On detection of emerging anomalous traffic patterns using GPS data
人工智能英文参考文献(最新120个)
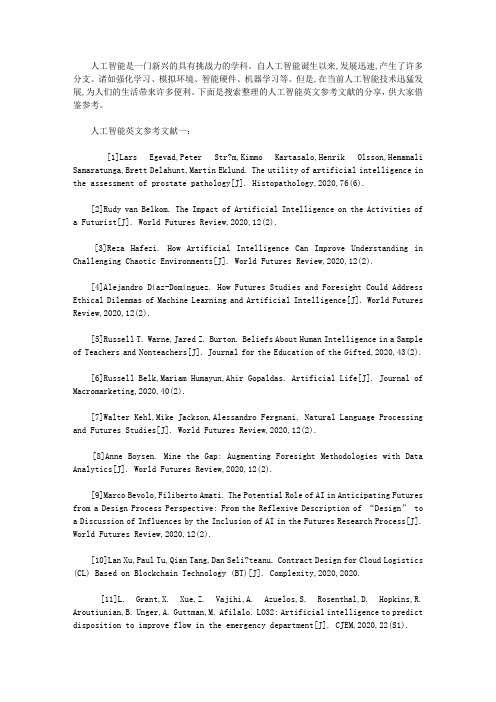
人工智能是一门新兴的具有挑战力的学科。
自人工智能诞生以来,发展迅速,产生了许多分支。
诸如强化学习、模拟环境、智能硬件、机器学习等。
但是,在当前人工智能技术迅猛发展,为人们的生活带来许多便利。
下面是搜索整理的人工智能英文参考文献的分享,供大家借鉴参考。
人工智能英文参考文献一:[1]Lars Egevad,Peter Str?m,Kimmo Kartasalo,Henrik Olsson,Hemamali Samaratunga,Brett Delahunt,Martin Eklund. The utility of artificial intelligence in the assessment of prostate pathology[J]. Histopathology,2020,76(6).[2]Rudy van Belkom. The Impact of Artificial Intelligence on the Activities ofa Futurist[J]. World Futures Review,2020,12(2).[3]Reza Hafezi. How Artificial Intelligence Can Improve Understanding in Challenging Chaotic Environments[J]. World Futures Review,2020,12(2).[4]Alejandro Díaz-Domínguez. How Futures Studies and Foresight Could Address Ethical Dilemmas of Machine Learning and Artificial Intelligence[J]. World Futures Review,2020,12(2).[5]Russell T. Warne,Jared Z. Burton. Beliefs About Human Intelligence in a Sample of Teachers and Nonteachers[J]. Journal for the Education of the Gifted,2020,43(2).[6]Russell Belk,Mariam Humayun,Ahir Gopaldas. Artificial Life[J]. Journal of Macromarketing,2020,40(2).[7]Walter Kehl,Mike Jackson,Alessandro Fergnani. Natural Language Processing and Futures Studies[J]. World Futures Review,2020,12(2).[8]Anne Boysen. Mine the Gap: Augmenting Foresight Methodologies with Data Analytics[J]. World Futures Review,2020,12(2).[9]Marco Bevolo,Filiberto Amati. The Potential Role of AI in Anticipating Futures from a Design Process Perspective: From the Reflexive Description of “Design” to a Discussion of Influences by the Inclusion of AI in the Futures Research Process[J]. World Futures Review,2020,12(2).[10]Lan Xu,Paul Tu,Qian Tang,Dan Seli?teanu. Contract Design for Cloud Logistics (CL) Based on Blockchain Technology (BT)[J]. Complexity,2020,2020.[11]L. Grant,X. Xue,Z. Vajihi,A. Azuelos,S. Rosenthal,D. Hopkins,R. Aroutiunian,B. Unger,A. Guttman,M. Afilalo. LO32: Artificial intelligence to predict disposition to improve flow in the emergency department[J]. CJEM,2020,22(S1).[12]A. Kirubarajan,A. Taher,S. Khan,S. Masood. P071: Artificial intelligence in emergency medicine: A scoping review[J]. CJEM,2020,22(S1).[13]L. Grant,P. Joo,B. Eng,A. Carrington,M. Nemnom,V. Thiruganasambandamoorthy. LO22: Risk-stratification of emergency department syncope by artificial intelligence using machine learning: human, statistics or machine[J]. CJEM,2020,22(S1).[14]Riva Giuseppe,Riva Eleonora. OS for Ind Robots: Manufacturing Robots Get Smarter Thanks to Artificial Intelligence.[J]. Cyberpsychology, behavior and social networking,2020,23(5).[15]Markus M. Obmann,Aurelio Cosentino,Joshy Cyriac,Verena Hofmann,Bram Stieltjes,Daniel T. Boll,Benjamin M. Yeh,Matthias R. Benz. Quantitative enhancement thresholds and machine learning algorithms for the evaluation of renal lesions using single-phase split-filter dual-energy CT[J]. Abdominal Radiology,2020,45(1).[16]Haytham H. Elmousalami,Mahmoud Elaskary. Drilling stuck pipe classification and mitigation in the Gulf of Suez oil fields using artificial intelligence[J]. Journal of Petroleum Exploration and Production Technology,2020,10(10).[17]Rüdiger Schulz-Wendtland,Karin Bock. Bildgebung in der Mammadiagnostik –Ein Ausblick <trans-title xml:lang="en">Imaging in breast diagnostics—an outlook [J]. Der Gyn?kologe,2020,53(6).</trans-title>[18]Nowakowski Piotr,Szwarc Krzysztof,Boryczka Urszula. Combining an artificial intelligence algorithm and a novel vehicle for sustainable e-waste collection[J]. Science of the Total Environment,2020,730.[19]Wang Huaizhi,Liu Yangyang,Zhou Bin,Li Canbing,Cao Guangzhong,Voropai Nikolai,Barakhtenko Evgeny. Taxonomy research of artificial intelligence for deterministic solar power forecasting[J]. Energy Conversion and Management,2020,214.[20]Kagemoto Hiroshi. Forecasting a water-surface wave train with artificial intelligence- A case study[J]. Ocean Engineering,2020,207.[21]Tomonori Aoki,Atsuo Yamada,Kazuharu Aoyama,Hiroaki Saito,Gota Fujisawa,Nariaki Odawara,Ryo Kondo,Akiyoshi Tsuboi,Rei Ishibashi,Ayako Nakada,Ryota Niikura,Mitsuhiro Fujishiro,Shiro Oka,Soichiro Ishihara,Tomoki Matsuda,Masato Nakahori,Shinji Tanaka,Kazuhiko Koike,Tomohiro Tada. Clinical usefulness of a deep learning‐based system as the first screening on small‐bowel capsule endoscopy reading[J]. Digestive Endoscopy,2020,32(4).[22]Masashi Fujii,Hajime Isomoto. Next generation of endoscopy: Harmony with artificial intelligence and robotic‐assisted devices[J]. Digestive Endoscopy,2020,32(4).[23]Roberto Verganti,Luca Vendraminelli,Marco Iansiti. Innovation and Design in the Age of Artificial Intelligence[J]. Journal of Product Innovation Management,2020,37(3).[24]Yuval Elbaz,David Furman,Maytal Caspary Toroker. Modeling Diffusion in Functional Materials: From Density Functional Theory to Artificial Intelligence[J]. Advanced Functional Materials,2020,30(18).[25]Dinesh Visva Gunasekeran,Tien Yin Wong. Artificial Intelligence in Ophthalmology in 2020: A Technology on the Cusp for Translation and Implementation[J]. Asia-Pacific Journal of Ophthalmology,2020,9(2).[26]Fu-Neng Jiang,Li-Jun Dai,Yong-Ding Wu,Sheng-Bang Yang,Yu-Xiang Liang,Xin Zhang,Cui-Yun Zou,Ren-Qiang He,Xiao-Ming Xu,Wei-De Zhong. The study of multiple diagnosis models of human prostate cancer based on Taylor database by artificial neural networks[J]. Journal of the Chinese Medical Association,2020,83(5).[27]Matheus Calil Faleiros,Marcello Henrique Nogueira-Barbosa,Vitor Faeda Dalto,JoséRaniery Ferreira Júnior,Ariane Priscilla Magalh?es Tenório,Rodrigo Luppino-Assad,Paulo Louzada-Junior,Rangaraj Mandayam Rangayyan,Paulo Mazzoncini de Azevedo-Marques. Machine learning techniques for computer-aided classification of active inflammatory sacroiliitis in magnetic resonance imaging[J]. Advances in Rheumatology,2020,60(1078).[28]Balamurugan Balakreshnan,Grant Richards,Gaurav Nanda,Huachao Mao,Ragu Athinarayanan,Joseph Zaccaria. PPE Compliance Detection using Artificial Intelligence in Learning Factories[J]. Procedia Manufacturing,2020,45.[29]M. Stévenin,V. Avisse,N. Ducarme,A. de Broca. Qui est responsable si un robot autonome vient à entra?ner un dommage ?[J]. Ethique et Santé,2020.[30]Fatemeh Barzegari Banadkooki,Mohammad Ehteram,Fatemeh Panahi,Saad Sh. Sammen,Faridah Binti Othman,Ahmed EL-Shafie. Estimation of Total Dissolved Solids (TDS) using New Hybrid Machine Learning Models[J]. Journal of Hydrology,2020.[31]Adam J. Schwartz,Henry D. Clarke,Mark J. Spangehl,Joshua S. Bingham,DavidA. Etzioni,Matthew R. Neville. Can a Convolutional Neural Network Classify Knee Osteoarthritis on Plain Radiographs as Accurately as Fellowship-Trained Knee Arthroplasty Surgeons?[J]. The Journal of Arthroplasty,2020.[32]Ivana Nizetic Kosovic,Toni Mastelic,Damir Ivankovic. Using Artificial Intelligence on environmental data from Internet of Things for estimating solar radiation: Comprehensive analysis[J]. Journal of Cleaner Production,2020.[33]Lauren Fried,Andrea Tan,Shirin Bajaj,Tracey N. Liebman,David Polsky,Jennifer A. Stein. Technological advances for the detection of melanoma: Part I. Advances in diagnostic techniques[J]. Journal of the American Academy of Dermatology,2020.[34]Mohammed Amoon,Torki Altameem,Ayman Altameem. Internet of things Sensor Assisted Security and Quality Analysis for Health Care Data Sets Using Artificial Intelligent Based Heuristic Health Management System[J]. Measurement,2020.[35]E. Lotan,C. Tschider,D.K. Sodickson,A. Caplan,M. Bruno,B. Zhang,Yvonne W. Lui. Medical Imaging and Privacy in the Era of Artificial Intelligence: Myth, Fallacy, and the Future[J]. Journal of the American College of Radiology,2020.[36]Fabien Lareyre,Cédric Adam,Marion Carrier,Juliette Raffort. Artificial Intelligence in Vascular Surgery: moving from Big Data to Smart Data[J]. Annals of Vascular Surgery,2020.[37]Ilesanmi Daniyan,Khumbulani Mpofu,Moses Oyesola,Boitumelo Ramatsetse,Adefemi Adeodu. Artificial intelligence for predictive maintenance in the railcar learning factories[J]. Procedia Manufacturing,2020,45.[38]Janet L. McCauley,Anthony E. Swartz. Reframing Telehealth[J]. Obstetrics and Gynecology Clinics of North America,2020.[39]Jean-Emmanuel Bibault,Lei Xing. Screening for chronic obstructive pulmonary disease with artificial intelligence[J]. The Lancet Digital Health,2020,2(5).[40]Andrea Laghi. Cautions about radiologic diagnosis of COVID-19 infection driven by artificial intelligence[J]. The Lancet Digital Health,2020,2(5).人工智能英文参考文献二:[41]K. Orhan,I. S. Bayrakdar,M. Ezhov,A. Kravtsov,T. ?zyürek. Evaluation of artificial intelligence for detecting periapical pathosis on cone‐beam computed tomography scans[J]. International Endodontic Journal,2020,53(5).[42]Avila A M,Mezi? I. Data-driven analysis and forecasting of highway traffic dynamics.[J]. Nature communications,2020,11(1).[43]Neri Emanuele,Miele Vittorio,Coppola Francesca,Grassi Roberto. Use of CT andartificial intelligence in suspected or COVID-19 positive patients: statement of the Italian Society of Medical and Interventional Radiology.[J]. La Radiologia medica,2020.[44]Tau Noam,Stundzia Audrius,Yasufuku Kazuhiro,Hussey Douglas,Metser Ur. Convolutional Neural Networks in Predicting Nodal and Distant Metastatic Potential of Newly Diagnosed Non-Small Cell Lung Cancer on FDG PET Images.[J]. AJR. American journal of roentgenology,2020.[45]Coppola Francesca,Faggioni Lorenzo,Regge Daniele,Giovagnoni Andrea,Golfieri Rita,Bibbolino Corrado,Miele Vittorio,Neri Emanuele,Grassi Roberto. Artificial intelligence: radiologists' expectations and opinions gleaned from a nationwide online survey.[J]. La Radiologia medica,2020.[46]?. ? ? ? ? [J]. ,2020,25(4).[47]Savage Rock H,van Assen Marly,Martin Simon S,Sahbaee Pooyan,Griffith Lewis P,Giovagnoli Dante,Sperl Jonathan I,Hopfgartner Christian,K?rgel Rainer,Schoepf U Joseph. Utilizing Artificial Intelligence to Determine Bone Mineral Density Via Chest Computed Tomography.[J]. Journal of thoracic imaging,2020,35 Suppl 1.[48]Brzezicki Maksymilian A,Bridger Nicholas E,Kobeti? Matthew D,Ostrowski Maciej,Grabowski Waldemar,Gill Simran S,Neumann Sandra. Artificial intelligence outperforms human students in conducting neurosurgical audits.[J]. Clinical neurology and neurosurgery,2020,192.[49]Lockhart Mark E,Smith Andrew D. Fatty Liver Disease: Artificial Intelligence Takes on the Challenge.[J]. Radiology,2020,295(2).[50]Wood Edward H,Korot Edward,Storey Philip P,Muscat Stephanie,Williams George A,Drenser Kimberly A. The retina revolution: signaling pathway therapies, genetic therapies, mitochondrial therapies, artificial intelligence.[J]. Current opinion in ophthalmology,2020,31(3).[51]Ho Dean,Quake Stephen R,McCabe Edward R B,Chng Wee Joo,Chow Edward K,Ding Xianting,Gelb Bruce D,Ginsburg Geoffrey S,Hassenstab Jason,Ho Chih-Ming,Mobley William C,Nolan Garry P,Rosen Steven T,Tan Patrick,Yen Yun,Zarrinpar Ali. Enabling Technologies for Personalized and Precision Medicine.[J]. Trends in biotechnology,2020,38(5).[52]Fischer Andreas M,Varga-Szemes Akos,van Assen Marly,Griffith L Parkwood,Sahbaee Pooyan,Sperl Jonathan I,Nance John W,Schoepf U Joseph. Comparison of Artificial Intelligence-Based Fully Automatic Chest CT Emphysema Quantification to Pulmonary Function Testing.[J]. AJR. American journal ofroentgenology,2020,214(5).[53]Moore William,Ko Jane,Gozansky Elliott. Artificial Intelligence Pertaining to Cardiothoracic Imaging and Patient Care: Beyond Image Interpretation.[J]. Journal of thoracic imaging,2020,35(3).[54]Hwang Eui Jin,Park Chang Min. Clinical Implementation of Deep Learning in Thoracic Radiology: Potential Applications and Challenges.[J]. Korean journal of radiology,2020,21(5).[55]Mateen Bilal A,David Anna L,Denaxas Spiros. Electronic Health Records to Predict Gestational Diabetes Risk.[J]. Trends in pharmacological sciences,2020,41(5).[56]Yao Xiang,Mao Ling,Lv Shunli,Ren Zhenghong,Li Wentao,Ren Ke. CT radiomics features as a diagnostic tool for classifying basal ganglia infarction onset time.[J]. Journal of the neurological sciences,2020,412.[57]van Assen Marly,Banerjee Imon,De Cecco Carlo N. Beyond the Artificial Intelligence Hype: What Lies Behind the Algorithms and What We Can Achieve.[J]. Journal of thoracic imaging,2020,35 Suppl 1.[58]Guzik Tomasz J,Fuster Valentin. Leaders in Cardiovascular Research: Valentin Fuster.[J]. Cardiovascular research,2020,116(6).[59]Fischer Andreas M,Eid Marwen,De Cecco Carlo N,Gulsun Mehmet A,van Assen Marly,Nance John W,Sahbaee Pooyan,De Santis Domenico,Bauer Maximilian J,Jacobs Brian E,Varga-Szemes Akos,Kabakus Ismail M,Sharma Puneet,Jackson Logan J,Schoepf U Joseph. Accuracy of an Artificial Intelligence Deep Learning Algorithm Implementing a Recurrent Neural Network With Long Short-term Memory for the Automated Detection of Calcified Plaques From Coronary Computed Tomography Angiography.[J]. Journal of thoracic imaging,2020,35 Suppl 1.[60]Ghosh Adarsh,Kandasamy Devasenathipathy. Interpretable Artificial Intelligence: Why and When.[J]. AJR. American journal of roentgenology,2020,214(5).[61]M.Rosario González-Rodríguez,M.Carmen Díaz-Fernández,Carmen Pacheco Gómez. Facial-expression recognition: An emergent approach to the measurement of tourist satisfaction through emotions[J]. Telematics and Informatics,2020,51.[62]Ru-Xi Ding,Iván Palomares,Xueqing Wang,Guo-Rui Yang,Bingsheng Liu,Yucheng Dong,Enrique Herrera-Viedma,Francisco Herrera. Large-Scale decision-making: Characterization, taxonomy, challenges and future directions from an Artificial Intelligence and applications perspective[J]. Information Fusion,2020,59.[63]Abdulrhman H. Al-Jebrni,Brendan Chwyl,Xiao Yu Wang,Alexander Wong,Bechara J. Saab. AI-enabled remote and objective quantification of stress at scale[J]. Biomedical Signal Processing and Control,2020,59.[64]Gillian Thomas,Elizabeth Eisenhauer,Robert G. Bristow,Cai Grau,Coen Hurkmans,Piet Ost,Matthias Guckenberger,Eric Deutsch,Denis Lacombe,Damien C. Weber. The European Organisation for Research and Treatment of Cancer, State of Science in radiation oncology and priorities for clinical trials meeting report[J]. European Journal of Cancer,2020,131.[65]Muhammad Asif. Are QM models aligned with Industry 4.0? A perspective on current practices[J]. Journal of Cleaner Production,2020,258.[66]Siva Teja Kakileti,Himanshu J. Madhu,Geetha Manjunath,Leonard Wee,Andre Dekker,Sudhakar Sampangi. Personalized risk prediction for breast cancer pre-screening using artificial intelligence and thermal radiomics[J]. Artificial Intelligence In Medicine,2020,105.[67]. Evaluation of Payer Budget Impact Associated with the Use of Artificial Intelligence in Vitro Diagnostic, Kidneyintelx, to Modify DKD Progression:[J]. American Journal of Kidney Diseases,2020,75(5).[68]Rohit Nishant,Mike Kennedy,Jacqueline Corbett. Artificial intelligence for sustainability: Challenges, opportunities, and a research agenda[J]. International Journal of Information Management,2020,53.[69]Hoang Nguyen,Xuan-Nam Bui. Soft computing models for predicting blast-induced air over-pressure: A novel artificial intelligence approach[J]. Applied Soft Computing Journal,2020,92.[70]Benjamin S. Hopkins,Aditya Mazmudar,Conor Driscoll,Mark Svet,Jack Goergen,Max Kelsten,Nathan A. Shlobin,Kartik Kesavabhotla,Zachary A Smith,Nader S Dahdaleh. Using artificial intelligence (AI) to predict postoperative surgical site infection: A retrospective cohort of 4046 posterior spinal fusions[J]. Clinical Neurology and Neurosurgery,2020,192.[71]Mei Yang,Runze Zhou,Xiangjun Qiu,Xiangfei Feng,Jian Sun,Qunshan Wang,Qiufen Lu,Pengpai Zhang,Bo Liu,Wei Li,Mu Chen,Yan Zhao,Binfeng Mo,Xin Zhou,Xi Zhang,Yingxue Hua,Jin Guo,Fangfang Bi,Yajun Cao,Feng Ling,Shengming Shi,Yi-Gang Li. Artificial intelligence-assisted analysis on the association between exposure to ambient fine particulate matter and incidence of arrhythmias in outpatients of Shanghai community hospitals[J]. Environment International,2020,139.[72]Fatemehalsadat Madaeni,Rachid Lhissou,Karem Chokmani,Sebastien Raymond,Yves Gauthier. Ice jam formation, breakup and prediction methods based on hydroclimatic data using artificial intelligence: A review[J]. Cold Regions Science and Technology,2020,174.[73]Steve Chukwuebuka Arum,David Grace,Paul Daniel Mitchell. A review of wireless communication using high-altitude platforms for extended coverage and capacity[J]. Computer Communications,2020,157.[74]Yong-Hong Kuo,Nicholas B. Chan,Janny M.Y. Leung,Helen Meng,Anthony Man-Cho So,Kelvin K.F. Tsoi,Colin A. Graham. An Integrated Approach of Machine Learning and Systems Thinking for Waiting Time Prediction in an Emergency Department[J]. International Journal of Medical Informatics,2020,139.[75]Matteo Terzi,Gian Antonio Susto,Pratik Chaudhari. Directional adversarial training for cost sensitive deep learning classification applications[J]. Engineering Applications of Artificial Intelligence,2020,91.[76]Arman Kilic. Artificial Intelligence and Machine Learning in Cardiovascular Health Care[J]. The Annals of Thoracic Surgery,2020,109(5).[77]Hossein Azarmdel,Ahmad Jahanbakhshi,Seyed Saeid Mohtasebi,Alfredo Rosado Mu?oz. Evaluation of image processing technique as an expert system in mulberry fruit grading based on ripeness level using artificial neural networks (ANNs) and support vector machine (SVM)[J]. Postharvest Biology and Technology,2020,166.[78]Wafaa Wardah,Abdollah Dehzangi,Ghazaleh Taherzadeh,Mahmood A. Rashid,M.G.M. Khan,Tatsuhiko Tsunoda,Alok Sharma. Predicting protein-peptide binding sites with a deep convolutional neural network[J]. Journal of Theoretical Biology,2020,496.[79]Francisco F.X. Vasconcelos,Róger M. Sarmento,Pedro P. Rebou?as Filho,Victor Hugo C. de Albuquerque. Artificial intelligence techniques empowered edge-cloud architecture for brain CT image analysis[J]. Engineering Applications of Artificial Intelligence,2020,91.[80]Masaaki Konishi. Bioethanol production estimated from volatile compositions in hydrolysates of lignocellulosic biomass by deep learning[J]. Journal of Bioscience and Bioengineering,2020,129(6).人工智能英文参考文献三:[81]J. Kwon,K. Kim. Artificial Intelligence for Early Prediction of Pulmonary Hypertension Using Electrocardiography[J]. Journal of Heart and Lung Transplantation,2020,39(4).[82]C. Maathuis,W. Pieters,J. van den Berg. Decision support model for effects estimation and proportionality assessment for targeting in cyber operations[J]. Defence Technology,2020.[83]Samer Ellahham. Artificial Intelligence in Diabetes Care[J]. The American Journal of Medicine,2020.[84]Yi-Ting Hsieh,Lee-Ming Chuang,Yi-Der Jiang,Tien-Jyun Chang,Chung-May Yang,Chang-Hao Yang,Li-Wei Chan,Tzu-Yun Kao,Ta-Ching Chen,Hsuan-Chieh Lin,Chin-Han Tsai,Mingke Chen. Application of deep learning image assessment software VeriSee? for diabetic retinopathy screening[J]. Journal of the Formosan Medical Association,2020.[85]Emre ARTUN,Burak KULGA. Selection of candidate wells for re-fracturing in tight gas sand reservoirs using fuzzy inference[J]. Petroleum Exploration and Development Online,2020,47(2).[86]Alberto Arenal,Cristina Armu?a,Claudio Feijoo,Sergio Ramos,Zimu Xu,Ana Moreno. Innovation ecosystems theory revisited: The case of artificial intelligence in China[J]. Telecommunications Policy,2020.[87]T. Som,M. Dwivedi,C. Dubey,A. Sharma. Parametric Studies on Artificial Intelligence Techniques for Battery SOC Management and Optimization of Renewable Power[J]. Procedia Computer Science,2020,167.[88]Bushra Kidwai,Nadesh RK. Design and Development of Diagnostic Chabot for supporting Primary Health Care Systems[J]. Procedia Computer Science,2020,167.[89]Asl? Bozda?,Ye?im Dokuz,?znur Begüm G?k?ek. Spatial prediction of PM 10 concentration using machine learning algorithms in Ankara, Turkey[J]. Environmental Pollution,2020.[90]K.P. Smith,J.E. Kirby. Image analysis and artificial intelligence in infectious disease diagnostics[J]. Clinical Microbiology and Infection,2020.[91]Alklih Mohamad YOUSEF,Ghahfarokhi Payam KAVOUSI,Marwan ALNUAIMI,Yara ALATRACH. Predictive data analytics application for enhanced oil recovery in a mature field in the Middle East[J]. Petroleum Exploration and Development Online,2020,47(2).[92]Omer F. Ahmad,Danail Stoyanov,Laurence B. Lovat. Barriers and pitfalls for artificial intelligence in gastroenterology: Ethical and regulatory issues[J]. Techniques and Innovations in Gastrointestinal Endoscopy,2020,22(2).[93]Sanne A. Hoogenboom,Ulas Bagci,Michael B. Wallace. Artificial intelligence in gastroenterology. The current state of play and the potential. How will it affect our practice and when?[J]. Techniques and Innovations in Gastrointestinal Endoscopy,2020,22(2).[94]Douglas K. Rex. Can we do resect and discard with artificial intelligence-assisted colon polyp “optical biopsy?”[J]. Techniques and Innovations in Gastrointestinal Endoscopy,2020,22(2).[95]Neal Shahidi,Michael J. Bourke. Can artificial intelligence accurately diagnose endoscopically curable gastrointestinal cancers?[J]. Techniques and Innovations in Gastrointestinal Endoscopy,2020,22(2).[96]Michael Byrne. Artificial intelligence in gastroenterology[J]. Techniques and Innovations in Gastrointestinal Endoscopy,2020,22(2).[97]Piet C. de Groen. Using artificial intelligence to improve adequacy of inspection in gastrointestinal endoscopy[J]. Techniques and Innovations in Gastrointestinal Endoscopy,2020,22(2).[98]Robin Zachariah,Andrew Ninh,William Karnes. Artificial intelligence for colon polyp detection: Why should we embrace this?[J]. Techniques and Innovations in Gastrointestinal Endoscopy,2020,22(2).[99]Alexandra T. Greenhill,Bethany R. Edmunds. A primer of artificial intelligence in medicine[J]. Techniques and Innovations in Gastrointestinal Endoscopy,2020,22(2).[100]Tomohiro Tada,Toshiaki Hirasawa,Toshiyuki Yoshio. The role for artificial intelligence in evaluation of upper GI cancer[J]. Techniques and Innovations in Gastrointestinal Endoscopy,2020,22(2).[101]Yahui Jiang,Meng Yang,Shuhao Wang,Xiangchun Li,Yan Sun. Emerging role of deep learning‐based artificial intelligence in tumor pathology[J]. Cancer Communications,2020,40(4).[102]Kristopher D. Knott,Andreas Seraphim,Joao B. Augusto,Hui Xue,Liza Chacko,Nay Aung,Steffen E. Petersen,Jackie A. Cooper,Charlotte Manisty,Anish N. Bhuva,Tushar Kotecha,Christos V. Bourantas,Rhodri H. Davies,Louise A.E. Brown,Sven Plein,Marianna Fontana,Peter Kellman,James C. Moon. The Prognostic Significance of Quantitative Myocardial Perfusion: An Artificial Intelligence–Based Approach Using Perfusion Mapping[J]. Circulation,2020,141(16).[103]Muhammad Asad,Ahmed Moustafa,Takayuki Ito. FedOpt: Towards Communication Efficiency and Privacy Preservation in Federated Learning[J]. Applied Sciences,2020,10(8).[104]Wu Wenzhi,Zhang Yan,Wang Pu,Zhang Li,Wang Guixiang,Lei Guanghui,Xiao Qiang,Cao Xiaochen,Bian Yueran,Xie Simiao,Huang Fei,Luo Na,Zhang Jingyuan,Luo Mingyan. Psychological stress of medical staffs during outbreak of COVID-19 and adjustment strategy.[J]. Journal of medical virology,2020.[105]. Eyenuk Fulfills Contract for Artificial Intelligence Grading of Retinal Images[J]. Telecomworldwire,2020.[106]Kim Tae Woo,Duhachek Adam. Artificial Intelligence and Persuasion: A Construal-Level Account.[J]. Psychological science,2020,31(4).[107]McCall Becky. COVID-19 and artificial intelligence: protecting health-care workers and curbing the spread.[J]. The Lancet. Digital health,2020,2(4).[108]Alca?iz Mariano,Chicchi Giglioli Irene A,Sirera Marian,Minissi Eleonora,Abad Luis. [Autism spectrum disorder biomarkers based on biosignals, virtual reality and artificial intelligence].[J]. Medicina,2020,80 Suppl 2.[109]Cong Lei,Feng Wanbing,Yao Zhigang,Zhou Xiaoming,Xiao Wei. Deep Learning Model as a New Trend in Computer-aided Diagnosis of Tumor Pathology for Lung Cancer.[J]. Journal of Cancer,2020,11(12).[110]Wang Fengdan,Gu Xiao,Chen Shi,Liu Yongliang,Shen Qing,Pan Hui,Shi Lei,Jin Zhengyu. Artificial intelligence system can achieve comparable results to experts for bone age assessment of Chinese children with abnormal growth and development.[J]. PeerJ,2020,8.[111]Hu Wenmo,Yang Huayu,Xu Haifeng,Mao Yilei. Radiomics based on artificial intelligence in liver diseases: where we are?[J]. Gastroenterology report,2020,8(2).[112]Batayneh Wafa,Abdulhay Enas,Alothman Mohammad. Prediction of the performance of artificial neural networks in mapping sEMG to finger joint angles via signal pre-investigation techniques.[J]. Heliyon,2020,6(4).[113]Aydin Emrah,Türkmen ?nan Utku,Namli G?zde,?ztürk ?i?dem,Esen Ay?e B,Eray Y Nur,Ero?lu Egemen,Akova Fatih. A novel and simple machine learning algorithm for preoperative diagnosis of acute appendicitis in children.[J]. Pediatric surgery international,2020.[114]Ellahham Samer. Artificial Intelligence in Diabetes Care.[J]. The Americanjournal of medicine,2020.[115]David J. Winkel,Thomas J. Weikert,Hanns-Christian Breit,Guillaume Chabin,Eli Gibson,Tobias J. Heye,Dorin Comaniciu,Daniel T. Boll. Validation of a fully automated liver segmentation algorithm using multi-scale deep reinforcement learning and comparison versus manual segmentation[J]. European Journal of Radiology,2020,126.[116]Binjie Fu,Guoshu Wang,Mingyue Wu,Wangjia Li,Yineng Zheng,Zhigang Chu,Fajin Lv. Influence of CT effective dose and convolution kernel on the detection of pulmonary nodules in different artificial intelligence software systems: A phantom study[J]. European Journal of Radiology,2020,126.[117]Georgios N. Kouziokas. A new W-SVM kernel combining PSO-neural network transformed vector and Bayesian optimized SVM in GDP forecasting[J]. Engineering Applications of Artificial Intelligence,2020,92.[118]Qingsong Ruan,Zilin Wang,Yaping Zhou,Dayong Lv. A new investor sentiment indicator ( ISI ) based on artificial intelligence: A powerful return predictor in China[J]. Economic Modelling,2020,88.[119]Mohamed Abdel-Basset,Weiping Ding,Laila Abdel-Fatah. The fusion of Internet of Intelligent Things (IoIT) in remote diagnosis of obstructive Sleep Apnea: A survey and a new model[J]. Information Fusion,2020,61.[120]Federico Caobelli. Artificial intelligence in medical imaging: Game over for radiologists?[J]. European Journal of Radiology,2020,126.以上就是关于人工智能参考文献的分享,希望对你有所帮助。
特殊天气条件下的目标检测方法综述

特殊天气条件下的目标检测方法综述特殊天气条件下的目标检测方法综述导言:目标检测是计算机视觉领域中的一项重要任务,它能够识别图像或视频中的特定物体,并将其与背景区分开来。
然而,特殊天气条件如雨雪、霾等可以严重影响图像质量,进而影响目标检测的效果。
针对特殊天气条件下的目标检测问题,研究人员提出了一系列创新的方法,本文将对这些方法进行综述,以便读者了解在特殊天气条件下如何提高目标检测的准确性和稳定性。
一、雨天目标检测方法雨天的图像中存在大量的雨滴杂乱背景,会对目标的边缘、颜色特征产生很大的干扰。
为了解决这个问题,研究人员提出了一些方法。
其中,一种常用的方法是利用双重密度图模型来检测雨滴,然后在目标上使用雨滴去除算法。
另一种方法则是基于暗通道先验原理,通过对雨滴的暗通道进行建模,进而去除雨滴的干扰。
此外,还有一些基于深度学习的方法,通过利用深度神经网络对雨天图像进行训练,从而消除雨滴的影响。
二、雪天目标检测方法雪天的图像中存在大量的雪花,且背景一片白茫茫,这会导致目标的边缘和细节信息丢失。
为了解决这个问题,研究人员提出了一些方法。
其中,一种常用的方法是通过增强对比度来提高雪天图像的清晰度。
另一种方法则是使用流形学习的方法,将雪花目标与背景进行分离。
此外,还有一些基于波段变换的方法,通过将图像从RGB空间转换到其他颜色空间,从而提高目标的可辨识度。
三、霾天目标检测方法霾天的图像中存在大量的灰尘和污染物,会导致图像细节模糊不清,进而影响目标检测的准确性。
为了解决这个问题,研究人员提出了一些方法。
其中,一种常用的方法是通过增强图像的对比度和清晰度,将目标从背景中分离出来。
另一种方法则是基于全局分割技术,通过对霾天图像进行分割,提取目标的特征信息。
此外,还有一些基于深度学习的方法,通过对霾天图像进行训练,提高目标检测的准确性。
结论:特殊天气条件下的目标检测是计算机视觉领域的一项重要研究课题。
本文对特殊天气条件下的目标检测方法进行了综述,并介绍了在雨天、雪天和霾天等特殊天气条件下的目标检测方法。
克隆的缺点 英语作文

Cloning,a scientific process that has been the subject of much debate,presents a myriad of potential drawbacks that are often overlooked in the face of its potential benefits.Here are some of the key disadvantages associated with cloning:1.Ethical Concerns:Cloning raises significant ethical issues.The process essentially involves creating a genetic copy of an individual,which can be seen as a violation of the sanctity of life and the uniqueness of each human being.2.Genetic Diversity:One of the fundamental principles of natural selection is the importance of genetic diversity for the survival and adaptation of species.Cloning could lead to a reduction in genetic diversity,making populations more susceptible to diseases and less adaptable to environmental changes.3.Health Risks:Cloned animals have shown a higher incidence of health problems and abnormalities.This could be due to the cloning process itself,which may introduce errors or mutations in the genetic material.If applied to humans,these health risks could be significant and potentially lifethreatening.4.Psychological Impact:The psychological effects of being a clone are not well understood,but it is likely that clones would face unique challenges in terms of identity and selfperception.They may struggle with the knowledge that they are not unique and could experience a sense of depersonalization or loss of individuality.5.Social Implications:Society may struggle to integrate clones,leading to potential discrimination or social ostracism.The concept of family and lineage could be fundamentally altered,with implications for inheritance,social roles,and interpersonal relationships.6.Economic and Legal Issues:The commercialization of cloning could lead to a market for human clones,with serious implications for human rights and dignity.There are also concerns about the legal status of clones,including their rights and responsibilities.7.Potential for Abuse:Cloning technology could be misused for nefarious purposes,such as creating a workforce of clones or for military applications.This could lead to a devaluation of human life and an increase in exploitation.8.Environmental Impact:The mass production of clones could have unforeseen environmental consequences,particularly if clones are used for agricultural or industrial purposes.The ecological balance could be disrupted,leading to unforeseen consequences for other species and ecosystems.9.Moral and Religious Objections:Many religious and philosophical traditions oppose cloning on moral grounds,arguing that it is an affront to the natural order and to the divine.This opposition can create societal divisions and conflicts.10.Unpredictability:The longterm effects of cloning are not fully known,and there is a risk that unforeseen consequences could arise as clones age and interact with the world in ways that are different from naturally conceived individuals.In conclusion,while cloning may offer some potential benefits,such as the ability to reproduce endangered species or to provide organs for transplantation,the risks and drawbacks are significant and must be carefully considered.The potential for harm to individuals,societies,and the environment must be weighed against the potential benefits, and a cautious approach is warranted.。
我们如何防止诈骗英语作文
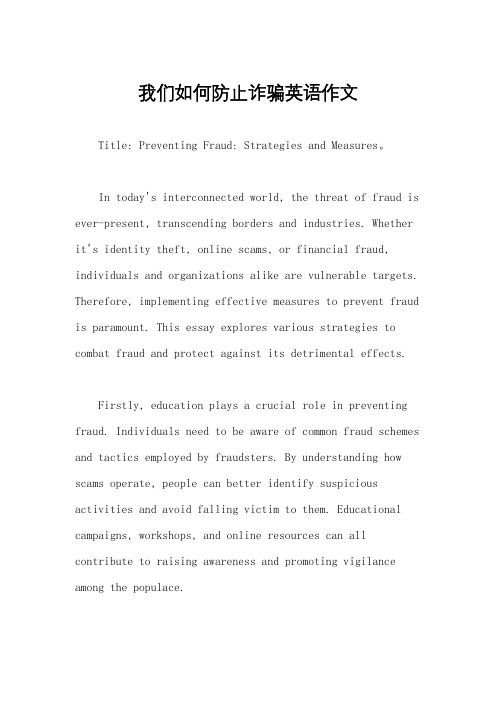
我们如何防止诈骗英语作文Title: Preventing Fraud: Strategies and Measures。
In today's interconnected world, the threat of fraud is ever-present, transcending borders and industries. Whether it's identity theft, online scams, or financial fraud, individuals and organizations alike are vulnerable targets. Therefore, implementing effective measures to prevent fraud is paramount. This essay explores various strategies to combat fraud and protect against its detrimental effects.Firstly, education plays a crucial role in preventing fraud. Individuals need to be aware of common fraud schemes and tactics employed by fraudsters. By understanding how scams operate, people can better identify suspicious activities and avoid falling victim to them. Educational campaigns, workshops, and online resources can all contribute to raising awareness and promoting vigilance among the populace.Secondly, leveraging technology is essential in the fight against fraud. Advancements in artificial intelligence, machine learning, and data analytics enable the development of sophisticated fraud detection systems. These systems can analyze large volumes of data in real-time, flagging anomalous patterns or transactions indicative of fraudulent behavior. By harnessing the power of technology, organizations can strengthen their defenses and stay one step ahead of fraudsters.Furthermore, robust authentication mechanisms are critical in verifying the identities of individuals and safeguarding against impersonation fraud. Multi-factor authentication, biometric authentication, and digital signatures are examples of authentication methods that add layers of security to online transactions and communications. By implementing these measures, organizations can mitigate the risk of unauthorized access and identity theft.In addition to technological solutions, regulatory frameworks play a pivotal role in combating fraud.Governments and regulatory bodies enact laws and regulations to enforce transparency, accountability, and ethical conduct in financial transactions and business practices. Compliance with these regulations not only deters fraudulent activities but also fosters trust and confidence in the integrity of the financial system.Moreover, fostering a culture of integrity and ethical behavior within organizations is essential in preventing internal fraud. Employers should promote ethical values, provide whistleblowing mechanisms, and conduct regular audits to detect and deter fraudulent activities perpetrated by employees or insiders. By instilling a sense of accountability and integrity among staff, organizations can create a hostile environment for fraudsters.Additionally, collaboration and information sharing among stakeholders are crucial in combating fraud effectively. Public-private partnerships, industry forums, and information-sharing networks facilitate the exchange of intelligence and best practices in fraud prevention. By working together, stakeholders can pool resources, identifyemerging threats, and coordinate responses to mitigate the impact of fraud across sectors.In conclusion, preventing fraud requires a multifaceted approach encompassing education, technology, regulation, ethics, and collaboration. By implementing comprehensive strategies and measures, individuals and organizations can enhance their resilience against fraud and protect themselves from its detrimental consequences. Vigilance, innovation, and cooperation are key in staying ahead of fraudsters and safeguarding the integrity of financial systems and digital ecosystems.。
单细胞拉曼研究环境功能微生物

单细胞拉曼研究环境功能微生物《单细胞拉曼研究环境功能微生物》近年来,随着单细胞技术的迅速发展,科学家们在微生物学领域取得了令人瞩目的突破。
其中,单细胞拉曼技术的应用使得研究人员能够在非破坏性的情况下对微生物进行高分辨率的分析和检测。
该技术已经被广泛应用于环境功能微生物研究中,为我们揭示了微生物的奥秘,促进了环境保护和生物工艺技术的发展。
环境功能微生物是指在环境中发挥重要生物功能的微生物,例如污水处理、土壤修复和废物降解等。
通过对这些微生物的研究,我们能够深入了解它们在不同环境中的生存策略和代谢途径,为环境保护和可持续发展提供科学依据。
在传统的微生物研究中,常常需要通过培养方法将微生物分离、纯化,然后进行进一步的分析。
然而,这种方法无法获得所有微生物的真实信息,因为许多微生物无法在实验室环境下培养或是极难培养。
单细胞拉曼技术的出现弥补了这一缺陷,使得科学家们能够直接对微生物进行非破坏性的分析。
单细胞拉曼技术利用拉曼散射的原理,通过激光与微生物相互作用产生的拉曼散射光谱来分析微生物的结构和成分。
这种技术具有高分辨率、高灵敏度和非破坏性等优点,能够准确地分析微生物的代谢产物、蛋白质结构和细胞内分子成分等信息。
通过单细胞拉曼技术的应用,科学家们已经在环境功能微生物研究中取得了一系列重要的发现。
例如,在污水处理领域,研究人员利用单细胞拉曼技术对活性污泥中的微生物进行了分析,揭示了不同微生物之间的关系和相互作用机制,为提高污水处理效率和降低能耗提供了重要的理论支持。
此外,单细胞拉曼技术还被广泛应用于土壤修复研究。
科学家们利用该技术对土壤中的微生物进行分析,探究了微生物参与的土壤有机质的降解和循环过程,为土壤修复技术的改进和应用提供了理论指导。
可以预见,随着单细胞拉曼技术的不断进步和应用范围的扩大,将进一步推动环境功能微生物研究的发展。
这将有助于深入了解微生物在环境中的功能和生态作用,为解决环境问题和开发生物能源等提供更加精确和优化的解决方案。
城市计算
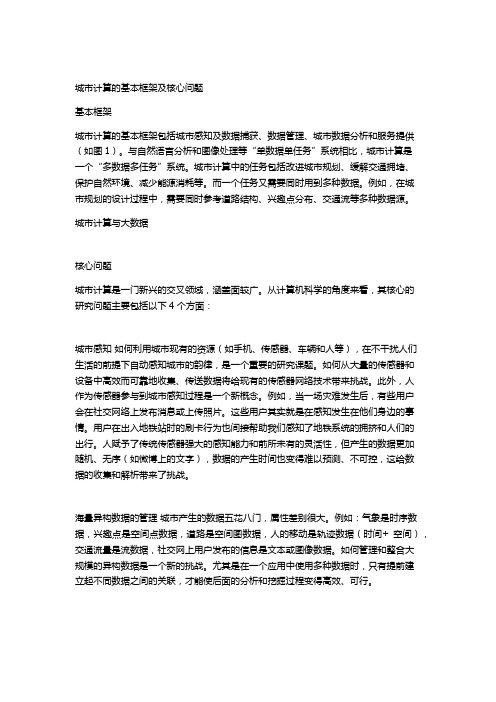
城市计算的基本框架及核心问题基本框架城市计算的基本框架包括城市感知及数据捕获、数据管理、城市数据分析和服务提供(如图1)。
与自然语言分析和图像处理等“单数据单任务”系统相比,城市计算是一个“多数据多任务”系统。
城市计算中的任务包括改进城市规划、缓解交通拥堵、保护自然环境、减少能源消耗等。
而一个任务又需要同时用到多种数据。
例如,在城市规划的设计过程中,需要同时参考道路结构、兴趣点分布、交通流等多种数据源。
城市计算与大数据核心问题城市计算是一门新兴的交叉领域,涵盖面较广。
从计算机科学的角度来看,其核心的研究问题主要包括以下4 个方面:城市感知如何利用城市现有的资源(如手机、传感器、车辆和人等),在不干扰人们生活的前提下自动感知城市的韵律,是一个重要的研究课题。
如何从大量的传感器和设备中高效而可靠地收集、传送数据将给现有的传感器网络技术带来挑战。
此外,人作为传感器参与到城市感知过程是一个新概念。
例如,当一场灾难发生后,有些用户会在社交网络上发布消息或上传照片。
这些用户其实就是在感知发生在他们身边的事情。
用户在出入地铁站时的刷卡行为也间接帮助我们感知了地铁系统的拥挤和人们的出行。
人赋予了传统传感器强大的感知能力和前所未有的灵活性,但产生的数据更加随机、无序(如微博上的文字),数据的产生时间也变得难以预测、不可控,这给数据的收集和解析带来了挑战。
海量异构数据的管理城市产生的数据五花八门,属性差别很大。
例如:气象是时序数据,兴趣点是空间点数据,道路是空间图数据,人的移动是轨迹数据(时间+ 空间),交通流量是流数据,社交网上用户发布的信息是文本或图像数据。
如何管理和整合大规模的异构数据是一个新的挑战。
尤其是在一个应用中使用多种数据时,只有提前建立起不同数据之间的关联,才能使后面的分析和挖掘过程变得高效、可行。
异构数据的协同计算这部分包括三个方面:(1) 如何从不同的数据源中获取相互增强的知识是一个新的课题。
传统的机器学习往往基于单一数据,如自然语言处理主要分析文本数据,图像视觉主要基于图像数据。
生态学部分词汇
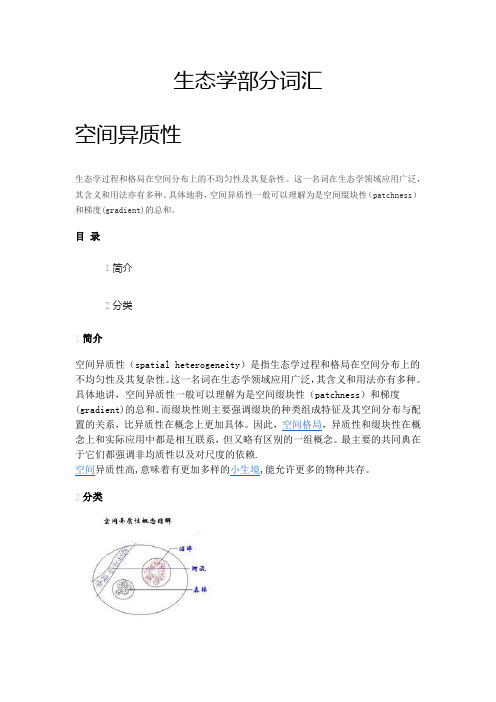
生态学部分词汇空间异质性生态学过程和格局在空间分布上的不均匀性及其复杂性。
这一名词在生态学领域应用广泛,其含义和用法亦有多种。
具体地将,空间异质性一般可以理解为是空间缀块性(patchness)和梯度(gradient)的总和。
目录1简介2分类1简介空间异质性(spatial heterogeneity)是指生态学过程和格局在空间分布上的不均匀性及其复杂性。
这一名词在生态学领域应用广泛,其含义和用法亦有多种。
具体地讲,空间异质性一般可以理解为是空间缀块性(patchness)和梯度(gradient)的总和。
而缀块性则主要强调缀块的种类组成特征及其空间分布与配置的关系,比异质性在概念上更加具体。
因此,空间格局,异质性和缀块性在概念上和实际应用中都是相互联系,但又略有区别的一组概念。
最主要的共同典在于它们都强调非均质性以及对尺度的依赖.空间异质性高,意味着有更加多样的小生境,能允许更多的物种共存。
2分类空间异质性(一)非生物环境的空间异质性Harman研究了淡水软体动物与空间异质性的相关性,他以水体底质的类型数作为空间异质性的指标,得到了正的相关关系:底质类型越多,淡水软体动物种数越多。
植物群落研究中大量资料说明,在土壤和地形变化频繁的地段,群落含有更多的植物种,而平坦同质土壤的群落多样性低。
(二)植物空间异质性MacArthur等曾研究鸟类多样性与植物的物种多样性和取食高度多样性之间的关系。
取食高度多样性是对植物垂直分布中分层和均匀性的测度。
层次多,各层次具更茂密的枝叶表示取食高度多样性高。
结果发现:鸟类多样性与植物种数的相关,不如与取食高度多样性相关紧密。
因此,根据森林层次和各层枝叶茂盛度来预测鸟类多样性是有可能的,对于鸟类生活,植被的分层结构比物种组成更为重要。
在草地和灌丛群落中,垂直结构对鸟类多样性就不如森林群落重要,而水平结构,即镶嵌性或斑块性(patchiness)就可能起决定作用宏观生态学常用空间异质性概念。
利用计算机视觉技术进行海洋底栖生物监测的研究
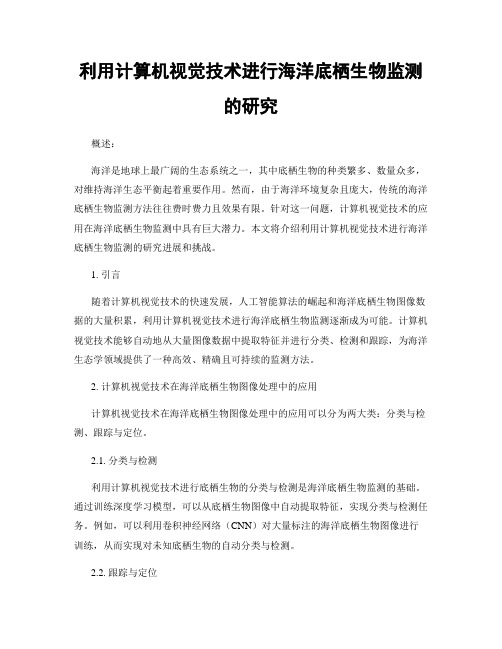
利用计算机视觉技术进行海洋底栖生物监测的研究概述:海洋是地球上最广阔的生态系统之一,其中底栖生物的种类繁多、数量众多,对维持海洋生态平衡起着重要作用。
然而,由于海洋环境复杂且庞大,传统的海洋底栖生物监测方法往往费时费力且效果有限。
针对这一问题,计算机视觉技术的应用在海洋底栖生物监测中具有巨大潜力。
本文将介绍利用计算机视觉技术进行海洋底栖生物监测的研究进展和挑战。
1. 引言随着计算机视觉技术的快速发展,人工智能算法的崛起和海洋底栖生物图像数据的大量积累,利用计算机视觉技术进行海洋底栖生物监测逐渐成为可能。
计算机视觉技术能够自动地从大量图像数据中提取特征并进行分类、检测和跟踪,为海洋生态学领域提供了一种高效、精确且可持续的监测方法。
2. 计算机视觉技术在海洋底栖生物图像处理中的应用计算机视觉技术在海洋底栖生物图像处理中的应用可以分为两大类:分类与检测、跟踪与定位。
2.1. 分类与检测利用计算机视觉技术进行底栖生物的分类与检测是海洋底栖生物监测的基础。
通过训练深度学习模型,可以从底栖生物图像中自动提取特征,实现分类与检测任务。
例如,可以利用卷积神经网络(CNN)对大量标注的海洋底栖生物图像进行训练,从而实现对未知底栖生物的自动分类与检测。
2.2. 跟踪与定位利用计算机视觉技术进行海洋底栖生物的跟踪与定位可以实现对特定个体或种群的长期监测。
基于目标跟踪算法,可以从视频序列中追踪目标生物的运动轨迹,并实现其位置的精确定位。
通过分析底栖生物的运动特征,可以深入了解其行为习性和生态环境,为海洋生态学的研究提供重要数据支持。
3. 计算机视觉技术在海洋底栖生物监测中的挑战尽管计算机视觉技术在海洋底栖生物监测中的应用具有巨大潜力,但仍面临一些挑战。
3.1. 数据质量海洋底栖生物图像数据的质量往往受到采集环境、光照条件和水质等因素的影响。
这些因素可能导致图像噪声、模糊和颜色失真等问题,从而对计算机视觉技术的应用造成困扰。
- 1、下载文档前请自行甄别文档内容的完整性,平台不提供额外的编辑、内容补充、找答案等附加服务。
- 2、"仅部分预览"的文档,不可在线预览部分如存在完整性等问题,可反馈申请退款(可完整预览的文档不适用该条件!)。
- 3、如文档侵犯您的权益,请联系客服反馈,我们会尽快为您处理(人工客服工作时间:9:00-18:30)。
On detection of emerging anomalous traf fic patterns using GPS dataLinsey Xiaolin Pang a,d,⁎,Sanjay Chawla a ,Wei Liu b ,Yu Zheng ca School of Information Technologies,University of Sydney,Australiab Dept.of Computer Science and Software Engineering,University of Melbourne,Australiac Microsoft Research Asia dNICTA,Sydney,Australiaa r t i c l e i n f o ab s t r ac tAvailable online 18May 2013The increasing availability of large-scale trajectory data provides us great opportunity to explore them for knowledge discovery in transportation systems using advanced data mining techniques.Nowadays,large number of taxicabs in major metropolitan cities are equipped with a GPS device.Since taxis are on the road nearly 24h a day (with drivers changing shifts),they can now act as reliable sensors to monitor the behavior of traffic.In this article,we use GPS data from taxis to monitor the emergence of unexpected behavior in the Beijing metropolitan area,which has the potential to estimate and improve traffic conditions in advance.We adapt likelihood ratio test statistic (LRT)which have previously been mostly used in epidemiological studies to describe traffic patterns.To the best of our knowledge the use of LRT in traffic domain is not only novel but results in accurate and rapid detection of anomalous behavior.Crown Copyright ©2013Published by Elsevier B.V.All rights reserved.Keywords:Data miningMining methods and algorithms Spatial/temporal databases1.IntroductionWith the increasing availability of high resolution GPS traces from vehicles in large metropolitan areas,there is an opportunity to infer sophisticated patterns and trends which till now has not been possible [1].The inferred trends can then be used as input into policy planning across a variety of domains including traffic management,urban planning and environmental monitoring.In this article,we apply statistical approach on massive taxi location traces,which are explored to extract the outlier traffic pattern in transportation systems.We know thousands of taxis ply the roads of large metropolitan cities like New York,London,Beijing and Tokyo every day.Most taxis are on the road 24h a day with drivers changing shifts.Many of these taxis are now equipped with GPS and their spatio-temporal coordinates are available.Thus if a city is partitioned into a grid then at a given time we can estimate the count of the number of taxis in the grid cells.Over time,the cell counts will settle into a pattern and vary periodically.For example,during morning rush hour more taxis will be concentrated in business districts than at other times of the day.Similarly taxi counts near airports will synchronize with aircraft arrival and departure schedules.Occasionally there will be a departure of the cell counts from periodic behavior due to unforeseen events like vehicle breakdowns or one-time events like big sporting events,fairs and conventions.Our objective is to identify contiguous set of cells and time intervals which have the largest statistically signi ficant departure from expected behavior.Once such regions and time intervals have been discovered then experts can begin identifying events which may have caused the unexpected behavior.This in turn can help make provisions to manage future traffic behavior.Similar problems appear in many other domains.For example,government healthcare agencies are interested in detecting emergence of disease patternswhich deviate from expected behavior.Data &Knowledge Engineering 87(2013)357–373⁎Corresponding author at:School of Information Technologies,University of Sydney,Australia.E-mail addresses:qlinsey@.au (L.X.Pang),sanjay.chawla@.au (S.Chawla),wei.liu@.au (W.Liu),yuzheng@ (Y.Zheng).0169-023X/$–see front matter.Crown Copyright ©2013Published by Elsevier B.V.All rights reserved./10.1016/j.datak.2013.05.002Contents lists available at ScienceDirectData &Knowledge Engineeringj o u r n a lh o m e p a ge :w ww.e l s e v i e r.c o m/l o c a t e /d a t a k358L.X.Pang et al./Data&Knowledge Engineering87(2013)357–373The number of contiguous regions and time intervals is very large.For example,if the spatial grid corresponds to a n×n matrix and there are T time intervals,then there are potentially O(n2T)spatio-temporal cells and O(n4T2)cubic regions.1The huge amount of spatio-temporal data,such as taxi count across different grid regions within different time steps from minutes to hours to days,requires an efficient approach to detect spatial–temporal outliers for predicting abnormal events and implementing traffic control measures in advance.For this motivation,we apply the road network of Beijing and partition it into grid to find outliers(Fig.1).In a paper of particular relevance to our work,the LRT framework[2]states the computation cost for single statistic value as well as enumerating all the spatial regions to be expensive.To avoid performing statistical computations for every region,it provides a pruning strategy based on classical likelihood test statistic.In this article,we extend the LRT framework to detect abnormal traffic pattern.More specifically,the contributions are:•A general and efficient pattern mining approach for spatio-temporal outlier detection is proposed.•Persistent and emerging outlier detection statistical models are provided.•We give our proof that the upper-bounding strategy of LRT is applicable to“persistent”and“emerging”outlier detection models.•Experiments are conducted on synthetic data to verify the extended pruning approach and show the significant improvement of searching when data set size is large;we also performed real data validation in the detection of emerging taxi count trend due to some major events.The rest of this article is organized as follows.Section2reviews related work.Section3illustrates the statistical background and upper-bounding methodology for pruning.Section4proposes our approach,in which the statistical detection models are provided.The upper-bounding and pruning mechanism in this framework based on our proof are presented in Section5. Computational complexity is also discussed in this section.Section6shows the experiments and case studies.Finally,Section7 concludes this work and Section8gives the future work.2.Related work2.1.Traffic outliersRecently,quite a few research projects started to find out traffic patterns and anomalies using taxi trajectories[3–6].For instance,to provide a user with the fastest route to a given destination at a given departure time,Yuan et al.[4]mine smart driving directions from historical GPS trajectories of a large number of taxis.It proposes a time-dependent landmark graph to model the properties of dynamic road networks and applies two-stage routing algorithm to find the efficient driving directions.In another work of Yuan et al.[3],it presents a cloud-based system to retrieve the fastest driving routes based on traffic conditions and driver behavior.The Cloud builds a model incorporating day of the week,time of day,weather conditions,and individual driving ing this model,the system predicts the traffic conditions of a future time by a given route and performs a self-adaptive driving direction service for a particular user.Mining traffic pattern is an important research approach,but detecting the outliers from main traffic flow is also meaningful. For instance,when a traffic incident or jam happens,traffic flow changes suddenly and this will be reflected by outliers.Traffic incidents can be detected through recognizing outliers.Such unusual traffic pattern reflects abnormal traffic streams on road networks and provides useful,important and valuable information.Unknown but potentially important patterns can be forecast by analyzing these outliers.Therefore,the detection of outliers/anomalies from trajectory data can help in sensing abnormal events and plan for their impact to ensure smoother flow of traffic.In Liu et al.'s[5]work,algorithms are presented for discovering spatio-temporal outliers and causal relationships.The discovery of relationships,especially causal interactions,among detected traffic outliers is investigated.Chawla and Zheng et al.[7]further diagnose detected traffic anomalies by studying the traffic flows (paths)that lead to an anomaly.In Zheng et al.'s[6]work,it detects flawed urban planning using the GPS trajectories of taxicabs traveling in urban areas.It finds anomalous patterns in a city using taxi trajectories which provides a deeper understanding of the flawed planning.Although these two approaches are used to detect traffic outliers,they are different from our work since we detect traffic outliers using statistical-based approach and we have a different definition for abnormal traffic patterns.2.2.Outlier detection methodsTill now,many anomaly detection techniques have been specifically developed for certain application domains,while others are more generic.The techniques can be categorized as classification-based,distanced-based,clustered-based,and statistical-based,etc. In these surveys[8,9],they give comprehensive and high-level overview of different outlier detection techniques and some of their applications.In this article,we focus on the statistic-based approach to detect spatio-temporal outlier.The underlying principle of any statistical outlier detection technique is:“An anomaly is an observation which is suspected of being partially or wholly irrelevant1In this work,n×n spatial grid and T time intervals are mapped to a three-dimensional grid.The unit cell is in the shape of a cube and every sub-region in the grid is called cubic region.because it is not generated by the stochastic model assumed ”[10].It is based on the key assumption:Normal data instances occur in high probability regions of a stochastic model,while anomalies occur in the low probability regions of the stochastic model.Statistical techniques fit a statistical model (usually for normal behavior)to the given data and then apply a statistical inference test to determine if an unseen instance belongs to this model or not.Instances that have a low probability from the applied test statistic are declared as outliers.Scoring techniques are used to assign an anomaly score to each instance in the test data depending on the degree to which that instance is considered an ually,the output of such techniques is a ranked list of outliers.We may choose to either analyze the top few outliers or use a cut-off threshold to select the outliers.Both parametric as well as non-parametric techniques have been applied to fit a statistical model [11,12].In our work,we only consider the parametric anomaly detection techniques based on the classical likelihood ratio test statistic (i.e.LRT).In the domain of spatio-temporal applications,most statistic outlier detection approaches proposed so far are on purely spatial searching [13–15].Even when considering time aspect,most of the existing work on spatio-temporal outlier detection treats the time dimension simply,either by applying purely spatial outlier detection methods at each time step,or by treating time as another spatial dimension and thus applying spatial outlier detection in one more dimensional space (original spatial dimensions plus time dimension).The disadvantage of the first approach is that by only examining one time step of data at a time,more slowly emerging outliers may not be detected.The disadvantage of the second approach is that less relevant outliers may be detected:those outliers that have constantly existed for a long time,rather than those that are newly emerging [16,17].In our following work,we differentiate temporal property with spatial property.More specifically,we investigate the spatial region in two different scenarios in which the temporal property is persistent or otherwise emerging.Persistent temporal data refers to data where its temporal property is consistent over time.Emerging temporal data refers to the data where its temporal property is non-decreasing over time.These two concepts provide more information for practical outlier detections.Among the various statistical methods for discovering outlier,the spatial and space –time scan statistic,introduced by Kulldorff [18–21],has been the most widely adopted.However,it is originally designed for Poisson and Bernoulli ter on,the different variations of ordinal,exponential and normal models are proposed [22–25].They have been implemented in the software (SaTScan)[26].In the space –time scan statistic of Kulldorff,the key parameter is assumed to be consistent over time.The technique simply applies time as one more dimension.Niell et al.[16]point out the distinct feature of time aspect and propose a modified test statistic to detect localized and globalized emerging cluster.Tango et al.[27]also propose a space –time scan statistic based on negative binomial model by taking into account the possibility of nonnegligible time-to-time variation of Poisson mean.Wu et al.[2]propose a generic framework called LRT for any underlying statistical model.It uses the classic likelihood ratio test (LRT)statistic as a scoring function to evaluate the “anomalousness ”of a given spatial region with respect to the rest of the spatial region.Moreover a generic pruning strategy was proposed to greatly reduce the number of likelihood ratio tests.However,it is used for spatial anomaly detection without considering the temporal property.Liu et al.[5]propose an approach to discover casual relationships among spatio-temporal outliers.Here,we only focus on detecting spatial –temporal outliers.2.3.Performance issueFurthermore,performance issue is also a big problem in spatial or spatio-temporal outlier detection.The naive computation of spatial outlier detection is very time-consuming,various strategies have been proposed to speed up the process [18,28–30].(a)Road Network of Beijing (b) Grid MapFig.1.An example of the traffic network of Beijing.Based on the longitude and latitude,the entire city is partitioned into a grid map.Sub-figure (a)is partitioned into sub-figure (b).359L.X.Pang et al./Data &Knowledge Engineering 87(2013)357–373These existing methods in the literature are based on Kulldorff's spatial scan statistic and they aim to actually avoid considering all O (n 4)rectangular areas.Also they are only applicable to those relatively simple density measures that are convex or monotonic with respect to the ratio of zone population over the entire population and the ratio of zone's event count over the entire event count.The LRT framework states the computation cost for single statistic value as well as enumerating all the spatial regions to be expensive [2].To avoid performing statistical computations for every region,it provides a pruning strategy based on classical likelihood test statistic.In this article,we extend it to be applicable to persistent and emerging outlier detection scenarios.3.Background3.1.The likelihood ratio test (LRT)We provide a brief but self-contained introduction for finding the most anomalous region (rectangle)in a spatial setting.The regions are rectangles mapped onto a spatial grid.We also explain a pruning strategy which can cut down the number of rectangles that need to be checked.The basic tool to find the anomalous region is the likelihood ratio test (LRT).Given a data set X ,the model distribution f (X ,θ),a null hypothesis H 0:θ∈Θ0and an alternate hypothesis H 1:θ∈Θ−Θ0,LRT is the ratioλ¼sup Θ0f L ðθX j ÞH 0j g Θ1j gðf where L ()is the likelihood function.θis a set of parameters coming from complete parameter space Θand null parameter space Θ0.See detail in [2,31].In a spatial setting,the null hypothesis is that the statistical aspect of the phenomenon of interest in a region R (that is currently being tested)is no different from rest of the spatial area (denoted as R ).Thus if a region R is anomalous then the alternate hypothesis will most likely be a better fit and the denominator of λwill have a higher value for the maximum likelihood estimator of θ.A remarkable fact about λis that under mild regularity conditions,the asymptotic distribution of Λ≡−2log λfollowsa χk 2distribution with k degrees of freedom,where k is the number of free parameters.2(see Fig.2).Thus regions whose Λvalue drops in the tail of χ2distribution are likely to be anomalous.3.2.Constrained maximum likelihood estimationConstrained maximum likelihood estimation is a set of procedures for the estimation of the parameters of models via the maximum likelihood method with general constraints on the parameters,along with an additional set of procedures for statistical inference [32].Barlow et al.[33,34]solved the maximum likelihood estimation based on a reliability growth model,which is applicable to our emerging scenario.It assumes that a system is being modified during K stages of development (in our application,we assume taxi counts in a region to be varying during K time steps).Data consists of x i successes in n i trials in stage i ,i =1,…,k .Let p i be the system reliability at the i-th stage (in our case,p i is the increasing rate of taxi count at i-th time step).Barlow et al.,obtained the maximum likelihood estimates of p 1,p 2,…,p k ,under the restriction that p 1≤p 2,≤…,≤p k .To obtain the maximum likelihood estimates of p 1,p 2,…,p k subject to the restriction that p 1≤p 2,…,≤p k ,first form the ratios x 1/n 1,x 2/n 2,…,x k /n k .If x 1/n 1≤x 2/n 2,≤…,≤x k /n k ,then x i /x n is the MLE ^pi of p i .If for some j (j =1,…,K −1),x j /n j ,combine the observations in the j-th and (j +1)-st stages and examine the ratios:x 1/n 1,…,x j −1/n j −1,x j +x j +1/n j +n j +1,x j +2/n j +2,…,x k /n k ,for the (k −1)stages thus formed.If these ratios are in non-decreasing order,they constitute the MLEs ofp 1≤p 2,≤…,≤p k with ^pj ¼^p j þ1¼x j þx j þ1ÀÁ=n j þn j þ1ÀÁ.If not,continue the process of combining stages until the ratios are in non-decreasing order.This process needs to be repeated at most (k −1)times,and the result is independent of the order in which stages are combined to eliminate reversals in the sequence of ratios.To simplify,we get:^pi ¼Max k ≥i Min s ≤i X k i ¼sx i =Xk i ¼sn i "#,where i =1,…,k .3.3.Monte Carlo simulationThe likelihood ratio,or equivalently its logarithm,can be used to compute a p -value,or compared to a critical value to decidewhether to reject null hypothesis (i.e.there is no anomaly in our case)in favor of the alternative hypothesis (i.e.there is anomaly).The probability distribution of likelihood ratio,assuming that the null hypothesis is true,can be approximated by chi-square distribution2If the χ2distribution is not applicable then Monte Carlo simulation can be used to ascertain the p-value.360L.X.Pang et al./Data &Knowledge Engineering 87(2013)357–373[35].But we cannot expect to find the distribution of the likelihood ratio test statistic in closed analytical form and thus Monte Carlo simulation can be performed to obtain p -value in some cases.Therefore,once we have discovered the region with maximum likelihood ratio value,the statistical significance of this region can be derived from the chi-square distribution or by conducting Monte Carlo simulations.To run the simulation test,a large number of replications of data sets are generated under the null hypothesis,for instance,9999such replicas are created to perform likelihood ratio test.The test is significant at the 5%level if the likelihood ratio value is among the 500highest values of the test statistic coming from the replications.3.4.Upper-bounding methodologyThe upper-bounding strategy for LRT for anomaly detection was introduced by Wu and Jermaine [2].The basic observation is that the likelihood value of any given region R under complete parameter space is not greater than the multiplication of the likelihood value of all its non-overlapping sub-regions under null parameter space.Therefore,the log likelihood of any given region R can be upper-bounded.For instance,if a region R is composed of two non-overlapping sub-regions R 1and R 2,thenL ðθR X R j Þ≤L ðθ′R 1j X R 1ÞÂL ðθ′R 2j X R 2Þ:It is equivalent tolog L ðθR X R j Þ≤log L ðθ′R 1j X R 1Þþlog L ðθ′R 2j X R 2Þ:Here θR ;θ′R 1and θ′R 2are the maximum likelihood estimators under complete and null parameter spaces separately.See Fig.3.Fig.2.Chi-square distribution with degree of freedom =3.Fig.3.The log likelihood of region R under complete parameter space is upper bounded by the sum of two non-overlapping subregions R 1and R 2under null parameter space.361L.X.Pang et al./Data &Knowledge Engineering 87(2013)357–373The upper-bounding strategy is used to prune non-outliers:If we replace the likelihood of a region R by the product of the likelihoods of its sub-regions and the new LRT is below the anomalous threshold (i.e.confidence level α),then R cannot be anomalous.3.5.Examples3.5.1.Example 1Using a simple but concrete example,we will now explain how to find anomalous region using traditional LRT computation and the upper-bounding pruning strategy.Consider the 4×4grid (G )in Fig.4.The number of successes (m i )independently generated by Poisson model P o (b i p )is displayed in each cell c i .The baseline b i in each cell c i is set to 10.The success rate p is 0.5for the region R and 0.1for the rest of cells.The significance level is set to α=0.05.We refer the success rate p as the test parameter.3.5.1.1.Procedures.For a given region R ,traditional LRT calculation involves several steps:maximum likelihood estimator for test parameter of R ,and G ;likelihood calculation of R ,and G ;the ratio calculation from the previous two steps.Although the calculation for Poisson distributed data can be simplified into 1EXP statistical model,in order to illustrate the traditional LRT computation,the original steps are carried out as the following:1.The likelihood function of each cell i is:f ðp c i j Þ¼b i p ðÞk i e −b i p ðÞk i !:ð1Þ2.The likelihood of any given region R ,which is composed of cell c 1,c 2,…,c i ,…,c t is:L ðp R j Þ¼Πc i ∈Rb i p ðÞk i e −b i pðÞi !:ð2Þ3.The MLE 0of p for a region R (denoted as ^p)is calculated as:^p ¼∑c i ∈R k i =∑c i∈R b ið3ÞThus ^p R ¼7þ8ðÞ10þ10ðÞ¼0:75.Similarly,^p R ,^p R 1,^p R 2and ^p G are obtained as:0.14,0.7,0.8and 0.21.4.The Λof region R is given byΛR ¼−2log L p G j Þþ2logL p ðj R ðÞþ2logL p ðj R ÀÁÞ¼−2log 0:2119þ15Âe −0:21Â160þ2log 0:7515Âe −0:75Â20þ2log 0:1419Âe −0:14Â140¼20:76:ð4ÞFrom the above steps,we get the exact log likelihood value of region R :log L (p |R )=−19.31;and the exact log likelihood of R 1and R 2:log L (p |R 1)=−9.49,and log L (p |R 2)=−9.78separately.We know the critical value of χ2(α)=3.84.Obviously,20.76is greater than 3.84.Therefore region R is treated as a potentialoutlier.Fig.4.An example of (4×4)grid to illustrate the LRT calculation and the upper-bounding pruning methodology.362L.X.Pang et al./Data &Knowledge Engineering 87(2013)357–3733.5.1.2.Upper-bounding pruning.We can also verify the upper-bound of region R :The sum of log likelihood of R 1and R 2is −19.27,which is greater than the exact log likelihood value of R which was computed as −19.31.Similarly,for region R ′,log L p R ′1 ¼−1:31 ,and log L p R ′2¼−1 ,the sum of log likelihood of R ′1and R ′2is −2.31.It is greater than the log likelihood value of R ′,which is −2.66.Furthermore,ΛR ′¼2:14,which is smaller than χ2(α)=3.84.This shows that the upper-bounded LRT value is smaller than the critical ing the pruning strategy,the actual value of region R ′need not be calculated and can be pruned.3.5.2.Example 2This example illustrates how to estimate maximum likelihood of system reliability in reliability growth model.To detect emerging outlier,we use this way to calculate maximum likelihood estimator.(1).The number of successes,population and success rate in each time step are shown in Table 1.(2).To estimate the maximum likelihood of ^Pi ,the process to get a sequence of non-decreasing ratios is summarized below according to the Barlow theorem (Table 2):From the above procedures,we obtain the maximum likelihood estimates:^P 1¼^P 2¼^P 3¼^P 4¼0:385;^P 5¼0:833.4.Proposed statistical modelsDe finition 1.KP :It refers to “key parameter ”,denoted as KP {θ1,θ2,…,θi ,…,θn }.θi is a parameter coming from the key parameterset.For instance,in epidemiology,if we are concerned about the trend of the disease rate in a spatio-temporal view,the disease rate is KP .In our application,the variation of taxi count within a period is KP .For simplicity,we only consider one parameter from the key parameter set in our work (denoted as KP ).4.1.PSTO model (Persistent Spatio-Temporal Outlier model)It is used to detect persistent spatio-temporal outliers.The null hypothesis H 0assumes that the KP is consistent for all regions over time.The alternative hypothesis H 1assumes that KP has a higher value in region r i ∈R than the value outside of region r j ∈G –R (i.e.R ),but the value in region r i ∈R is consistent over time.We calculate the likelihood ratio test as follows:D R ðÞ¼Πr i∈R L ðθr X R j ÞΠr i ∈RL ðθr j X r ÞΠr i ∈G G G j Þfor θr ≥θr ;1otherwise :8><>:This formula is the classical LRT statistic.We first calculate the MLE of θr and θr to maximize the numerator and the MLE of θG tomaximize the denominator.Then the ratio is the score we use to evaluate the “anomalousness ”of a given spatio-temporal region.4.2.ESTO model (Emerging Spatio-Temporal Outlier model)This model is used to detect emerging spatio-temporal outliers.The null hypothesis H 0assumes that the KP is consistent for all regions over time.The alternative hypothesis H 1assumes that KP is non-decreasing with every time step over region r i ∈R and higher than r j ∈R .We calculate the likelihood ratio test as follows:D R ðÞMax θr≤θt min ≤…≤θT Πr i ∈R L ðθt r j X t r ÞΠr i ∈R L ðθr t j X r t ÞΠr i ∈G L ðθt G j X t G Þfor θr ≤θt min ≤…≤θT ;1otherwise :8>>><>>>:9>>>=>>>;Table 1Reliability growth procedure.Time step (i )Number of successes (x i )Population (n i )Success rate (x i /n i )020500.400130700.429230800.375320600.333450600.833363L.X.Pang et al./Data &Knowledge Engineering 87(2013)357–373This formula is derived from the classical LRT statistic and designed for the emerging er needs to find a solution to maximize the numerator with the increasing KP .For instance,Barlow [34]provides an approach to solve the constrained maximum likelihood estimation on the reliability growth model in which the relative risk is non-decreasing over time.Or EM algorithm can be performed to estimate the key parameter.5.Upper-bounding strategy and pruning mechanism for proposed framework 5.1.Upper-bounding strategy(1).In PSTO model,the upper-bounding strategy explained in Section 3.4can be extended directly to spatio-temporaldimension.(2).In ESTO model,KP is assumed to vary at different time steps;we show below that the upper-bounding strategy is stillapplicable to this model.Theorem 1.Let region R =R t 1∪R t 2,for non-overlapping time intervals t1and t2,we have:L ðθR X R j Þ≤L ðθ′R t 1j X R t 1ÞÂL ðθ′R t 2j X R t 2Þð5Þ,where θR ¼θR t 1∪θR t 2and X R ¼X R t 1∪X R t 2.Proof.We know L θR X R j Þ¼L θR t 1X R t 1 ÁÂL θR t 2X R t 2 ÁÀÀÀ.Using the LRT upper-bounding basic concepts,we know that θR t 1is chosen under more strict complete parameter space and θ′R t 1is chosen under loosen null parameter space.This means that performing MLE 0on a sub-interval of R has loosen the constraints comparing with performing MLE 1on R .Thus,we have L ðθR t 1j X R t 1Þ≤L ðθ′R t 1j X R t 1Þand L ðθR t 2j X R t 2Þ≤L ðθR ′t 2j X R t 2Þ.Therefore,L ðθR X R j Þ≤L ðθ′R t 1j X R t 1ÞÂL ðθ′R t 2j X R t 2Þ.Theorem 2.Let region R =R 1∪R 2,for non-overlapping spatial regions R1and R2,we have:L ðθR 1;θR 2X R 1;X R 2j Þ≤L ðθ′R 1t 1;θ′R 1t 2j X R 1t 1;X R 1t 2ÞÂL ðθ′R 2t 1;θ′R 2t 2j X R 2t 1;X R 2t 2Þð6Þ,where R,R1,and R2are composed of (t1,t2)time steps respectively.Here we just use two time steps to illustrate.It is applicable to any t time steps.Proof.For each time step i ,we have:L ðθR ti j X R ti Þ≤L ðθ′R 1ti j X R 1ti ÞÂL ðθ′R 2ti j X R 2ti ÞL θR 1;θR 2X R 1;X R 2j Þ¼L θR 1ðj X R 1ðÞÂL θR 2ðj X R 2ÞL ðθR 1t 1;θR 1t 2j X R 1t 1;X R 1t 2Þ¼L ðθR 1t 1j X R 1t 1ÞÂL ðθR 1t 2j X R 1t 2ÞL ðθR 2t 1;θR 2t 2j X R 2t 1;X R 2t 2Þ¼L ðθR 2t 1j X R 2t 2ÞÂL ðθR 2t 2j X R 2t 2ÞTherefore we getL ðθR 1;θR 2X R 1;X R 2j Þ≤L ðθ′R 1t 1;θ′R 1t 2j X R 1t 1;X R 1t 2ÞÂL ðθ′R 2t 1;θ′R 2t 2j X R 2t 1;X R 2t 2Þ:From Theorems 1and 2,we know that the upper-bounding strategy is applicable to emerging model (ESTO ).Table 2The maximum likelihood estimator procedure.i x i n i x i /n i First calculation Second calculation Third calculation 120500.4000.40080/210=0.381100/260=0.385230700.42960/150=0.400330800.375420600.3330.333550600.8330.8330.8330.833364L.X.Pang et al./Data &Knowledge Engineering 87(2013)357–373。