On Default Correlation A Copula Function Approach
A Model for Counterparty Risk with Geometric Attenuation Effect and the Valuation of CDS

a r X i v :0706.3331v 1 [m a t h .P R ] 22 J u n 2007A Model for Counterparty Risk with Geometric Attenuation Effectand the Valuation of CDSYunfen BAI†‡,Xinhua HU †Zhongxing YE ††Department of Mathematics,Shanghai Jiaotong University,Shanghai 200240,China ‡Department of Mathematics,Shijiazhuang College,Shijiazhuang,Hebei 050035,ChinaJanuary,2007Abstract:In this paper,a geometric function is introduced to reflect the attenuation speedof impact of one firm’s default to its partner.If two firms are competitions (copartners),the default intensity of one firm will decrease (increase)abruptly when the other firm defaults.As time goes on,the impact will decrease gradually until extinct.In this model,the joint distribution and marginal distributions of default times are derived by employing the change of measure,so can we value the fair swap premium of a CDS.Key words:Dependent default;Geometric attenuation function;Change of measure;Credit Default Swap(CDS)2000Mathematics Subject Classification:62P051.IntroductionThe rapid expansion in recent years of market for the credit derivatives had led to a growing interest in the valuation of these instruments including the credit default swaps(CDS).The refer-ence issuers and the derivative issuers not only have default risk,but also correlate in some way.As remarked by Jarrow and Yu (2001),”an investigation of counterparty risk is incomplete with-out studying its impact on the pricing of credit derivatives”.We can distinguish three different approaches to model default correlation in the literature of intensity credit risk modeling.The first approach to model default correlation makes use of copula functions.A copula is a function that links univariate marginal distributions to the joint multivariate distribution with auxiliary correlating variables.Li (2000)was probably the first to explicitly use the concept of copulas in the context of basket default derivatives pricing.The second approach introduces correlation in firms’default intensities making them dependent on a set of common variables X t and on a firm specific factor.These models have received the name of conditionally independent defaults (CID)models,because conditioned to the realizationof the state variables X t thefirm’s default intensities are independent as are the default times that they generate.for example Duffie and Singleton(1999)and Lando(1994,1998).The last approach to model default correlation,contagion models,relies on the works by Davis and Lo(1999)and Jarrow and Yu(2001).It is based on the idea of default contagion in which, when afirm defaults,the default intensities of relatedfirms jump upwards.Leung and Kwok(2005) gave the analytic solution for the CDS premium for Jarrow and Yu(2001)model by employing the change of measure which was introduced in Collin-Dufresne(2004).But it is unrealistic for them to assume that onefirm’s default intensity keep a constant jump after the otherfirm defaults.In this paper,we introduce a geometric function to reflect the attenuation impact of onefirm’s default to otherfirms’default intensities.In our model,onefirm’s default will influence otherfirms’default intensities and the impact will decrease until extinct as time goes on.That is to say after a period of time,onefirm’s default intensity will depend thefirm itself while the impact of otherfirm’s default will disappear.The model is more realistic.The paper is organized as follows.In section2,we introduce a geometric function to reflect the attenuation impact of onefirm’s default to its partners,and give emphasis on the case when the twofirms are competitions.The joint and marginal distributions of the twofirms’default times are got by employing the change of measure which was introduced in Collin-Dufresne(2004). In section3,we price the CDS premium using the conclusion of section2and get the analytic solution.The paper is ended with conclusion in the last section.2.A Model for Dependent Default with Geometric Attenuation functionWe consider an uncertain economy with a time horizon of T∗described by afiltered space (Ω,F,{F t}T∗t=0,P)satisfying F=F T∗,where P is the risk-neutral(equivalent martingale)measure in the sense of Harison and Kreps(1981),that is,all security prices discounted by the risk-free interest rate r t are martingale under P.In this section,we construct a two-firm model with default correlation.Supposefirm B and firm C have high direct linkage which are competitions or copartners.The default time offirm i (i=B,C)is denoted byτi which posses a strictly positive F t-predictable intensity processλi t with right-continuous sample paths.If we define N i t=I(τi≤t)as the default indicator function which equals to0beforefirm i defaults and1otherwise.Let H t=σ(N B s,0≤s≤t)∨σ(N C s,0≤s≤t),then we can get thatM i t=N i t− tΛτi0λi s ds(1) is an F t-local martingale and the conditional survival probability offirm i is given byP(τi>T|H t∨F t)=I(τi>t)E[exp(− T tλi s ds)|F t].(2)2The default correlation betweenfirm B andfirm C is characterized by the correlated default intensities:λB t=b0+I(τC≤t)b1c2(t−τB)+1,(4) where b0,c0,b2,c2are nonnegative real numbers,and b1,c1are real numbers satisfying b0+b1> 0,c0+c1>0.In this model,the default offirm C(B)will bring abrupt change to the default intensity offirm B(C).If B is one competition(copartner)offirm C,b1<0(>0),and whenfirm C defaults,the default intensityλB t jumps by the amount of|b1|from b0to b0+b1.As time goes on,the impulsion effect will attenuate until extinct with geometric speed,that is to say,λB t will come back to b0at last.This explanation is the same as C is one competition(copartner)of firm B.Parameters b0and c0are thefirm-specific default intensity.Parameters b1and c1reflect the impact intensities of counterparty’s default,and when b1=0and c1=0,firm B andfirm C are default-independent.Parameters b2and c2are non-negative real numbers,which reflect the attenuation speed.When b2=0and c2=0,the model becomes the one in Jarrow and Yu(2001) and Yuen and Yue(2005).To calculate the joint distribution of the two default time offirm B andfirm C in[0,T](T<T∗), we adopt the change of measure introduced by Collin-Dufresne(2004),defining afirm-specific probability measure P i which puts zero probability on the pathes where default occurs prior to the maturity T.Specifically,the change of measure is defined byZ i T .=dP ifound to be P (τB>t 1,τC>t 2)=c (t 2−t 1+1b 0)e −b 0t 1−c 0t 2+cb−1c 0e−(b 0+c 0)t 1,for t 2<t 1≤T,(6)and the joint density isf (t 1,t 2)=cb 0c 0[(t 2−t 1)+1c 0]e−b 0t 1−c 0t 2,for t 1≤t 2≤Tbb 0c 0[(t 1−t 2)+1b 0]e−b 0t 1−c 0t 2,for t 2<t 1≤T.(7)4Proof:If t2≤t1≤T,(τB>t1,τC>t2)∈H t1⊂G B t1.(8)So we can getP(τB>t1,τC>t2)=E B[I(τB>t2)exp{− t10(b0+I(τC≤t)−b1(t−τC+1)b2dt}If b2=1,the above equation equals to=e−b0t1E B[I(t2<τC≤t1)exp{−b11−b2}[ t1t2c0e−c0t[b(t1−t)+1]bt+e−c0t1]=b(t1−t2+1c0)e−c0t2−b0t1+bb −1c0e−(c0+b0)t1,(9)where E C denotes the expectation under the measure P C.Thefirst equation follows from the definition of P C,and the fourth from the fact thatλB t=b0for t<t2under P C.By a similar argument for t2≤t1≤T,the joint distribution is given byP(τB>t1,τC>t2)=b(t1−t2+1c0)e−b0t1−c0t2+b∂t1∂t2= cb0c0[t2−t1+1c0]e−b0t1−c0t2,for t1<t2≤T bb0c0[t1−t2+1b0]e−b0t1−c0t2,for t2<t1≤T,(11)The proof is completed.♯Remark1It is worth noting that if cb0=bc0,then f(t1,t2)is not continuous on the plane t1=t2.Corollary1Under the assumption of Proposition1,if b1=c1=0,then the joint distribution of default times(τB,τC)with the default intensity defined by Eq.(3)(4)on[0,T]×[0,T]isP(τB>t1,τC>t2)=e−b0t1−c0t2,(12)in other words,when b1=c1=0,(τB,τC)are default-independent on[0,T]×[0,T].Proof We can get(11)by taking limit in Eq.(9)or Eq.(10)as c→0+.♯5Corollary2If−b1=b2=b>0,−c1=c2=c>0,the marginal distributions ofτB,τC on [0,T]are given byP(τB>t1)=e−b0t1+bb0e−c0t2[e−b0t2−1+b0t2],t2≤T.(14) Proof We can get Eqs.(13)and(14)by taking t1=0and t2=0in Eqs.(9)and(10) respectively.♯Remark2Thefirst term in Eq.(13)denotes thefirm-specific survival probability,and the second one denotes the increment offirm B’s survival probability because of the default offirm C and the geometric attenuation speed.As the result ofe−c0t1−1+c0t1≤1c0e−b0t1[e−c0t1−1+c0t1]≤1b0e−2for22bc0T2e−b0T for2 2bc0t21e−b0t1.An analogous argument also holds forfirm C.Section3CDS Valuation in the Model of Dependent Default with Geometric Attenuation functionIn this section we use the conclusion of Section2to price the premium of a CDS.A CDS is a contract agreement between protection buyer and seller,in which the protection buyer pays periodically to the protection seller afixed amount fee(swap premium pr spread)asking for a payment when the reference asset defaults.A institute can use a CDS to transfer,elude and hedge the credit risk of a risky asset(or basket of risky assets)from one party to the other.So a CDS is a very important instrument to manage credit risk.Suppose interest rate r t is a constant r.Assume that party A holds a corporate bond and faces the credit risk arising from default of the bond issuer(reference party C).To seek protection against such default risk,party A enters a CDS contract in which he agrees to make a stream of periodic premium payments,known as the swap premium to party B(CDS protection seller).In exchange,party B promises to compensate A(CDS protection buyer)for its loss in the event of default of the bond(reference asset).Without loss of generality,we take the notional to be$1 and assume zero recovery under default.Firm B paysfirm A after a settlement periodδwhen the6reference asset c defaults.Furthermore in Leung and Kwok (2005),they conclude the expression for the swap premium has little dependence on the default intensity of the protection buyer,so we impose that during the entire contract,firm A doesn’t default.The default intensity processes of firm B and C are given by Eqs.(3)and (4)in special casesλB t =b 0−I (τC ≤t )bc (t −τB )+1.(4)Since it takes no cost to enter a CDS,the value of the swap premium S (T )is determined by ni =1E [e −rT iS (T )I (τB V τC >T i )]+S (T )A (T )=E [e −r (τC+δ)I (τC ≤T )I (τB >τ+δ)](15)where {T 1,...,T n }are the swap payment dates with 0=T 0<T 1<...<T n =T,T i −T i −1=∆T,T +δ<T ∗,and δis the length of settlement period.Here τC +δrepresents the settlement date at the end of the settlement period.The first term in Eq.(15)gives the present value of the sum of periodic swap payments(determined when either B or C defaults or at maturity)and S (T )A (T )is the present value of the accrued swap premium for the fraction of period between τC and the last payment date.The present value of accrued swap premium is given byS (T )A (T )=S (T )n i =1E [e−rτCτC −T i −11−e −β∆T.(18)From Eq.(11)it can be foundE [e −r (τC+δ)I (τC ≤T )I (τB >τC +δ)]= T 0 +∞t 2+δe−r (t 2+δ)bb 0c 0[t 1−t 2+1b 0]e−b 0t 1−c 0t 2dt 1dt 2=bc 0b +δ)e−(r +b 0)δ[1−e −βT ](19)andE [e −rτCτC −T i −1∆Tbb 0c 0[t 1−t 2+1b 0]e−b 0t 1−c 0t 2dt 1dt 2=c 0β)(e−βT i−e −βT i −1)].(20)7SoS(T)A(T)=S(T)c0β n i=1(e−βT i−e−βT i−1)]=S(T)c0β(e−βT−1)+1β2∆T1β2∆T1β(11−e−β∆T+A(T)]−1.(22)where A(T)is given by Eq.(21).Remark2:Due to1−e−β∆T−β∆T e−β∆T≥1β(1c0∆T[3]Duffie,D.,and K.Singleton(1999):”Modeling Term Structures of Defaultable Bonds,”Reviewof Financial Studies,12:687-720.[4]Harrison M,Pliska S.Martingales and stochastic integrals in the theorey of continuous trading.Stochastics processes and their applications,1981,11:215-260.[5]Jarrow R A,Yu F.Counterparty risk and the pricing of defaultable securities.Journalof F inance,2001,56(5):1765-1799.[6]Kijima M,Muromachi Y.Credit Events and the Valuation of Credit Derivatives of Basket Type.Review of Derivatives Research,2000,4:55-79.[7]Lando D.Three essays on contigent claims pricing,Ph.D.dissertation,Cornell University,1994.[8]Lando D.On Cox processes and credit risky securities.Review of Derivatives Research,1998,2:99-120.[9]Laurent J-P Gregory J.Basket Default Swaps,CDO’s and Factor Copulas,W orkingP aper,ISFA Actuarial School,University of Lyon,2003.[10]Leung S Y,Kwok Y K.Credit default swap valuation with counterparty risk.Kyoto EconomicReview,2005,74(1):25-45.[11]Li D X.On Default Correlation:A Copula function Approach.Journal of F ixed Income,2000,Vol9,March:43-54.[12]Schonbucher P,Schubert D.Copula-dependent default risk in intensity models.W orking paper,Bonn University,2001.[13]Shreve S E.Stochastic Calculus for Finance I:The Binomial Asset Pricing Model.Springer,New York.2004.[14]Zhou C.An analysis of default correlations and multiple defaults.T he Review of F inancial Studies,2001,14(2):555-576.9。
copula函数 广义帕累托分布gp r语言

《探讨copula函数在广义帕累托分布中的应用》1. 引言在统计学和金融领域,copula函数作为一种多变量分布函数的工具,被广泛应用于风险管理、极值理论等方面。
而广义帕累托分布(GP分布)作为一种重要的概率分布模型,对特殊事件的建模和预测具有重要意义。
本文旨在探讨copula函数在广义帕累托分布中的应用,以及利用R语言进行相关分析和建模。
2. copula函数的基本概念让我们来了解一下copula函数的基本概念。
在概率论和统计学中,copula函数是用来描述多维随机变量的边际分布函数之间的相关性结构的函数。
它将边际分布和相关性结构分开,使得模型更加灵活,能够更准确地描述变量之间的相关关系。
在实际应用中,copula函数可以帮助我们更好地理解和分析多个变量之间的相关性,从而提高预测和决策的准确性。
3. 广义帕累托分布的特点接下来,让我们来了解一下广义帕累托分布的特点。
GP分布是对极值理论中的尾部分布进行建模的重要工具,它能够更好地描述特殊事件的分布特性。
GP分布具有长尾分布的特点,适用于描述尾部特殊事件的概率分布。
在风险管理和可靠性分析领域,GP分布被广泛应用于对特殊事件的建模和预测。
4. copula函数在广义帕累托分布中的应用现在,让我们探讨一下copula函数在广义帕累托分布中的应用。
通过将copula函数与GP分布相结合,我们可以更准确地描述多个特殊事件之间的相关性,从而提高风险管理和极值事件预测的准确性。
利用copula函数,我们可以更好地理解多个特殊事件之间的相关性结构,并通过GP分布对特殊事件的概率分布进行建模,从而更好地应对特殊事件带来的风险。
5. R语言在建模分析中的应用让我们来谈谈R语言在建模分析中的应用。
作为一种功能强大的统计分析和数据可视化工具,R语言提供了丰富的工具包和函数,能够帮助我们更好地进行copula函数和GP分布的建模和分析。
通过R语言,我们可以轻松地对多变量数据进行分析和建模,从而更好地理解特殊事件之间的相关性,并进行风险管理和可靠性分析。
copula r语言 参数估计方法

在深度学习的数据分析中,参数估计方法是一项至关重要的工作。
在R语言中,参数估计方法有多种,其中copula是一种非常重要的方法之一。
本文将对copula在R语言中的参数估计方法进行深入探讨,以便读者更好地理解并运用这一方法。
1. copula的概念和应用copula是一种用来描述随机变量联合分布的方法。
它的重要性在于可以独立变换边际分布和相关关系,从而更灵活地建模多维随机变量之间的依赖关系。
在实际数据分析中,copula方法被广泛应用于金融风险管理、气象预测、医学统计等领域。
2. copula在R语言中的参数估计方法在R语言中,对copula进行参数估计主要使用copula包。
该包提供了各种参数估计的方法,如极大似然估计、矩方法等。
其中,极大似然估计是最常用的方法之一,通过最大化样本的似然函数来估计copula的参数。
3. 极大似然估计方法的实现步骤- 数据准备:首先需要加载相关的R包,然后准备好待分析的数据集。
- 模型选择:根据具体的数据特点和研究目的选择合适的copula模型。
- 参数估计:利用copula包中的相应函数进行参数的极大似然估计。
- 参数诊断:对估计的参数进行诊断和检验,确保参数估计的准确性和可靠性。
4. copula参数估计的应用案例分析为了更好地说明copula在R语言中的参数估计方法,我们以金融风险管理为例进行实际应用。
假设我们需要分析股票收益率之间的相关性,我们可以使用copula方法来建模多个股票收益率之间的依赖关系,从而更准确地评估投资组合的风险。
5. 个人观点和理解作为一种灵活而有效的参数估计方法,copula在R语言中的应用为我们提供了更多的数据建模选择。
通过合理选择copula模型和有效进行参数估计,我们可以更好地理解和应用多维随机变量之间的依赖关系,从而提高数据分析的深度和广度。
总结回顾本文对copula在R语言中的参数估计方法进行了全面的探讨,包括概念和应用、极大似然估计方法的实现步骤、以及应用案例分析。
copula函数的基本原理
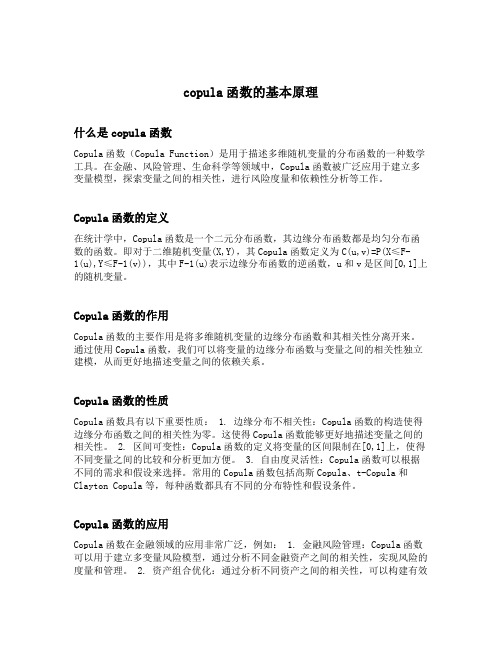
copula函数的基本原理什么是copula函数Copula函数(Copula Function)是用于描述多维随机变量的分布函数的一种数学工具。
在金融、风险管理、生命科学等领域中,Copula函数被广泛应用于建立多变量模型,探索变量之间的相关性,进行风险度量和依赖性分析等工作。
Copula函数的定义在统计学中,Copula函数是一个二元分布函数,其边缘分布函数都是均匀分布函数的函数。
即对于二维随机变量(X,Y),其Copula函数定义为C(u,v)=P(X≤F-1(u),Y≤F-1(v)),其中F-1(u)表示边缘分布函数的逆函数,u和v是区间[0,1]上的随机变量。
Copula函数的作用Copula函数的主要作用是将多维随机变量的边缘分布函数和其相关性分离开来。
通过使用Copula函数,我们可以将变量的边缘分布函数与变量之间的相关性独立建模,从而更好地描述变量之间的依赖关系。
Copula函数的性质Copula函数具有以下重要性质: 1. 边缘分布不相关性:Copula函数的构造使得边缘分布函数之间的相关性为零。
这使得Copula函数能够更好地描述变量之间的相关性。
2. 区间可变性:Copula函数的定义将变量的区间限制在[0,1]上,使得不同变量之间的比较和分析更加方便。
3. 自由度灵活性:Copula函数可以根据不同的需求和假设来选择。
常用的Copula函数包括高斯Copula、t-Copula和Clayton Copula等,每种函数都具有不同的分布特性和假设条件。
Copula函数的应用Copula函数在金融领域的应用非常广泛,例如: 1. 金融风险管理:Copula函数可以用于建立多变量风险模型,通过分析不同金融资产之间的相关性,实现风险的度量和管理。
2. 资产组合优化:通过分析不同资产之间的相关性,可以构建有效的投资组合,实现资产配置和风险控制的优化。
3. 衍生品定价:Copula函数可以用于对不同衍生品之间的相关性进行建模,从而实现衍生品的定价和风险度量。
Copula函数的选择及其在金融分析中的若干应用

摘 要Copula理论是一种基于联合分布的建模方法,最大的优点就是把边缘分布和相关结构分离开,它的提出为解决多元联合分布的构建以及变量间的非线性相关性问题提供了一个灵活实用的方法,人们将其广泛的用于金融领域,应用于投资组合、资产定价等方面,对金融数据相关性进行建模、分析有着非常重要的意义和作用。
本文主要讨论了Copula理论在金融领域的应用,分析了基于Copula理论的多金融资产的投资组合优化及风险度量的问题。
主要工作如下:首先介绍Copula函数的相关概念和性质,目前国内外Copula理论研究的进展情况,本文的研究思路、方法及相关应用。
传统的金融数据分析是基于正态分布的假设,但正态假设有其局限性,往往不能满足,本文打破传统的基于正态分布的假设,讨论了Copula理论和Monte Carlo模拟在风险VaR估计中的应用,并选用股票数据实例分析了基于Archimedean Copula的风险VaR估计,结果表明此方法是有效的,而传统的VaR计算方法低估了风险。
并进一步将此方法推广到多维资产的情形,说明与单支股票风险均值相比采用此方法确定的投资组合降低了金融风险。
文章为了进一步提高模型构造的有效性,提出了一种基于Bayes理论的混合Copula构造方法,把多个Copula函数所具有的优点融合到一个混合函数中,通过调整各个函数的权重系数来调整函数尾部相关性强弱,比单个Copula相关结构更为灵活。
另外,将Bootstrap方法引入到Copula中的参数估计中,实例表明采用Bootstrap 方法对参数的估计与实际值比较接近,为我们提供了解决问题的另一种有效的思路。
最后,对本文进行了总结,并对一些本文中可以继续探讨研究的方向进行了进一步的前景展望。
关键词:Copula函数;VaR估计;Bootstrap方法;投资组合Selection of Copulas and its Application on FinanceAbstractCopula functions which based on joint distribution provide a flexible and useful statistic tool to construct multivariate joint distribution and solve the nonlinear correlation problem. One of its advantages is the dependence structure of variables no longer depending on the marginal distributions. Copula theory has gained increasing attention in asset pricing, risk management, portfolio management and other applications. In detail, my research is as follows:We first introduce the ideas of copula theory and several copula functions which belong to Archimedean families. Then we discuss the use of Archimedean Copula in VaR and CVaR calculation without the traditional multidimensional normal distribution assumption in financial risk management. Our empirical analysis which based on stock market data uses Monte Carlo simulation method and the results show that the VaR calculated by copula method is larger than traditional method. It means that traditional method underestimated the risk of stock market, and the Monte Carlo simulation based on Copula is effective for financial Market. Then, this method is extended to the multidimensional case, to show that the VaR of proper portfolio is lower than means of risk with single stock.In order to improve the validation of model construction, we introduce a simple Bayesian method to choose the “best” copula which is a mixture of several different copulas. By estimating parameters of each chosen copula and adjusting their weight coefficients in the mixed copula, the model has all the advantages of the chosen copulas and has more flexibility because different weight coefficient combinations describe different asset correlations. In addition, we introduce Bootstrap method to estimate the parameters of Archimedean Copula. The real analysis also shows the estimated parameter by Bootstrap method gets closer to actual value. So it is another efficient way to solve our problems.At last, we make a summary of the whole paper, and look into the future of some aspects that could be discussed in the coming days.Key Words:Copulas; VaR estimation; Bootstrap method; portfolio目录摘 要 (1)Abstract (III)第一章 绪论 (1)1.1 研究背景与意义 (1)1.2 国内外研究现状 (2)1.3 论文组织结构 (3)第二章 Copula选择及检验 (4)2.1 Copula函数的基本概念 (4)2.1.1 Copula函数定义及性质 (4)2.1.2 阿基米德Copula (5)2.1.3 相关性度量 (6)2.2 常用的二元Archimedean Copula函数与相关性分析 (8)2.2.1 Gumbel Copula函数 (8)2.2.2 Clayton Copula函数 (9)2.2.3 Frank Copula函数 (10)2.3 Copula模型参数估计 (11)2.3.1 Genest and Rivest的非参数估计法 (11)2.3.2 极大似然估计法(The Maximum Likelihood Method) (12)2.3.3 边缘分布函数推断法(The method of Inference of Functionsfor Margins) (13)2.3.4 典型极大似然法(The Canonical Maximum Likelihood Method) (13)2.4 Copula的检验 (13)2.4.1 Klugman-Parsa法则 (13)2.4.2 Copula分布函数检验法则 (14)2.4.3 非参数检验法则 (14)第三章 基于Copula的VaR分析计算 (15)3.1 VaR的基本概念 (15)3.1.1 VaR的定义 (15)3.1.2 VaR的计算要素 (16)3.2 基于Copula的投资组合VaR的计算 (16)3.2.1 Copula-VaR的解析方法 (16)3.2.2 用Copula变换相关系数的VaR分析方法 (17)3.2.3 基于Copula的蒙特卡洛模拟法 (18)3.2.4 实证分析 (19)3.3 基于三维Copula的VaR计算 (25)3.3.1 多元阿基米德Copula的构造 (25)3.3.2 基于Copula的Monte Carlo模拟法 (26)3.3.3 实证分析 (27)第四章 混合Copula的构造与Bootstrap方法的应用 (30)4.1 混合Copula的构造与应用 (30)4.1.1 基于Bayes理论的混合Copula构造 (30)4.1.2 实证分析 (32)4.2 Bootstrap方法的应用 (35)4.2.1 Bootstrap基本原理 (35)4.2.2 Bootstrap估计Copula参数 (36)第五章 结论与展望 (38)5.1 结论 (38)5.2 展望 (38)参考文献 (39)在校期间研究成果 (42)致 谢 (43)附录 数据 (44)附录 程序 (50)第一章 绪论1.1 研究背景与意义当今金融市场的发展达到了空前的规模,国际化、自由化、证券化、金融创新得到了飞速发展,但其不稳定因素也随之增加,脆弱性体现了出来。
Copula方法简介PPT课件

計算分券[C,D]在每個t付 息時點資產池期望損失
現金流入=現金流出
計算分券投資人風險中立測度 下之期望現金流入(premium lag)
計算出分券[C,D2]信0用21價差
3
Copula原理及其運用
Copula函數通常為多變量之累積機率分配,假設n個隨 機變數X1,…,Xn,其所有經過機率轉換的邊際累積機率 分配皆服從均勻分配U(0,1)。
1
0
0
A0.39565 0.9184
0
0.46036 0.076963 0.88439
0.46069 0.11869 0.13764
0
0
0
0.86875
步驟2:從常態分配中抽取一組獨立的隨機亂數
0 .2 0 2 9 2
x
1
.
5
1
3
1
1 .1 2 6 4
0
1.將R進行cholesky分解A 2.抽取隨機亂數Z 3.產生違約時點T=F-1(AZ)
違約時間點相關係數 矩陣R、自由度ν
相關參數α
計算標的資產池內資產違約時點
1.利用條件抽樣法,抽出具違約相關邊際違約機率U 2. .產生違約時點T=F-1(U)
計算CDO資產池違約損失機率
蒙地卡羅法
計算分券投資人風險中立測度 下之期望現金流出(default lag)
T
Theexpectedloss:0 B(0, t)(1R)f (t)dt
expectedexcesspaymentt expectedloss
Wt[1
F(t)]
(1
R)f
(t)
0h(t)
2021
Wt 1 R
8
copula函数 python实现
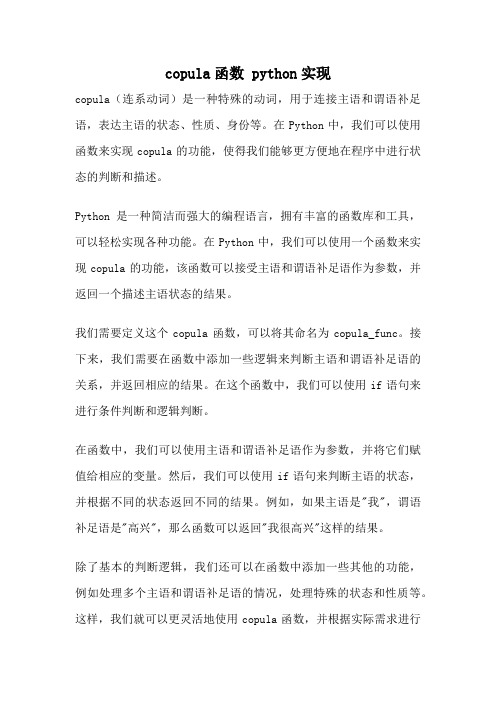
copula函数 python实现copula(连系动词)是一种特殊的动词,用于连接主语和谓语补足语,表达主语的状态、性质、身份等。
在Python中,我们可以使用函数来实现copula的功能,使得我们能够更方便地在程序中进行状态的判断和描述。
Python是一种简洁而强大的编程语言,拥有丰富的函数库和工具,可以轻松实现各种功能。
在Python中,我们可以使用一个函数来实现copula的功能,该函数可以接受主语和谓语补足语作为参数,并返回一个描述主语状态的结果。
我们需要定义这个copula函数,可以将其命名为copula_func。
接下来,我们需要在函数中添加一些逻辑来判断主语和谓语补足语的关系,并返回相应的结果。
在这个函数中,我们可以使用if语句来进行条件判断和逻辑判断。
在函数中,我们可以使用主语和谓语补足语作为参数,并将它们赋值给相应的变量。
然后,我们可以使用if语句来判断主语的状态,并根据不同的状态返回不同的结果。
例如,如果主语是"我",谓语补足语是"高兴",那么函数可以返回"我很高兴"这样的结果。
除了基本的判断逻辑,我们还可以在函数中添加一些其他的功能,例如处理多个主语和谓语补足语的情况,处理特殊的状态和性质等。
这样,我们就可以更灵活地使用copula函数,并根据实际需求进行扩展和修改。
在使用copula函数时,我们可以将其作为其他程序的一部分来调用,也可以直接在交互式环境中使用。
无论是哪种方式,我们都可以得到一个描述主语状态的结果,以便更好地理解和处理数据。
总结一下,copula函数的实现可以帮助我们更方便地描述主语的状态、性质和身份等。
通过使用函数,我们可以在Python程序中轻松地进行状态的判断和描述,使得我们的程序更加灵活和强大。
使用copula函数,我们可以更好地理解和处理数据,提高程序的可读性和可维护性。
OnDefaultCorrelation债务违约相关性的度量
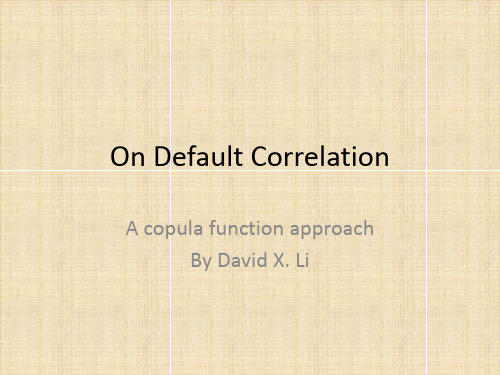
•
1
(t) ( ln t) ,[1](s) es , 1
• (3)Frank Copula:
(t)
ln
e t e
1 1
,
先来看一个未使用各个信用资产相关性数据的例子:
而更有效的一个方法是:
• 相关度 是什么变量的相关度?
而传统意义上的违约概率相关度是: 先用Copula函数的结论得出资产组合的概率密度函数
• 对于任意一项信用资产,“距违约时间”的分布构成了该项信 用资产的“违约期限结构”。
违约期限结构的表现形式——信用曲线
先做一些定义: • 连续随机变量“距违约时间”T(time-until-default) ,
它表示从现在到违约(default)事件发生时的时间长度。 • F(t)表示在t时刻已经违约的概率 • S(t) 表示在t时刻还没有违约的概率,它也被称为生存
distinguishing risk and uncertainty
夫子自道
• “People got very excited about the Gaussian copula because of its mathematical elegance, but the thing never worked. Co-association between securities is not measurable using correlation”; in other words because past history is not predictive of the future. “Anything that relies on correlation is charlatanism(庸医术)."
- 1、下载文档前请自行甄别文档内容的完整性,平台不提供额外的编辑、内容补充、找答案等附加服务。
- 2、"仅部分预览"的文档,不可在线预览部分如存在完整性等问题,可反馈申请退款(可完整预览的文档不适用该条件!)。
- 3、如文档侵犯您的权益,请联系客服反馈,我们会尽快为您处理(人工客服工作时间:9:00-18:30)。
The RiskMetrics GroupWorking Paper Number99-07On Default Correlation:A Copula Function ApproachDavid X.LiThis draft:April2000First draft:September199944Wall St.New York,NY10005david.li@ On Default Correlation:A Copula Function ApproachDavid X.LiApril2000AbstractThis paper studies the problem of default correlation.Wefirst introduce a random variable called“time-until-default”to denote the survival time of each defaultable entity orfinancial instrument,and define the default correlation between two credit risks as the correlation coefficient between their survival times. Then we argue why a copula function approach should be used to specify the joint distribution of survival times after marginal distributions of survival times are derived from market information,such as risky bond prices or asset swap spreads.The definition and some basic properties of copula functions are given.We show that the current CreditMetrics approach to default correlation through asset correlation is equivalent to using a normal copula function.Finally,we give some numerical examples to illustrate the use of copula functions in the valuation of some credit derivatives,such as credit default swaps and first-to-default contracts.1IntroductionThe rapidly growing credit derivative market has created a new set offinancial instruments which can be used to manage the most important dimension offinancial risk-credit risk.In addition to the standard credit derivative products,such as credit default swaps and total return swaps based upon a single underlying credit risk,many new products are now associated with a portfolio of credit risks.A typical example is the product with payment contingent upon the time and identity of thefirst or second-to-default in a given credit risk portfolio.Variations include instruments with payment contingent upon the cumulative loss before a given time in the future.The equity tranche of a collateralized bond obligation(CBO)or a collateralized loan obligation(CLO)is yet another variation,where the holder of the equity tranche incurs thefirst loss. Deductible and stop-loss in insurance products could also be incorporated into the basket credit derivatives structure.As morefinancialfirms try to manage their credit risk at the portfolio level and the CBO/CLO market continues to expand,the demand for basket credit derivative products will most likely continue to grow.Central to the valuation of the credit derivatives written on a credit portfolio is the problem of default correlation.The problem of default correlation even arises in the valuation of a simple credit default swap with one underlying reference asset if we do not assume the independence of default between the reference asset and the default swap seller.Surprising though it may seem,the default correlation has not been well defined and understood infinance.Existing literature tends to define default correlation based on discrete events which dichotomize according to survival or nonsurvival at a critical period such as one year.For example,if we denoteq A=Pr[E A],q B=Pr[E B],q AB=Pr[E A E B]where E A,E B are defined as the default events of two securities A and B over1year.Then the default correlationρbetween two default events E A and E B,based on the standard definition of correlation of two random variables,are defined as follows1ρ=q AB−q A·q B√q A(1−q A)q B(1−q B).(1)This discrete event approach has been taken by Lucas[1995].Hereafter we simply call this definition of default correlation the discrete default correlation.However the choice of a specific period like one year is more or less arbitrary.It may correspond with many empirical studies of default rate over one year period.But the dependence of default correlation on a specific time interval has its disadvantages.First,default is a time dependent event,and so is default correlation.Let us take the survival time of a human being as an example.The probability of dying within one year for a person aged50years today is about0.6%,but the probability of dying for the same person within50years is almost a sure event.Similarly default correlation is a time dependent quantity.Let us now take the survival times of a couple,both aged50years today.The correlation between the two discrete events that each dies within one year is very small.But the correlation between the two discrete events that each dies within100 years is1.Second,concentration on a single period of one year wastes important information.There are empirical studies which show that the default tendency of corporate bonds is linked to their age since issue. Also there are strong links between the economic cycle and defaults.Arbitrarily focusing on a one year period neglects this important information.Third,in the majority of credit derivative valuations,what we need is not the default correlation of two entities over the next year.We may need to have a joint distribution of survival times for the next10years.Fourth,the calculation of default rates as simple proportions is possible only when no samples are censored during the one year period1.This paper introduces a few techniques used in survival analysis.These techniques have been widely applied to other areas,such as life contingencies in actuarial science and industry life testing in reliability studies, which are similar to the credit problems we encounter here.Wefirst introduce a random variable called1A company who is observed,default free,by Moody’s for5-years and then withdrawn from the Moody’s study must have a survival time exceeding5years.Another company may enter into Moody’s study in the middle of a year,which implies that Moody’s observes the company for only half of the one year observation period.In the survival analysis of statistics,such incomplete observation of default time is called censoring.According to Moody’s studies,such incomplete observation does occur in Moody’s credit default samples.2“time-until-default”to denote the survival time of each defaultable entity orfinancial instrument.Then, we define the default correlation of two entities as the correlation between their survival times.In credit derivative valuation we needfirst to construct a credit curve for each credit risk.A credit curve gives all marginal conditional default probabilities over a number of years.This curve is usually derived from the risky bond spread curve or asset swap spreads observed currently from the market.Spread curves and asset swap spreads contain information on default probabilities,recovery rate and liquidity factors etc.Assuming an exogenous recovery rate and a default treatment,we can extract a credit curve from the spread curve or asset swap spread curve.For two credit risks,we would obtain two credit curves from market observable information.Then,we need to specify a joint distribution for the survival times such that the marginal distributions are the credit curves.Obviously,this problem has no unique solution.Copula functions used in multivariate statistics provide a convenient way to specify the joint distribution of survival times with given marginal distributions.The concept of copula functions,their basic properties,and some commonly used copula functions are introduced.Finally,we give a few numerical examples of credit derivative valuation to demonstrate the use of copula functions and the impact of default correlation.2Characterization of Default by Time-Until-DefaultIn the study of default,interest centers on a group of individual companies for each of which there is defined a point event,often called default,(or survival)occurring after a length of time.We introduce a random variable called the time-until-default,or simply survival time,for a security,to denote this length of time. This random variable is the basic building block for the valuation of cashflows subject to default.To precisely determine time-until-default,we need:an unambiguously defined time origin,a time scale for measuring the passage of time,and a clear definition of default.We choose the current time as the time origin to allow use of current market information to build credit curves.The time scale is defined in terms of years for continuous models,or number of periods for discrete models.The meaning of default is defined by some rating agencies,such as Moody’s.32.1Survival FunctionLet us consider an existing security A.This security’s time-until-default,T A,is a continuous random variable which measures the length of time from today to the time when default occurs.For simplicity we just use T which should be understood as the time-until-default for a specific security A.Let F(t)denote the distribution function of T,F(t)=Pr(T≤t),t≥0(2)and setS(t)=1−F(t)=Pr(T>t),t≥0.(3)We also assume that F(0)=0,which implies S(0)=1.The function S(t)is called the survival function. It gives the probability that a security will attain age t.The distribution of T A can be defined by specifying either the distribution function F(t)or the survival function S(t).We can also define a probability density function as followsf(t)=F (t)=−S (t)=lim→0+Pr[t≤T<t+ ].To make probability statements about a security which has survived x years,the future life time for this security is T−x|T>x.We introduce two more notationst q x=Pr[T−x≤t|T>x],t≥0t p x=1−t q x=Pr[T−x>t|T>x],t≥0.(4)The symbol t q x can be interpreted as the conditional probability that the security A will default within the next t years conditional on its survival for x years.In the special case of X=0,we havet p0=S(t)x≥0.4If t=1,we use the actuarial convention to omit the prefix1in the symbols t q x and t p x,and we havep x=Pr[T−x>1|T>x]q x=Pr[T−x≤1|T>x].The symbol q x is usually called the marginal default probability,which represents the probability of default in the next year conditional on the survival until the beginning of the year.A credit curve is then simply defined as the sequence of q0,q1,···,q n in discrete models.2.2Hazard Rate FunctionThe distribution function F(t)and the survival function S(t)provide two mathematically equivalent ways of specifying the distribution of the random variable time-until-default,and there are many other equiva-lent functions.The one used most frequently by statisticians is the hazard rate function which gives the instantaneous default probability for a security that has attained age x.Pr[x<T≤x+ x|T>x]=F(x+ x)−F(x)1−F(x)≈f(x) x 1−F(x).The functionf(x)1−F(x)has a conditional probability density interpretation:it gives the value of the conditional probability density function of T at exact age x,given survival to that time.Let’s denote it as h(x),which is usually called the hazard rate function.The relationship of the hazard rate function with the distribution function and survival function is as follows5h(x)=f(x)1−F(x)=−S (x)S(x).(5)Then,the survival function can be expressed in terms of the hazard rate function,S(t)=e− th(s)ds.Now,we can express t q x and t p x in terms of the hazard rate function as followst p x=e− th(s+x)ds,(6)t q x=1−e− th(s+x)ds.In addition,F(t)=1−S(t)=1−e− th(s)ds,andf(t)=S(t)·h(t).(7) which is the density function for T.A typical assumption is that the hazard rate is a constant,h,over certain period,such as[x,x+1].In this case,the density function isf(t)=he−ht6which shows that the survival time follows an exponential distribution with parameter h.Under this assump-tion,the survival probability over the time interval[x,x+t]for0<t≤1ist p x=1−t q x=e− th(s)ds=e−ht=(p x)twhere p x is the probability of survival over one year period.This assumption can be used to scale down the default probability over one year to a default probability over a time interval less than one year. Modelling a default process is equivalent to modelling a hazard function.There are a number of reasons why modelling the hazard rate function may be a good idea.First,it provides us information on the immediate default risk of each entity known to be alive at exact age t.Second,the comparisons of groups of individuals are most incisively made via the hazard rate function.Third,the hazard rate function based model can be easily adapted to more complicated situations,such as where there is censoring or there are several types of default or where we would like to consider stochastic defaultfluctuations.Fourth,there are a lot of similarities between the hazard rate function and the short rate.Many modeling techniques for the short rate processes can be readily borrowed to model the hazard rate.Finally,we can define the joint survival function for two entities A and B based on their survival times T A and T B,S TA T B(s,t)=Pr[T A>s,T B>t].The joint distributional function isF(s,t)=Pr[T A≤s,T B≤t]=1−S TA (s)−S TB(t)+S TA T B(s,t).The aforementioned concepts and results can be found in survival analysis books,such as Bowers et al. [1997],Cox and Oakes[1984].73Definition of Default CorrelationsThe default correlation of two entities A and B can then be defined with respect to their survival times T A and T B as followsρAB=Cov(T A,T B)√V ar(T A)V ar(T B)=E(T A T B)−E(T A)E(T B)√V ar(T A)V ar(T B).(8)Hereafter we simply call this definition of default correlation the survival time correlation.The survival time correlation is a much more general concept than that of the discrete default correlation based on a one period.If we have the joint distribution f(s,t)of two survival times T A,T B,we can calculate the discrete default correlation.For example,if we defineE1=[T A<1],E2=[T B<1],then the discrete default correlation can be calculated using equation(1)with the following calculationq12=Pr[E1E2]= 11f(s,t)dsdtq1= 1f A(s)dsq2= 1f B(t)dt.However,knowing the discrete default correlation over one year period does not allow us to specify the survival time correlation.4The Construction of the Credit CurveThe distribution of survival time or time-until-default can be characterized by the distribution function, survival function or hazard rate function.It is shown in Section2that all default probabilities can be8calculated once a characterization is given.The hazard rate function used to characterize the distribution of survival time can also be called a credit curve due to its similarity to a yield curve.But the basic question is: how do we obtain the credit curve or the distribution of survival time for a given credit?There exist three methods to obtain the term structure of default rates:(i)Obtaining historical default information from rating agencies;(ii)Taking the Merton option theoretical approach;(iii)Taking the implied approach using market prices of defaultable bonds or asset swap spreads.Rating agencies like Moody’s publish historical default rate studies regularly.In addition to the commonly cited one-year default rates,they also present multi-year default rates.From these rates we can obtain the hazard rate function.For example,Moody’s(see Carty and Lieberman[1997])publishes weighted average cumulative default rates from1to20years.For the B rating,thefirst5years cumulative default rates in percentage are7.27,13.87,19.94,25.03and29.45.From these rates we can obtain the marginal conditional default probabilities.Thefirst marginal conditional default probability in year one is simply the one-year default probability,7.27%.The other marginal conditional default probabilities can be obtained using the following formula:n+1q x=n q x+n p x·q x+n,(9) which simply states that the probability of default over time interval[0,n+1]is the sum of the probability of default over the time interval[0,n],plus the probability of survival to the end of n th year and default in the following ing equation(9)we have the marginal conditional default probability:q x+n=n+1q x−n q x1−n q xwhich results in the marginal conditional default probabilities in year2,3,4,5as7.12%,7.05%,6.36%and 5.90%.If we assume a piecewise constant hazard rate function over each year,then we can obtain the hazard rate function using equation(6).The hazard rate function obtained is given in Figure(1).9Using diffusion processes to describe changes in the value of thefirm,Merton[1974]demonstrated that a firm’s default could be modeled with the Black and Scholes methodology.He showed that stock could be considered as a call option on thefirm with strike price equal to the face value of a single payment debt. Using this framework we can obtain the default probability for thefirm over one period,from which we can translate this default probability into a hazard rate function.Geske[1977]and Delianedis and Geske [1998]extended Merton’s analysis to produce a term structure of default ing the relationship between the hazard rate and the default probabilities we can obtain a credit curve.Alternatively,we can take the implicit approach by using market observable information,such as asset swap spreads or risky corporate bond prices.This is the approach used by most credit derivative trading desks.The extracted default probabilities reflect the market-agreed perception today about the future default tendency of the underlying credit.Li[1998]presents one approach to building the credit curve from market information based on the Duffie and Singleton[1996]default treatment.In that paper the author assumes that there exists a series of bonds with maturity1,2,..,n years,which are issued by the same company and have the same seniority.All of those bonds have observable market prices.From the market price of these bonds we can calculate their yields to ing the yield to maturity of corresponding treasury bonds we obtain a yield spread curve over treasury(or asset swap spreads for a yield spread curve over LIBOR).The credit curve construction is based on this yield spread curve and an exogenous assumption about the recovery rate based on the seniority and the rating of the bonds,and the industry of the corporation.The suggested approach is contrary to the use of historical default experience information provided by rating agencies such as Moody’s.We intend to use market information rather than historical information for the following reasons:•The calculation of profit and loss for a trading desk can only be based on current market information.This current market information reflects the market agreed perception about the evolution of the market in the future,on which the actual profit and loss depend.The default rate derived from current market information may be much different than historical default rates.•Rating agencies use classification variables in the hope that homogeneous risks will be obtained10after classification.This technique has been used elsewhere like in pricing automobile insurance.Unfortunately,classification techniques omit often somefirm specific information.Constructing a credit curve for each credit allows us to use morefirm specific information.•Rating agencies reacts much slower than the market in anticipation of future credit quality.A typical example is the rating agencies reaction to the recent Asian crisis.•Ratings are primarily used to calculate default frequency instead of default severity.However,much of credit derivative value depends on both default frequency and severity.•The information available from a rating agency is usually the one year default probability for each rating group and the rating migration matrix.Neither the transition matrixes,nor the default probabilities are necessarily stable over long periods of time.In addition,many credit derivative products have maturities well beyond one year,which requires the use of long term marginal default probability.It is shown under the Duffie and Singleton approach that a defaultable instrument can be valued as if it is a default free instrument by discounting the defaultable cashflow at a credit risk adjusted discount factor.The credit risk adjusted discount factor or the total discount factor is the product of risk-free discount factor and the pure credit discount factor if the underlying factors affecting default and those affecting the interest rate are independent.Under this framework and the assumption of a piecewise constant hazard rate function,we can derive a credit curve or specify the distribution of the survival time.5Dependent Models-Copula FunctionsLet us study some problems of an n credit ing either the historical approach or the market implicit approach,we can construct the marginal distribution of survival time for each of the credit risks in the portfolio.If we assume mutual independence among the credit risks,we can study any problem associated with the portfolio.However,the independence assumption of the credit risks is obviously not realistic;in reality,the default rate for a group of credits tends to be higher in a recession and lower when the economy11is booming.This implies that each credit is subject to the same set of macroeconomic environment,and that there exists some form of positive dependence among the credits.To introduce a correlation structure into the portfolio,we must determine how to specify a joint distribution of survival times,with given marginal distributions.Obviously,this problem has no unique solution.Generally speaking,knowing the joint distribution of random variables allows us to derive the marginal distributions and the correlation structure among the random variables,but not vice versa.There are many different techniques in statistics which allow us to specify a joint distribution function with given marginal distributions and a correlation structure.Among them,copula function is a simple and convenient approach.We give a brief introduction to the concept of copula function in the next section.5.1Definition and Basic Properties of Copula FunctionA copula function is a function that links or marries univariate marginals to their full multivariate distribution. For m uniform random variables,U1,U2,···,U m,the joint distribution function C,defined asC(u1,u2,···,u m,ρ)=Pr[U1≤u1,U2≤u2,···,U m≤u m]can also be called a copula function.Copula functions can be used to link marginal distributions with a joint distribution.For given univariate marginal distribution functions F1(x1),F2(x2),···,F m(x m),the functionC(F1(x1),F2(x2),···,F m(x m))=F(x1,x2,···x m),which is defined using a copula function C,results in a multivariate distribution function with univariate marginal distributions as specified F1(x1),F2(x2),···,F m(x m).This property can be easily shown as follows:12C(F 1(x 1),F 2(x 2),···,F m (x m ),ρ)=Pr [U 1≤F 1(x 1),U 2≤F 2(x 2),···,U m ≤F m (x m )]=Pr F −11(U 1)≤x 1,F −12(U 2)≤x 2,···,F −1m (U m )≤x m=Pr [X 1≤x 1,X 2≤x 2,···,X m ≤x m ]=F (x 1,x 2,···x m ).The marginal distribution of X i isC(F 1(+∞),F 2(+∞),···F i (x i ),···,F m (+∞),ρ)=Pr [X 1≤+∞,X 2≤+∞,···,X i ≤x i ,X m ≤+∞]=Pr[X i ≤x i ]=F i (x i ).Sklar [1959]established the converse.He showed that any multivariate distribution function F can be written in the form of a copula function.He proved the following:If F (x 1,x 2,···x m )is a joint multivariate distribution function with univariate marginal distribution functions F 1(x 1),F 2(x 2),···,F m (x m ),then there exists a copula function C(u 1,u 2,···,u m )such thatF (x 1,x 2,···x m )=C(F 1(x 1),F 2(x 2),···,F m (x m )).If each F i is continuous then C is unique.Thus,copula functions provide a unifying and flexible way to study multivariate distributions.For simplicity’s sake,we discuss only the properties of bivariate copula functions C(u,v,ρ)for uniform random variables U and V ,defined over the area {(u,v)|0<u ≤1,0<v ≤1},where ρis a correlation parameter.We call ρsimply a correlation parameter since it does not necessarily equal the usual correlation coefficient defined by Pearson,nor Spearman’s Rho,nor Kendall’s Tau.The bivariate copula function has the following properties:(i)Since U and V are positive random variables,C(0,v,ρ)=C(u,0,ρ)=0.13(ii)Since U and V are bounded above by1,the marginal distributions can be obtained by C(1,v,ρ)=v, C(u,1,ρ)=u.(iii)For independent random variables U and V,C(u,v,ρ)=uv.Frechet[1951]showed there exist upper and lower bounds for a copula functionmax(0,u+v−1)≤C(u,v)≤min(u,v).The multivariate extension of Frechet bounds is given by Dall’Aglio[1972].5.2Some Common Copula FunctionsWe present a few copula functions commonly used in biostatistics and actuarial science.Frank Copula The Frank copula function is defined asC(u,v)=1αln1+(eαu−1)(eαv−1)eα−1,−∞<α<∞.Bivariate NormalC(u,v)= 2( −1(u), −1(v),ρ),−1≤ρ≤1,(10) where 2is the bivariate normal distribution function with correlation coefficientρ,and −1is the inverse of a univariate normal distribution function.As we shall see later,this is the copula function used in CreditMetrics.Bivariate Mixture Copula Function We can form new copula function using existing copula functions. If the two uniform random variables u and v are independent,we have a copula function C(u,v)=uv.If the two random variables are perfect correlated we have the copula function C(u,v)=min(u,v).Mixing the two copula functions by a mixing coefficient(ρ>0)we obtain a new copula function as follows14C(u,v)=(1−ρ)uv+ρmin(u,v),ifρ>0.Ifρ≤0we haveC(u,v)=(1+ρ)uv−ρ(u−1+v) (u−1+v),ifρ≤0,where(x)=1,if x≥0=0,if x<0.5.3Copula Function and Correlation MeasurementTo compare different copula functions,we need to have a correlation measurement independent of marginal distributions.The usual Pearson’s correlation coefficient,however,depends on the marginal distributions (See Lehmann[1966]).Both Spearman’s Rho and Kendall’s Tau can be defined using a copula function only as followsρs=12[C(u,v)−uv]dudv,τ=4C(u,v)dC(u,v)−1.Comparisons between results using different copula functions should be based on either a common Spear-man’s Rho or a Kendall’s Tau.Further examination of copula functions can be found in a survey paper by Frees and Valdez[1988]and a recent book by Nelsen[1999].155.4The Calibration of Default Correlation in Copula FunctionHaving chosen a copula function,we need to compute the pairwise correlation of survival ing the CreditMetrics(Gupton et al.[1997])asset correlation approach,we can obtain the default correlation of two discrete events over one year period.As it happens,CreditMetrics uses the normal copula function in its default correlation formula even though it does not use the concept of copula function explicitly.First let us summarize how CreditMetrics calculates joint default probability of two credits A and B.Suppose the one year default probabilities for A and B are q A and q B.CreditMetrics would use the following steps•Obtain Z A and Z B such thatq A=Pr[Z<Z A]q B=Pr[Z<Z B]where Z is a standard normal random variable•Ifρis the asset correlation,the joint default probability for credit A and B is calculated as follows,Pr[Z<Z A,Z<Z B]=Z A−∞Z B−∞φ2(x,y|ρ)dxdy= 2(Z A,Z B,ρ)(11)whereφ2(x,y|ρ)is the standard bivariate normal density function with a correlation coefficientρ,and 2is the bivariate accumulative normal distribution function.If we use a bivariate normal copula function with a correlation parameterγ,and denote the survival times for A and B as T A and T B,the joint default probability can be calculated as followsPr[T A<1,T B<1]= 2( −1(F A(1)), −1(F B(1),γ)(12) where F A and F B are the distribution functions for the survival times T A and T B.If we notice that16。