LETTER Predicting Properties of the Rat Somatosensory System by Sparse Coding
高中化学英文词汇

高中化学英文词汇High School Chemistry English VocabularyChemistry is a fascinating subject that explores the composition, properties, and behavior of matter. Whether you are a high school student diving into the world of chemistry for the first time or a seasoned science enthusiast, it is essential to have a good understanding of the English vocabulary commonly used in this field. In this article, we will introduce some key terms and concepts in high school chemistry, along with their English equivalents.1. Elements and AtomsIn chemistry, an element is a pure substance that cannot be broken down into simpler substances by chemical means. Each element is made up of atoms, which are the smallest units of matter that retain the properties of the element. Some common elements include hydrogen, oxygen, carbon, and nitrogen.2. Compounds and MoleculesCompounds are substances composed of two or more elements chemically combined in fixed proportions. The smallest unit of a compound is called a molecule, which consists of two or more atoms bonded together. Water (H2O) and carbon dioxide (CO2) are examples of compounds.3. Chemical ReactionsChemical reactions involve the breaking and forming of chemical bonds between atoms. During a chemical reaction, reactants are transformed intoproducts through the rearrangement of atoms. Some common types of chemical reactions include synthesis, decomposition, combustion, and single displacement.4. Acids and BasesAcids are substances that donate protons (H+) in a chemical reaction, while bases are substances that accept protons. The strength of an acid or base is determined by its pH level, with acids having a pH below 7 and bases having a pH above 7. Examples of acids include hydrochloric acid (HCl) and sulfuric acid (H2SO4), while common bases include sodium hydroxide (NaOH) and ammonia (NH3).5. Chemical EquationsChemical equations are symbolic representations of chemical reactions, with reactants on the left side and products on the right side. Coefficients are used to balance chemical equations and ensure the conservation of mass. For example, the reaction between hydrogen gas (H2) and oxygen gas (O2) to form water can be represented as 2H2 + O2 → 2H2O.6. States of MatterMatter can exist in three states: solid, liquid, and gas. Solids have a fixed shape and volume, liquids have a fixed volume but can change shape, and gases have neither a fixed shape nor volume. Changes in temperature and pressure can cause substances to change states, such as melting, freezing, boiling, and condensation.7. The Periodic TableThe periodic table is a tabular arrangement of the elements based on their atomic number and chemical properties. Elements are organized into groups and periods, with similar properties grouped together. The periodic table provides valuable information about the properties of elements, such as their atomic mass, symbol, and electron configuration.8. StoichiometryStoichiometry is the branch of chemistry that deals with the calculation of quantities of reactants and products in a chemical reaction. It involves balancing chemical equations, determining the limiting reactant, and calculating the theoretical yield of a reaction. Stoichiometry is essential for understanding the composition of compounds and predicting the outcome of reactions.In conclusion, mastering the English vocabulary of high school chemistry is crucial for success in the subject. By familiarizing yourself with key terms and concepts, you can enhance your understanding of chemical principles and communicate effectively with peers and educators. Remember to review and practice these terms regularly to solidify your knowledge and confidence in the exciting world of chemistry.。
Materials Characterization

Materials Characterization Materials characterization is a crucial aspect of materials science and engineering, providing valuable insights into the properties and behavior of various materials. This process involves a range of techniques and methods to analyze the structure, composition, and properties of materials at the micro- and nano-scale. The information obtained from materials characterization is essential for understanding the performance and reliability of materials in different applications, such as in the development of new materials, quality control, and failure analysis. One of the key perspectives in materials characterization is the structural analysis of materials. This involves the study of the arrangement of atoms, crystalline structure, grain boundaries, and defects within a material. Techniques such as X-ray diffraction (XRD), electron microscopy, and atomic force microscopy (AFM) are commonly used to investigate the structural properties of materials. By understanding the structural characteristics of a material, researchers and engineers can determine its mechanical, thermal, and electrical properties, as well as its suitability for specific applications. Another important perspective in materials characterization is the chemical analysis of materials. This involves the identification and quantification of the elements and compounds present in a material. Techniques such as energy-dispersive X-ray spectroscopy (EDS), X-ray photoelectron spectroscopy (XPS), and Fourier-transform infrared spectroscopy (FTIR) are used to analyze the chemical composition of materials. This information is vital for assessing the purity, stability, and reactivity of materials, and for ensuring that they meet the required specifications for a particular application. Furthermore, materials characterization also encompasses the study of the mechanical properties of materials. This involves the measurement of parameters such as strength, hardness, elasticity, and toughness. Techniques such as tensile testing, hardness testing, and impact testing are used to evaluate the mechanical behavior of materials under different conditions. Understanding the mechanical properties of materials is essential for designing components and structures that can withstand various loads and environmental conditions, as well as for predicting their performance and durability. In addition to the structural, chemical, and mechanical perspectives,materials characterization also involves the analysis of the thermal andelectrical properties of materials. This includes the study of parameters such as thermal conductivity, electrical conductivity, thermal expansion, and dielectric properties. Techniques such as differential scanning calorimetry (DSC), thermal conductivity measurements, and impedance spectroscopy are employed to assess the thermal and electrical behavior of materials. This information is crucial for the design and optimization of materials for applications in electronics, energy storage, and thermal management. Moreover, materials characterization plays a significant role in the development of new materials with tailored properties. By gaining a deep understanding of the structure, composition, and properties of materials, researchers can design and engineer materials with specific characteristics to meet the demands of advanced technologies and industries. For example, the development of high-performance alloys for aerospace applications, advanced ceramics for electronic devices, and functional materials for biomedical implants relies heavily on materials characterization to guide the material design process. Finally, materials characterization is essential for quality control and failure analysis in various industries. By employing a range of analytical techniques, manufacturers can ensure the consistency and reliability of materials used in their products, as well as investigate the root causes of materialfailures and defects. This is critical for maintaining the safety and performance of products in sectors such as automotive, aerospace, healthcare, and consumer electronics. In conclusion, materials characterization is a multidimensionalfield that encompasses various perspectives, including structural, chemical, mechanical, thermal, electrical, and design aspects. The insights obtained from materials characterization are invaluable for understanding the properties and behavior of materials, developing new materials with tailored properties, and ensuring the quality and reliability of materials in different applications. This field continues to advance with the development of new techniques and instruments, enabling researchers and engineers to gain a deeper understanding of materials and push the boundaries of material science and engineering.。
岩土力学英文版

岩土力学英文版IntroductionGeotechnical Engineering, also known as Soil Mechanics or Rock Mechanics, is a branch of civil engineering that deals with the behavior of soil and rock materials under various conditions. It is an important field of study as it helps engineers understand the properties and characteristics of these materials, which in turn enables them to design and construct safe and stable structures.Soil MechanicsSoil Mechanics is the study of the behavior of soil materials, including its formation, classification, and properties. Various aspects of soil mechanics are essential in geotechnical engineering, such as soil compaction, permeability, and soil stability.Soil formation is a complex process that involves the weathering and erosion of existing rocks, resulting in the formation of different soil types. The composition and particle size distribution of soil influence its properties, including its bearing capacity, shear strength, and compressibility.Soil classification is an important step in understanding the behavior of various soil types. The Unified Soil Classification System, which categorizes soils based on their particle size and organic content, is widely used in geotechnical engineering. Common soil types include gravel, sand, silt, clay, and organic soils.Understanding soil properties is crucial in determining its suitability for construction projects. Soil compaction refers to the process of densifying soil by applying mechanical force, ensuring stability and reducing settlement. Permeability is the ability of soil to transmit fluids such as water or gas, which is essential in designing drainage systems.Shear strength is another critical property of soil, as it determines its ability to resist sliding or deformation. Soil stability can be assessed through various laboratory tests, such as direct shear tests or triaxial tests, which simulate the conditions that soil experiences in real-world applications.Rock MechanicsRock Mechanics, on the other hand, is the study of thebehavior of rock materials, including its strength, deformation, and stability. It plays a crucial role in the design and construction of underground structures, such as tunnels and mines, as well as in slope stability analysis.Rock strength is an essential characteristic to consider when designing structures in rock formations. Different rock types have varying strength properties, with factors such as mineral composition, rock structure, and geological history influencing their behavior. Lab testing, such as uniaxial compression tests or point load tests, is typically conducted to determine the rock's strength.Rock deformation refers to the response of rock materials to applied stresses, including compression, tension, and shear. Understanding the deformation behavior of rock is crucial in predicting stability and designing support systems for underground excavations. Slope stability analysis is a critical aspect of geotechnical engineering, especially in hilly or mountainous regions. An unstable slope can lead to landslides or slope failures with disastrousconsequences. Various methods, including limit equilibrium analysis and numerical modeling, are used to assess slope stability and design appropriate reinforcement measures.ConclusionGeotechnical engineering plays a vital role in the construction industry as it helps design safe and stable structures by understanding the behavior of soil and rock materials. Soil mechanics focuses on the properties and characteristics of soil, including its formation, classification, and behavior under various conditions. Rock mechanics, on the other hand, studies the properties of rock materials such as strength, deformation, and stability. These fields of study are essential for engineers to ensure the safety and integrity of construction projects.。
The Physics of Fluids
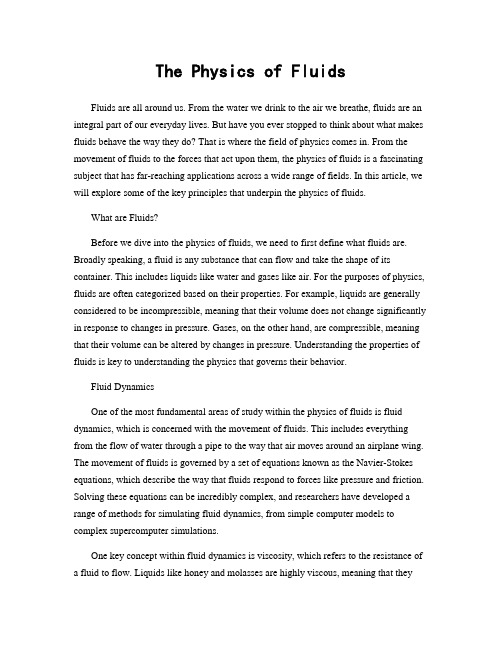
The Physics of FluidsFluids are all around us. From the water we drink to the air we breathe, fluids are an integral part of our everyday lives. But have you ever stopped to think about what makes fluids behave the way they do? That is where the field of physics comes in. From the movement of fluids to the forces that act upon them, the physics of fluids is a fascinating subject that has far-reaching applications across a wide range of fields. In this article, we will explore some of the key principles that underpin the physics of fluids.What are Fluids?Before we dive into the physics of fluids, we need to first define what fluids are. Broadly speaking, a fluid is any substance that can flow and take the shape of its container. This includes liquids like water and gases like air. For the purposes of physics, fluids are often categorized based on their properties. For example, liquids are generally considered to be incompressible, meaning that their volume does not change significantly in response to changes in pressure. Gases, on the other hand, are compressible, meaning that their volume can be altered by changes in pressure. Understanding the properties of fluids is key to understanding the physics that governs their behavior.Fluid DynamicsOne of the most fundamental areas of study within the physics of fluids is fluid dynamics, which is concerned with the movement of fluids. This includes everything from the flow of water through a pipe to the way that air moves around an airplane wing. The movement of fluids is governed by a set of equations known as the Navier-Stokes equations, which describe the way that fluids respond to forces like pressure and friction. Solving these equations can be incredibly complex, and researchers have developed a range of methods for simulating fluid dynamics, from simple computer models to complex supercomputer simulations.One key concept within fluid dynamics is viscosity, which refers to the resistance of a fluid to flow. Liquids like honey and molasses are highly viscous, meaning that theyresist flow and require significant force to move. Gases, on the other hand, are typically less viscous and flow more easily. Understanding viscosity is important for a range of practical applications, from designing more efficient engines to improving the stability of industrial processes.Fluid ForcesFluids are subject to a range of forces that can influence their behavior. Some of the most important forces include pressure, buoyancy, and drag. Pressure arises when a fluid is confined, and is equal in all directions. This can result in interesting phenomena like Bernoulli's principle, which explains the way that air moves around a wing and is crucial for understanding the physics of flight. Buoyancy is the upward force exerted by a fluid on an object immersed within it, and is why objects float in water. Drag is the resistance experienced by an object moving through a fluid, and is a key consideration in the design of everything from cars to spacecraft.Applications of Fluid PhysicsThe principles of fluid physics have a wide range of applications across many different fields. One of the most obvious is in engineering, where fluid mechanics is essential for designing everything from engines to airplanes. Understanding fluid dynamics is also important for weather modeling and predicting natural disasters like hurricanes and floods. In the medical field, fluid mechanics plays a role in everything from blood flow to the dynamics of respiratory fluids. Other applications include everything from oil drilling to the design of microfluidic devices for use in the lab.ConclusionThe physics of fluids is a complex and fascinating subject with many different applications. From the movement of water through a pipe to the forces that act on a spacecraft during reentry, the principles of fluid physics have far-reaching implications for our world. By understanding the properties of fluids and the forces that act upon them, researchers are able to design more efficient engines, predict natural disasters withgreater accuracy, and even improve medical treatments. As our understanding of fluid physics continues to develop, the possibilities for its applications are truly endless.。
科研预测验证 英语
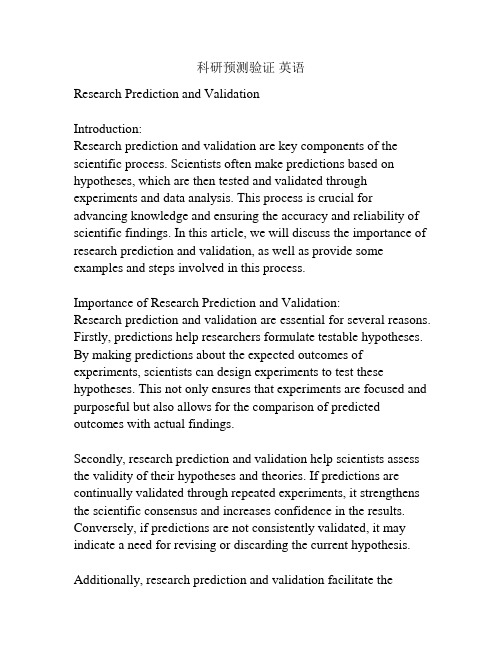
科研预测验证英语Research Prediction and ValidationIntroduction:Research prediction and validation are key components of the scientific process. Scientists often make predictions based on hypotheses, which are then tested and validated through experiments and data analysis. This process is crucial for advancing knowledge and ensuring the accuracy and reliability of scientific findings. In this article, we will discuss the importance of research prediction and validation, as well as provide some examples and steps involved in this process.Importance of Research Prediction and Validation:Research prediction and validation are essential for several reasons. Firstly, predictions help researchers formulate testable hypotheses. By making predictions about the expected outcomes of experiments, scientists can design experiments to test these hypotheses. This not only ensures that experiments are focused and purposeful but also allows for the comparison of predicted outcomes with actual findings.Secondly, research prediction and validation help scientists assess the validity of their hypotheses and theories. If predictions are continually validated through repeated experiments, it strengthens the scientific consensus and increases confidence in the results. Conversely, if predictions are not consistently validated, it may indicate a need for revising or discarding the current hypothesis. Additionally, research prediction and validation facilitate thereplication and reproducibility of scientific findings. Replication is a fundamental principle of science, as it ensures that the reported results are not mere anomalies or outliers. By validating predictions through replication, scientists can establish the robustness and generalizability of their findings, enhancing the reliability of scientific knowledge.Steps in Research Prediction and Validation:1. Formulate a hypothesis: Start by identifying a research question and formulating a hypothesis. The hypothesis should be testable and specific, allowing for the prediction of expected outcomes.2. Design an experiment: Develop a research design and methodology to test the hypothesis. Carefully plan the experimental conditions, variables, and data collection methods. Ensure that the experiment is controlled, and any potential confounding factors are accounted for.3. Make predictions: Based on the hypothesis, make specific predictions about the expected outcomes of the experiment. These predictions should be clear and measurable, enabling the comparison of actual results with the predicted outcomes.4. Conduct the experiment: Carry out the experiment following the designed methodology. Collect and record data systematically, ensuring accuracy and precision. Pay attention to any unexpected observations or variables that may influence the results.5. Analyze the data: Use appropriate statistical and analytical methods to analyze the collected data. Compare the actual resultswith the predicted outcomes to determine whether the predictions have been validated.6. Interpret the findings: Interpret the results in light of the predictions and the original hypothesis. Discuss any discrepancies or unexpected findings and evaluate their potential implications. Consider alternative explanations and factors that may have influenced the results.7. Communicate the results: Publish the findings in peer-reviewed journals or present them at conferences. Clearly state the predictions, experimental methodology, and the validation of the hypothesis. This allows other researchers to scrutinize and replicate the study, contributing to the scientific consensus. Conclusion:Research prediction and validation are integral to the scientific process as they enable scientists to verify the accuracy and reliability of their hypotheses. By making predictions and validating them through experiments, scientists can advance knowledge, establish robust findings, and contribute to the scientific community. Following a systematic approach, researchers can ensure the rigor and validity of their research, promoting the credibility and impact of their work.。
大地构造与石油勘探读后感
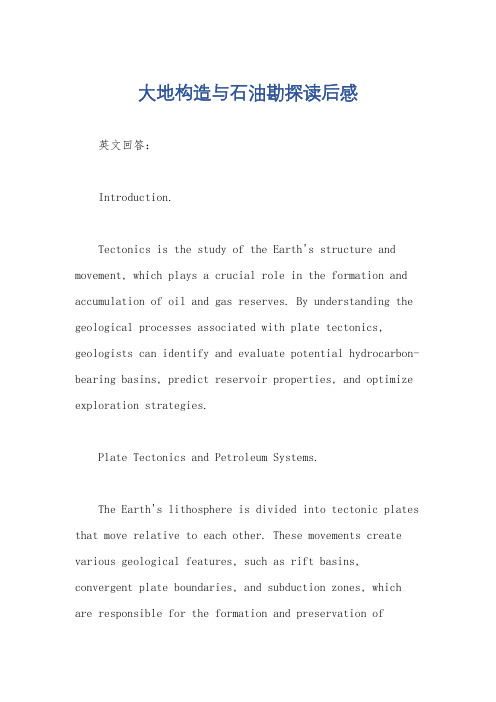
大地构造与石油勘探读后感英文回答:Introduction.Tectonics is the study of the Earth's structure and movement, which plays a crucial role in the formation and accumulation of oil and gas reserves. By understanding the geological processes associated with plate tectonics, geologists can identify and evaluate potential hydrocarbon-bearing basins, predict reservoir properties, and optimize exploration strategies.Plate Tectonics and Petroleum Systems.The Earth's lithosphere is divided into tectonic plates that move relative to each other. These movements create various geological features, such as rift basins, convergent plate boundaries, and subduction zones, which are responsible for the formation and preservation ofpetroleum systems.In rift basins, formed during the initial stages ofplate separation, organic-rich material is deposited and preserved in anoxic environments. As the rift basin matures, extensional forces stretch the crust, creating faults and fractures that serve as conduits for hydrocarbon migration.Convergent plate boundaries, where one plate overrides another, produce thrust faults and folds that can trap hydrocarbons within the deformed strata. Subduction zones, where an oceanic plate sinks beneath a continental plate, provide heat and pressure that can generate hydrocarbons from subducted organic matter or enhance the maturity of existing reservoirs.Predicting Reservoir Properties.Tectonic processes not only influence the formation of petroleum systems but also impact the properties of the reservoirs where hydrocarbons accumulate. The type of tectonic setting and the associated geological structuresaffect factors such as reservoir porosity, permeability, and compartmentalization.In extensional settings, reservoirs are typically characterized by high porosity and fractures, facilitating hydrocarbon flow. In compressional settings, reservoirs may exhibit lower porosity due to compaction and deformation, and the presence of faults and folds can compartmentalize the reservoir, affecting hydrocarbon recovery.Exploration Strategies.A comprehensive understanding of tectonics enables geologists to develop targeted exploration strategies. By integrating knowledge of plate tectonics, structural geology, and geophysics, explorationists can identify areas with high potential for hydrocarbon accumulation and focus their efforts on these prospective regions.Tectonic analysis helps in recognizing areas with favorable source rocks, migration pathways, and potential traps. It also provides insights into the structuralcomplexity of the subsurface, allowing geologists to design drilling programs that maximize the chances of encountering hydrocarbons.Conclusion.Tectonics plays a vital role in the formation, accumulation, and prediction of petroleum resources. By understanding the geological processes associated with plate tectonics, geologists can enhance their ability to identify and evaluate potential hydrocarbon-bearing basins, predict reservoir properties, and develop effective exploration strategies.中文回答:大地构造与石油勘探读后感。
渗透系数的英语

渗透系数的英语The concept of permeability is a fundamental aspect of fluid mechanics and is crucial in various fields, including civil engineering, petroleum engineering, and environmental science. The permeability coefficient, also known as the coefficient of permeability, is a quantitative measure of a material's ability to allow the flow of fluids through its porous structure. This parameter is essential in understanding and predicting the behavior of fluids in porous media, such as soil, rock, and other materials.Permeability is a measure of the ease with which a fluid can flow through a porous medium. It is influenced by the size, shape, and interconnectivity of the pores within the material. The permeability coefficient is a numerical value that represents the ease of fluid flow through a specific porous medium under a given set of conditions. This coefficient is typically denoted by the symbol "k" and is expressed in units of area, such as square meters (m²) or darcies (D).The permeability coefficient is not a constant value and can vary depending on several factors, including the properties of the porousmedium, the properties of the fluid, and the flow conditions. The primary factors that affect the permeability coefficient are the porosity, tortuosity, and pore size distribution of the material.Porosity is the ratio of the volume of voids or pore spaces to the total volume of the material. Materials with higher porosity generally have a higher permeability coefficient, as they offer less resistance to fluid flow. Tortuosity, on the other hand, is a measure of the complexity of the flow paths within the porous medium. Materials with a higher tortuosity have a lower permeability coefficient, as the fluid must navigate through a more convoluted path.The pore size distribution also plays a crucial role in determining the permeability coefficient. Materials with larger and more interconnected pores typically have a higher permeability coefficient, as the fluid can flow more easily through the porous structure. Conversely, materials with smaller and less interconnected pores have a lower permeability coefficient, as the fluid encounters greater resistance to flow.In addition to the properties of the porous medium, the properties of the fluid, such as viscosity and density, can also affect the permeability coefficient. Fluids with lower viscosity, such as water, generally have a higher permeability coefficient compared to fluids with higher viscosity, such as oil or honey.The measurement of the permeability coefficient is an essential aspect of understanding and predicting the behavior of fluids in porous media. There are several methods used to determine the permeability coefficient, including laboratory experiments, field measurements, and numerical simulations.One of the most common laboratory methods for measuring the permeability coefficient is the constant-head or falling-head permeameter test. In this test, a sample of the porous material is placed in a permeameter, and a constant or falling head of fluid is applied across the sample. The rate of fluid flow through the sample is then measured, and the permeability coefficient is calculated using Darcy's law, which relates the fluid flow rate to the pressure drop across the sample.Another method for measuring the permeability coefficient is the use of numerical simulations, such as computational fluid dynamics (CFD) or pore-scale modeling. These techniques involve the use of computer models to simulate the flow of fluids through the porous medium, taking into account the complex geometry and topology of the porous structure. By comparing the simulated flow rates to experimental data, the permeability coefficient can be estimated.The permeability coefficient is a crucial parameter in variousapplications, including:1. Soil mechanics and geotechnical engineering: The permeability coefficient is used to determine the rate of groundwater flow, the stability of soil slopes, and the design of drainage systems.2. Petroleum engineering: The permeability coefficient is essential in understanding the flow of oil and gas through reservoir rocks, which is crucial for the exploration, production, and management of hydrocarbon resources.3. Environmental engineering: The permeability coefficient is used to model the transport of contaminants through soil and groundwater, which is important for the design of waste disposal facilities and the remediation of contaminated sites.4. Civil engineering: The permeability coefficient is used in the design of concrete structures, as it influences the durability and performance of the material under various environmental conditions.5. Materials science: The permeability coefficient is studied in the context of porous materials, such as ceramics, membranes, and filters, to understand their ability to allow the flow of fluids or gases.In conclusion, the permeability coefficient is a fundamentalparameter in the study of fluid flow through porous media. It is influenced by the properties of the porous medium and the fluid, and its measurement and understanding are crucial in various fields of engineering and science. The accurate determination and application of the permeability coefficient are essential for the design, analysis, and optimization of systems and processes that involve the flow of fluids through porous materials.。
卫星轨道外推:第一部分(英文批注)

Orbital Propagation: Part I轨道外推:第一部分By Dr. T.S. KelsoWelcome to the Computers and Satellites column of Satellite Times. We are about to embark on an adventure of discovery—an adventure I have been looking forward to for quite some time. I only hope you will enjoy the experience as much as I and want to follow along with each new episode.欢迎来到《卫星时刻》计算机与卫星专栏,我们即将踏上我期待已久的探索之旅。
我谨希望你能和我一同享受这份经历并追随每一段新的旅程。
Along the way, I hope to enlighten you, the reader—whether novice or expert—on the subtleties involved in the theory and practical application of computers to the process of tracking satellites in earth orbit. Whether you simply want to be able to know where to point your TVRO antenna to pick up your favorite television shows, are curious as to where to look to see the Mir space station on a twilight pass, or want to know when you will be able to DX with the space shuttle on the next SAREX mission, we'll cover it all.一路上,我希望无论新手还是专家的每一位读者在卫星地球轨道计算的详细理论和实际应用方面能有所启迪。
- 1、下载文档前请自行甄别文档内容的完整性,平台不提供额外的编辑、内容补充、找答案等附加服务。
- 2、"仅部分预览"的文档,不可在线预览部分如存在完整性等问题,可反馈申请退款(可完整预览的文档不适用该条件!)。
- 3、如文档侵犯您的权益,请联系客服反馈,我们会尽快为您处理(人工客服工作时间:9:00-18:30)。
Predicting Properties of the Rat Somatosensory Systemby Sparse CodingVerena Vanessa Hafner1, Miriam Fend2, Peter K¨onig3, and Konrad Paul K¨ording41Sony Computer Science Laboratory Paris, France,2 AI Lab, Dept. of Inf. Tech., University of Zurich, Switzerland,3 Institute of Cognitive Science, University of Osnabruck, Germany,4 Institute of Neurology, UCL, London, UKE-mail: hafner@csl.sony.fr, fend@ifi.unizh.chpkoenig@uni-osnabrueck.de, konrad@(Submitted on June 4, 2004)Abstract Many studies address how neurons in the barrel cortex of rats react to stimula-tion of the rat’s whiskers. In this study we analyse how the statistical properties of whiskerdeßections from typical surfaces relate to the properties of neurons in the somatosensorysystem. We built an artiÞcial whisker system to record realistic natural tactile data. AnartiÞcial whisker is moved about a set of surfaces of everyday objects. We analyse howsimulated neurons can represent such stimuli in an optimally sparse fashion. Theserepresentations predict a number of interesting properties of neurons in the somatosensorysystem that have yet to be measured.1.IntroductionWhiskers provide an important source of information to rats and other rodents [26]. Rats can, for example, distinguish surface properties (texture) purely on the basis of cues from their whiskers [7, 5]. They can furthermore use their whiskers to discriminate objects [4] based on their shape. As the rat explores its environment, its whiskers are moved over surfaces of various shape and texture. Neurons in the sensorimotor system thus need to transmit the relevant information to subsequent brain areas. The whisker deßections caused by these stimulations deÞne the input to the rat’s somatosensory system. Although a large number of studies analyse the electrophysiological properties of the barrel cortex [1, 17, 18], the relevant features of its input that should be transmitted by neurons have remained unknown.Recent studies show a growing interest in the texture discrimination abilities of rats (for a review see [16]). Frequencies induced in the vibrissa hair are discussed as the relevant information used for this behaviour [19, 9]. Furthermore, it was shown that for an artiÞcial whisker sensor, different textures could be discriminated analytically using power spectra [6].In an emerging branch of neuroscience, optimal coding of natural scenes, it is studied in what respect neurons optimally encode natural stimuli. As animals grow up and evolve in a world of approximately constant properties, the properties of the brain should be well matched to the properties of the world [3]. Within this Þeld, many studies address the properties of natural stimuli in the visual domain addressing the scaling behaviour of natural images [22] or the properties of higher order statistics using sparse coding [21, 20, 25]. Theses studies showed that many properties of the visual cortex can be understood as sparsely encoding the stimuli it typically encounters. A number of studies also address sparse coding in the auditory domain addressing the auditory nerve [14] or the primary auditory cortex [13]. Again these studies showed that many properties of the auditory system can be understood as sparsely encoding natural sounds.Optimally sparse in these studies means that the neurons often have an activity close to zero and then sometimes have very high activity. Drawing upon this inspiration, we analyse the somatosensory system with similar methods. Sparseness has two distinct albeit related meanings: (1) At any point of time only a small number of neurons should be active (sparseness over the population). (2) Over the course of time each neuronshould be active only rarely (sparseness over time). While the early explorations of sparseness often used deÞnition 1, most modern studies use deÞnition 2 as the implementation is typically a lot faster and in many cases the results are identical. There are a large number of discussed reasons why sparseness should be useful. Just to name two of them: (1) Sparseness ensures that information is transmitted using a minimal number of spikes emitted by the neurons and therefore results in a minimal energy consumption of the brain. (2) Sparse representations also maximise the independence between neural outputs and thus make recognition tasks easier for subsequent stages of cortical processing.In this paper, we examine the statistics of natural stimuli to the somatosensory system. We thus examine if not only visual and auditory but also somatosensory stimuli can be understood as sparsely encoding typical stimuli. In analogy to the databases of natural images used for visual studies, we Þrst need a database of natural whisker deßections. We thus built an artiÞcial whisker system with a real rat whisker attached to a capacitor microphone. This set-up was described in previous papers [15, 8]. This extends previous robotics studies that used simple whisking devices measuring distances or contact only [10, 23, 11], but do not capture the rich information picked up by natural whiskers in a biologically plausible way. We collect whisker data by actively moving the whisker over a set of complex stimuli. The motion pattern of the whisker in this conÞguration closely matches the motion patterns of the whiskers in natural conditions (rough visual observation) and has similar movement frequency and the same shape.We analyse if the neurons in the vibrissal system can be understood in terms of leading to sparse activity in response to these natural inputs. We represent the data coming from our artiÞcial whisker system in the spectro-temporal domain to allow for a large class of spectrotemporal responses. Simulated neurons optimally coding for these data are analysed and generate predictions about neurons in the somatosensory system.2. Hardware Design and MethodsThis section describes the artiÞcial whisker system we built and the responses we recorded in response to moving the whisker over natural surfaces. The desired artiÞcial whisker should be functionally comparable to a natural rat whisker and therefore be sensitive to small amplitude deßections and fast oscillations. We investigated different designs, including piezo-electric crystals and small capacitor microphones. The inßuence of different whisker materials (metal wire, polyvinyl, human hair, rat whiskers) has also been compared [15]. Rat whiskers respond to a range of spatial frequencies and showed little oscillations. The most promising results were gained with a combination of the rat whisker with a capacitor microphone technique, which is described in the following subsection.2.1. The ArtiÞcial Whisker SystemFigure 1. Basic schematic of the artiÞcial whisker with an electret microphone picking up the oscillations and converting them into electrically measurable signals. The whisker is glued onto the membrane of the microphone. The deßection of the membrane is measured by the change of capacitance. The related change of voltage is fed into a preampliÞer circuit.We attached a rat whisker to the diaphragm of a capacitor microphone using cyanoacrylic superglue. Vibrations and displacement of the hair results in deformations of the microphone membrane. The resulting change in voltage is pre-ampliÞed and digitally recorded. This technique allows us to measure fast oscillations ofthe whisker even if the amplitude is very low. The microphone with the rat whisker is attached to a servo motor to produce active whisking in a controlled way as described in the next section. A schematic drawing of the device is shown in Þgure 1, a picture of the artiÞcial whisker system can be seen in Þgure 2.Figure 2. Image of the artiÞcial whisker system used for recording the data.2.2. The Deßections of the ArtiÞcial WhiskerWe recorded deßections from a single whisker being automatically swept over different objects (sandpaper, leather, wool, etc.) with a servo motor turning back and forth at frequencies of either 1 Hz or 4 Hz. While this is a slow movement it is of the same order of magnitude as natural whisking of rats which is at about 8 Hz. Capacitance readings are sampled at 4000 Hz. In contrast to a previous study [8], the stimulation of the whisker was modelled on the active whisking behaviour of rats and mice. Previously, data was acquired either by manually sweeping the sensor across different surfaces or by stimulating the whisker by a rotating drum covered with sandpaper of varying roughness. In the Þrst case, small variations in distance and speed could not be controlled and the whisker was not tilted as in natural whisking. The latter stimulation does not correspond to the biological reality and results in continuous, uniform stimulation. As some electrophysiological Þndings about differing responses in the whisker processing pathway suggest, this distinction might be highly relevant [24]. A typical trace of capacitance of the artiÞcial whisker system can be seen in Þgure 3. It has eight signal peaks per second because of the forth and back movement.Figure 3. Capacitance trace measured by the artiÞcial whisker system while whisking back and forth over an object.3. Results3.1. Representation in Spectrogram SpaceTime varying data are conveniently analysed in spectrogram space, the space spanned by frequency and time. In this space both changes over time and over frequency are easily understood. This representation is particularly useful for the whisker system since rats are able to discriminate surfaces of different spatial frequencies [5]. It has also been shown analytically that the whisker oscillation frequencies elicited by different textures can be used to discriminate between different surfaces [6]. We thus present the input signals as spectrograms. The resolution on the tonotopic axis is 64 points, covering a frequency range from 1 to 512 Hz. In Þgure 4, three typical samples of such transformed whisker data can be seen. These spectrograms show that whisker deßections lead to a largely conserved frequency-time response.Figure 4. Sample spectrogram of whisker data (left: 1 Hz data, right: 4 Hz data). The frequency axis ranges from 1 Hz to 512 Hz while time runs from 0 to 1000 ms in steps of 10 ms. The colour codes for the intensity, red for high values and blue for low ones.3.2. Principal Component AnalysisNeurons usually represent the properties of stimuli over a localised window of time. To analyse the properties of these stimuli we cut the spectrogram data in windows of 250 ms each, overlapping by 100 ms. The temporal resolution of these windows is 25 points. We subsequently assemble a set of 24360 samples of data spectrograms from a recording time of about 4 minutes.Figure 5. First 10 principal components of the spectrogram data. The PCA is applied to the whisker data in spectrogram space, using windows of 250 ms.For the learning studies, the spectrograms are Þrst compressed by a principal component analysis (PCA)using the Þrst nP CA = 100 principal components (out of 25 × 64 = 1600). These components capture more than96% of the variance. In Figure 5, the Þrst 10 principal components of the spectrogram data are shown, sorted bythe size of their corresponding Eigenvalues. The purpose of the PCA is merely the compression of the data. Itdoes not signi Þcantly in ßuence the results of the sparse coding described in the following subsection.3.3. Sparse Coding and ICAA set of 32 simulated neurons is trained to optimally code for the recorded dataset. The activity of theneurons is calculated asA i (t)= I(t)W i (t),where A i is the activity of the neuron, W i is the weight vector of the neuron i. I(t) is the input vector of lengthn PCA = 100 shared by all neurons. This input vector itself again contains a representation of time as it encodes thewhole spectrotemporal window. The weight vector of each neuron is optimised by scaled gradient descent tominimise the following loss function:decorr std cauchy total < < < < ,with: • Cauchy: ¦!<it i couchy t A n 2)(1ln(1, with < · >t being the average over time t• Standard deviation: ¦ <i Ai std n 2)1(1V • Decorrelation: )1)(2(10,2 <¦n n C ji ijdecorr ,with C = cov(A) being the n × n covariance matrix of A\cauchy is a function that favours sparse representations. The two other loss functions ensure the standard criterionused in Independent Component Analysis (ICA) and sparse coding studies that the output variances should beunitary and the output covariances should be vanishing. It can be shown (see [12]) that the decorrelation term isequivalent to minimising the reconstruction error for the original dataset, given a linear system and anovercomplete set of neurons.3.4. Spectrotemporal Receptive FieldsSimulated neurons are optimised to sparsely encode naturally occurring whisker de ßections. Figure 6 showsthe general properties of the resulting spectrotemporal receptive Þelds. Out of 32 receptive Þelds, 18 are similarto plot A, 6 are similar to plot B, and there are some receptive Þelds looking like C, D, and E. Since \cauchy issymmetric, the receptive Þelds can have positive or negative localisation features. Most of the analysed neuronsare localised in time and frequency.Figure 6. Five typical samples of colour-coded spectrotemporal receptive Þelds out of 32 neurons. y-axis:frequency (1 Hz to 512 Hz), x-axis: time (0 to 250 ms).To further quantify this property, we introduce two measures of localisedness (Þgure 7). For the analysis, we calculate the average energy over time, and the frequency for each receptive Þeld, respectively. We also measure the width of the maximum peak at half the peak value for time localisation, and the octaves log(fl /fh) for frequency localisation. More than 96% of the receptive Þelds have a localisation measure in time of less than 80 ms. This seems to be necessary for texture discrimination. Arabzadeh et al. [2] report that rats can distinguish textures already after an offset of 5í15 msec after stimulus onset. The receptive Þelds have a tuning width in frequency of less than one octave in 81% of the neurons. The cells coding for the data recorded for this research show signiÞcantly higher localisedness than cells coding for the sandpaper data (see [8]).This is in analogy to sparse simulated neurons in the visual system that obtain localised receptive Þelds in space and orientation [21]. In addition to this, they are often tuned to changes or even modulations of the energy of the input over time, such as C and D in Þgure 6. This property might be useful for tactile texture recognition. We predict that in a similar setup for electrophysiological measurements, the somatosensory neurons should be tuned to both energy and frequency.Figure 7. Histograms showing the localisedness of the spatiotemporal receptive Þelds for frequency in octaves (left Þgure) and for time in ms (right Þgure). The number of examined receptive Þelds is 32.4. DiscussionWe predicted properties of cells as they might be found in the somatosensory system of a rat by simulating neurons that receive input from an artiÞcial whisker system and optimising their properties so that they exhibit optimally sparse response patterns.There are two major assumptions that have to be considered: One is the choice of the preprocessing of the data. We decided to use spectrograms since data received from whiskers have very similar properties to auditory data with regards to their dimensionality and structure. It thus is likely that similar analysis methods should be used. It is up to date not known, what preprocessing is performed on the information travelling from the whisker follicle in rats to the barrel cortex. The other assumption is that the natural input is not assigned a class by any means of supervised learning, the clustering happens completely unsupervised on input data varying in material, speed of movement, frequencies, etc.Our study shows that a pressure for sparse coding together with the chosen pre-processing would results in neurons that are typically localised both in time and in frequency. Modulations in time has been shown for neurons in the barrel cortex: A recent study by Arabzadeh et al. [2] has investigated neurons in the barrel cortex of rats and found no speciÞty for speciÞc frequencies, but an encoding of the product of frequency and amplitude of the whisker movement. This seems to be in contradiction to our results, however, there are major differences between the two studies. The experiments described in [2] have been performed on anaesthetised rats without active whisking. Our experiments use active whisking frequencies of 1 or 4 Hz. Their stimulus to the whisker is a very controlled signal consisting of a single frequency presented as a sine wave each, we are presenting the whisker system with natural stimuli consisting of a whole range of frequencies. It is therefore impossible to directly compare the results, but further experiments are needed to elucidate the way by which the brain combines signals at different frequencies.5. Future WorkThe research described in this paper results in properties of simulated cells coding for natural whisker stimuli. In a next step, we will perform behavioural experiments on an artiÞcial mouse robot. One of the advantages to use sparse coding in a robotic setup is the task independence of the sensory modality. Sparse models for the visual domain have already been applied successfully to biologically inspired sensorimotor tasks [27]. The receptive Þelds of the simulated neurons from our studies will be used to learn to discriminate different objects and textures. This will be based on the activation of a small number of neurons which are optimally tuned to the nature of the stimuli instead of using the original raw signal.AcknowledgementsThis research has been supported by the IST-2000-28127 European project (AMOUSE). Thanks go to Michele Bezzi for helpful comments on the manuscript.References[1] E. Ahissar and A. Arieli. Figuring space by time. Neuron, 32:185–201, October 2001.[2] E. Arabzadeh, R. Petersen, and M. E. Diamond. Enconding of whisker vibration by rat barrel cortex neurons:Implications for texture discrimination. Journal of Neuroscience, 23(27):9146–9154, 2003.[3] H. B. Barlow. Single units and sensation: a neuron doctrine for perceptual psychology? Perception,1:371–394, 1972.[4] M. Brecht, B. Preilowski, and M. M. Merzenich. Functional architecture of the mystacial vibrissae.Behavioral Brain Ressearch, 84(1-2):81–97, 1997.[5] G. E. Carvell and J. Simons. Biometric analyses of vibrissal tactile discrimination in the rat. Journal ofNeuroscience, 10(8):2638–2648, 1990.[6] M. Fend, S. Bovet, H. Yokoi, and R. Pfeifer. An active artiÞcial whisker array for texture discrimination. InIEEE/RSJ International Conference on Intelligent Robots and Systems (IROS), 2003.[7] E. Guic-Robles, C. Valdivesco, and G. Guajardo. Rats can learn a roughness discrimination using only theirvibrissal system. Behavioral Brain Research, 31:285–289, 1989.[8] V. V. Hafner, M. Fend, M. Lungarella, R. Pfeifer, P. K¨onig, and K. P. K¨ording. Optimal coding fornaturally occurring whisker deßections. Proceedings of the the Joint International Conference on ArtiÞcial Neural Networks and Neural Information Processing (ICANN/ICONIP), pages 805–812, 2003.[9] M. J. Hartmann, N. J. Johnson, R. B. Towal, and C. Assad. Mechanical characteristics of rat vibrissae:resonant frequencies and damping in isolated whiskers and in the awake behaving animal. Journal of Neuroscience, 23(16):6510–6519, 2003.[10] D. Jung and A. Zelinsky. Whisker-Based Mobile Robot Navigation. Proceedings of the IEEE/RSJInternational Conference on Intelligent Robots and Systems (IROS), 2:497–504, 1996.[11] M. Kaneko, K. Kanayama, and T. Tsuji. Active antenna for contact sensing. IEEE Transactions on Roboticsand Automation, 14(2):278–291, 1998.[12] K. P. K¨ording, C. Kayser, and P. K¨onig. On the choice of a sparse prior. Reviews in the Neurosciences,14:53–62, 2003.[13] K. P. K¨ording, P. K¨onig, and D. J. Klein. Learning of sparse auditory receptive Þelds. In Proceedings ofthe International Joint Conference on Neural Networks (IJCNN), 2002.[14] M. S. Lewicki. EfÞcient coding of natural sounds. Nature Neuroscience, 5(4):356–63, 2002. 1097-6256Journal Article.[15] M. Lungarella, V. V. Hafner, R. Pfeifer, and Hiroshi Yokoi. An ArtiÞcial Whisker Sensor for Robotics. InProceedings of the IEEE/RSJ International Conference on Intelligent Robots and Systems (IROS), pages 2931–2936, 2002.[16] S. B. Mehta and D. Kleinfeld. Frisking the whiskers: Patterned sensory input in the rat vibrissa system.Neuron, 41:181–184, 2004.[17] K. D. Miller, D. J. Pinto, and D. J. Simons. Processing in layer 4 of the neocortical circuit: new insightsfrom visual and somatosensory cortex. Current opinion in neurobiology, 11:488–497, 2001.[18] C. I. Moore, S. B. Nelson, and M. Sur. Dynamics of neuronal processing in rat somatosensory cortex.Trends in Neurosciences, 22(11):513–520, 1999.[19] M. A. Neimark, M. L. Andermann, J. J. HopÞeld, and C. I. Moore. Vibrissa resonance as a transductionmechanism for tactile encoding. Journal of Neuroscience, 23(16):6499– 6509, 2003.[20] B. A. Olshausen. Sparse codes and spikes. In R.P.N. Rao and B.A. Olshausen and M.S. Lewicki, editor,Probabilistic Models of the Brain: Perception and Neural Function, pages 257–272. MIT Press, 2002. [21] B. A. Olshausen and D. J. Field. Emergence of simple-cell receptive Þeld properties by learning a sparsecode for natural images. Nature, 381(6583):607–609, 1996.[22] D. L. Ruderman and W. Bialek. Statistics of natural images: Scaling in the woods. Physical Review Letters,73(6):814–817, 1994.[23] R. A. Russell. Using tactile whiskers to measure surface contours. In Proceedings IEEE InternationalConference on Robotics and Automation, pages 1295–1300, 1992.[24] M. Szwed, K. Bagdasarian, and E. Ahissar. Encoding of vibrissal active touch. Neuron, 40(3):621–631,2003.[25] J. H. van Hateren and D. L. Ruderman. Independent component analysis of natural image sequences yieldsspatio-temporal Þlters similar to simple cells in primary visual cortex. Procedings of the Royal Society London B, 265:2315–2320, 1998.[26] S. B. Vincent. The function of the vibrissae in the behavior of the white rat. Behavior Monographs,1(5):1–81, 1912.[27] L. Yang and M. Jabri. Sparse visual models for biologically inspired sensorimotor control. In ProceedingsThird International Workshop on Epigenetic Robotics, pages 131–138, 2003。