NEW-Synative
英汉口译教程

Methods to Improve Memory
• • • • • • Organization Repetition Visualization Chunking Association Note-taking
Organization
• Sequential – chronological; cause/effect; building to climax. • Directional – north/south & east/west; front/back; left/right; bottom/up or top/down, etc. • Transitional (connective) – relational words or phrases used to indicate qualitative change over time • Component (part/whole) – classification by category or concept • Relevance— topic sentence or supporting sentences
中餐常见的翻译方法
• 烹饪方法 cookery 煮 boiling 煲/炖 stewing 煎 frying 炒 stir-frying 爆 quick-frying 炸 deep-frying 熏 smoking 烤 roasting/barbecuing 蒸 steaming 红烧 braising with soy sauce
“旧词”在计算机语言中的“新意”
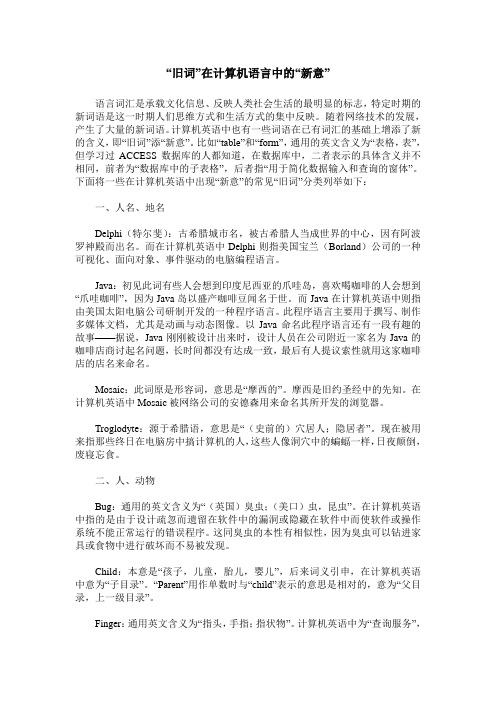
“旧词”在计算机语言中的“新意”语言词汇是承载文化信息、反映人类社会生活的最明显的标志,特定时期的新词语是这一时期人们思维方式和生活方式的集中反映。
随着网络技术的发展,产生了大量的新词语。
计算机英语中也有一些词语在已有词汇的基础上增添了新的含义,即“旧词”添“新意”。
比如“table”和“form”,通用的英文含义为“表格,表”,但学习过ACCESS数据库的人都知道,在数据库中,二者表示的具体含义并不相同,前者为“数据库中的子表格”,后者指“用于简化数据输入和查询的窗体”。
下面将一些在计算机英语中出现“新意”的常见“旧词”分类列举如下:一、人名、地名Delphi(特尔斐):古希腊城市名,被古希腊人当成世界的中心,因有阿波罗神殿而出名。
而在计算机英语中Delphi则指美国宝兰(Borland)公司的一种可视化、面向对象、事件驱动的电脑编程语言。
Java:初见此词有些人会想到印度尼西亚的爪哇岛,喜欢喝咖啡的人会想到“爪哇咖啡”,因为Java岛以盛产咖啡豆闻名于世。
而Java在计算机英语中则指由美国太阳电脑公司研制开发的一种程序语言。
此程序语言主要用于撰写、制作多媒体文档,尤其是动画与动态图像。
以Java命名此程序语言还有一段有趣的故事——据说,Java刚刚被设计出来时,设计人员在公司附近一家名为Java的咖啡店商讨起名问题,长时间都没有达成一致,最后有人提议索性就用这家咖啡店的店名来命名。
Mosaic:此词原是形容词,意思是“摩西的”。
摩西是旧约圣经中的先知。
在计算机英语中Mosaic被网络公司的安德森用来命名其所开发的浏览器。
Troglodyte:源于希腊语,意思是“(史前的)穴居人;隐居者”。
现在被用来指那些终日在电脑房中搞计算机的人,这些人像洞穴中的蝙蝠一样,日夜颠倒,废寝忘食。
二、人、动物Bug:通用的英文含义为“(英国)臭虫;(美口)虫,昆虫”。
在计算机英语中指的是由于设计疏忽而遗留在软件中的漏洞或隐藏在软件中而使软件或操作系统不能正常运行的错误程序。
Java中冷门的synthetic关键字原理解读

Java中冷门的synthetic关键字原理解读看JAVA的反射时,看到有个synthetic ,还有⼀个⽅法isSynthetic() 很好奇,就了解了⼀下:1.定义Any constructs introduced by a Java compiler that do not have a corresponding construct in the source code must be marked as synthetic, except for default constructors, the class initialization method, and the values and valueOf methods of the Enum class.⼤意为:由java编译器⽣成的(除了像默认构造函数这⼀类的)⽅法,或者类2.实例既然知道synthetic⽅法和synthetic类是由编译器⽣成的,那到底编译器会怎么⽣成这些东西,⼜在什么情况下会⽣成这些东西呢?先看⼀段代码:import static ng.System.out;public final class DemonstrateSyntheticMethods{public static void main(final String[] arguments){DemonstrateSyntheticMethods.NestedClass nested =new DemonstrateSyntheticMethods.NestedClass();out.println("String: " + nested.highlyConfidential);}private static final class NestedClass{private String highlyConfidential = "Don't tell anyone about me";private int highlyConfidentialInt = 42;private Calendar highlyConfidentialCalendar = Calendar.getInstance();private boolean highlyConfidentialBoolean = true;}}编译之后,可以看到三个⽂件:其中,最下⾯的这个类⽂件很好解释,就是我们的主class,中间的⽂件,是我们的内部类,上⾯的⽂件,后⾯再讲,我们先看⼀下中间这个内部类2.1 内部类的反编译结果⽤javap 反编译DemonstrateSyntheticMethods$NestedClass.class,得到如下结果:javap DemonstrateSyntheticMethods\$NestedClass.classCompiled from "DemonstrateSyntheticMethods.java"final class DemonstrateSyntheticMethods$NestedClass {DemonstrateSyntheticMethods$NestedClass(DemonstrateSyntheticMethods$1);static ng.String access$100(DemonstrateSyntheticMethods$NestedClass);}先把构造函数放⼀边,我们来看这个标⿊的⽅法access$100 这个是怎么回事呢?我们的源⽂件⾥找不到这个access⽅法啊?2.2 synthetic⽅法这个⽅法就是编译器⽣成的synthetic⽅法,读者不信的话,可以⽤method.isSynthetic() 去验证⼀下。
15-ISO 4992-1:2006 钢铸件 超声波检查 第1部分:一般用途的钢铸件

Bibliography ..................................................................................................................................................... 30
ISO copyright office Case postale 56 • CH-1211 Geneva 20 Tel. + 41 22 749 01 11 Fax + 41 22 749 09 47 E-mail copyright@ Web
Published in Switzerland
5.5 Examination procedure ........................................................................................................................ 6
5.6 Examination report ............................................................................................................................... 9
© ISO 2006
All rights reserved. Unless otherwise specified, no part of this publication may be reproduced or utilized in any form or by any means, electronic or mechanical, including photocopying and microfilm, without permission in writing from either ISO at the address below or ISO's member body in the country of the requester.
IEC vibration test standard
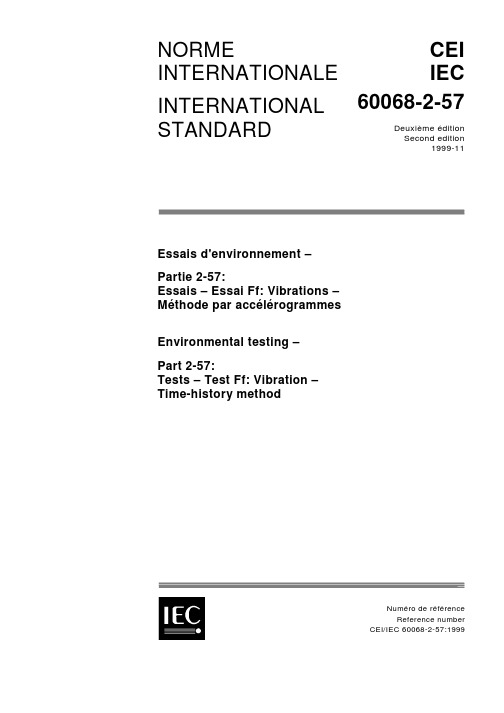
* * Voir adresse «site web» sur la page de titre.
See web site address on title page.
NORME INTERNATIONALE INTERNATIONAL STANDARD
CEI IEC 60068-2-57
Deuxième édition Second edition 1999-11
Numéro de référence Reference number CEI/IEC 60068-2ications
Depuis le 1er janvier 1997, les publications de la CEI sont numérotées à partir de 60000.
•
•
Terminologie, symboles graphiques et littéraux
En ce qui concerne la terminologie générale, le lecteur se reportera à la CEI 60050: Vocabulaire Electrotechnique International (VEI). Pour les symboles graphiques, les symboles littéraux et les signes d'usage général approuvés par la CEI, le lecteur consultera la CEI 60027: Symboles littéraux à utiliser en électrotechnique, la CEI 60417: S ymboles graphiques utilisables sur le matériel. Index, relevé et compilation des feuilles individuelles, et la CEI 60617: Symboles graphiques pour schémas.
ChatGPT技术对话生成的主题一致性的保证方法

ChatGPT技术对话生成的主题一致性的保证方法ChatGPT是一种基于人工智能的技术,它可以生成自然语言的对话。
然而,在使用ChatGPT进行对话生成时,一个重要的问题是如何保证生成的对话主题一致性。
本文将探讨一些方法,帮助确保ChatGPT生成的对话具有一致的主题。
首先,我们可以通过限制ChatGPT的输入话题来保证对话的一致性。
通过引入一个话题模块,我们可以预先将对话限定在特定的主题范围内。
这样做可以防止ChatGPT在生成回复时跳跃到不相关的话题上。
例如,如果对话的主题是关于电影的,我们可以将对话模型的输入设置为电影相关的问题或提示,确保生成的回复始终与电影有关。
其次,我们可以使用上下文信息来维持对话的一致性。
ChatGPT可以通过上下文理解对话的前后文,并生成具有连贯性的回复。
在对话的每一轮中,ChatGPT可以通过对上一轮对话内容的理解来生成回复,从而确保回复与之前的对话主题保持一致。
这可以通过对模型进行预训练和微调来实现,让ChatGPT能够更好地理解上下文并生成连贯的回复。
此外,我们还可以使用评估指标来衡量对话的一致性,并对ChatGPT的回复进行评估和调整。
一种常用的评估指标是BLEU得分,它可以衡量生成的回复与参考回复之间的相似度。
通过设置合适的阈值,我们可以过滤掉那些与对话主题不一致的回复,并优先选择与主题一致的回复作为最终的生成结果。
另外,引入外部知识库或常识推理可以进一步提升ChatGPT的对话一致性。
通过将外部知识集成到ChatGPT中,模型可以更好地理解和生成与主题相关的回复。
例如,ChatGPT可以通过检索相关的知识或根据常识进行推理,以确保生成的回复与对话主题保持一致。
然而,需要注意的是,保证对话的一致性并不意味着完全限制ChatGPT的创造力。
有时候,一些略微偏离主题的回复也可以增加对话的多样性和趣味性。
因此,在确保对话一致性的同时,我们也需要平衡对话的多样性和创造性,以提供更好的对话体验。
RNA Seq 数据分析

2) Template immobilization – DNA separated into single strands and captured onto beads (1 DNA molecule/bead)
3) Clonal Amplification – Solid Phase Amplification 4) Sequencing and Imaging – Cyclic reversible termination (CRT) reaction
Hybridization Based Gene Expression Quantification
Limitations of Sanger Sequencing Low throughput Inconsistent base quality Expensive Not quantitative
RNA Purification and Analysis
RNA Purification: Can use Qiagen Kit or Phenol/Chloroform Extraction, do not use Invitrogen RNA isolation kit RNA Quality Assessment (Agilent 2100 BioAnalyzer)
RNA Seq: A (soon to be outdated) Tutorial
A Brief History of Sequencing and Gene Expression
Tag Based Sequencing Approaches Frederick “Fred” Sanger
Serial Analysis of Gene Expression (SAGE) Cap Analysis of Gene Expression (CAGE) Massively parallel signature sequence (MPSS)
intriguing properties of neural networks 精读

intriguing properties of neural networks 精读Intriguing Properties of Neural NetworksIntroduction:Neural networks are a type of machine learning model inspired by the human brain's functioning. They are composed of interconnected nodes known as neurons that work together to process and analyze complex data. Neural networks have gained immense popularity due to their ability to learn, adapt, and make accurate predictions. In this article, we will delve into some of the intriguing properties of neural networks and explore how they contribute to their success in various fields.1. Non-linearity:One of the key properties of neural networks is their ability to model nonlinear relationships in data. Traditional linear models assume a linear relationship between input variables and the output. However, neural networks introduce non-linear activation functions that allow them to capture complex patterns and correlations. This property enables neural networks to excel in tasks such as image recognition, natural language processing, and voice recognition.2. Parallel Processing:Neural networks possess the remarkable ability to perform parallel processing. Unlike traditional algorithms that follow a sequential execution path, neural networks operate by simultaneously processing multiple inputs in parallel. This parallel architecture allows for faster and efficientcomputations, making neural networks suitable for handling large-scale datasets and real-time applications.3. Distributed Representation:Neural networks utilize distributed representation to process and store information. In traditional computing systems, data is stored in a centralized manner. However, neural networks distribute information across interconnected neurons, enabling efficient storage, retrieval, and association of knowledge. This distributed representation enhances their ability to learn complex patterns and generalize from limited training examples.4. Adaptability:Neural networks exhibit a high degree of adaptability, enabling them to adjust their internal parameters and optimize their performance based on changing input. Through a process called backpropagation, neural networks continuously learn from the errors they make during training. This iterative learning process allows them to adapt to new data and improve their accuracy over time. The adaptability of neural networks makes them robust to noise, varying input patterns, and changing environments.5. Feature Extraction:Neural networks are adept at automatically extracting relevant features from raw data. In traditional machine learning approaches, feature engineering is often a time-consuming and manual process. However, neural networks can learn to identify important features directly from the input data. This property eliminates the need for human intervention and enables neuralnetworks to handle complex, high-dimensional data without prior knowledge or domain expertise.6. Capacity for Representation:Neural networks possess an impressive capacity for representation, making them capable of modeling intricate relationships in data. Deep neural networks, in particular, with multiple layers, can learn hierarchies of features, capturing both low-level and high-level representations. This property allows neural networks to excel in tasks such as image recognition, where they can learn to detect complex shapes, textures, and objects.Conclusion:The intriguing properties of neural networks, such as non-linearity, parallel processing, distributed representation, adaptability, feature extraction, and capacity for representation, contribute to their exceptional performance in various domains. These properties enable neural networks to tackle complex problems, make accurate predictions, and learn from diverse datasets. As researchers continue to explore and enhance the capabilities of neural networks, we can expect these models to revolutionize fields such as healthcare, finance, and autonomous systems.。
- 1、下载文档前请自行甄别文档内容的完整性,平台不提供额外的编辑、内容补充、找答案等附加服务。
- 2、"仅部分预览"的文档,不可在线预览部分如存在完整性等问题,可反馈申请退款(可完整预览的文档不适用该条件!)。
- 3、如文档侵犯您的权益,请联系客服反馈,我们会尽快为您处理(人工客服工作时间:9:00-18:30)。
Synative™ Komponenten für alle Arten von Hochleistungsschmierstoff-Anwendungen werden im Wesentlichen aus natürlichen Ölen und Fetten hergestellt. Diese natürlichen Rohstoffe werden mit oleochemischen Mitteln modifiziert und bieten ein Höchstmaß an Umweltverträglichkeit in Verbindung mit hervorragender technischer Leistungsfähigkeit.
Synlubes Technology encompasses the comprehensive product competence available at Cognis in relation to high-performance synthetic lubricants and their components. Used in a wide range of different industrial machinery and processes, they are able to withstand extreme loads and the toughest of service conditions while offering exceptional environmental compatibility.
max.
Amine Value / Aminzahl
max.
pH-Value / pH-Wert
typ.
Defoamer for oil-based systems / Entschäumer für ölbasierte Systeme
SYNATIVE AC AMH 2
–
5
15
–
Corrosion inhibitors for water-misc. Metalworking Fluids / Korrosionsinhibitoren für wassermischb. Kühlschmierstoffe DEA-free / DEA-frei
Synative™ Product Range Synative™ Produktkatalog
Additives / Additive Dicarbonic Acid-Esters / Dicarbonsäure-Ester Emulsifiers (Vegetable Basis) / Emulgatoren (Pflanzliche Basis) Fatty Alcohols (vegetable) / Fettalkohole (pflanzlich) Glycerol-Esters / Glycerin-Ester Methyl-Esters / Methyl-Ester Mono-Esters / Mono-Ester Polyol-Esters / Polyol-Ester – Unsaturated TMP / Ungesättigte TMP – Saturated TMP / Gesättigte TMP – Unsaturated NPG/PE / Ungesättigte NPG/PE – Saturated NPG/PE / Gesättigte NPG/PE
cst @ 40 °C
cst @ 100 °C typ.
max.
SYNATIVE ES DBA 3.6
1.5
–
0.5
SYNATIVE ES DEHA 7.7
2.3
119
<0.1
SYNATIVE ES DEHS 11.5
3.4
150
0.2
SYNATIVE ES DIDA 13.9
3.6
156
0.1
SYNATIVE ES DITA 27.5
70
0.98
–10
140
0.92*)
–5
240
*) @ 70 °C
Dicarbonic Acid-Esters – Typical Product data / Dicarbonsäure-Ester – typische Produktdaten
Synative™ Product Range / Synative™ Produktkatalog
für die Schlüsselanwendungen: – Metallbearbeitungs-Flüssigkeiten – Walzöle – Umweltverträgliche Schmierstoffe
– Additive – Schmierstoffe im Automobilbereich – Industrie-Schmierstoffe
Evaporation loss / Verdampfungsverlust typ. 99 54 18 22 10
Emulsifiers (Vegetable Basis) / Emulgatoren (Pflanzliche Basis)
Synative™ Product Range / Synative™ Produktkatalog
Die neue Marke Synative™ als Vereinigung von SYNthetic und nATIVE symbolisiert den hohen Anteil von erneuerbaren Rohstoffen in Synlubes Technology Komponenten.
SYNATIVE AC 3160
350 – 550
34
195
10 – 10.5
SYNATIVE AC 3499
200 – 350
1
–
9.5 – 11.0
Density / Dichte typ. g/cm3@ 20 °C
0.82
Pour Point / Pourpoint max. °C
–
As of January 2006 / Stand Januar 2006 Flash Point / Flammpunkt max. °C
As of March 2005 / Stand März 2005
Product name / Produktname
Kinem. Viscosity / Kinem. Viskosität
Viscosity Index / Acid Value / Viskositätsindex Säurezahl
for the key application areas: – Metalworking Fluids – Rolling Oils – Environmentally Acceptable Lubricants
– Additives – Automotive – Industrial Lubricants
Chemical Description / Chemische Bezeichnung
Oleyl-Cetylalcohol * 1 PO / Oleyl-Cetylalkohol * 1 PO Oleyl-Cetylalcohol * 2 PO / Oleyl-Cetylalkohol * 2 PO Oleyl-Cetylalcohol * 3 PO / Oleyl-Cetylalkohol * 3 PO Dicarbonic Acid Polyglycol Esters / Dicarbonsäurepolyglykol-Ester Package for Triglyceride-Emulsions / Emulgator für Triglycerid-Emulsionen Oleyl-Cetylalcohol * 2 EO / Oleyl-Cetylalkohol * 2 EO Oleyl-Cetylalcohol * 2 EO / Oleyl-Cetylalkohol * 2 EO Oleyl-Cetylalcohol * 5 EO / Oleyl-Cetylalkohol * 5 EO Oleyl-Cetylalcohol * 5 EO / Oleyl-Cetylalkohol * 5 EO Castor Oil * 5 EO / Rizinusöl * 5 EO Castor Oil * 11 EO / Rizinusöl * 11 EO Talloil Fatty Acid * 5 EO / Tallölfettsäure * 5 EO
Product name / Produktname
SYNATIVE AC 3651 SYNATIVE AC 3652 SYNATIVE AC 3653 SYNATIVE ES 2458 SYNATIVE AC 2142 SYNATIVE AC 3370 V SYNATIVE AC 3412 V SYNATIVE AC EP 5 L V SYNATIVE AC ET 5 V SYNATIVE AC RT 5 SYNATIVE AC RT 11 SYNATIVE AC TL 55
Synlubes Technology steht für die umfassende Produktkompetenz der synthetischen Hochleistungsschmierstoffe und Schmierstoffkomponenten von Cognis. In den unterschiedlichsten industriellen Produkten und Verfahren halten sie extremsten Belastungen stand und sind gleichzeitig besonders umweltverträglich.