2016_ICM_Problem_D
华工第十六届数理大赛赛题发布会
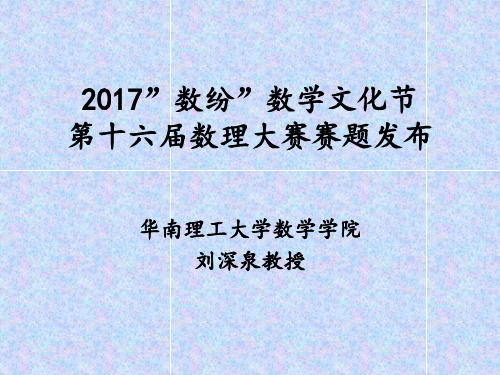
2014全国数学建模竞赛题目 2015美国数学建模竞赛题目
2014全国数学建模竞赛题目 A题 嫦娥三号软着陆轨道设计与控制策略 B题 创意平板折叠桌 C题 生猪养殖场的经营管理 D题 储药柜的设计
2015 MCM&ICM Problems • Problem A:Eradicating Ebola • Problem B:Searching for a lost plane • Problem C:Managing Human Capital in Organizations • Problem D:Is it sustainable?
• Convex Optimization • Duality-Theory • Lagrange Multipliers • Kernels function
Deep Learning Neural Network
• • • • AlphaGo Zero强化学习战胜AlphaGo 人工智能推动数学建模 数学建模制造新的信息机器 丘成桐:工程上取得很大发展 但理论基础仍非常 薄弱 • 人工智能需要一个可以被证明的理论作为基础。 • 人工智能需要新数学理论
SVHN – real world image dataset
Image classification
Convolutiona Neural Network
全 连 接 卷 积
池 化
CNN- 图像分类和场景特色
数学建模生产的图形处理机器
LSTM- 翻译语言和语音识别机器
RNN - 语音识别和自然语言分析
2017A题
CT系统参数标定及成像
• 请建立相应的数学模型和算法,解决以下问题: • (1) 在正方形托盘上放置两个均匀固体介质组成的标定模板,模板的 几何信息如图2所示,相应的数据文件见附件1,其中每一点的数值反 映了该点的吸收强度,这里称为“吸收率”。对应于该模板的接收信 息见附件2。请根据这一模板及其接收信息,确定CT系统旋转中心在 正方形托盘中的位置、探测器单元之间的距离以及该CT系统使用的X 射线的180个方向。 • (2) 附件3是利用上述CT系统得到的某未知介质的接收信息。利用(1) 中得到的标定参数,确定该未知介质在正方形托盘中的位置、几何形 状和吸收率等信息。另外,请具体给出图3所给的10个位置处的吸收 率,相应的数据文件见附件4。 • (3) 附件5是利用上述CT系统得到的另一个未知介质的接收信息。利 用(1)中得到的标定参数,给出该未知介质的相关信息。另外,请具 体给出图3所给的10个位置处的吸收率。 • (4) 分析(1)中参数标定的精度和稳定性。在此基础上自行设计新模 板、建立对应的标定模型,以改进标定精度和稳定性,并说明理由。
基于主动学习和二次有理核的模型无关局部解释方法
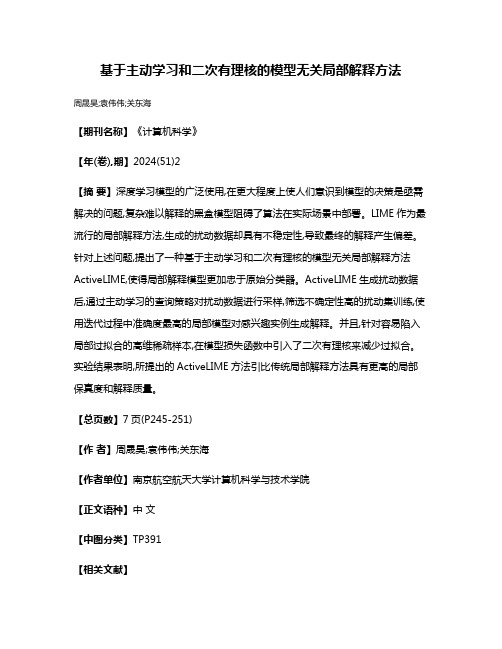
基于主动学习和二次有理核的模型无关局部解释方法
周晟昊;袁伟伟;关东海
【期刊名称】《计算机科学》
【年(卷),期】2024(51)2
【摘要】深度学习模型的广泛使用,在更大程度上使人们意识到模型的决策是亟需解决的问题,复杂难以解释的黑盒模型阻碍了算法在实际场景中部署。
LIME作为最流行的局部解释方法,生成的扰动数据却具有不稳定性,导致最终的解释产生偏差。
针对上述问题,提出了一种基于主动学习和二次有理核的模型无关局部解释方法ActiveLIME,使得局部解释模型更加忠于原始分类器。
ActiveLIME生成扰动数据后,通过主动学习的查询策略对扰动数据进行采样,筛选不确定性高的扰动集训练,使用迭代过程中准确度最高的局部模型对感兴趣实例生成解释。
并且,针对容易陷入局部过拟合的高维稀疏样本,在模型损失函数中引入了二次有理核来减少过拟合。
实验结果表明,所提出的ActiveLIME方法引比传统局部解释方法具有更高的局部保真度和解释质量。
【总页数】7页(P245-251)
【作者】周晟昊;袁伟伟;关东海
【作者单位】南京航空航天大学计算机科学与技术学院
【正文语种】中文
【中图分类】TP391
【相关文献】
1.一种基于因果强度的局部因果结构主动学习方法
2.基于主动形状模型算法的局部灰度模型的加权改进方法
3.基于局部主动轮廓模型的飞机壁板铆接孔定位方法研究
4.基于模糊核聚类和主动学习的异常检测方法
5.基于核极限学习机的快速主动学习方法及其软测量应用
因版权原因,仅展示原文概要,查看原文内容请购买。
2016美国数模竞赛题目中文翻译
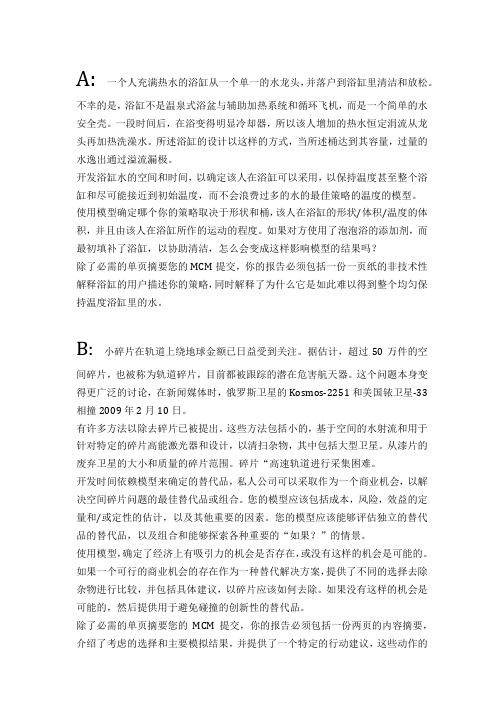
A: 一个人充满热水的浴缸从一个单一的水龙头,并落户到浴缸里清洁和放松。
不幸的是,浴缸不是温泉式浴盆与辅助加热系统和循环飞机,而是一个简单的水安全壳。
一段时间后,在浴变得明显冷却器,所以该人增加的热水恒定涓流从龙头再加热洗澡水。
所述浴缸的设计以这样的方式,当所述桶达到其容量,过量的水逸出通过溢流漏极。
开发浴缸水的空间和时间,以确定该人在浴缸可以采用,以保持温度甚至整个浴缸和尽可能接近到初始温度,而不会浪费过多的水的最佳策略的温度的模型。
使用模型确定哪个你的策略取决于形状和桶,该人在浴缸的形状/体积/温度的体积,并且由该人在浴缸所作的运动的程度。
如果对方使用了泡泡浴的添加剂,而最初填补了浴缸,以协助清洁,怎么会变成这样影响模型的结果吗?除了必需的单页摘要您的MCM提交,你的报告必须包括一份一页纸的非技术性解释浴缸的用户描述你的策略,同时解释了为什么它是如此难以得到整个均匀保持温度浴缸里的水。
B: 小碎片在轨道上绕地球金额已日益受到关注。
据估计,超过50万件的空间碎片,也被称为轨道碎片,目前都被跟踪的潜在危害航天器。
这个问题本身变得更广泛的讨论,在新闻媒体时,俄罗斯卫星的Kosmos-2251和美国铱卫星-33相撞2009年2月10日。
有许多方法以除去碎片已被提出。
这些方法包括小的,基于空间的水射流和用于针对特定的碎片高能激光器和设计,以清扫杂物,其中包括大型卫星。
从漆片的废弃卫星的大小和质量的碎片范围。
碎片“高速轨道进行采集困难。
开发时间依赖模型来确定的替代品,私人公司可以采取作为一个商业机会,以解决空间碎片问题的最佳替代品或组合。
您的模型应该包括成本,风险,效益的定量和/或定性的估计,以及其他重要的因素。
您的模型应该能够评估独立的替代品的替代品,以及组合和能够探索各种重要的“如果?”的情景。
使用模型,确定了经济上有吸引力的机会是否存在,或没有这样的机会是可能的。
如果一个可行的商业机会的存在作为一种替代解决方案,提供了不同的选择去除杂物进行比较,并包括具体建议,以碎片应该如何去除。
扩散模型损失函数推导
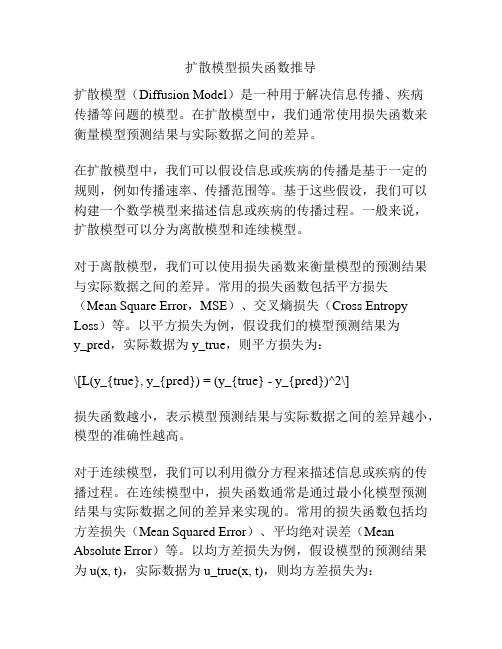
扩散模型损失函数推导扩散模型(Diffusion Model)是一种用于解决信息传播、疾病传播等问题的模型。
在扩散模型中,我们通常使用损失函数来衡量模型预测结果与实际数据之间的差异。
在扩散模型中,我们可以假设信息或疾病的传播是基于一定的规则,例如传播速率、传播范围等。
基于这些假设,我们可以构建一个数学模型来描述信息或疾病的传播过程。
一般来说,扩散模型可以分为离散模型和连续模型。
对于离散模型,我们可以使用损失函数来衡量模型的预测结果与实际数据之间的差异。
常用的损失函数包括平方损失(Mean Square Error,MSE)、交叉熵损失(Cross Entropy Loss)等。
以平方损失为例,假设我们的模型预测结果为y_pred,实际数据为y_true,则平方损失为:\[L(y_{true}, y_{pred}) = (y_{true} - y_{pred})^2\]损失函数越小,表示模型预测结果与实际数据之间的差异越小,模型的准确性越高。
对于连续模型,我们可以利用微分方程来描述信息或疾病的传播过程。
在连续模型中,损失函数通常是通过最小化模型预测结果与实际数据之间的差异来实现的。
常用的损失函数包括均方差损失(Mean Squared Error)、平均绝对误差(Mean Absolute Error)等。
以均方差损失为例,假设模型的预测结果为u(x, t),实际数据为u_true(x, t),则均方差损失为:\[L(u_{true}, u_{pred}) = \int \int (u_{true}(x, t) - u_{pred}(x, t))^2 dx dt\]通过最小化损失函数,我们可以得到最优的模型参数,从而使得模型的预测结果与实际数据最为接近。
需要注意的是,损失函数的具体形式取决于问题的特点和模型的设计。
在实际应用中,我们需要根据具体情况选择合适的损失函数,以达到最佳的模型效果。
2016美国大学生数学建模大赛C题特等奖(原版论文)C42939Tsinghua University, China
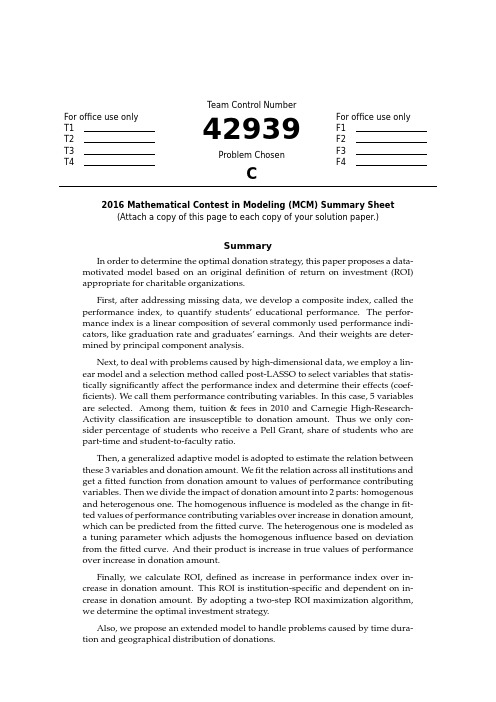
For office use only T1T2T3T4T eam Control Number42939Problem ChosenCFor office use onlyF1F2F3F42016Mathematical Contest in Modeling(MCM)Summary Sheet (Attach a copy of this page to each copy of your solution paper.)SummaryIn order to determine the optimal donation strategy,this paper proposes a data-motivated model based on an original definition of return on investment(ROI) appropriate for charitable organizations.First,after addressing missing data,we develop a composite index,called the performance index,to quantify students’educational performance.The perfor-mance index is a linear composition of several commonly used performance indi-cators,like graduation rate and graduates’earnings.And their weights are deter-mined by principal component analysis.Next,to deal with problems caused by high-dimensional data,we employ a lin-ear model and a selection method called post-LASSO to select variables that statis-tically significantly affect the performance index and determine their effects(coef-ficients).We call them performance contributing variables.In this case,5variables are selected.Among them,tuition&fees in2010and Carnegie High-Research-Activity classification are insusceptible to donation amount.Thus we only con-sider percentage of students who receive a Pell Grant,share of students who are part-time and student-to-faculty ratio.Then,a generalized adaptive model is adopted to estimate the relation between these3variables and donation amount.Wefit the relation across all institutions and get afitted function from donation amount to values of performance contributing variables.Then we divide the impact of donation amount into2parts:homogenous and heterogenous one.The homogenous influence is modeled as the change infit-ted values of performance contributing variables over increase in donation amount, which can be predicted from thefitted curve.The heterogenous one is modeled as a tuning parameter which adjusts the homogenous influence based on deviation from thefitted curve.And their product is increase in true values of performance over increase in donation amount.Finally,we calculate ROI,defined as increase in performance index over in-crease in donation amount.This ROI is institution-specific and dependent on in-crease in donation amount.By adopting a two-step ROI maximization algorithm, we determine the optimal investment strategy.Also,we propose an extended model to handle problems caused by time dura-tion and geographical distribution of donations.A Letter to the CFO of the Goodgrant FoundationDear Chiang,Our team has proposed a performance index quantifying the students’educational per-formance of each institution and defined the return of investment(ROI)appropriately for a charitable organization like Goodgrant Foundation.A mathematical model is built to help predict the return of investment after identifying the mechanism through which the donation generates its impact on the performance.The optimal investment strategy is determined by maximizing the estimated return of investment.More specifically,the composite performance index is developed after taking all the pos-sible performance indicators into consideration,like graduation rate and graduates’earnings. The performance index is constructed to represents the performance of the school as well as the positive effect that a college brings to students and the community.From this point of view, our definition manages to capture social benefits of donation.And then we adopt a variable selection method tofind out performance contributing vari-ables,which are variables that strongly affect the performance index.Among all the perfor-mance contributing variables we select,three variables which can be directly affected by your generous donation are kept to predict ROI:percentage of students who receive a Pell Grant, share of students who are part-time and student-to-faculty ratio.Wefitted a relation between these three variables and the donation amount to predict change in value of each performance contributing variable over your donation amount.And we calculate ROI,defined as increase in the performance index over your donation amount, by multiplying change in value of each performance contributing variable over your donation amount and each performance contributing variable’s effect on performance index,and then summing up the products of all performance contributing variables.The optimal investment strategy is decided after maximizing the return of investment according to an algorithm for selection.In conclusion,our model successfully produced an investment strategy including a list of target institutions and investment amount for each institution.(The list of year1is attached at the end of the letter).The time duration for the investment could also be determined based on our model.Since the model as well as the evaluation approach is fully data-motivated with no arbitrary criterion included,it is rather adaptable for solving future philanthropic educational investment problems.We have a strong belief that our model can effectively enhance the efficiency of philan-thropic educational investment and provides an appropriate as well as feasible way to best improve the educational performance of students.UNITID names ROI donation 197027United States Merchant Marine Academy21.85%2500000 102711AVTEC-Alaska’s Institute of Technology21.26%7500000 187745Institute of American Indian and Alaska Native Culture20.99%2000000 262129New College of Florida20.69%6500000 216296Thaddeus Stevens College of Technology20.66%3000000 229832Western Texas College20.26%10000000 196158SUNY at Fredonia20.24%5500000 234155Virginia State University20.04%10000000 196200SUNY College at Potsdam19.75%5000000 178615Truman State University19.60%3000000 199120University of North Carolina at Chapel Hill19.51%3000000 101648Marion Military Institute19.48%2500000187912New Mexico Military Institute19.31%500000 227386Panola College19.28%10000000 434584Ilisagvik College19.19%4500000 199184University of North Carolina School of the Arts19.15%500000 413802East San Gabriel Valley Regional Occupational Program19.09%6000000 174251University of Minnesota-Morris19.09%8000000 159391Louisiana State University and Agricultural&Mechanical Col-19.07%8500000lege403487Wabash Valley College19.05%1500000 Yours Sincerely,Team#42939An Optimal Strategy of Donation for Educational PurposeControl Number:#42939February,2016Contents1Introduction51.1Statement of the Problem (5)1.2Baseline Model (5)1.3Detailed Definitions&Assumptions (8)1.3.1Detailed Definitions: (8)1.3.2Assumptions: (9)1.4The Advantages of Our Model (9)2Addressing the Missing Values93Determining the Performance Index103.1Performance Indicators (10)3.2Performance Index via Principal-Component Factors (10)4Identifying Performance Contributing Variables via post-LASSO115Determining Investment Strategy based on ROI135.1Fitted Curve between Performance Contributing Variables and Donation Amount145.2ROI(Return on Investment) (15)5.2.1Model of Fitted ROIs of Performance Contributing Variables fROI i (15)5.2.2Model of the tuning parameter P i (16)5.2.3Calculation of ROI (17)5.3School Selection&Investment Strategy (18)6Extended Model186.1Time Duration (18)6.2Geographical Distribution (22)7Conclusions and Discussion22 8Reference23 9Appendix241Introduction1.1Statement of the ProblemThere exists no doubt in the significance of postsecondary education to the development of society,especially with the ascending need for skilled employees capable of complex work. Nevertheless,U.S.ranks only11th in the higher education attachment worldwide,which makes thefinancial support from large charitable organizations necessary.As it’s essential for charitable organizations to maximize the effectiveness of donations,an objective and systematic assessment model is in demand to develop appropriate investment strategies.To achieve this goal,several large foundations like Gates Foundation and Lumina Foundation have developed different evaluation approaches,where they mainly focus on spe-cific indexes like attendance and graduation rate.In other empirical literature,a Forbes ap-proach(Shifrin and Chen,2015)proposes a new indicator called the Grateful Graduates Index, using the median amount of private donations per student over a10-year period to measure the return on investment.Also,performance funding indicators(Burke,2002,Cave,1997,Ser-ban and Burke,1998,Banta et al,1996),which include but are not limited to external indicators like graduates’employment rate and internal indicators like teaching quality,are one of the most prevailing methods to evaluate effectiveness of educational donations.However,those methods also arise with widely acknowledged concerns(Burke,1998).Most of them require subjective choice of indexes and are rather arbitrary than data-based.And they perform badly in a data environment where there is miscellaneous cross-section data but scarce time-series data.Besides,they lack quantified analysis in precisely predicting or measuring the social benefits and the positive effect that the investment can generate,which serves as one of the targets for the Goodgrant Foundation.In accordance with Goodgrant Foundation’s request,this paper provides a prudent def-inition of return on investment(ROI)for charitable organizations,and develops an original data-motivated model,which is feasible even faced with tangled cross-section data and absent time-series data,to determine the optimal strategy for funding.The strategy contains selection of institutions and distribution of investment across institutions,time and regions.1.2Baseline ModelOur definition of ROI is similar to its usual meaning,which is the increase in students’educational performance over the amount Goodgrant Foundation donates(assuming other donationsfixed,it’s also the increase in total donation amount).First we cope with data missingness.Then,to quantify students’educational performance, we develop an index called performance index,which is a linear composition of commonly used performance indicators.Our major task is to build a model to predict the change of this index given a distribution of Goodgrant Foundation$100m donation.However,donation does not directly affect the performance index and we would encounter endogeneity problem or neglect effects of other variables if we solely focus on the relation between performance index and donation amount. Instead,we select several variables that are pivotal in predicting the performance index from many potential candidates,and determine their coefficients/effects on the performance index. We call these variables performance contributing variables.Due to absence of time-series data,it becomes difficult tofigure out how performance con-tributing variables are affected by donation amount for each institution respectively.Instead, wefit the relation between performance contributing variables and donation amount across all institutions and get afitted function from donation amount to values of performance contribut-ing variables.Then we divide the impact of donation amount into2parts:homogenous and heteroge-nous one.The homogenous influence is modeled as the change infitted values of performance contributing variables over increase in donation amount(We call these quotientsfitted ROI of performance contributing variable).The heterogenous one is modeled as a tuning parameter, which adjusts the homogenous influence based on deviation from thefitted function.And their product is the institution-specific increase in true values of performance contributing variables over increase in donation amount(We call these values ROI of performance contributing vari-able).The next step is to calculate the ROI of the performance index by adding the products of ROIs of performance contributing variables and their coefficients on the performance index. This ROI is institution-specific and dependent on increase in donation amount.By adopting a two-step ROI maximization algorithm,we determine the optimal investment strategy.Also,we propose an extended model to handle problems caused by time duration and geographical distribution of donations.Note:we only use data from the provided excel table and that mentioned in the pdffile.Table1:Data SourceVariable DatasetPerformance index Excel tablePerformance contributing variables Excel table and pdffileDonation amount PdffileTheflow chart of the whole model is presented below in Fig1:Figure1:Flow Chart Demonstration of the Model1.3Detailed Definitions&Assumptions 1.3.1Detailed Definitions:1.3.2Assumptions:A1.Stability.We assume data of any institution should be stable without the impact from outside.To be specific,the key factors like the donation amount and the performance index should remain unchanged if the college does not receive new donations.A2.Goodgrant Foundation’s donation(Increase in donation amount)is discrete rather than continuous.This is reasonable because each donation is usually an integer multiple of a minimum amount,like$1m.After referring to the data of other foundations like Lumina Foundation,we recommend donation amount should be one value in the set below:{500000,1000000,1500000, (10000000)A3.The performance index is a linear composition of all given performance indicators.A4.Performance contributing variables linearly affect the performance index.A5.Increase in donation amount affects the performance index through performance con-tributing variables.A6.The impact of increase in donation amount on performance contributing variables con-tains2parts:homogenous one and heterogenous one.The homogenous influence is repre-sented by a smooth function from donation amount to performance contributing variables.And the heterogenous one is represented by deviation from the function.1.4The Advantages of Our ModelOur model exhibits many advantages in application:•The evaluation model is fully data based with few subjective or arbitrary decision rules.•Our model successfully identifies the underlying mechanism instead of merely focusing on the relation between donation amount and the performance index.•Our model takes both homogeneity and heterogeneity into consideration.•Our model makes full use of the cross-section data and does not need time-series data to produce reasonable outcomes.2Addressing the Missing ValuesThe provided datasets suffer from severe data missing,which could undermine the reliabil-ity and interpretability of any results.To cope with this problem,we adopt several different methods for data with varied missing rate.For data with missing rate over50%,any current prevailing method would fall victim to under-or over-randomization.As a result,we omit this kind of data for simplicity’s sake.For variables with missing rate between10%-50%,we use imputation techniques(Little and Rubin,2014)where a missing value was imputed from a randomly selected similar record,and model-based analysis where missing values are substituted with distribution diagrams.For variables with missing rate under10%,we address missingness by simply replace miss-ing value with mean of existing values.3Determining the Performance IndexIn this section,we derive a composite index,called the performance index,to evaluate the educational performance of students at every institution.3.1Performance IndicatorsFirst,we need to determine which variables from various institutional performance data are direct indicators of Goodgrant Foundation’s major concern–to enhance students’educational performance.In practice,other charitable foundations such as Gates Foundation place their focus on core indexes like attendance and graduation rate.Logically,we select performance indicators on the basis of its correlation with these core indexes.With this method,miscellaneous performance data from the excel table boils down to4crucial variables.C150_4_P OOLED_SUP P and C200_L4_P OOLED_SUP P,as completion rates for different types of institutions,are directly correlated with graduation rate.We combine them into one variable.Md_earn_wne_p10and gt_25k_p6,as different measures of graduates’earnings,are proved in empirical studies(Ehren-berg,2004)to be highly dependent on educational performance.And RP Y_3Y R_RT_SUP P, as repayment rate,is also considered valid in the same sense.Let them be Y1,Y2,Y3and Y4.For easy calculation and interpretation of the performance index,we apply uniformization to all4variables,as to make sure they’re on the same scale(from0to100).3.2Performance Index via Principal-Component FactorsAs the model assumes the performance index is a linear composition of all performance indicators,all we need to do is determine the weights of these variables.Here we apply the method of Customer Satisfaction Index model(Rogg et al,2001),where principal-component factors(pcf)are employed to determine weights of all aspects.The pcf procedure uses an orthogonal transformation to convert a set of observations of pos-sibly correlated variables into a set of values of linearly uncorrelated variables called principal-component factors,each of which carries part of the total variance.If the cumulative proportion of the variance exceeds80%,it’s viable to use corresponding pcfs(usually thefirst two pcfs)to determine weights of original variables.In this case,we’ll get4pcfs(named P CF1,P CF2,P CF3and P CF4).First,the procedure provides the linear coefficients of Y m in the expression of P CF1and P CF2.We getP CF1=a11Y1+a12Y2+a13Y3+a14Y4P CF2=a21Y1+a22Y2+a23Y3+a24Y4(a km calculated as corresponding factor loadings over square root of factor k’s eigenvalue) Then,we calculate the rough weights c m for Y m.Let the variance proportions P CF1and P CF2 represent be N1and N2.We get c m=(a1m N1+a2m N2)/(N1+N2)(This formulation is justifiedbecause the variance proportions can be viewed as the significance of pcfs).If we let perfor-mance index=(P CF 1N 1+P CF 2N 2)/(N 1+N 2),c m is indeed the rough weight of Y m in terms of variance)Next,we get the weights by adjusting the sum of rough weights to 1:c m =c m /(c 1+c 2+c 3+c 4)Finally,we get the performance index,which is the weighted sum of the 4performance indicator.Performance index= m (c m Y m )Table 2presents the 10institutions with largest values of the performance index.This rank-ing is highly consistent with widely acknowledged rankings,like QS ranking,which indicates the validity of the performance index.Table 2:The Top 10Institutions in Terms of Performance IndexInstitutionPerformance index Los Angeles County College of Nursing and Allied Health79.60372162Massachusetts Institute of Technology79.06066895University of Pennsylvania79.05044556Babson College78.99269867Georgetown University78.90468597Stanford University78.70586395Duke University78.27719116University of Notre Dame78.15843964Weill Cornell Medical College 78.143341064Identifying Performance Contributing Variables via post-LASSO The next step of our model requires identifying the factors that may exert an influence on the students’educational performance from a variety of variables mentioned in the excel table and the pdf file (108in total,some of which are dummy variables converted from categorical variables).To achieve this purpose,we used a model called LASSO.A linear model is adopted to describe the relationship between the endogenous variable –performance index –and all variables that are potentially influential to it.We assign appropriate coefficient to each variable to minimize the square error between our model prediction and the actual value when fitting the data.min β1J J j =1(y j −x T j β)2where J =2881,x j =(1,x 1j ,x 2j ,...,x pj )THowever,as the amount of the variables included in the model is increasing,the cost func-tion will naturally decrease.So the problem of over fitting the data will arise,which make the model we come up with hard to predict the future performance of the students.Also,since there are hundreds of potential variables as candidates.We need a method to identify the variables that truly matter and have a strong effect on the performance index.Here we take the advantage of a method named post-LASSO (Tibshirani,1996).LASSO,also known as the least absolute shrinkage and selection operator,is a method used for variableselection and shrinkage in medium-or high-dimensional environment.And post-LASSO is to apply ordinary least squares(OLS)to the model selected byfirst-step LASSO procedure.In LASSO procedure,instead of using the cost function that merely focusing on the square error between the prediction and the actual value,a penalty term is also included into the objective function.We wish to minimize:min β1JJj=1(y j−x T jβ)2+λ||β||1whereλ||β||1is the penalty term.The penalty term takes the number of variables into con-sideration by penalizing on the absolute value of the coefficients and forcing the coefficients of many variables shrink to zero if this variable is of less importance.The penalty coefficient lambda determines the degree of penalty for including variables into the model.After min-imizing the cost function plus the penalty term,we couldfigure out the variables of larger essence to include in the model.We utilize the LARS algorithm to implement the LASSO procedure and cross-validation MSE minimization(Usai et al,2009)to determine the optimal penalty coefficient(represented by shrinkage factor in LARS algorithm).And then OLS is employed to complete the post-LASSO method.Figure2:LASSO path-coefficients as a function of shrinkage factor sFigure3:Cross-validated MSEFig2.displays the results of LASSO procedure and Fig3displays the cross-validated MSE for different shrinkage factors.As specified above,the cross-validated MSE reaches minimum with shrinkage factor between0.4-0.8.We choose0.6andfind in Fig2that6variables have nonzero coefficients via the LASSO procedure,thus being selected as the performance con-tributing variables.Table3is a demonstration of these6variables and corresponding post-LASSO results.Table3:Post-LASSO resultsDependent variable:performance_indexPCTPELL−26.453∗∗∗(0.872)PPTUG_EF−14.819∗∗∗(0.781)StudentToFaculty_ratio−0.231∗∗∗(0.025)Tuition&Fees20100.0003∗∗∗(0.00002)Carnegie_HighResearchActivity 5.667∗∗∗(0.775)Constant61.326∗∗∗(0.783)Observations2,880R20.610Adjusted R20.609Note:PCTPELL is percentage of students who receive aPell Grant;PPTUG_EF is share of students who are part-time;Carnegie_HighResearchActivity is Carnegie classifica-tion basic:High Research ActivityThe results presented in Table3are consistent with common sense.For instance,the pos-itive coefficient of High Research Activity Carnegie classification implies that active research activity helps student’s educational performance;and the negative coefficient of Student-to-Faculty ratio suggests that decrease in faculty quantity undermines students’educational per-formance.Along with the large R square value and small p-value for each coefficient,the post-LASSO procedure proves to select a valid set of performance contributing variables and describe well their contribution to the performance index.5Determining Investment Strategy based on ROIWe’ve identified5performance contributing variables via post-LASSO.Among them,tu-ition&fees in2010and Carnegie High-Research-Activity classification are quite insusceptible to donation amount.So we only consider the effects of increase in donation amount on per-centage of students who receive a Pell Grant,share of students who are part-time and student-to-faculty ratio.We denote them with F1,F2and F3,their post-LASSO coefficients withβ1,β2andβ3.In this section,wefirst introduce the procedure used tofit the relation between performance contributing variables and donation amount.Then we provide the model employed to calcu-latefitted ROIs of performance contributing variables(the homogenous influence of increase in donation amount)and the tuning parameter(the heterogenous influence of increase in dona-tion amount).Next,we introduce how to determine stly,we show how the maximiza-tion determines the investment strategy,including selection of institutions and distribution of investments.5.1Fitted Curve between Performance Contributing Variables and Donation AmountSince we have already approximated the linear relation between the performance index with the3performance contributing variables,we want to know how increase in donation changes them.In this paper,we use Generalized Adaptive Model(GAM)to smoothlyfit the relations. Generalized Adaptive Model is a generalized linear model in which the dependent variable depends linearly on unknown smooth functions of independent variables.Thefitted curve of percentage of students who receive a Pell Grant is depicted below in Fig4(see the other two fitted curves in Appendix):Figure4:GAM ApproximationA Pell Grant is money the U.S.federal government provides directly for students who needit to pay for college.Intuitively,if the amount of donation an institution receives from other sources such as private donation increases,the institution is likely to use these donations to alleviate students’financial stress,resulting in percentage of students who receive a Pell Grant. Thus it is reasonable to see afitted curve downward sloping at most part.Also,in commonsense,an increase in donation amount would lead to increase in the performance index.This downward sloping curve is consistent with the negative post-LASSO coefficient of percentage of students who receive a Pell Grant(as two negatives make a positive).5.2ROI(Return on Investment)5.2.1Model of Fitted ROIs of Performance Contributing Variables fROI iFigure5:Demonstration of fROI1Again,we usefitted curve of percentage of students who receive a Pell Grant as an example. We modeled the bluefitted curve to represent the homogeneous relation between percentage of students who receive a Pell Grant and donation amount.Recallfitted ROI of percentage of students who receive a Pell Grant(fROI1)is change in fitted values(∆f)over increase in donation amount(∆X).SofROI1=∆f/∆XAccording to assumption A2,the amount of each Goodgrant Foundation’s donation falls into a pre-specified set,namely,{500000,1000000,1500000,...,10000000}.So we get a set of possible fitted ROI of percentage of students who receive a Pell Grant(fROI1).Clearly,fROI1is de-pendent on both donation amount(X)and increase in donation amount(∆X).Calculation of fitted ROIs of other performance contributing variables is similar.5.2.2Model of the tuning parameter P iAlthough we’ve identified the homogenous influence of increase in donation amount,we shall not neglect the fact that institutions utilize donations differently.A proportion of do-nations might be appropriated by the university’s administration and different institutions allocate the donation differently.For example,university with a more convenient and well-maintained system of identifying students who needfinancial aid might be willing to use a larger portion of donations to directly aid students,resulting in a lower percentage of under-graduate students receiving Pell grant.Also,university facing lower cost of identifying and hiring suitable faculty members might be inclined to use a larger portion of donations in this direction,resulting in a lower student-to-faculty ratio.These above mentioned reasons make institutions deviate from the homogenousfitted func-tion and presents heterogeneous influence of increase in donation amount.Thus,while the homogenous influence only depends on donation amount and increase in donation amount, the heterogeneous influence is institution-specific.To account for this heterogeneous influence,we utilize a tuning parameter P i to adjust the homogenous influence.By multiplying the tuning parameter,fitted ROIs of performance con-tributing variables(fitted value changes)convert into ROI of performance contributing variable (true value changes).ROI i=fROI i·P iWe then argue that P i can be summarized by a function of deviation from thefitted curve (∆h),and the function has the shape shown in Fig6.The value of P i ranges from0to2,because P i can be viewed as an amplification or shrinkage of the homogenous influence.For example,P i=2means that the homogeneous influence is amplified greatly.P i=0means that this homogeneous influence would be entirely wiped out. The shape of the function is as shown in Fig6because of the following reasons.Intuitively,if one institution locates above thefitted line,when deviation is small,the larger it is,the larger P i is.This is because the institution might be more inclined to utilize donations to change that factor.However,when deviation becomes even larger,the institution grows less willing to invest on this factor.This is because marginal utility decreases.The discussion is similar if one institution initially lies under thefitted line.Thus,we assume the function mapping deviation to P i is similar to Fig6.deviation is on the x-axis while P i is on the y-axis.Figure6:Function from Deviation to P iIn order to simplify calculation and without loss of generality,we approximate the function。
Unit1Topic2SectionC课件2023-2024学年仁爱版英语九年级年级上册
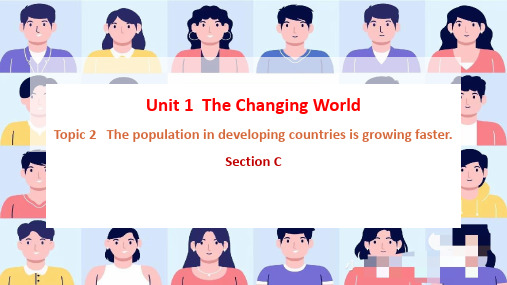
Situation now
Living conditions __a_r_e_im__p_r_o_v_in_g__ra_p_i_d_ly_____ The natural environment _is__b_e_c_o_m_i_n_g_b_e_t_te_r__a_n_d_b_e.tter
serious The population problem is still
What a large population!
Large population caused many social problems.
People had less living space.
Large population caused many social problems.
There was more pollution.
Listen to Par.2 and fill in the blanks. 参考1c的表格
the problems caused by large population
less _l_iv_i_n_g_s_p_a_c_e___
Social problems
fewer __j_o_b___ opportunities less __w_a_t_e_r_ and _e_n_e_r_g_y crowded __c_it_ie_s___
Two thirds of the water__i_s__in the glass.
heavy __t_ra_f_fi_c___
one of the measures to deal with the problems
One-child policy 独生子女政策
Read Par.3 and mark T / F.
RD活动的就业效应:基于中国数据的实证分析
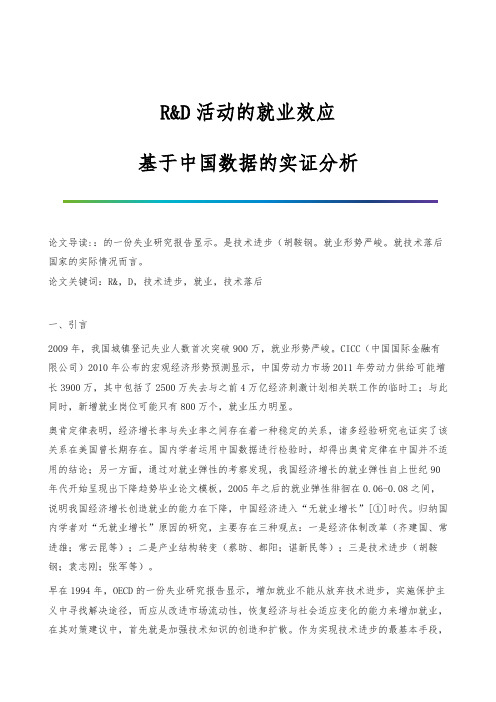
R&D活动的就业效应基于中国数据的实证分析论文导读::的一份失业研究报告显示。
是技术进步(胡鞍钢。
就业形势严峻。
就技术落后国家的实际情况而言。
论文关键词:R&,D,技术进步,就业,技术落后一、引言2009年,我国城镇登记失业人数首次突破900万,就业形势严峻。
CICC(中国国际金融有限公司)2010年公布的宏观经济形势预测显示,中国劳动力市场2011年劳动力供给可能增长3900万,其中包括了2500万失去与之前4万亿经济刺激计划相关联工作的临时工;与此同时,新增就业岗位可能只有800万个,就业压力明显。
奥肯定律表明,经济增长率与失业率之间存在着一种稳定的关系,诸多经验研究也证实了该关系在美国曾长期存在。
国内学者运用中国数据进行检验时,却得出奥肯定律在中国并不适用的结论;另一方面,通过对就业弹性的考察发现,我国经济增长的就业弹性自上世纪90年代开始呈现出下降趋势毕业论文模板,2005年之后的就业弹性徘徊在0.06-0.08之间,说明我国经济增长创造就业的能力在下降,中国经济进入“无就业增长”[①]时代。
归纳国内学者对“无就业增长”原因的研究,主要存在三种观点:一是经济体制改革(齐建国、常进雄;常云昆等);二是产业结构转变(蔡昉、都阳;谌新民等);三是技术进步(胡鞍钢;袁志刚;张军等)。
早在1994年,OECD的一份失业研究报告显示,增加就业不能从放弃技术进步,实施保护主义中寻找解决途径,而应从改进市场流动性,恢复经济与社会适应变化的能力来增加就业,在其对策建议中,首先就是加强技术知识的创造和扩散。
作为实现技术进步的最基本手段,将R&D活动纳入到分析就业问题中具有一定的理论意义和现实意义。
本文旨在明确R&D活动与就业之间是否存在一定的关系?其具体的传导途径是什么?并结合技术落后国家(中国)的实际情况进行理论与实证分析。
本文接下来的安排是:第二部分是R&D、技术进步与就业的相关文献综述;第三部分是所需变量的选取及测算;第四部分是实证分析;最后是本文的相关结论中国期刊全文数据库。
Finding community structure in networks using the eigenvectors of matrices
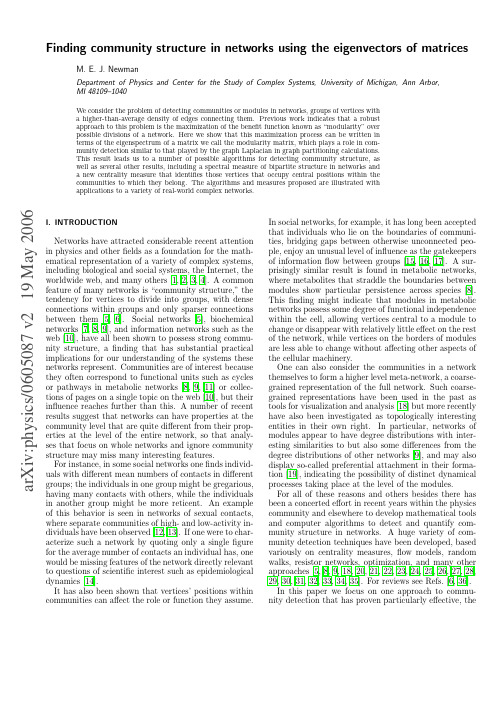
M. E. J. Newman
Department of Physics and Center for the Study of Complex Systems, University of Michigan, Ann Arbor, MI 48109–1040
We consider the problem of detecting communities or modules in networks, groups of vertices with a higher-than-average density of edges connecting them. Previous work indicates that a robust approach to this problem is the maximization of the benefit function known as “modularity” over possible divisions of a network. Here we show that this maximization process can be written in terms of the eigenspectrum of a matrix we call the modularity matrix, which plays a role in community detection similar to that played by the graph Laplacian in graph partitioning calculations. This result leads us to a number of possible algorithms for detecting community structure, as well as several other results, including a spectral measure of bipartite structure in neteasure that identifies those vertices that occupy central positions within the communities to which they belong. The algorithms and measures proposed are illustrated with applications to a variety of real-world complex networks.
- 1、下载文档前请自行甄别文档内容的完整性,平台不提供额外的编辑、内容补充、找答案等附加服务。
- 2、"仅部分预览"的文档,不可在线预览部分如存在完整性等问题,可反馈申请退款(可完整预览的文档不适用该条件!)。
- 3、如文档侵犯您的权益,请联系客服反馈,我们会尽快为您处理(人工客服工作时间:9:00-18:30)。
2016 ICMProblem DMeasuring the Evolution and Influence in Society’s Information NetworksI nformation is spread quickly in today’s tech-connected communications network; sometimes it is due to the inherent value of the information itself, and other times it is due to the information finding its way to influential or central network nodes that accelerate its spread through social media. While content has varied -- in the 1800s, news was more about local events (e.g., weddings, storms, deaths) rather than viral videos of cats or social lives of entertainers -- the prevailing premise is that this cultural characteristic to share information (both serious and trivial) has always been there. However, the flow of information has never been as easy or wide-ranging as it is today, allowing news of various levels of importance to spread quickly across the globe in our tech connected world. By taking a historical perspective of flow of information relative to inherent value of information, the Institute of Communication Media (ICM) seeks to understand the evolution of the methodology, purpose, and functionality of society’s networks. Specifically, your team, as part of ICM’s Information Analytics Division, has been assigned to analyze the relationship between speed/flow of information vs inherent value of information based on consideration of 5 periods: in the 1870s, when newspapers were delivered by trains and stories were passed by telegraph; in the 1920s, when radios became a more common household item; in the 1970s, when televisions were in most homes; in the 1990s, when households began connecting to the early internet; in the 2010s, when we can carry a connection to the world on our phones. Your supervisor reminds you to be sure to report the assumptions you make and the data you use to build your models.Your specific tasks are:(a) Develop one or more model(s) that allow(s) you to explore the flow of information and filteror find what qualifies as news.(b) Validate your model’s reliability by using data from the past and the prediction capability ofyour model to predict the information communication situation for today and compare that with today’s reality.(c) Use your model to predict the communication networks’ relationships and capacities aroundthe year 2050.(d) Use the theories and concepts of information influence on networks to model how publicinterest and opinion can be changed through information networks in tod ay’s connected world.(e) Determine how in formation value, people’s initial opini on and bias, form of the message orits source, and the topology or strength of the information network in a region, country, orworldwide could be used to spread information and influence public opinion.Possible Data Sources:As you develop your model and prepare to test it, you will need to assemble a collection of data. Below are just some examples of the types of data you may find useful in this project. Depending on your exact model, some types of data may be very important and others may be entirely irrelevant. In addition to the sample sources provided below, you might want to consider a few important world events throughout history – if some recent big news events, such as the rumors of country-turned-pop singer Tay lor Swift’s possible engagement had instead happened in 1860, what percentage of the population would know about it and how quickly; likewise, if an important person was assassinated today, how would that news spread? How might that compare to the news of US President Abraham Lincoln’s assassination?Sample Circulation Data and Media Availability:/downloads/Sixty_Years_Daily_Newspaper_Circulation_Trends_050611.pdf /2/hi/technology/8552410.stm.scot/Publications/2006/01/12104731/6/news/427787/are-smart-phones-spreading-faster-than-any-technology-in-human-history//content/default.aspx?NewsAreaId=22/news/mediawire/189819/pew-tv-viewing-habit-grays-as-digital-news-consumption-tops-print-radio//2012/09/27/section-1-watching-reading-and-listening-to-the-news-3//hard-evidence-how-does-false-information-spread-online-25567Historical Perspectives of News and Media:https:///How-did-news-get-around-the-world-before-the-invention-of-newspapers-and-other-media/books/a-primer-on-communication-studies/s15-media-technology-and-communica.html/article/view/885/794Richard Campbell, Christopher R. Martin, and Bettina Fabos, Media & Culture: An Introduction to Mass Communication, 5th ed. (Boston, MA: Bedford St. Martin’s, 2007)Marshall T. Poe, A History of Communications: Media and Society from the Evolution of Speech to the Internet (New York: Cambridge, 2011)Shirley Biagi, Media/Impact: An Introduction to Mass Media (Boston, MA: Wadsworth, 2007)Your ICM submission should consist of a 1 page Summary Sheet and your solution cannot exceed 20 pages for a maximum of 21 pages. Note: The appendix and references do not count toward the 20 page limit.。