管理杂志。
基于受限玻尔兹曼机的手写字符识别算法研究

基于受限玻尔兹曼机的手写字符识别算法研究目录目录 (1)摘要 (2)ABSTRACT (2)第1章绪论 (3)1.1 研究背景 (3)1.2 研究现状 (3)1.3 本文的主要工作 (3)1.4 论文的组织结构 (4)第2章神经网络基本原理与RBM模型探讨 (5)2.1 启发式思想与模拟退火算法 (5)2.1.1 贪心算法的局部最优限制与启发式思想 (5)2.1.2 模拟退火模型及其概率转移计算 (5)2.2 玻尔兹曼机与受限玻尔兹曼机 (6)2.3 受限玻尔兹曼机模型概述 (7)2.3.1 受限玻尔兹曼机网络结构 (7)2.3.2 受限玻尔兹曼机能量函数及概率分布计算 (8)第3章 受限玻尔兹曼机中的数学理论 (11)3.1 随机梯度法解对数似然函数 (11)3.2 马尔可夫链蒙特卡罗策略与Gibbs采样 (13)3.2.1 马尔科夫链与马氏定理 (13)3.2.2 三种采样算法 (15)3.2.3 用采样技术解受限玻尔兹曼机中的计算难题 (19)3.3 对比散度算法 (20)第4章 基于受限玻尔兹曼机的手写字符识别模型 (23)4.1 手写字符特征提取 (23)4.1.1 图像特征提取概念及背景 (23)4.1.2 常见的手写字符图像特征提取方法 (23)4.1.3 数据降维与受限玻尔兹曼机提取特征 (25)4.1.4 深度信念网络与贪婪学习 (26)4.2 手写字符特征分类 (27)4.3 手写字符识别算法流程 (27)第5章 基于受限玻尔兹曼机的手写字符识别模型示例 (29)5.1 手写数字数据集MNIST解析 (29)5.2 结果分析 (30)第6章 总结与展望 (33)致谢 (34)参考文献 (35)附录1 英文原文 (37)附录2 译文 (39)摘要受限玻尔兹曼机(RBM)是一种内含两层结构,对称链接,无自反馈的深度学习网络模型。
受限玻尔兹曼机的快速学习算法近年来一直是研究热点,随着对比散度算法的出现,受限玻尔兹曼机在机器学习节掀起了应用和研究的热潮。
辩论:能力比责任重要
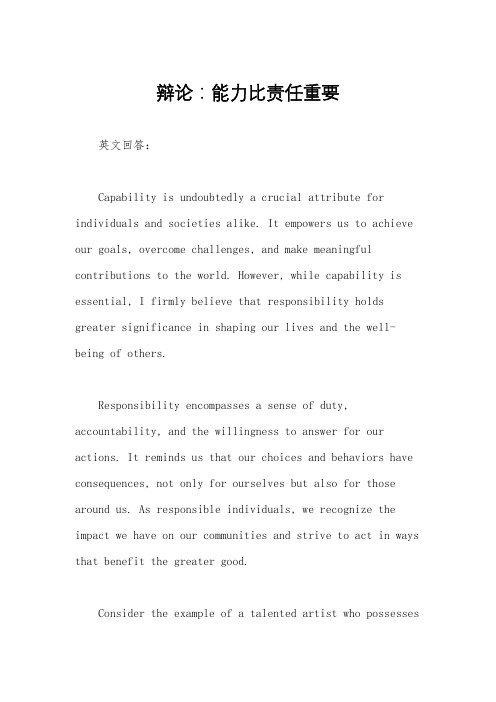
辩论:能力比责任重要英文回答:Capability is undoubtedly a crucial attribute for individuals and societies alike. It empowers us to achieve our goals, overcome challenges, and make meaningful contributions to the world. However, while capability is essential, I firmly believe that responsibility holds greater significance in shaping our lives and the well-being of others.Responsibility encompasses a sense of duty, accountability, and the willingness to answer for our actions. It reminds us that our choices and behaviors have consequences, not only for ourselves but also for those around us. As responsible individuals, we recognize the impact we have on our communities and strive to act in ways that benefit the greater good.Consider the example of a talented artist who possessesexceptional painting skills. While their capability enables them to create beautiful works of art, it is their sense of responsibility that drives them to use their talent for meaningful purposes. They may choose to paint murals in underprivileged neighborhoods, inspiring young minds and bringing joy to the community. Their art becomes a vehicle for social change and personal growth.In contrast, individuals who prioritize capability over responsibility may face moral dilemmas and ethical conflicts. They may possess great knowledge or abilities but lack the integrity to use them wisely. History is replete with examples of brilliant minds who succumbed to the temptations of power and wealth, ultimately causing harm to others.Moreover, responsibility fosters a sense of community and belonging. When individuals embrace their responsibilities, they contribute to the collective well-being of society. They volunteer their time, participate in local organizations, and work towards common goals. By taking ownership of our actions and decisions, we create amore cohesive and supportive social fabric.In the realm of technology, the debate betweencapability and responsibility becomes particularly relevant. Advances in artificial intelligence (AI) have created immense potential for progress and innovation. However, itis our responsibility as users and developers to ensurethat AI systems are deployed ethically and sustainably. We must consider the societal implications and potential risks associated with AI and act responsibly to prevent any negative consequences.In conclusion, while capability provides us with the means to act, it is responsibility that guides our actions, ensuring that we use our powers for good. Responsibility cultivates moral character, promotes social harmony, and empowers us to make meaningful contributions to society. By embracing responsibility as the guiding principle of our lives, we create a world where talent and abilities are harnessed for the betterment of humanity.中文回答:在我看来,责任比能力更重要,它塑造着我们的生活和影响着他人。
领导力的英语
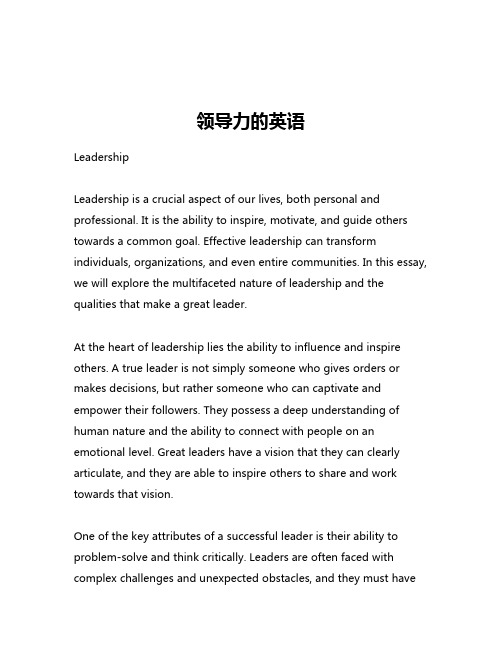
领导力的英语LeadershipLeadership is a crucial aspect of our lives, both personal and professional. It is the ability to inspire, motivate, and guide others towards a common goal. Effective leadership can transform individuals, organizations, and even entire communities. In this essay, we will explore the multifaceted nature of leadership and the qualities that make a great leader.At the heart of leadership lies the ability to influence and inspire others. A true leader is not simply someone who gives orders or makes decisions, but rather someone who can captivate and empower their followers. They possess a deep understanding of human nature and the ability to connect with people on an emotional level. Great leaders have a vision that they can clearly articulate, and they are able to inspire others to share and work towards that vision.One of the key attributes of a successful leader is their ability to problem-solve and think critically. Leaders are often faced with complex challenges and unexpected obstacles, and they must havethe cognitive flexibility to adapt and find innovative solutions. They must be able to analyze situations from multiple perspectives, weighing the pros and cons of various courses of action. This ability to think critically and make well-informed decisions is essential for effective leadership.Another crucial aspect of leadership is the ability to foster a positive and collaborative work environment. Great leaders understand the importance of building strong, cohesive teams and empowering their followers to contribute their unique skills and perspectives. They create a culture of trust, open communication, and mutual respect, which in turn leads to increased productivity, creativity, and job satisfaction among team members.Effective leaders also possess strong communication skills, both verbal and nonverbal. They are able to articulate their ideas clearly and concisely, and they are skilled listeners who can truly hear and understand the concerns and perspectives of their followers. Additionally, they are adept at using body language and facial expressions to convey confidence, empathy, and authority.Integrity is another essential quality of a great leader. Leaders must be authentic, transparent, and accountable for their actions. They must have a strong moral compass and consistently demonstrate their commitment to ethical behavior. When followers trust that theirleader is acting with integrity, they are more likely to be loyal, committed, and willing to follow that leader's lead.In addition to the qualities mentioned above, successful leaders also possess a certain degree of emotional intelligence. They are able to recognize and manage their own emotions, as well as the emotions of their followers. They can empathize with others, read social cues, and adjust their communication and leadership style accordingly. This emotional intelligence allows them to create a positive and supportive environment, which in turn fosters loyalty, motivation, and high performance among their team members.Finally, great leaders are continuous learners who are always striving to improve and expand their knowledge and skills. They are open to feedback, willing to admit their mistakes, and eager to seek out new perspectives and experiences. This commitment to personal and professional growth not only benefits the leader themselves but also inspires and encourages their followers to do the same.In conclusion, leadership is a multifaceted and complex concept that encompasses a wide range of qualities and skills. From inspiring and motivating others to problem-solving and fostering a positive work environment, effective leaders possess a unique blend of cognitive, emotional, and interpersonal abilities. By cultivating these essential leadership qualities, individuals can not only improve their ownperformance and success but also have a transformative impact on the lives of those they lead.。
- 1、下载文档前请自行甄别文档内容的完整性,平台不提供额外的编辑、内容补充、找答案等附加服务。
- 2、"仅部分预览"的文档,不可在线预览部分如存在完整性等问题,可反馈申请退款(可完整预览的文档不适用该条件!)。
- 3、如文档侵犯您的权益,请联系客服反馈,我们会尽快为您处理(人工客服工作时间:9:00-18:30)。
On the G eneralization Capability of Multi-Layered Networks in the Extraction of Speech Properties Renato DE MORI*, Yoshua BENG IO*, and Piero COSI **School of Computer Science, McGill University,3480 University St.,Montreal, Que., Canada H3A 2A7 **per le RicercheAbstractThe paper describes a speech coding system based on an ear model followed by a set of Multi-Layer Networks (MLN). MLNs are trained to learn how to recognize articulatory features like the place and manner of articulation. Experiments are performed on 10 English vowels showing a recognition rate higher than 95% for new speakers. When features are used for recognition, comparable results are obtained for vowels and diphthongs not used for training and pronouncedby new speakers. This suggests that MLNs suitably fed by the data computed by an ear model have good generalization capabilities over new speakers and new sounds.1. IntroductionCoding speech for Automatic Speech Recognition (ASR) can be performed with Multi-Layer Networks (MLN). This approach is interesting because it captures relevant speech properties useful for ASR at the stage of coding. A large number of scientists is currently investigating and applying learning systems based on MLNs [Rumelhart et al. 1986, Plout & Sejnowski 1987]. Applications have shown that MLNs have interesting generalization behaviour capable of capturing information related to pattern structures as well as characterization of parameter variation [Bengio et al. 1989, Bourlard & Wellekens 1987, Watrous & Shastri 1987]. Algorithms exist for MLNs with proven mathematical properties that allow learning to be discriminative and to focus on the properties that permit the separation of patterns belonging to different classes.Centro di Studiodi Fonetica,C.N.R.,Via G. Oberdan, 10,35122 PADOVA, ITALYIf we interpret each output of the coder as representing a phonetic property, then an output value can be seen as a degree of evidence with which that property has been observed in the data. An important research problem can be studied with such an approach; it deals with the possibility of learning all the required features and their use in correctly hypothesizing phonemes that were not used for learning. As a first attempt at solving this problem, we have chosen to represent vowels and diphthongs with the place of articulation and the manner of articulation related to tongue position since these features are well characterized by physical parameters that can be measured or estimated. Phoneticians have characterized vowels and other sounds by discretizing place of articulation and mannerof articulation related to tongue position which are in nature continuous acoustic parameters. We have inferred an MLN for each feature and discretized each feature with five qualitative values, namely PL1 ,....PLi,...,PL5 for the place and MN1 ,...MNj,....MN5 for the manner.Various tests have been performed, always with new speakers. The first test consists of pronouncing the same vowels in the same context as in the data used for learning. This test is useful for comparing the results obtained with a mathematical model of the ear [Seneff 1988] with those obtained with the more popular Fast-Fourier Transformation (FFT). This test is also useful for assessing the capabilities of the network learning method in generalizing knowledge about acoustic properties of speakers pronouncing vowels. The second test has the objective of recognizing vowels through features. This test has been useful for investigating the power of the networks with respect to possible confusion with vowels not used for learning. The third experiment is an attempt to recognize new vowels pronounced by new speakers. This showed how the MLNs generalize to combinations of values of features not seen in the training set. This generalization capability was verified with 8 new sounds pronounced by 20 new speakers. Without any learning of the new sounds, but just using expectations based on phonetic knowledge on theDe Mori, Bengio and Cosi 1531composing features and their time evolution, an error rate of 7.5% was found.2. Training of the MLNsThe Error Back Propagation Algorithm (EBPA)was used for training. EBPA was recently introduced [Rumelhart et al. 1986] for a class of non-linear MLNs. The networks used for the experiments described in this paper are feedforward (non-recurrent) and organized in layers. A weight is associated with the (unidirectional) connections between two nodes.With EBPA the weights are computed iterativelyin such a way that the network minimizes a cost C (thesum of the square of the differences between output unit values and target output values for the trainingexamples). The EBPA uses gradient descent in thespace of weights to minimize the error:(1In order to reduce the training time andaccelerate learning, various techniques can be used. The classical gradient descent procedure modifies the weights after all the examples have been presented to the network. This is called batch learning. However, it was experimentally found, at least for pattern recognition applications, that it is much more convenient to perform on-line learning, i.e., updating the weights after the presentation of each example. When using on-line learning, one has to be careful in choosing the order of presentation ofexamples. We presented examples of each class oneafter the other, going through all the different classes.Batch learning provides an accurate measure of the performance of the network as well as of the gradient 3E/3W. These two parameters can be used to adapt the learning rate during training in order to minimize the number of training iterations. In our experiments we used various types of acceleration techniques. The simplest one is to add a "momentum" term to the weight update rule [Rumelhart et al. 1986]. More interesting techniques involve adapting the learningrate as a function of 1) the evolution of the cost(deviation from target output), and 2) the evolution ofthe direction of the gradient. In other words, when thecost is improving sufficiently or when the gradient tends to point in the same direction from cycle to cycle, the learning rate should be increased. A further refinement consists of using (and adapting) a different learning rate for each connection. To improve learning time, a subset S of the training examples is used fortraining: those that produce errors. Once every few learning iterations on this subset, all the patterns aretested in order to decide which ones will go in S. Ofcourse, with this technique the global cost and theglobal gradient are not evaluated at each iteration, so it is more suited to on-line learning. Another way to 1532 Speech and Natural Languagereduce the learning time is to divide the problem into subproblems which are as independent as possible and assign those subproblems to subnetworks: this is modularization. In our case we used separate networks for place of articulation and for manner of articulation. Outputs of small modules can be combined heuristically according to our knowledge ofthe functions they perform based on speech theory. They can also be combined to form a bigger network using Waibel's (1988) glue units. Another technique we used to train big networks was first to minimize the error using a simple architecture (e.g., no hiddenunits). Once the simple network has been trained, it can be augmented with more hidden units (and many more weights) in order to reduce the error significantly. This strategy provided significant gains in training time in some cases.3. Experimental results 3.1 Speaker-Independent recognition of ten vowels in fixed contextA first experiment was performed for speaker-independent vowel recognition. The purpose was that of training an MLN capable of discriminating among 10 different American-English vowels represented withthe ARPABET by the following VSET: (2) Our interest was in investigating the generalization capability of the network with respect to inter-speaker variability. Some vowels and diphthongs (ix,ax,ey,ay,oy,aw,ow) were not used in this experiment because we attempted to recognize them through features learned by using only VSET. Speech material consisted of 5 pronunciations by 19 speakers of 10 monosyllabic words containing the vowels of VSET. The tokens from 12 of the speakers (6 males, 6 female) were used for training (600 tokens) and and the remaining ones (from 3 males, 4 females) were used for tests (350 tokens). Data acquisition was performed with a 12 bit A/D converter at a 16 kHz sampling frequency. The words used are those belonging to the WSET defined in thefollowing: (3) Two signal processing methods were used for this experiment. One was based on 128 point FFT spectra reduced to energy values in 40 bands, the other used the output of the G eneralized Synchrony Detector (GSD), represented by a 40-coefficient vector. In bothcases spectra were sampled every 5 ms. Spectral values were normalized to lie in the range 0 to 1. In order to capture the essential information of each vowel it was decided to use 10 equally-spaced frames per vowel for a total of 400 network input nodes. Best results were obtained with a single hidden layer with a total of 20 nodes. Ten output nodes were introduced, one for each vowel.Vowels were automatically singled out by an algorithm proposed in [De Mori et al 1985] and a linear interpolation procedure was used to obtain 10 equally-spaced frames per vowel (the first and the last 20 ms of the vowel segment were not considered in the interpolation procedure). The resulting 400 (40 spectral coefficients per frame x 10 frames) spectral coefficients became the inputs of the MLN.Training was stopped when the MLN made 0 errors on the training set. For the test set, the network produces degrees of evidence varying between zero and one, hence candidate hypotheses can be ranked according to the corresponding degree of evidence.The error rates on the test set were 4.3% with the ear model and 13.0% with the FFT. The reason for such a difference is probably due to the fact that the use of the ear model allowed us to produce spectra with a limited number of well defined spectral lines. This represents a good use of speech knowledge according to which formants are vowel parameters with low variance.Encouraged by the results of this first experiment, other problems appeared worth investigating with the proposed approach. The problems are all related to the possibilities of extending what has been learned for ten vowels to recognize new vowels. follows. A network with features as target outputs was slower to train and did not generalize as well on new speakers than a network with vowels as target outputs. This counterintuitive result might be explained by the possibility that the regions in the input space defined by the feature values are not as easily drawn (e.g., including several disjoint regions) as the regions in the input space defined by the vowel discrimination. Note that the acoustic definition of these features is imposed on the network based on speech production theory and might not represent the best choice of representation. Hence using 10 additional outputs representing the vowels forced the creation of hidden units that were useful to perform the vowel discrimination. These hidden units in turn could be used to produce the target feature values. The resulting network still does not generalize as well as the vowel discrimination network on new speakers, but it does generalize on new vowels.3.2. Recognition of phonetic featuresThe same procedure introduced in the previoussection was used for learning in three networks, namely MLNV1, MLNV2 and MLNV3. These networks have the same structure as the one introduced previously, the only difference being that they have more outputs. MLNV1 has five additional outputs corresponding to the five places of articulation PL1,...,PLi PL5. MLNV2 has five new outputs, namely MN1,...,MNj,...MN5. MLNV3 has two additional outputs, namely T=tense and U=lax. The ten vowels used for this experiment have the features defined in Table 1. Training the first 10 outputs to correspond to the 10 vowels improved generalization over nets with only feature outputs. This might be explained asAfter having learned the weights of the three networks with the same methodology as for the first experiment, confusion matrices were derived only for the outputs corresponding to the phonetic features. An error was determined by comparing the feature value with the highest degree of evidence with the correct feature.The overall error rates on the test sets were 4.57%, 5.71% and 5.43% respectively for the three sets of features. Error rates on the training set were always zero after a number of training cycles (between 60 and 70) of the three networks. Several rules can beDe Mori, Bengio and Cosi 1533conceived for recognizing vowels through their features. The most severe rule is that a vowel is recognized if all three features have been scored withthe highest evidence. With such a rule, 313 out of 350vowels are correctly recognized corresponding to 10.5% error rate.In 28 cases, combinations of features having the highest score did not correspond to any vowel, so a decision criterion had to be introduced in order to generate the best vocalic hypothesis. In 2.57% of the examples, the three features corresponded to a wrong vocalic hypothesis. This leads to the conclusion that an error rate between 2.57% and 10.57% can be obtained depending on the decision criterion used for those cases in which the set of features having the highest membership in each network do not correspond to any vowel.An appealing criterion consists of computing the centers of gravity of the place and manner of articulation using the following relation:(4),The MLNs, trained as described in section 3, have as input 10 frames of 40 parameters each. During training these frames were chosen so as to span the length of a stable vowel segment. In the test experiments described below, the 10 frames are 10 consecutive frames each representing 5 ms of speech. The MLN thus has an input window of 50 ms which scans the input speech data with a 5 ms step. According to other experimental work on vowel recognition [Leung & Zue 1988], there are 13 vowels in American English and 3 diphthongs. The vowels and diphthongs that were not used in the previous experiments belong to the NSET:(5)The vowel /ax/ does not exhibit transitions in time of the parameters CGM and CGP so its recognition was based on the recognition of the expected features as defined in Table 1. The other five elements of NSET exhibit evolution of CGP and CGM in the time domain. For this reason, it was decided to use such evolutions as the basis for recognition.where ji(i) is the degree of evidence for feature level i obtained by the MLNs. Let CG P and CG M be, respectively, the center of gravity of the place and manner of articulation. A degree of "tenseness" has been computed by dividing the membership of "tense" by the sum of the memberships of "tense" and "lax". Each sample can now be represented as a point in a three-dimensional space having CGP, CGM and the degree of tenseness as dimensions. Euclidean distances are computed from choices of feature values not corresponding to any vowel to the points representing theoretical values for each vowel. With centers of gravity and Euclidean distance an error rate of 7.24% was obtained. The error rate obtained with gravity centers is not far from that obtained with ten vowels but is higher because the system was allowed to recognize feature combinations for all the vowels of American English.3.3. Recognition of new phonemesIn order to test the generalization power of the networks for feature hypothesization a new experiment was performed involving 20 new speakers from 6 different mother tongues (English, French, Spanish, Italian, G erman and Vietnamese) pronouncing isolated letters and words in English.1534 Speech and Natural Languagefeature values, a crude classification criterion was applied in this experiment. Recognition was based purely on time evolution of place and manner of articulation according to descriptions predictable from theory or past experience and not learned by actual examples. The centers of gravity CGP and CGM were computed every 5 ms and vector-quantized using fivesymbols for CGP according to the following alphabet:(6),where F represents "strong front". Analogously, the following alphabet was used for quantizing the mannerof articulation:(7),where H represents "strong high". Coding of CGP and CGM is based on values computed from the data of the ten vowels used for training the network.Transitions of CGP and CGM were simply identified by sequences of pairs of symbols from Σ1 and Σ2. Figure 1 shows definitions of Σ1 and X2 and gives an example of the time evolution of CGP and CGM for letters A (/ey/) and Y (/way/) together with their codes.The following regular expressions were used to characterize the words containing the new vowels and diphthongs:In theory the asterisk means "any repetition", but in our case a minimum of two repetitions was required. The symbol V means logical disjunction while a concatenation of terms between parentheses means a sequence in time. A short sequence with intermediate symbols was tolerated in transitions B-F , L-H and vice-versa, as well as in initial and final transients.For each vowel and diphthong, twenty samples were available based on the idea that speaker-independent recognition has to be tested with data from new speakers and repetition of data from the same speaker is not essential. The errors observed were quite systematic. For /ax/, 1 token was confused with /ah/. For /ey/ (letter A), three errors were observed, all corresponding to a sequence (f,h)* meaning that the transition from /eh/ was not detected. For /ow/ (letter O), three errors were observedcorresponding to the sequence (b,l)* meaning that the transition from /oh/ was not detected, which may correspond to an intention of the speaker. Three errors were found for /oy/ confused with /ay/ and two errors for /aw/ confused with /ow/. The repeatability of the describing strings was remarkable. Performance can be improved with a more rigorous word recognition algorithm. 4 ConclusionsThe work reported in this paper shows that a combination of an ear model and multi-layer networks results in an effective generalization among speakers in coding vowels. The results obtained in the speaker-independent recognition of ten vowels add a contribution that justifies the interest in the investigation of the use of ML Ns for ASR [L eung & Zue 1988, Waibel et al, 1988].Furthermore, training a set of MLNs with a sma'l number of training speakers on a number of well distinguishable vowels resulted in a very good generalization on new speakers (with a variety of accents) as well as on new vowels and diphthongs if recognition is based on features.By learning how to assign degrees of evidence to articulatory features it is possible to estimate normalized values for the place and manner of articulation which appear to be highly consistent with qualitative expectations based on speech knowledge. The error-back propagation algorithm seems to be a suitable one for learning weights of internode links in MLNs. A better understanding of the problems related to its convergence is a key factor for the success of an application. The choice of the number of MLNs, their architecture, the coding of their input and output and the learning strategy are also of great importance, especially for generalization.The computation time of the system proposed in this paper is about 150 times real-time on a Sun 4/280. The system structure is suitable for parallelization with special purpose architectures and accelerator chips. It is not unrealistic to expect that with a suitable architecture, such a system could operate in real-time. AcknowledgementsThis work was supported by the Natural Science and Engineering Council of Canada (NSERC). The Centre de recherche informatique de Montr6al (CRIM) kindly provided computing time on its facilities.De Mori, Bengio and Cosi 1535References[Bengio et al. 1989] Bengio, Y., Cardin, R., De Mori, R., Merlo, E., "Programmable Execution of Multi-Layered Networks for Automatic Speech Recognition", Communications of the Association for Computing Machinery, February 1989, vol. 32, no. 2, pp. 195-199.[Bourlard & Wellekens 1987] H. Bourlard and C.J. Wellekens, "Multilayer perceptron and automatic speech recognition", IEEE first International Conference on Neural Networks, San Diego, June 1987, pp. IV407-IV416.[De Mori et al. 1985] R. De Mori, P. Laface and Y. Mong Y.f "Parallel algorithms for syllable recognition in continuous speech", IEEE Transactions on Pattern Analysis and Machine Intelligence, Vol. PAMI-7, N. 1,1985, pp. 56-69.[Leung & Zue 1988] H. C. Leung and V. W. Zue, "Some phonetic recognition experiments using artificial neural nets". Proc. International Conference on Acoustics, Speech and Signal Processing, New York, N.Y., 1988, pp. 422-425.[Plout & Hinton 1987] D.C. Plout & G.E. Hinton, "Learning sets of filters using back propagation", Computer Speech and Language, 1987, vol. 2, pp.35-61.[Rumelhart et al. 1986] D.E. Rumelhart, G.E. Hinton and R.J. Williams, "Learning internal representation by error propagation", Parallel Distributed Processing: Exploration in the Microstructure of Cognition, vol. 1, MIT Press, 1986, pp.318-362.[Seneff 1988] S. Seneff, "A joint synchrony/mean-rate model of auditory speech processing", Journal of Phonetics, January 1988, pp. 55-76.[Waibel et al. 1988] A. Waibel, T. Hanazawa, K. Shikano, "Phoneme recognition: neural networks vs hidden Markov models", Proc. International Conference on Acoustics, Speech and Signal Processing 1988, New York, N.Y., paper 8.S3.3. [Watrous & Shastri 1987] R.L. Watrous and L. Shastri, "Learning phonetic features using connectionist networks", Proceedings of the 10th International Joint Conference on Artificial Intelligence, 1987, pp.851-854.1536 Speech and Natural Language。