demo2--shape of molecule5
“Discovery Studio 2.5中基于药效团的药物设计方法和应用 ”

36 Compounds
1150compounds
+ Training set (16) Ligandfit
化合物库筛选
可以构建自己的化合物库
database
安装其他化合物库,CNPD,ACD等 DS中自带的化合物库
构建具有活性预测能力的药效团
• 寻找与化合物活性相关的药效团特性 • 构建的药效团模型具有活性预测功能
实例分析2(先导化合物优化)
Training set:
Test set
Pharmacophore modes
IC50=1.43
IC50=0.004
实例分析3
qualitative
Search 3D database
Dock(Ligandfit)
基于特性结构产生药效团
• 对于训练集要求:
- 输入的分子结构具有多样性
catalyst
Conformer Generation • FAST • BEST
Discovery studio
Conformer Generation • FAST • BEST • CAESAR • Systematic Search • Random Search • Boltzmann Jump
Common Feature Pharmacophore Generation HipHop(quanlitative)
- 化合物数目在2-32个,6个左右比较理想 - 只选用具有活性的分子
- 需要包含Principal和MaxOmitFeat性质
• 方法总结 - 找到一组化合物的公共药效特性
- 不需要SAR的信息
单击此处编辑母版标题样式
DS2.5中基于药效团的药物 单击此处编辑母版文本样式 设计方法和应用 第二级
沸石分子筛的性能特点

Fig. Stereoscan of zeolite X crystal
Fig. Stereoscan of zeolite X crystal about 50 m in size showing spinel-type contact twin and spheroids of zeolite P
•Байду номын сангаас溶液中旳反应:
25
① 骨架Si、Al可用Ga、P等取代→杂原子取代分子筛② 可调变表面酸性及其他活性中心旳强度和浓度,或者调变分子筛表面旳吸附性质,从亲水性到疏水性。 如:阳离子互换→酸性分子筛、碱性分子筛 a、取得酸性:Na型 → H型 例如:NaY → HY 互换剂:NH4NO3、也可直接用酸溶液进行互换。 b、取得较强碱性: Na型 → K、Rb、Cs型 互换剂:碱金属旳硝酸盐等可分解型盐类。 碱性强弱:NaY< KY< RbY< CsY、NaX< KX< RbX< CsX
• 水热转化
Table. Steam stability of zeolite XaCation Form % Exchange Structureb Adsorptionc K+ 77 - 60 % - 89 % Na+ 100 - 80 % - 84 % Ca2+ 84 - 60 % - 71 % Ce3+ 77 no change - 21 % a Loose powder (300 C, 8 hr in 100% steam) b Determined from loss in intensity of selected X-ray powder reflections c As determined from argon adsorption at -183 C and 700 torr
J. Comput. Chem.
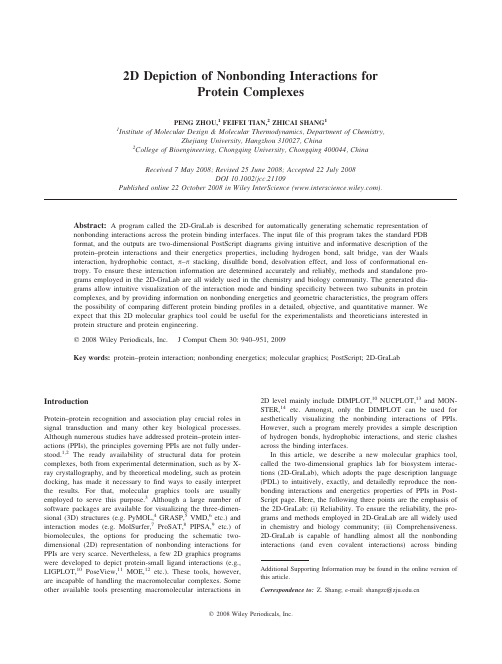
2D Depiction of Nonbonding Interactions forProtein ComplexesPENG ZHOU,1FEIFEI TIAN,2ZHICAI SHANG11Institute of Molecular Design&Molecular Thermodynamics,Department of Chemistry,Zhejiang University,Hangzhou310027,China2College of Bioengineering,Chongqing University,Chongqing400044,ChinaReceived7May2008;Revised25June2008;Accepted22July2008DOI10.1002/jcc.21109Published online22October2008in Wiley InterScience().Abstract:A program called the2D-GraLab is described for automatically generating schematic representation of nonbonding interactions across the protein binding interfaces.The inputfile of this program takes the standard PDB format,and the outputs are two-dimensional PostScript diagrams giving intuitive and informative description of the protein–protein interactions and their energetics properties,including hydrogen bond,salt bridge,van der Waals interaction,hydrophobic contact,p–p stacking,disulfide bond,desolvation effect,and loss of conformational en-tropy.To ensure these interaction information are determined accurately and reliably,methods and standalone pro-grams employed in the2D-GraLab are all widely used in the chemistry and biology community.The generated dia-grams allow intuitive visualization of the interaction mode and binding specificity between two subunits in protein complexes,and by providing information on nonbonding energetics and geometric characteristics,the program offers the possibility of comparing different protein binding profiles in a detailed,objective,and quantitative manner.We expect that this2D molecular graphics tool could be useful for the experimentalists and theoreticians interested in protein structure and protein engineering.q2008Wiley Periodicals,Inc.J Comput Chem30:940–951,2009Key words:protein–protein interaction;nonbonding energetics;molecular graphics;PostScript;2D-GraLabIntroductionProtein–protein recognition and association play crucial roles in signal transduction and many other key biological processes. Although numerous studies have addressed protein–protein inter-actions(PPIs),the principles governing PPIs are not fully under-stood.1,2The ready availability of structural data for protein complexes,both from experimental determination,such as by X-ray crystallography,and by theoretical modeling,such as protein docking,has made it necessary tofind ways to easily interpret the results.For that,molecular graphics tools are usually employed to serve this purpose.3Although a large number of software packages are available for visualizing the three-dimen-sional(3D)structures(e.g.PyMOL,4GRASP,5VMD,6etc.)and interaction modes(e.g.MolSurfer,7ProSAT,8PIPSA,9etc.)of biomolecules,the options for producing the schematic two-dimensional(2D)representation of nonbonding interactions for PPIs are very scarce.Nevertheless,a few2D graphics programs were developed to depict protein-small ligand interactions(e.g., LIGPLOT,10PoseView,11MOE,12etc.).These tools,however, are incapable of handling the macromolecular complexes.Some other available tools presenting macromolecular interactions in 2D level mainly include DIMPLOT,10NUCPLOT,13and MON-STER,14etc.Amongst,only the DIMPLOT can be used for aesthetically visualizing the nonbinding interactions of PPIs. However,such a program merely provides a simple description of hydrogen bonds,hydrophobic interactions,and steric clashes across the binding interfaces.In this article,we describe a new molecular graphics tool, called the two-dimensional graphics lab for biosystem interac-tions(2D-GraLab),which adopts the page description language (PDL)to intuitively,exactly,and detailedly reproduce the non-bonding interactions and energetics properties of PPIs in Post-Script page.Here,the following three points are the emphasis of the2D-GraLab:(i)Reliability.To ensure the reliability,the pro-grams and methods employed in2D-GraLab are all widely used in chemistry and biology community;(ii)Comprehensiveness. 2D-GraLab is capable of handling almost all the nonbonding interactions(and even covalent interactions)across binding Additional Supporting Information may be found in the online version of this article.Correspondence to:Z.Shang;e-mail:shangzc@interface of protein complexes,such as hydrogen bond,salt bridge,van der Waals(vdW)interaction,hydrophobic contact, p–p stacking,disulfide bond,desolvation effect,and loss of con-formational entropy.The outputted diagrams are diversiform, including individual schematic diagram and summarized sche-matic diagram;(iii)Artistry.We elaborately scheme the layout, color match,and page style for different diagrams,with the goal of producing aesthetically pleasing2D images of PPIs.In addi-tion,2D-GraLab provides a graphical user interface(GUI), which allows users to interact with this program and displays the spatial structure and interfacial feature of protein complexes (see .Fig.S1).Identifying Protein Binding InterfacesAn essential step in understanding the molecular basis of PPIs is the accurate identification of interprotein contacts,and based upon that,subsequent works are performed for analysis and lay-out of nonbonding mon methods identifyingprotein–protein binding interfaces include a Voronoi polyhedra-based approach,changes in solvent accessible surface area(D SASA),and various radial cutoffs(e.g.,closest atom,C b,andcentroid,etc.).152D-GraLab allows for the identification of pro-tein–protein binding interfaces at residue and atom levels.Identifying Binding Interfaces at Residue LevelAll the identifying interface methods at residue level belong toradial cutoff approach.In the radial cutoff approach,referencepoint is defined in advance for each residue,and the residues areconsidered in contact if their reference points fell within thedefined cutoff ually,the C a,C b,or centroid are usedas reference point.16–18In2D-GraLab,cutoff distance is moreflexible:cutoff distance5r A1r B1d,where r A and r B are residue radii and d is set by users(as the default d54A˚,which was suggested by Cootes et al.19).Identifying Binding Interfaces at Atom LevelAt atom level,binding interfaces are identified using closestatom-based radial cutoff approach20and D SASA-basedapproach.21For the closest atom-based radial cutoff approach,ifthe distance between any two atoms of two residues from differ-ent chains is less than a cutoff value,the residues are consideredin contact;In the D SASA-based approach,the SASA is calcu-lated twice to identify residues involved in a binding interface,once for the monomers and once for the complex,if there is achange in the SASA(D SASA)of a residue when going from themonomers to the dimer form,then it is considered involved inthe binding interface.In2D-GraLab,three manners are provided for visualizing thebinding interfaces,including spatial structure exhibition,residuedistance plot,and residue-pair contact map(see .Figs.S2–S4).Analysis and2D Layout of NonbondingInteractionsThe inputfile of2D-GraLab is standard PDB format,and the outputs are two-dimensional PostScriptfile giving intuitive and informative representation of the PPIs and their strengths, including hydrogen bond,salt bridge,vdW interaction,desolva-tion effect,ion-pair,side-chain conformational entropy(SCE), etc.The outputs are in two forms as individual schematic dia-gram and summarized schematic diagram.The individual sche-matic diagram is a detailed depiction of each nonbonding profile,whereas the summarized schematic diagram covers all nonbonding interactions and disulfide bonds across the binding interface.To produce the aesthetically high quality layouts,which pos-sess reliable and accurate parameters,several widely used pro-grams listed in Table1are employed in2D-GraLab to perform the core calculations and analysis of different nonbonding inter-actions.2D-GraLab carries out prechecking procedure for pro-tein structures and warns the structural errors,but not providing revision and refinement functions.Therefore,prior to2D-GraLab analysis,protein structures are strongly suggested to be prepro-cessed by programs such as PROCHECK(structure valida-tion),27Scwrl3(side-chain repair),28and X-PLOR(structure refinement).29Individual Schematic DiagramHydrogen BondThe program we use for analyzing hydrogen bonds across bind-ing interfaces is HBplus,23which calculates all possible posi-tions for hydrogen atoms attached to donor atoms which satisfy specified geometrical criteria with acceptor atoms in the vicinity. In2D-GraLab,users can freely select desired hydrogen bonds involving N,O,and/or S atoms.Besides,the water-mediated hydrogen bond is also given consideration.Bond strength of conventional hydrogen bonds(except those of water-mediated Table1.Standalone Programs Employed in2D-GraLab.Program FunctionReduce v3.0322Adding hydrogen atoms for proteinsHBplus v3.1523Identifying hydrogen bonds and calculatingtheir geometric parametersProbe v2.1224Identifying steric contacts and clashes at atomlevelMSMS v2.6125Calculating SASA values of protein atoms andresiduesDelphi v4.026Calculating Coulombic energy and reactionfield energy,determining electrostatic energyof ion-pairsDIMPLOT v4.110Providing application programming interface,users can directly set and executeDIMPLOT in the2D-GraLab GUI9412D Depiction of Nonbonding Interactions for Protein ComplexesFigure1.(a)Schematic representation of a conventional hydrogen bond and a water-mediated hydro-gen bond across the binding interface of IGFBP/IGF complex(PDB entry:2dsr).This diagram was produced using2D-Gralab.The conventional hydrogen bond is formed between the atom N(at the backbone of residue Leu69in chain B)and the atom OE1(at the side-chain of residue Glu3in chain I);The water-mediated hydrogen bond is formed between the atom ND1(at the side-chain of residue His5in chain B)and the atom O(at the backbone of residue Asp20in chain I),and because hydrogen positions of water are almost never known in the PDBfile,the water molecule,when serving as hydrogen bond donor,is not yet determined for its H...A length and D—H...A angle,denoted as mark ‘‘????.’’In this diagram,chains,residues,and atoms are labeled according to the PDB format.(b)Spa-tial conformation of the conventional hydrogen bond.(c)Spatial conformation of the water-mediated hydrogen bond.hydrogen bonds)is calculated using Lennard-Jones 8-6potential with angle weighting.30D U HB¼E m 3d m 8À4d m6"#cos 4h ðh >90 Þ(1)where d is the separation between the heavy acceptor atom andthe donor hydrogen atom in angstroms;E m ,the optimum hydro-gen-bond energy for the particular hydrogen-bonding atoms con-sidered;d m ,the optimum hydrogen-bond length for the particu-lar hydrogen-bonding atoms considered.E m and d m vary accord-ing to the chemical type of the hydrogen-bonding atoms.The hydrogen bond potential is set to zero when angle h 908.31Hydrogen bond parameters are taken from CHARMM force field (for N and O atoms)and Autodock (for S atom).32,33Figure 1a is the schematic representation of a conventional hydrogen bond and a water-mediated hydrogen bond across the binding interface of insulin-like growth factor-binding protein (IGFBP)/insulin-like growth factor (IGF)complex.In this dia-gram,abundant information about the hydrogen bond geometry and energetics properties is presented in a readily acceptant manner.Figures 1b and 1c are spatial conformations of the cor-responding conventional hydrogen bond and water-mediated hydrogen bond.Van der Waals InteractionThe small-probe approach developed in Richardson’s laboratory enables us to detect the all atom contact profile in protein pack-ing.2D-GraLab uses program Probe 24to realize this method to identity steric contacts and clashes on the binding interfaces.Word et al.pointed out that explicit hydrogen atoms can effec-tively improve Probe’s performance.24However,considering calculations with explicit hydrogen atoms are time-consuming,and implicit hydrogen mode is also possibly used in some cases;therefore,in 2D-GraLab,both explicit and implicit hydrogen modes are provided for users.In addition,2D-GraLab uses the Reduce 22to add hydrogen atoms for proteins,and this programis also developed in Richardson’s laboratory and can be wellcompatible with Probe.According to previous definition,vdW interaction between two adjacent atoms is classified into wide contact,close contact,small overlap,and bad overlap.24Typically,vdW potential function has two terms,a repulsive term and an attractive term.In 2D-GraLab,vdW interaction is expressed as Lennard-Jones 12-6potential.34D U SI ¼E m d m d 12À2d md6"#(2)where E m is the Lennard-Jones well depth;d m is the distance at the Lennard-Jones minimum,and d is the distance between two atoms.The Lennard-Jones parameters between pairs of different atom types are obtained from the Lorentz–Berthelodt combina-tion rules.35Atomic Lennard-Jones parameters are taken from Probe and AMBER force field.24,36Figure 2a was produced using 2D-GraLab and gives a sche-matic representation of steric contacts and clashes (overlaps)between the heavy chain residue Tyr131and two light chain res-idues Ser121and Gln124of cross-reaction complex FAB (the antibody fragment of hen egg lysozyme).By this diagram,we can obtain the detail about the local vdW interactions around the residue Tyr131.In contrast,such information is inaccessible in the 3D structural figure (Fig.2b).Desolvation EffectIn 2D-GraLab,program MSMS 25is used to calculate the SASA values of interfacial residues at atom level,and four atomic radii sets are provided for calculating the SASA,including Bondi64,Chothia75,Li98,and CHARMM83.32,37–39Bondi64is based on contact distances in crystals of small molecules;Chothia75is based on contact distances in crystals of amino acids;Li98is derived from 1169high-resolution protein crystal structures;CHARMM83is the atomic radii set of CHARMM force field.Desolvation free energy of interfacial residues is calculated using empirical additive model proposed by Eisenberg andFigure 2.(a)Schematic representation of steric contacts and overlaps between the residue Tyr131in heavy chain (chain H)and the surrounding residues Ser121and Gln124in light chain (chain L)of cross-reaction complex FAB (PDB entry:1fbi).This diagram was produced using 2D-Gralab in explicit hydrogen mode.In this diagram,interface is denoted by the broken line;Wide contact,close contact,small overlap,and bad overlap are marked by blue circle,green triangle,yellow square,and pink rhombus,respectively;Moreover,vdW potential of each atom-pair is given in the histogram,with the value measured by energy scale,and the red and blue indicate favorable (D U \0)and unfav-orable (D U [0)contributions to the binding,respectively;Interaction potential 20.324kcal/mol in the center circle denotes the total vdW contribution by residue Tyr131;Chains,residues,and heavy atoms are labeled according to the PDB format,and hydrogen atoms are labeled in Reduce format.(b)Spatial conformation of chain H residue Tyr131and its local environment.Green or yellow stands forgood contacts (green for close contact and yellow for slight overlaps \0.2A˚),blue for wide contacts [0.25A˚,hot pink spikes for bad overlaps !0.4A ˚.It is revealed that Tyr131is in an intensive clash with chain L Gln124,while in slight contact with chain L Ser121,which is well consistent with the 2D schematic diagram.9432D Depiction of Nonbonding Interactions for Protein Complexes944Zhou,Tian,and Shang•Vol.30,No.6•Journal of Computational ChemistryFigure2.(Legend on page943.)Maclachlam,40and the conformation of interfacial residues is assumed to be invariant during the binding process.D G dslv¼Xic i D A i(3)where the sum is over all the atoms;c i and D A i are the atomic solvation parameter(ASP)and the changes in solvent accessible surface area(D SASA)of atom i,respectively.Juffer et al.41 found that although desolvation free energies calculated from different ASP sets are linear correlation to each other,the abso-lute values are greatly different.In view of that,2D-GraLab pro-vides four ASP sets published in different periods:Eisenberg86, Kim90,Schiffer93,and Zhou02.40,42–44As shown in Figure3,the D SASA and desolvation free energy of interfacial residues in chain A of HLA-A*0201pro-tein complex during the binding process are reproduced in a rotiform diagram form using2D-GraLab.In this diagram,the desolvation free energy contributed by chain A is28.056kcal/ mol,and moreover,the D SASA value of each interfacial residue is also presented clearly.Ion-PairThere are six types of residue-pairs in the ion-pairs:Lys-Asp, Lys-Glu,Arg-Asp,Arg-Glu,His-Asp,and ually,ion-pairs include three kinds:salt bridge,NÀÀO bridge,and longer-range ion-pair,and found that most of the salt bridges are stabi-lizing toward proteins;the majority of NÀÀO bridges are stabi-lizing;the majority of the longer-range ion-pairs are destabiliz-ing toward the proteins.45The salt bridge can be further distin-guished as hydrogen-bonded salt bridge(HB-salt bridge)and nonhydrogen-bonded salt bridge(NHB-salt bridge or salt bridge).46In2D-GraLab,the longer-range ion-pair is neglected, and for short-range ion-pair,four kinds are defined:HB-salt bridge,NHB-salt bridge or salt bridge,hydrogen-bonded NÀÀO bridge(HB-NÀÀO bridge),and nonhydrogen-bonded N-O bridge (NHB-NÀÀO bridge or NÀÀO bridge).Although both the N-terminal and C-terminal residues of a given protein are also charged,the large degree offlexibility usually experienced by the ends of a chain and the poor structural resolution resulting from it.47Therefore,we preclude these terminal residues in the 2D-GraLab.A modified Hendsch–Tidor’s method is used for calculating association energy of ion-pairs across binding interfaces.48D G assoc¼D G dslvþD G brd(4)where D G dslv represents the sum of the unfavorable desolvation penalties incurred by the individual ion-pairing residues due to the change in their environment from a high dielectric solvent (water)in the unassociated state;D G brd represents the favorable bridge energy due to the electrostatic interaction of the side-chain charged groups.We usedfinite difference solutions to the linearized Poisson–Boltzmann equations in Delphi26to calculate the D G dslv and D G brd.Centroid of the ion-pair system is used as grid center,with temperature of298.15K(in this way,1kT50.593kcal/mol),and the Debye-Huckel boundary conditions are applied.49Considering atomic parameter sets have a great influ-ence on the continuum electrostatic calculations of ion-pair asso-ciation energy,502D-GraLab provides three classical atomic parameter sets for users,including PARSE,AMBER,and CHARMM.51–53Figure4is the schematic representation of four ion-pairs formed across the binding interface of penicillin acylase enzyme complex.This diagram clearly illustrates the information about the geometries and energetics properties of ion-pairs,such as bond length,centroid distance,association energy,and angle. The ion-pair angle is defined as the angle between two unit vec-tors,and each unit vector joins a C a atom and a side-chain charged group centroid in an ion-pairing residue.54In this dia-gram,the four ion-pairs,two HB-salt bridges,and two HB-NÀÀO bridges formed across the binding interface are given out. Association energies of the HB-salt bridges are both\21.5 kcal/mol,whereas that of the HB-NÀÀO bridges are all[20.5 kcal/mol.Therefore,it is believed that HB-salt bridge is more stable than HB-NÀÀO bridge,which is well consistent with the conclusion of Kumar and Nussinov.45,46Side-Chain Conformational EntropyIn general,SCE can be divided into the vibrational and the con-formational.55Comparison of several sets of results using differ-ent techniques shows that during protein folding process,the mean conformational free energy change(T D S)is1kcal/mol per side-chain or0.5kcal/mol per bond.Changes in vibrational entropy appear to be negligible compared with the entropy change resulted from the loss of accessible rotamers.56SCE(S) can be calculated quite simply using Boltzmann’s formulation.57S¼ÀRXip i ln p i(5)where R is the universal gas constant;The sum is taken over all conformational states of the system and p i is the probability of being in state i.Typical methods used for SCE calculations, include self-consistent meanfield theory,58molecular dynam-ics,59Monte Carlo simulation,60etc.,that are all time-consum-ing,thus not suitable for2D-GraLab.For that,the case is sim-plified,when we calculate the SCE of an interfacial residue,its local surrounding isfixed(adopting crystal conformation).In this way,SCE of each interfacial residue is calculated in turn.For the20coded amino acids,Gly,Ala,Pro,and Cys in disulfide bonds are excluded.57For other cases,each residue’s side-chain conformation is modeled as a rotamer withfinite number of discrete states.61The penultimate rotamer library used was developed by Lovell et al.,62as recommended by Dun-brack for the study of SCE.63For an interfacial residue,the potential E i of each rotamer i is calculated in both binding state and unbinding state,and subsequently,rotamer’s probability dis-tribution(p)of this residue is resulted by Boltzmann’s distribu-tion law,then the SCE in different states are solved out using eq.(5).The situation of rotamer i is defined as serious clash or nonclash:serious clash is the clash score of rotamer i more than a given threshold value,and then E i511;whereas for the9452D Depiction of Nonbonding Interactions for Protein Complexes946Zhou,Tian,and Shang•Vol.30,No.6•Journal of Computational ChemistryFigure3.Schematic representation of desolvation effect for interfacial residues in chain A of HLA-A*0201complex(PDB entry:1duz).This diagram was produced using2D-GraLab.In this diagram,the pie chart is equally divided,with each section indicates an interfacial residue in chain A;In a sec-tor,red1blue is the SASA of corresponding residue in unbinding state,the blue is in binding state,and the red is thus of D SASA;The green polygonal line is made by linking desolvation free energy ofeach interfacial residue,and at the purple circle,desolvation free energy is0(D U50),beyond thiscircle indicates unfavorable contributions to binding(D U[0),otherwise is favorable(D U\0);Inthe periphery,residue symbols are colored in red,blue,and black in terms of favorable,unfavorable,and neutral contributions to the binding,respectively;The SASA and desolvation free energy for eachinterfacial residue can be measured qualitatively by the horizontally black and green scales.[Colorfigure can be viewed in the online issue,which is available at .]Figure4.Four ion-pairs formed across the binding interface of penicillin acylase enzyme complex (PDB entry:1gkf).In thisfigure,left is2D schematic diagram produced using2D-GraLab,and posi-tively and negatively charged residues are colored in blue and red,respectively;Bridge-bonds formed between the charged atoms of ion-pairs are colored in green,blue,and yellow dashed lines for the hydrogen-bonded bridge,nonhydrogen-bonded bridge,and long-range interactions,respectively;The three parameters in bracket are ion-pair type,angle,and association energy.The right in thisfigure is the spatial conformations of corresponding ion-pairs.[Colorfigure can be viewed in the online issue, which is available at .]Figure5.(a)Loss of side-chain conformational entropy of chain B interfacial residues in HIV-1 reverse transcriptase complex(PDB entry:1rt1).This diagram was produced using2D-GraLab.In this diagram,the pie chart is equally divided,with each section indicates an interfacial residue in chain B; In a sector,side-chain conformational entropies in unbinding and binding state are colored in yellow and blue,respectively;The green polygonal line is made by linking conformational free energy of each interfacial residue;The conformational entropy and conformational free energy for each interfa-cial residue can be measured qualitatively by the horizontally black and green scales,respectively;In the periphery,residue symbols are colored in yellow,blue,and black in terms of favorable,unfavora-ble,and neutral contributions to binding,respectively.(b)The rotamers of chain B interfacial residues Lys20,Lys22,Tyr56,Asn136,Ile393,and Trp401in HIV-1reverse transcriptase complex.These rotamers were generated using2D-GraLab.[Colorfigure can be viewed in the online issue,which is available at .]9472D Depiction of Nonbonding Interactions for Protein Complexes948Zhou,Tian,and Shang•Vol.30,No.6•Journal of Computational ChemistryFigure5.(Legend on page947.)Figure6.The summarized schematic diagram of nonbonding interactions and disulfide bond across the interface of AIV hemagglutinin H5complex(PDB entry:1jsm).Length of chain A and chain B are321and160,represented as two bold horizontal lines.Interface parts in the bold lines are colored in orange,and residue-pairs in interactions are linearly linked;Conventional hydrogen bond,water-mediated hydrogen bond,ionpair,hydrophobic force,steric clash,p–p stacking,and disulfide bond are colored in aqua,bottle green,red,blue,purple,yellow,and brown,respectively;In the‘‘dumbbell shape’’symbols,residue-pair types and distances are also presented.[Colorfigure can be viewed in the online issue,which is available at .]9492D Depiction of Nonbonding Interactions for Protein Complexescase of nonclash,four potential functions are used in2D-Gra-Lab:(i)E i5E0,a constant61;(ii)statistical potential,the poten-tial energy E i of rotamer i is calculated from database-derived probability61;(iii)coarse-grained model,E i of rotamer i is esti-mated by atomic contact energies(ACE)64;and(iv)Lennard-Jones potential.58Loss of binding entropy of chain B interfacial residues in HIV-1reverse transcriptase complex is schematically repre-sented in Figure5a.Similar to desolvation effect diagram,loss of binding entropy is also presented in a rotiform diagram form. This diagram reveals that during the process of forming HIV-1 reverse transcriptase complex,the total loss of conformational free energy of chain B is9.14kcal/mol,indicating a strongly unfavorable contribution to binding(D G[0),and the average loss of conformational free energy for each residue is about0.3 kcal/mol,much less than those in protein folding(about1kcal/ mol56).Figure5b shows the rotamers of six interfacial residues in chain B.Summarized Schematic DiagramFigure6illustrates nonbonding interactions and disulfide bond formed across the binding interface of avian influenza virus (AIV)hemagglutinin H5.This protein is a dimer linked by a disulfide bond.In this diagram,conventional hydrogen bond, water-mediated hydrogen bond,ion-pair,hydrophobic force, steric clash,p–p stacking,and disulfide bond are represented in different colors.Hydrogen bonds,colored in aqua,are calculated by program HBplus.23Data in this diagram are the separation between the acceptor atom and the heavy donor atom.Water-mediated hydrogen bonds are colored in bottle green, also calculated by HBplus.23Ion-pairs,colored in red,include salt bridge and NÀÀO bridge,determined by the Kumar’s rule.45,46Data in this dia-gram are centroid distance of ion-pair.Hydrophobic forces are colored in blue.According to the D SASA rule,if the two apolar and/or aromatic interfacial resi-dues(Leu,Ala,Val,Ile,Met,Cys,Pro,Tyr,Phe,and Trp)are within the distance d\r A1r B12.8(r A and r B are side-chain radii,2.8is the diameter of water molecule),they are considered in hydrophobic contact.Data in this diagram are centroid–cent-roid separation between the two residues.Steric clashes are colored in purple.Here,only bad overlaps calculated by Probe24are presented.In2D-GraLab,explicit and implicit hydrogen modes are provided,hydrogen atoms in explicit hydrogern mode are added using Reduce.22Data in this diagram are the centroid–centroid separation when the two atoms are badly overlapped.p–p stacking are colored in yellow.Presently,studies on pro-tein stacking interactions are in lack.In2D-GraLab,p–p stack-ing is identified using the McGaughey’s rule,65i.e.,if the cent-roid–centroid separation between two aromatic rings is within 7.5A˚,they are regarded as p–p stacking(aromatic residues are Phe,Tyr,Trp,and His).This rule has been successfully adopted to study the p–p stacking across protein interfaces by Cho et al.66Besides,2D-GraLab also sets the constraints of stacking angle(dihedral angel between the planes of two aromatic rings).Data in this diagram are centroid–centroid separations between two aromatic rings in stacking state.Disulfide bonds are colored in brown,taken from the PDB records.Data in this diagram are the separations of two sulfide atoms.ConclusionsMost,if not all,biological processes are regulated through asso-ciation and dissociation of protein molecules and essentially controlled by nonbonding energetics.67Graphically-intuitive vis-ualization of these nonbonding interactions is an important approach for understanding the mechanism of a complex formed between two proteins.Although a large number of software packages are available for visualizing the3D structures,the options for producing schematic2D summaries of nonbonding interactions for a protein complex are comparatively few.In practice,the2D and3D visualization methods are complemen-tary.In this article,we have described a new2D molecular graphics tool for analyzing and visualizing PPIs from spatial structures,and the intended goal is to schematically present the nonbonding interactions stabilizing the macromolecular complex in a graphically-intuitive manner.We anticipate that renewed in-terest in automated generation of2D diagrams will significantly reduce the burden of protein structure analysis and make insights into the mechanism of PPIs.2D-GraLab is written in C11and OpenGL,and the output-ted2D schematic diagrams of nonbinding interactions are described in PostScript.Presently,2D-GraLab v1.0is available to academic users free of charge by contacting us. References1.Chothia,C.;Janin,J.Nature1974,256,705.2.Jones,S.;Thornton,J.M.Proc Natl Acad Sci USA1996,93,13.3.Luscombe,N.M.;Laskowski,R.A.;Westhead,D.R.;Milburn,D.;Jones,S.;Karmirantzoua,M.;Thornton,J.M.Acta Crystallogr D 1998,54,1132.4.DeLano,W.L.The PyMOL Molecular Graphics System;DeLanoScientific:San Carlos,CA,2002.5.Petrey,D.;Honig,B.Methods Enzymol2003,374,492.6.Humphrey,W.;Dalke,A.;Schulten,K.J Mol Graphics1996,14,33.7.Gabdoulline,R.R.;Wade,R.C.;Walther,D.Nucleic Acids Res2003,31,3349.8.Gabdoulline,R.R.;Hoffmann,R.;Leitner,F.;Wade,R.C.Bioin-formatics2003,19,1723.9.Wade,R. C.;Gabdoulline,R.R.;De Rienzo, F.Int J QuantumChem2001,83,122.10.Wallace, A. C.;Laskowski,R. A.;Thornton,J.M.Protein Eng1995,8,127.11.Stierand,K.;Maaß,P.C.;Rarey,M.Bioinformatics2006,22,1710.12.Clark,A.M.;Labute,P.J Chem Inf Model2007,47,1933.13.Luscombe,N.M.;Laskowski,R. A.;Thorntonm J.M.NucleicAcids Res1997,25,4940.14.Salerno,W.J.;Seaver,S.M.;Armstrong,B.R.;Radhakrishnan,I.Nucleic Acids Res2004,32,W566.15.Fischer,T.B.;Holmes,J.B.;Miller,I.R.;Parsons,J.R.;Tung,L.;Hu,J.C.;Tsai,J.J Struct Biol2006,153,103.950Zhou,Tian,and Shang•Vol.30,No.6•Journal of Computational Chemistry。
A Fast and Accurate Plane Detection Algorithm for Large Noisy Point Clouds Using Filtered Normals

A Fast and Accurate Plane Detection Algorithm for Large Noisy Point CloudsUsing Filtered Normals and Voxel GrowingJean-Emmanuel DeschaudFranc¸ois GouletteMines ParisTech,CAOR-Centre de Robotique,Math´e matiques et Syst`e mes60Boulevard Saint-Michel75272Paris Cedex06jean-emmanuel.deschaud@mines-paristech.fr francois.goulette@mines-paristech.frAbstractWith the improvement of3D scanners,we produce point clouds with more and more points often exceeding millions of points.Then we need a fast and accurate plane detection algorithm to reduce data size.In this article,we present a fast and accurate algorithm to detect planes in unorganized point clouds usingfiltered normals and voxel growing.Our work is based on afirst step in estimating better normals at the data points,even in the presence of noise.In a second step,we compute a score of local plane in each point.Then, we select the best local seed plane and in a third step start a fast and robust region growing by voxels we call voxel growing.We have evaluated and tested our algorithm on different kinds of point cloud and compared its performance to other algorithms.1.IntroductionWith the growing availability of3D scanners,we are now able to produce large datasets with millions of points.It is necessary to reduce data size,to decrease the noise and at same time to increase the quality of the model.It is in-teresting to model planar regions of these point clouds by planes.In fact,plane detection is generally afirst step of segmentation but it can be used for many applications.It is useful in computer graphics to model the environnement with basic geometry.It is used for example in modeling to detect building facades before classification.Robots do Si-multaneous Localization and Mapping(SLAM)by detect-ing planes of the environment.In our laboratory,we wanted to detect small and large building planes in point clouds of urban environments with millions of points for modeling. As mentioned in[6],the accuracy of the plane detection is important for after-steps of the modeling pipeline.We also want to be fast to be able to process point clouds with mil-lions of points.We present a novel algorithm based on re-gion growing with improvements in normal estimation and growing process.For our method,we are generic to work on different kinds of data like point clouds fromfixed scan-ner or from Mobile Mapping Systems(MMS).We also aim at detecting building facades in urban point clouds or little planes like doors,even in very large data sets.Our input is an unorganized noisy point cloud and with only three”in-tuitive”parameters,we generate a set of connected compo-nents of planar regions.We evaluate our method as well as explain and analyse the significance of each parameter. 2.Previous WorksAlthough there are many methods of segmentation in range images like in[10]or in[3],three have been thor-oughly studied for3D point clouds:region-growing, hough-transform from[14]and Random Sample Consen-sus(RANSAC)from[9].The application of recognising structures in urban laser point clouds is frequent in literature.Bauer in[4]and Boulaassal in[5]detect facades in dense3D point cloud by a RANSAC algorithm.V osselman in[23]reviews sur-face growing and3D hough transform techniques to de-tect geometric shapes.Tarsh-Kurdi in[22]detect roof planes in3D building point cloud by comparing results on hough-transform and RANSAC algorithm.They found that RANSAC is more efficient than thefirst one.Chao Chen in[6]and Yu in[25]present algorithms of segmentation in range images for the same application of detecting planar regions in an urban scene.The method in[6]is based on a region growing algorithm in range images and merges re-sults in one labelled3D point cloud.[25]uses a method different from the three we have cited:they extract a hi-erarchical subdivision of the input image built like a graph where leaf nodes represent planar regions.There are also other methods like bayesian techniques. In[16]and[8],they obtain smoothed surface from noisy point clouds with objects modeled by probability distribu-tions and it seems possible to extend this idea to point cloud segmentation.But techniques based on bayesian statistics need to optimize global statistical model and then it is diffi-cult to process points cloud larger than one million points.We present below an analysis of the two main methods used in literature:RANSAC and region-growing.Hough-transform algorithm is too time consuming for our applica-tion.To compare the complexity of the algorithm,we take a point cloud of size N with only one plane P of size n.We suppose that we want to detect this plane P and we define n min the minimum size of the plane we want to detect.The size of a plane is the area of the plane.If the data density is uniform in the point cloud then the size of a plane can be specified by its number of points.2.1.RANSACRANSAC is an algorithm initially developped by Fis-chler and Bolles in[9]that allows thefitting of models with-out trying all possibilities.RANSAC is based on the prob-ability to detect a model using the minimal set required to estimate the model.To detect a plane with RANSAC,we choose3random points(enough to estimate a plane).We compute the plane parameters with these3points.Then a score function is used to determine how the model is good for the remaining ually,the score is the number of points belonging to the plane.With noise,a point belongs to a plane if the distance from the point to the plane is less than a parameter γ.In the end,we keep the plane with the best score.Theprobability of getting the plane in thefirst trial is p=(nN )3.Therefore the probability to get it in T trials is p=1−(1−(nN )3)ing equation1and supposing n minN1,we know the number T min of minimal trials to have a probability p t to get planes of size at least n min:T min=log(1−p t)log(1−(n minN))≈log(11−p t)(Nn min)3.(1)For each trial,we test all data points to compute the score of a plane.The RANSAC algorithm complexity lies inO(N(Nn min )3)when n minN1and T min→0whenn min→N.Then RANSAC is very efficient in detecting large planes in noisy point clouds i.e.when the ratio n minN is 1but very slow to detect small planes in large pointclouds i.e.when n minN 1.After selecting the best model,another step is to extract the largest connected component of each plane.Connnected components mean that the min-imum distance between each point of the plane and others points is smaller(for distance)than afixed parameter.Schnabel et al.[20]bring two optimizations to RANSAC:the points selection is done locally and the score function has been improved.An octree isfirst created from point cloud.Points used to estimate plane parameters are chosen locally at a random depth of the octree.The score function is also different from RANSAC:instead of testing all points for one model,they test only a random subset and find the score by interpolation.The algorithm complexity lies in O(Nr4Ndn min)where r is the number of random subsets for the score function and d is the maximum octree depth. Their algorithm improves the planes detection speed but its complexity lies in O(N2)and it becomes slow on large data sets.And again we have to extract the largest connected component of each plane.2.2.Region GrowingRegion Growing algorithms work well in range images like in[18].The principle of region growing is to start with a seed region and to grow it by neighborhood when the neighbors satisfy some conditions.In range images,we have the neighbors of each point with pixel coordinates.In case of unorganized3D data,there is no information about the neighborhood in the data structure.The most common method to compute neighbors in3D is to compute a Kd-tree to search k nearest neighbors.The creation of a Kd-tree lies in O(NlogN)and the search of k nearest neighbors of one point lies in O(logN).The advantage of these region growing methods is that they are fast when there are many planes to extract,robust to noise and extract the largest con-nected component immediately.But they only use the dis-tance from point to plane to extract planes and like we will see later,it is not accurate enough to detect correct planar regions.Rabbani et al.[19]developped a method of smooth area detection that can be used for plane detection.Theyfirst estimate the normal of each point like in[13].The point with the minimum residual starts the region growing.They test k nearest neighbors of the last point added:if the an-gle between the normal of the point and the current normal of the plane is smaller than a parameterαthen they add this point to the smooth region.With Kd-tree for k nearest neighbors,the algorithm complexity is in O(N+nlogN). The complexity seems to be low but in worst case,when nN1,example for facade detection in point clouds,the complexity becomes O(NlogN).3.Voxel Growing3.1.OverviewIn this article,we present a new algorithm adapted to large data sets of unorganized3D points and optimized to be accurate and fast.Our plane detection method works in three steps.In thefirst part,we compute a better esti-mation of the normal in each point by afiltered weighted planefitting.In a second step,we compute the score of lo-cal planarity in each point.We select the best seed point that represents a good seed plane and in the third part,we grow this seed plane by adding all points close to the plane.Thegrowing step is based on a voxel growing algorithm.The filtered normals,the score function and the voxel growing are innovative contributions of our method.As an input,we need dense point clouds related to the level of detail we want to detect.As an output,we produce connected components of planes in the point cloud.This notion of connected components is linked to the data den-sity.With our method,the connected components of planes detected are linked to the parameter d of the voxel grid.Our method has 3”intuitive”parameters :d ,area min and γ.”intuitive”because there are linked to physical mea-surements.d is the voxel size used in voxel growing and also represents the connectivity of points in detected planes.γis the maximum distance between the point of a plane and the plane model,represents the plane thickness and is linked to the point cloud noise.area min represents the minimum area of planes we want to keep.3.2.Details3.2.1Local Density of Point CloudsIn a first step,we compute the local density of point clouds like in [17].For that,we find the radius r i of the sphere containing the k nearest neighbors of point i .Then we cal-culate ρi =kπr 2i.In our experiments,we find that k =50is a good number of neighbors.It is important to know the lo-cal density because many laser point clouds are made with a fixed resolution angle scanner and are therefore not evenly distributed.We use the local density in section 3.2.3for the score calculation.3.2.2Filtered Normal EstimationNormal estimation is an important part of our algorithm.The paper [7]presents and compares three normal estima-tion methods.They conclude that the weighted plane fit-ting or WPF is the fastest and the most accurate for large point clouds.WPF is an idea of Pauly and al.in [17]that the fitting plane of a point p must take into consider-ation the nearby points more than other distant ones.The normal least square is explained in [21]and is the mini-mum of ki =1(n p ·p i +d )2.The WPF is the minimum of ki =1ωi (n p ·p i +d )2where ωi =θ( p i −p )and θ(r )=e −2r 2r2i .For solving n p ,we compute the eigenvec-tor corresponding to the smallest eigenvalue of the weightedcovariance matrix C w = ki =1ωi t (p i −b w )(p i −b w )where b w is the weighted barycenter.For the three methods ex-plained in [7],we get a good approximation of normals in smooth area but we have errors in sharp corners.In fig-ure 1,we have tested the weighted normal estimation on two planes with uniform noise and forming an angle of 90˚.We can see that the normal is not correct on the corners of the planes and in the red circle.To improve the normal calculation,that improves the plane detection especially on borders of planes,we propose a filtering process in two phases.In a first step,we com-pute the weighted normals (WPF)of each point like we de-scribed it above by minimizing ki =1ωi (n p ·p i +d )2.In a second step,we compute the filtered normal by us-ing an adaptive local neighborhood.We compute the new weighted normal with the same sum minimization but keep-ing only points of the neighborhood whose normals from the first step satisfy |n p ·n i |>cos (α).With this filtering step,we have the same results in smooth areas and better results in sharp corners.We called our normal estimation filtered weighted plane fitting(FWPF).Figure 1.Weighted normal estimation of two planes with uniform noise and with 90˚angle between them.We have tested our normal estimation by computing nor-mals on synthetic data with two planes and different angles between them and with different values of the parameter α.We can see in figure 2the mean error on normal estimation for WPF and FWPF with α=20˚,30˚,40˚and 90˚.Us-ing α=90˚is the same as not doing the filtering step.We see on Figure 2that α=20˚gives smaller error in normal estimation when angles between planes is smaller than 60˚and α=30˚gives best results when angle between planes is greater than 60˚.We have considered the value α=30˚as the best results because it gives the smaller mean error in normal estimation when angle between planes vary from 20˚to 90˚.Figure 3shows the normals of the planes with 90˚angle and better results in the red circle (normals are 90˚with the plane).3.2.3The score of local planarityIn many region growing algorithms,the criteria used for the score of the local fitting plane is the residual,like in [18]or [19],i.e.the sum of the square of distance from points to the plane.We have a different score function to estimate local planarity.For that,we first compute the neighbors N i of a point p with points i whose normals n i are close toFigure parison of mean error in normal estimation of two planes with α=20˚,30˚,40˚and 90˚(=Nofiltering).Figure 3.Filtered Weighted normal estimation of two planes with uniform noise and with 90˚angle between them (α=30˚).the normal n p .More precisely,we compute N i ={p in k neighbors of i/|n i ·n p |>cos (α)}.It is a way to keep only the points which are probably on the local plane before the least square fitting.Then,we compute the local plane fitting of point p with N i neighbors by least squares like in [21].The set N i is a subset of N i of points belonging to the plane,i.e.the points for which the distance to the local plane is smaller than the parameter γ(to consider the noise).The score s of the local plane is the area of the local plane,i.e.the number of points ”in”the plane divided by the localdensity ρi (seen in section 3.2.1):the score s =card (N i)ρi.We take into consideration the area of the local plane as the score function and not the number of points or the residual in order to be more robust to the sampling distribution.3.2.4Voxel decompositionWe use a data structure that is the core of our region growing method.It is a voxel grid that speeds up the plane detection process.V oxels are small cubes of length d that partition the point cloud space.Every point of data belongs to a voxel and a voxel contains a list of points.We use the Octree Class Template in [2]to compute an Octree of the point cloud.The leaf nodes of the graph built are voxels of size d .Once the voxel grid has been computed,we start the plane detection algorithm.3.2.5Voxel GrowingWith the estimator of local planarity,we take the point p with the best score,i.e.the point with the maximum area of local plane.We have the model parameters of this best seed plane and we start with an empty set E of points belonging to the plane.The initial point p is in a voxel v 0.All the points in the initial voxel v 0for which the distance from the seed plane is less than γare added to the set E .Then,we compute new plane parameters by least square refitting with set E .Instead of growing with k nearest neighbors,we grow with voxels.Hence we test points in 26voxel neigh-bors.This is a way to search the neighborhood in con-stant time instead of O (logN )for each neighbor like with Kd-tree.In a neighbor voxel,we add to E the points for which the distance to the current plane is smaller than γand the angle between the normal computed in each point and the normal of the plane is smaller than a parameter α:|cos (n p ,n P )|>cos (α)where n p is the normal of the point p and n P is the normal of the plane P .We have tested different values of αand we empirically found that 30˚is a good value for all point clouds.If we added at least one point in E for this voxel,we compute new plane parameters from E by least square fitting and we test its 26voxel neigh-bors.It is important to perform plane least square fitting in each voxel adding because the seed plane model is not good enough with noise to be used in all voxel growing,but only in surrounding voxels.This growing process is faster than classical region growing because we do not compute least square for each point added but only for each voxel added.The least square fitting step must be computed very fast.We use the same method as explained in [18]with incre-mental update of the barycenter b and covariance matrix C like equation 2.We know with [21]that the barycen-ter b belongs to the least square plane and that the normal of the least square plane n P is the eigenvector of the smallest eigenvalue of C .b0=03x1C0=03x3.b n+1=1n+1(nb n+p n+1).C n+1=C n+nn+1t(pn+1−b n)(p n+1−b n).(2)where C n is the covariance matrix of a set of n points,b n is the barycenter vector of a set of n points and p n+1is the (n+1)point vector added to the set.This voxel growing method leads to a connected com-ponent set E because the points have been added by con-nected voxels.In our case,the minimum distance between one point and E is less than parameter d of our voxel grid. That is why the parameter d also represents the connectivity of points in detected planes.3.2.6Plane DetectionTo get all planes with an area of at least area min in the point cloud,we repeat these steps(best local seed plane choice and voxel growing)with all points by descending order of their score.Once we have a set E,whose area is bigger than area min,we keep it and classify all points in E.4.Results and Discussion4.1.Benchmark analysisTo test the improvements of our method,we have em-ployed the comparative framework of[12]based on range images.For that,we have converted all images into3D point clouds.All Point Clouds created have260k points. After our segmentation,we project labelled points on a seg-mented image and compare with the ground truth image. We have chosen our three parameters d,area min andγby optimizing the result of the10perceptron training image segmentation(the perceptron is portable scanner that pro-duces a range image of its environment).Bests results have been obtained with area min=200,γ=5and d=8 (units are not provided in the benchmark).We show the re-sults of the30perceptron images segmentation in table1. GT Regions are the mean number of ground truth planes over the30ground truth range images.Correct detection, over-segmentation,under-segmentation,missed and noise are the mean number of correct,over,under,missed and noised planes detected by methods.The tolerance80%is the minimum percentage of points we must have detected comparing to the ground truth to have a correct detection. More details are in[12].UE is a method from[12],UFPR is a method from[10]. It is important to notice that UE and UFPR are range image methods and our method is not well suited for range images but3D Point Cloud.Nevertheless,it is a good benchmark for comparison and we see in table1that the accuracy of our method is very close to the state of the art in range image segmentation.To evaluate the different improvements of our algorithm, we have tested different variants of our method.We have tested our method without normals(only with distance from points to plane),without voxel growing(with a classical region growing by k neighbors),without our FWPF nor-mal estimation(with WPF normal estimation),without our score function(with residual score function).The compari-son is visible on table2.We can see the difference of time computing between region growing and voxel growing.We have tested our algorithm with and without normals and we found that the accuracy cannot be achieved whithout normal computation.There is also a big difference in the correct de-tection between WPF and our FWPF normal estimation as we can see in thefigure4.Our FWPF normal brings a real improvement in border estimation of planes.Black points in thefigure are non classifiedpoints.Figure5.Correct Detection of our segmentation algorithm when the voxel size d changes.We would like to discuss the influence of parameters on our algorithm.We have three parameters:area min,which represents the minimum area of the plane we want to keep,γ,which represents the thickness of the plane(it is gener-aly closely tied to the noise in the point cloud and espe-cially the standard deviationσof the noise)and d,which is the minimum distance from a point to the rest of the plane. These three parameters depend on the point cloud features and the desired segmentation.For example,if we have a lot of noise,we must choose a highγvalue.If we want to detect only large planes,we set a large area min value.We also focus our analysis on the robustess of the voxel size d in our algorithm,i.e.the ratio of points vs voxels.We can see infigure5the variation of the correct detection when we change the value of d.The method seems to be robust when d is between4and10but the quality decreases when d is over10.It is due to the fact that for a large voxel size d,some planes from different objects are merged into one plane.GT Regions Correct Over-Under-Missed Noise Duration(in s)detection segmentation segmentationUE14.610.00.20.3 3.8 2.1-UFPR14.611.00.30.1 3.0 2.5-Our method14.610.90.20.1 3.30.7308Table1.Average results of different segmenters at80%compare tolerance.GT Regions Correct Over-Under-Missed Noise Duration(in s) Our method detection segmentation segmentationwithout normals14.6 5.670.10.19.4 6.570 without voxel growing14.610.70.20.1 3.40.8605 without FWPF14.69.30.20.1 5.0 1.9195 without our score function14.610.30.20.1 3.9 1.2308 with all improvements14.610.90.20.1 3.30.7308 Table2.Average results of variants of our segmenter at80%compare tolerance.4.1.1Large scale dataWe have tested our method on different kinds of data.We have segmented urban data infigure6from our Mobile Mapping System(MMS)described in[11].The mobile sys-tem generates10k pts/s with a density of50pts/m2and very noisy data(σ=0.3m).For this point cloud,we want to de-tect building facades.We have chosen area min=10m2, d=1m to have large connected components andγ=0.3m to cope with the noise.We have tested our method on point cloud from the Trim-ble VX scanner infigure7.It is a point cloud of size40k points with only20pts/m2with less noise because it is a fixed scanner(σ=0.2m).In that case,we also wanted to detect building facades and keep the same parameters ex-ceptγ=0.2m because we had less noise.We see infig-ure7that we have detected two facades.By setting a larger voxel size d value like d=10m,we detect only one plane. We choose d like area min andγaccording to the desired segmentation and to the level of detail we want to extract from the point cloud.We also tested our algorithm on the point cloud from the LEICA Cyrax scanner infigure8.This point cloud has been taken from AIM@SHAPE repository[1].It is a very dense point cloud from multiplefixed position of scanner with about400pts/m2and very little noise(σ=0.02m). In this case,we wanted to detect all the little planes to model the church in planar regions.That is why we have chosen d=0.2m,area min=1m2andγ=0.02m.Infigures6,7and8,we have,on the left,input point cloud and on the right,we only keep points detected in a plane(planes are in random colors).The red points in thesefigures are seed plane points.We can see in thesefig-ures that planes are very well detected even with high noise. Table3show the information on point clouds,results with number of planes detected and duration of the algorithm.The time includes the computation of the FWPF normalsof the point cloud.We can see in table3that our algo-rithm performs linearly in time with respect to the numberof points.The choice of parameters will have little influence on time computing.The computation time is about one mil-lisecond per point whatever the size of the point cloud(we used a PC with QuadCore Q9300and2Go of RAM).The algorithm has been implented using only one thread andin-core processing.Our goal is to compare the improve-ment of plane detection between classical region growing and our region growing with better normals for more ac-curate planes and voxel growing for faster detection.Our method seems to be compatible with out-of-core implemen-tation like described in[24]or in[15].MMS Street VX Street Church Size(points)398k42k7.6MMean Density50pts/m220pts/m2400pts/m2 Number of Planes202142Total Duration452s33s6900sTime/point 1ms 1ms 1msTable3.Results on different data.5.ConclusionIn this article,we have proposed a new method of plane detection that is fast and accurate even in presence of noise. We demonstrate its efficiency with different kinds of data and its speed in large data sets with millions of points.Our voxel growing method has a complexity of O(N)and it is able to detect large and small planes in very large data sets and can extract them directly in connected components.Figure 4.Ground truth,Our Segmentation without and with filterednormals.Figure 6.Planes detection in street point cloud generated by MMS (d =1m,area min =10m 2,γ=0.3m ).References[1]Aim@shape repository /.6[2]Octree class template /code/octree.html.4[3] A.Bab-Hadiashar and N.Gheissari.Range image segmen-tation using surface selection criterion.2006.IEEE Trans-actions on Image Processing.1[4]J.Bauer,K.Karner,K.Schindler,A.Klaus,and C.Zach.Segmentation of building models from dense 3d point-clouds.2003.Workshop of the Austrian Association for Pattern Recognition.1[5]H.Boulaassal,ndes,P.Grussenmeyer,and F.Tarsha-Kurdi.Automatic segmentation of building facades using terrestrial laser data.2007.ISPRS Workshop on Laser Scan-ning.1[6] C.C.Chen and I.Stamos.Range image segmentationfor modeling and object detection in urban scenes.2007.3DIM2007.1[7]T.K.Dey,G.Li,and J.Sun.Normal estimation for pointclouds:A comparison study for a voronoi based method.2005.Eurographics on Symposium on Point-Based Graph-ics.3[8]J.R.Diebel,S.Thrun,and M.Brunig.A bayesian methodfor probable surface reconstruction and decimation.2006.ACM Transactions on Graphics (TOG).1[9]M.A.Fischler and R.C.Bolles.Random sample consen-sus:A paradigm for model fitting with applications to image analysis and automated munications of the ACM.1,2[10]P.F.U.Gotardo,O.R.P.Bellon,and L.Silva.Range imagesegmentation by surface extraction using an improved robust estimator.2003.Proceedings of Computer Vision and Pat-tern Recognition.1,5[11] F.Goulette,F.Nashashibi,I.Abuhadrous,S.Ammoun,andurgeau.An integrated on-board laser range sensing sys-tem for on-the-way city and road modelling.2007.Interna-tional Archives of the Photogrammetry,Remote Sensing and Spacial Information Sciences.6[12] A.Hoover,G.Jean-Baptiste,and al.An experimental com-parison of range image segmentation algorithms.1996.IEEE Transactions on Pattern Analysis and Machine Intelligence.5[13]H.Hoppe,T.DeRose,T.Duchamp,J.McDonald,andW.Stuetzle.Surface reconstruction from unorganized points.1992.International Conference on Computer Graphics and Interactive Techniques.2[14]P.Hough.Method and means for recognizing complex pat-terns.1962.In US Patent.1[15]M.Isenburg,P.Lindstrom,S.Gumhold,and J.Snoeyink.Large mesh simplification using processing sequences.2003.。
demo2--shapeofmolecule5

• The shape is modified by the lone pairs.
• Repulsion of the bond pairs by any lone pairs reduces the bond angle.
4
Species with two electron pairs
• The two electron pairs repel each other to a position of minimum repulsion(maximum separation) with a linear arrangement.
two electron pairs, linear
Beryllium Chloride, BeCl2
• Group 2, two σ-bonds, no lone pairs • Bond angle 180°
5
• Carbon dioxide. CO2 • O=C=O two σ-bonds and two π-bonds, no lone pairs, linear.
9
Species with four electron pairs
• The four electron pairs repel each other to a position of minimum repulsion(maximum separation) with a threedimension tetrahedral arrangement.
2
Valence Share Electron Pair Repulsion
– The multiple electron pairs in a double bond or triple bond are treated as though they were a single "pair". – The repulsion caused by the lone pair is greater than the repulsion caused by the bonding pair. – In other words, the lone pair-lone pair repulsion is considered to be stronger than the lone pair-bonding pair repulsion, which in turn is stronger than the bonding pair-bonding pair repulsion.
硅藻页岩的调湿性能的比较研究和结构分析
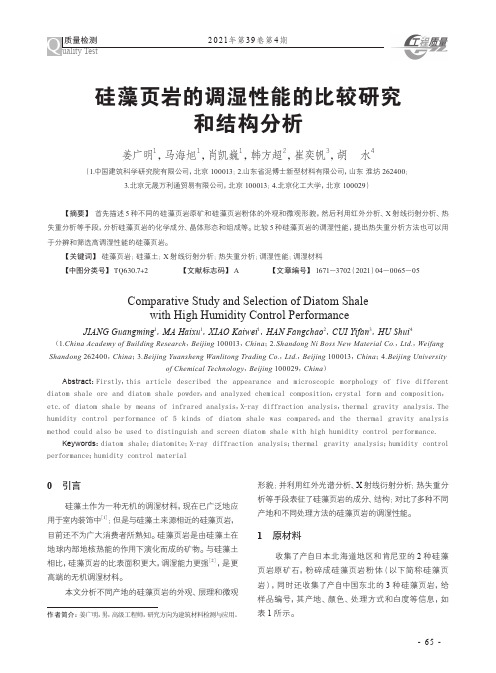
- 65 -硅藻页岩的调湿性能的比较研究和结构分析姜广明1,马海旭1,肖凯巍1,韩方超2,崔奕帆3,胡 水4(1.中国建筑科学研究院有限公司,北京 100013;2.山东省泥博士新型材料有限公司,山东 淮坊 262400;3.北京元晟万利通贸易有限公司,北京 100013;4.北京化工大学,北京 100029) 【摘要】 首先描述 5 种不同的硅藻页岩原矿和硅藻页岩粉体的外观和微观形貌,然后利用红外分析、X 射线衍射分析、热失重分析等手段,分析硅藻页岩的化学成分、晶体形态和组成等。
比较 5 种硅藻页岩的调湿性能,提出热失重分析方法也可以用于分辨和筛选高调湿性能的硅藻页岩。
【关键词】 硅藻页岩;硅藻土;X 射线衍射分析;热失重分析;调湿性能;调湿材料 【中图分类号】 TQ630.7+2 【文献标志码】 A 【文章编号】 1671-3702(2021)04-0065-050 引言硅藻土作为一种无机的调湿材料,现在已广泛地应用于室内装饰中[1];但是与硅藻土来源相近的硅藻页岩,目前还不为广大消费者所熟知。
硅藻页岩是由硅藻土在地球内部地核热能的作用下演化而成的矿物。
与硅藻土相比,硅藻页岩的比表面积更大,调湿能力更强[2],是更高端的无机调湿材料。
本文分析不同产地的硅藻页岩的外观、层理和微观形貌;并利用红外光谱分析、X 射线衍射分析;热失重分析等手段表征了硅藻页岩的成分、结构;对比了多种不同产地和不同处理方法的硅藻页岩的调湿性能。
1 原材料收集了产自日本北海道地区和肯尼亚的 2 种硅藻页岩原矿石,粉碎成硅藻页岩粉体(以下简称硅藻页岩),同时还收集了产自中国东北的 3 种硅藻页岩,给样品编号,其产地、颜色、处理方式和白度等信息,如表 1 所示。
作者简介:姜广明,男,高级工程师,研究方向为建筑材料检测与应用。
Comparative Study and Selection of Diatom Shalewith High Humidity Control PerformanceJIANG Guangming 1,MA Haixu 1,XIAO Kaiwei 1,HAN Fangchao 2,CUI Yifan 3,HU Shui 4(1.China Academy of Building Research ,Beijing 100013,China ;2.Shandong Ni Boss New Material Co.,Ltd.,Weifang Shandong 262400,China ;3.Beijing Yuansheng Wanlitong Trading Co.,Ltd.,Beijing 100013,China ;4.Beijing Universityof Chemical Technology ,Beijing 100029,China ) Abstract :Firstly,this article described the appearance and microscopic morphology of five different diatom shale ore and diatom shale powder,and analyzed chemical composition,crystal form and composition,etc. of diatom shale by means of infrared analysis,X-ray diffraction analysis,thermal gravity analysis. The humidity control performance of 5 kinds of diatom shale was compared,and the thermal gravity analysis method could also be used to distinguish and screen diatom shale with high humidity control performance. Keywords :diatom shale;diatomite;X-ray diffraction analysis;thermal gravity analysis;humidity control performance;humidity control material- 66 -2 实验设备SEM 使用热场发射扫描电子显微镜,样品经喷金处理。
“Discovery Studio 2.5中基于药效团的药物设计 方法和应用 ”
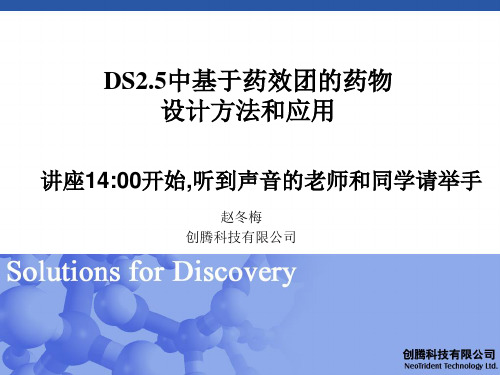
- 化合物数目在 化合物数目在2-32个,6个左右比较理想 个 个左右比较理想 - 只选用具有活性的分子 - 需要包含 需要包含Principal和MaxOmitFeat性质 和 性质 • 方法总结 - 找到一组化合物的公共药效特性 - 不需要 不需要SAR的信息 的信息 - 使用有活性的化合物作为输入结构 - 返回一组公共的药效特性 - 产生的药效团根据打分排序 - 可同时处理大量分子 - 多种不同格式的分子 多种不同格式的分子(*.cpd, *.mol2, *.mmod)
36 Compounds
1150compounds
+ Training set (16) Ligandfit
化合物库筛选
可以构建自己的化合物库
database
安装其他化合物库, 安装其他化合物库,CNPD,ACD等 , 等 DS中自带的化合物库 中自带的化合物库
构建具有活性预测能力的药效团
• • 寻找与化合物活性相关的药效团特性 构建的药效团模型具有活性预测功能 3D-QSAR Pharmacophore Generation HypoGen(quantitative)
2010年其他市场活动 年其他市场活动
– Spotfire Seminar(主题:Spotfire在药物研究中的应用)
• 5月31日上午,北京Seminar • 6月2日上午,上海Seminar • 具体通知请关注我们的网站
筛选 对接 36compounds
1150compounds
模型构建
安捷伦产品目录
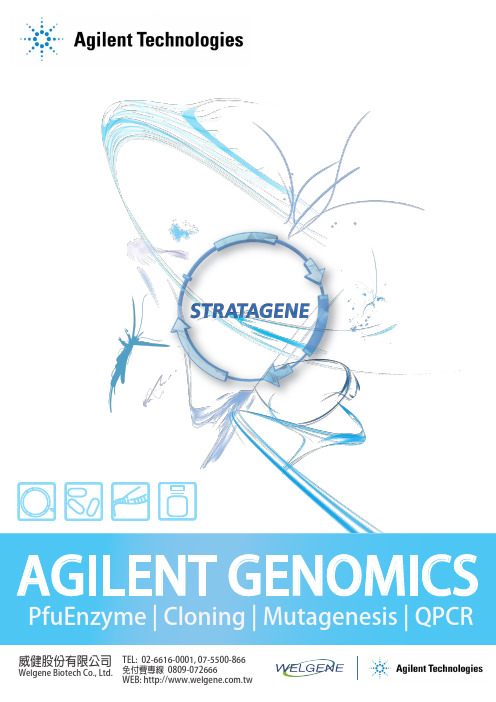
15
Real-Time PCR
16
Mx3000P QPCR System
17
Brilliant III Ultra-Fast SYBR Green QPCR and QRT-PCR Reagents
18
Brilliant III Ultra-Fast QPCR and QRT-PCR Reagents
Agilent / STRATAGENE
Agilent website: /genomics
Welgene | Agilent Stratagene
威健股份有限公司 | Stratagene 總代理
Table of Content
Table of Contents
/ XL1-Red Competent Cells SoloPack Gold Supercompetent Cells
/ TK Competent Cells Specialty Cells
/ Classic Cells / Fine Chemicals For Competent Cells
適用於 UNG 去汙染或 bisulphite
sequencing
適用於 TA Cloning
最高敏感性
取代傳統 Taq 的好選擇
-
2
威健股份有限公司 | Stratagene 總代理
PCR Enzyme & Instrument
Agilent SureCycler 8800
市場上領先的 cycling 速度和 sample 體積 10 ~ 100 μL 簡易快速可以選擇 96 well 和 384 well 操作盤 優秀的溫控設備讓各個 well 都能保持溫度的穩定 七吋的高解析度觸控螢幕讓操作上更為簡便 可以透過網路遠端操控儀器及監控儀器 Agilent 專業的技術支援可以幫助您應對各種 PCR 的問題
- 1、下载文档前请自行甄别文档内容的完整性,平台不提供额外的编辑、内容补充、找答案等附加服务。
- 2、"仅部分预览"的文档,不可在线预览部分如存在完整性等问题,可反馈申请退款(可完整预览的文档不适用该条件!)。
- 3、如文档侵犯您的权益,请联系客服反馈,我们会尽快为您处理(人工客服工作时间:9:00-18:30)。
three electron pairs, trigonal planar
Boron trichloride BCl3
• Group 3, threeσ-bonds, no lone pairs • Bond angle 120°
7
Three bond pairs
• Sulfur trioxide, SO3 • three σ-bonds and three π-bonds, no lone pairs, trigonal planar.
The shape is a triangular-based pyramid The repulsion between the lone pair and the bond pairs is greater than that of bond pairs. This reduces the H-N-H bond angle from 109.5° to 107°.
• The shape is modified by the lone pairs.
• Repulsion of the bond pairs by any lone pairs reduces the bond angle.
4
Species with two electron pairs
• The two electron pairs repel each other to a position of minimum repulsion(maximum separation) with a linear arrangement.
Chapter 8
Shapes of molecules and ions,polarity and intermolecular forces
1
Valence Share Electron Pair Repulsion
The shape of a molecule or ion is caused by repulsion between the pairs of electrons, both bond pairs and lone pairs,that surround the central atom. The electron pairs repel each other to the position of minimum repulsion, which is also the position of maximum separation
9
Speci
• The four electron pairs repel each other to a position of minimum repulsion(maximum separation) with a threedimension tetrahedral arrangement.
4 electron pairs, tetrahedral
5 electron pairs, trigonal bipyramidal
6 electron pairs, Octahedral
15
Polarity of Molecules
• Electrons are not always shared equally between two bonding atoms; one atom might exert more of a force on the electron cloud than the other. • Electronegativity--- The ability of an element to attract the pair of electrons in a covalent bond.
3
Steps to predict the shape
• Work out the number of σ-bonds and lone pairs. This gives the arrangement of electrons.
– The multiple electron pairs in a double bond or triple bond are treated as though they were a single "pair".
2
Valence Share Electron Pair Repulsion
– The multiple electron pairs in a double bond or triple bond are treated as though they were a single "pair". – The repulsion caused by the lone pair is greater than the repulsion caused by the bonding pair. – In other words, the lone pair-lone pair repulsion is considered to be stronger than the lone pair-bonding pair repulsion, which in turn is stronger than the bonding pair-bonding pair repulsion.
8
Two bond pairs and one lone pair
Sulfur dioxide, SO2 two σ-bonds and two πbonds, one lone pair. The three electron pairs are in a trigonal planar arrangement. V-shaped or bent.
six electron pairs, Octahedral Sulfuryl fluoride, SF6
14
Effect of the number of electron pairs around the central atom
2 electron pairs, linear
3 electron pairs, trigonal planar
two electron pairs, linear
Beryllium Chloride, BeCl2
• Group 2, two σ-bonds, no lone pairs • Bond angle 180°
5
• Carbon dioxide. CO2 • O=C=O two σ-bonds and two π-bonds, no lone pairs, linear.
12
Species with five electron pairs
• The position of maximum separation is trigonal bipyramidal. • Group 5, fiveσ-bonds, no lone pairs • The bond angles between the chlorine atoms and phosphorus in the triangular plane are all 120°. • The angle between the top or bottom chlorine and those in the plane is 90°
13
five electron pairs, trigonal bipyramidal Phosphorus pentachloride, PCl5
Species with six electron pairs
• Six electrons pairs repel and take up an octahedral arrangement. • Group 6, sixσ-bonds, no lone pairs • The bond angle 90°
11
Water, H2O Group 6, six valence electrons, twoσ-bonds, 2 lone pairs The four pairs of electrons are arranged tetrahedrally. However, the shape observed is Vshaped. The repulsion between the lone pair and the bond pairs is greater than that of bond pairs. This reduces the H-N-H bond angle from 109.5° to 104.5°.
17
Electronegativity of elements in the periodic table