Relationship Extraction from Biomedical Documents using Conditional Random Fields
取样分离法的英文缩写

取样分离法的英文缩写The Abbreviation of Sampling Separation MethodIntroduction:Sampling separation is a crucial process in various scientific fields, aiming to isolate and extract specific components from a mixture for further analysis or application. In order to simplify its usage, an abbreviation, also known as an acronym or initialism, is often assigned to represent the sampling separation method. This article explores the significance of abbreviations for sampling separation methods in the English language.Abbreviations in Sampling Separation Methods:Abbreviations play a vital role in simplifying complex terminologies, especially in scientific research. By condensing a long and technical name into a shorter form, abbreviations make it easier for researchers, professionals, and even general readers to refer to a particular sampling separation method efficiently. These abbreviations are widely accepted and recognized across the scientific community and help in creating a standardized language for communication.Importance of Abbreviations:1. Enhanced Communication:Abbreviations act as effective communication tools, as they facilitate easy and precise exchange of information between researchers and experts. The use of abbreviations enables efficient and concise communication in scientific journals, research papers, and conferences. Additionally,abbreviations assist in the dissemination of knowledge, allowing researchers to present their work without the cumbersome repetition of lengthy methodologies.2. Time-Saving:The utilization of abbreviations saves valuable time for both the writer and the reader. Instead of repeatedly writing the complete name of a sampling separation method, researchers can use the respective abbreviation, reducing the overall length of the text. This time-saving benefit enables authors to focus on explaining the nuances of the method rather than wasting words on repetitive instances of its name.3. Standardization:Standardization plays a pivotal role in establishing a common platform for sharing and understanding scientific concepts. Abbreviations ensure uniformity and consistency in scientific literature and research, as the same abbreviation is used for a specific sampling separation method by different scientists and researchers. This standardization eliminates confusion and ambiguity that may arise due to variations in the nomenclature of different techniques.Examples of Abbreviations:1. Liquid-Liquid Extraction (LLE): LLE is a widely used method for extracting a compound of interest from a liquid mixture by partitioning it between two immiscible liquids.2. Solid Phase Extraction (SPE): SPE is a technique that involves the use of a solid adsorbent to extract and isolate specific analytes from a sample matrix.3. Gas Chromatography (GC): GC is an analytical method used to separate and analyze volatile compounds in a gaseous mixture.4. High-Performance Liquid Chromatography (HPLC): HPLC is a technique that utilizes high-pressure pumps to separate and identify components in a liquid mixture.Conclusion:Sampling separation methods are pivotal in various scientific fields for accurate analysis and understanding of mixtures. Abbreviations associated with these methods have become essential tools for efficient communication, time-saving writing practices, and standardization of terminologies in scientific literature. It is crucial for scientists and researchers to utilize these abbreviations accurately and consistently, ensuring effective knowledge dissemination across the scientific community.。
马齿苋中抗炎活性物质的提取、分离及结构鉴定
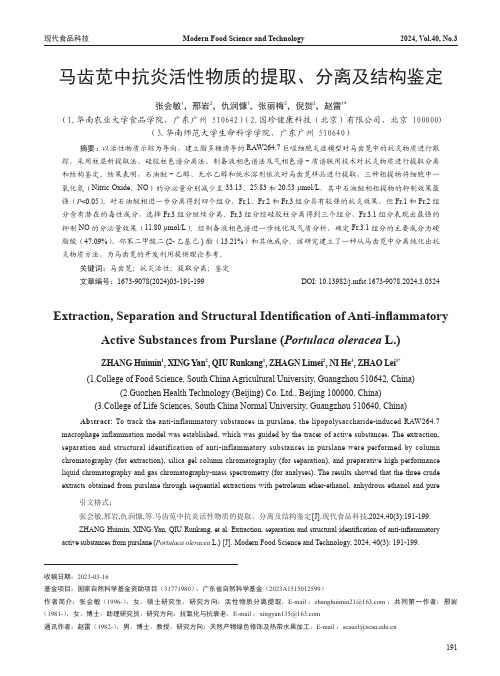
马齿苋中抗炎活性物质的提取、分离及结构鉴定张会敏1,邢岩2,仇润慷1,张丽梅2,倪贺3,赵雷1*(1.华南农业大学食品学院,广东广州 510642)(2.国珍健康科技(北京)有限公司,北京 100000)(3.华南师范大学生命科学学院,广东广州 510640)摘要:以活性物质示踪为导向,建立脂多糖诱导的RAW264.7巨噬细胞炎症模型对马齿苋中的抗炎物质进行跟踪,采用柱层析提取法、硅胶柱色谱分离法、制备液相色谱法及气相色谱-质谱联用技术对抗炎物质进行提取分离和结构鉴定。
结果表明,石油醚-乙醇、无水乙醇和纯水溶剂依次对马齿苋样品进行提取,三种粗提物将细胞中一氧化氮(Nitric Oxide,NO)的分泌量分别减少至33.13、25.83和20.53 μmol/L,其中石油醚相粗提物的抑制效果最强(P<0.05)。
对石油醚相进一步分离得到四个组分,Fr.1、Fr.2和Fr.3组分具有较强的抗炎效果,但Fr.1和Fr.2组分含有潜在的毒性成分,选择Fr.3组分继续分离。
Fr.3组分经硅胶柱分离得到三个组分,Fr.3.1组分表现出最强的抑制NO的分泌量效果(11.80 μmol/L)。
经制备液相色谱进一步纯化及气质分析,确定Fr.3.1组分的主要成分为硬脂酸(47.09%)、邻苯二甲酸二(2-乙基己)酯(13.21%)和其他成分。
该研究建立了一种从马齿苋中分离纯化出抗炎物质方法,为马齿苋的开发利用提供理论参考。
关键词:马齿苋;抗炎活性;提取分离;鉴定文章编号:1673-9078(2024)03-191-199 DOI: 10.13982/j.mfst.1673-9078.2024.3.0324Extraction, Separation and Structural Identification of Anti-inflammatory Active Substances from Purslane (Portulaca oleracea L.)ZHANG Huimin1, XING Y an2, QIU Runkang1, ZHAGN Limei2, NI He3, ZHAO Lei1*(1.College of Food Science, South China Agricultural University, Guangzhou 510642, China)(2.Guozhen Health Technology (Beijing) Co. Ltd., Beijing 100000, China)(3.College of Life Sciences, South China Normal University, Guangzhou 510640, China)Abstract: To track the anti-inflammatory substances in purslane, the lipopolysaccharide-induced RAW264.7 macrophage inflammation model was established, which was guided by the tracer of active substances. The extraction, separation and structural identification of anti-inflammatory substances in purslane were performed by column chromatography (for extraction), silica gel column chromatography (for separation), and preparative high performance liquid chromatography and gas chromatography-mass spectrometry (for analyses). The results showed that the three crude extracts obtained from purslane through sequential extractions with petroleum ether-ethanol, anhydrous ethanol and pure引文格式:张会敏,邢岩,仇润慷,等.马齿苋中抗炎活性物质的提取、分离及结构鉴定[J] .现代食品科技,2024,40(3):191-199.ZHANG Huimin, XING Yan, QIU Runkang, et al. Extraction, separation and structural identification of anti-inflammatory active substances from purslane (Portulaca oleracea L.) [J] . Modern Food Science and Technology, 2024, 40(3): 191-199.收稿日期:2023-03-16基金项目:国家自然科学基金资助项目(31771980);广东省自然科学基金(2023A1515012599)作者简介:张会敏(1996-),女,硕士研究生,研究方向:活性物质分离提取,E-mail:;共同第一作者:邢岩(1981-),女,博士,助理研究员,研究方向:抗氧化与抗衰老,E-mail:通讯作者:赵雷(1982-),男,博士,教授,研究方向:天然产物绿色修饰及热带水果加工,E-mail:191water solvents reduced the secretion of nitric oxide (NO) in the cells to 33.13, 25.83 and 20.53 μmol/L, respectively, with the crude petroleum ether extract exhibiting the strongest inhibitory effect (P<0.05). The petroleum ether phase was further separated into four fractions, with the Fr.1, Fr.2 and Fr.3 fractions had stronger anti-inflammatory effects, though the Fr.1 and Fr.2 fractions contained potential toxic components. Therefore, the Fr.3 fraction was selected for further separation. The Fr.3 fraction was separated through a silica gel column to obtain three fractions. The Fr.3.1 subfraction exhibited the strongest inhibitory effect against the NO secretion (11.80 μmol/L). The Fr.3.1 subfraction was further purified by the preparative liquid chromatography and GC-MS analysis, and the main components of the Fr.3.1 subfraction were identified as stearic acid (47.09%), di(2-ethylhexyl)phthalate (13.21%) and other components. This study established a method for separating and purifying anti-inflammatory substances from purslane, and provides a theoretical reference for the development and utilization of purslane.Key words: Portulaca oleracea L.; anti-inflammatory activity; extraction and isolation; identification炎症是机体受到外部刺激时做出的一种保护性生理反应,能够及时清除体内受损或死亡的细胞,帮助机体恢复内部平衡[1] 。
生物分离工程的英语

生物分离工程的英语Biological Separation Engineering is a specialized field that focuses on the isolation and purification of biological products. It plays a crucial role in the pharmaceutical, food, and biotechnology industries, where the extraction ofbioactive compounds from natural sources is essential.The process typically begins with the selection of an appropriate feedstock, which could be anything from plant material to microorganisms. Once the feedstock is identified, it undergoes a series of steps to separate the desired components. These steps may include:1. Pre-treatment: This involves breaking down the complex structure of the feedstock to release the target molecules. Techniques such as mechanical disruption, enzymatic digestion, or chemical treatment may be used.2. Extraction: The target molecules are then extractedfrom the pre-treated material. This can be done using solvent extraction, where a solvent is used to dissolve the desired compounds, or by using methods like supercritical fluid extraction, which employs high-pressure gases to extract the compounds.3. Concentration: After extraction, the solution is often diluted and needs to be concentrated to increase the concentration of the target molecules. This can be achievedthrough evaporation, membrane filtration, or centrifugation.4. Purification: The concentrated solution may still contain impurities, so further purification is necessary. Chromatography is a common technique used at this stage, which separates molecules based on their affinity to the stationary phase.5. Polishing: The final step is to polish the purified product to ensure it meets the required specifications. This may involve additional rounds of purification or the use of specific techniques to remove any remaining impurities.Biological separation engineering is a complex process that requires a deep understanding of both the properties of the target molecules and the various separation techniques available. Advances in this field are continually improving the efficiency and selectivity of these processes, making it possible to produce high-quality biological products for a wide range of applications.。
Senserelations语义关系

Semantic relationships are the foundation of language understanding. By analyzing semantic relationships, one can understand the meaning of words and sentences, and thus comprehend the meaning of the entire text.
要点一
要点二
Detailed description
Semantic conflict refers to the situation where two concepts or entities are contradictory or mutually exclusive in meaning and nature. For example, "peace" and "war" are conflicting because they represent opposite meanings and states.
Semantic relevance
Refers to the existence or attribute of one concept or entity containing the existence or attribute of another concept or entity.
Summary word
Statistical methods
Deep learning based methods
Summary: Based on deep learning methods, neural network models are used to recognize and calculate semantic relationships by learning semantic patterns from corpora.
不同方法保存的患病异育银鲫中鲤疱疹病毒2型DNA提取和PCR扩增效果分析

第45卷第6期Vol.45 No.6淡水渔业Freshwater Fisheries2015年11月Nov.2015不同方法保存的患病异育银鲫中鲤疱疹病毒2型D N A提取和P C R扩增效果分析卢俊,陆宏达,岳蒙蒙,操艮萍(上海海洋大学水产与生命学院,上海201306)摘要:以患鲤疱瘆病毒2型(C y A n i h#AeOTAu 2, CyHV-2)疾病的异育银卿(C a ra s u auratos —纟!/)肾脏为实验材料,采用-20丈冷冻、100%乙醇、Dafan o P液、10%福尔马林、Zenker P液、Muller S液、 2.5%戊二醛、Hel-l y p液、Mossmanp液、Bouinp液和Cam oyp液不同方法进行保存,通过微量分光光度计检测和琼脂糖凝胶电泳探讨不同保存方法对病毒DNA提取效果、常规PC R扩增效果和巢式PC R扩增效果的影响。
结果表明:从病鱼肾脏中提取D NA时,除100%乙醇保存方法与-20丈冷冻保存组一样可以提取高质量的DNA外,其它保存方法对DNA提取有不同程度的影响,100%乙醇保存方法和保存的材料可以用作于鲤疱疹病毒2型D N A的提取;常规PCR扩增时,100%乙醇、ZenkemS液、 2.5%戊二醛、Helly P液、BO u in P液和CamO y P液保存方法与-20m冷冻保存组具有相同的效果,在362 b p处出现明亮一致的清晰单一目的条带,这6种保存方法及其保存的材料可以用作鲤疱疹病毒2型的常规PCR扩增;巢式PCR扩增时,100%乙醇、DafanoP液、10%福尔马林、Zenkers液、Muller’s液、2. 5%戊二醛、H ellyp液、Mossman’s液、Bouin’s液和Carnoy’s液所有保存方法与-20 m冷冻保存组具有相同的效果,在339 b p处出现明亮一致的清晰单一目的条带,这些保存方法及其保存的材料均可以用作于鲤疱疹病毒2型的巢式P CR扩增,在采用巢式PCR进行检测和诊断患鲤疱疹病毒2型疾病上具有应用价。
Microbial DNA Extract

Microbial DNA ExtractMicrobial DNA extraction is a crucial process in microbiology and molecular biology, playing a fundamental role in various scientific and medical applications. The extraction of microbial DNA involves isolating and purifying the genetic material from microorganisms such as bacteria, fungi, and viruses. This process is essential for studying the genetic makeup of microorganisms, understanding their roles in various ecosystems, and diagnosing infectious diseases. In this discussion, we will explore the significance of microbial DNA extraction, the methods and techniques involved, as well as the applications and implications of this process. First and foremost, it is important to recognize the significanceof microbial DNA extraction in scientific research and medical diagnostics. Microorganisms play a pivotal role in various ecological processes, and understanding their genetic makeup is crucial for comprehending their functionsand interactions within different environments. Additionally, microbial DNA extraction is essential for the diagnosis of infectious diseases caused by bacteria, viruses, and fungi. By isolating and analyzing the DNA of pathogenic microorganisms, healthcare professionals can accurately identify and treat infectious diseases, thereby contributing to public health and disease management. In terms of methods and techniques, there are several approaches to microbial DNA extraction, each with its own advantages and limitations. Common methods include the use of chemical and mechanical lysis to break open microbial cells, followedby purification of the DNA using techniques such as phenol-chloroform extraction, silica membrane-based purification, or magnetic bead-based purification. Thechoice of extraction method depends on various factors such as the type of microorganism, the sample source, and the downstream applications of the extracted DNA. It is essential to carefully select the most suitable method to ensure the purity and integrity of the extracted DNA for accurate and reliable analysis. Furthermore, the applications of microbial DNA extraction are diverse and far-reaching. In research settings, extracted microbial DNA is used for various molecular biology techniques, including polymerase chain reaction (PCR), DNA sequencing, and metagenomic analysis. These techniques allow scientists to study the genetic diversity of microorganisms, investigate their evolutionaryrelationships, and explore their potential biotechnological and industrial applications. Moreover, in clinical diagnostics, microbial DNA extraction is integral to the detection and identification of pathogenic microorganisms, enabling rapid and accurate diagnosis of infectious diseases, as well as the monitoring of antimicrobial resistance. From an ethical standpoint, the implications of microbial DNA extraction raise important considerations regarding biosecurity, biosafety, and the responsible use of genetic information. The handling and storage of microbial DNA must adhere to strict biosafety protocols to prevent accidental release or misuse of potentially hazardous genetic material. Additionally, the ethical implications of accessing and analyzing microbial genetic information should be carefully considered to ensure that privacy and confidentiality are maintained, particularly in the context of human-associated microorganisms and infectious disease diagnostics. In conclusion, microbial DNA extraction is a fundamental process with significant implications for scientific research, medical diagnostics, and ethical considerations. The extraction of microbial DNA enables the study of microorganisms' genetic makeup, their roles in ecosystems, and the diagnosis of infectious diseases. The diverse methods and techniques involved in microbial DNA extraction, along with its wide-ranging applications, underscore its importance in various fields of microbiology and molecular biology. It is imperative to approach microbial DNA extraction with a thorough understanding of its significance, methods, applications, and ethical implications to ensure its responsible and beneficial use in scientific and medical endeavors.。
孟德尔随机化方法在胃癌危险因素研究中的应用
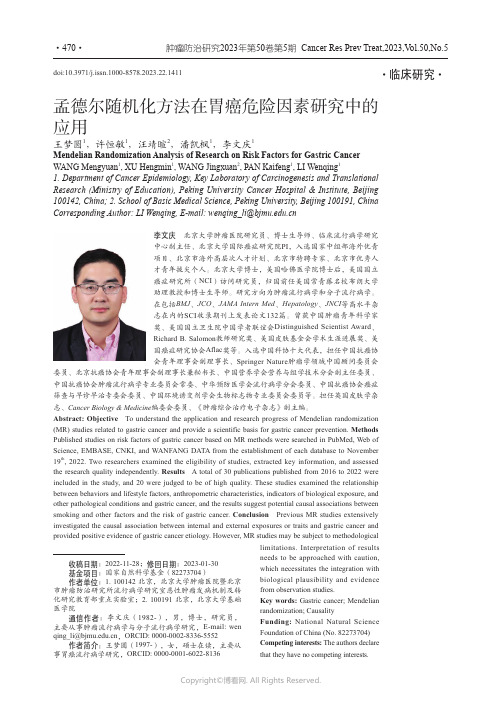
doi:10.3971/j.issn.1000-8578.2023.22.1411孟德尔随机化方法在胃癌危险因素研究中的应用王梦圆1,许恒敏1,汪靖暄2,潘凯枫1,李文庆1Mendelian Randomization Analysis of Research on Risk Factors for Gastric Cancer WANG Mengyuan 1, XU Hengmin 1, WANG Jingxuan 2, PAN Kaifeng 1, LI Wenqing 11. Department of Cancer Epidemiology, Key Laboratory of Carcinogenesis and Translational Research (Ministry of Education), Peking University Cancer Hospital & Institute, Beijing 100142, China;2. School of Basic Medical Science, Peking University, Beijing 100191, China CorrespondingAuthor:LIWenqing,E-mail:*******************.cn李文庆 北京大学肿瘤医院研究员、博士生导师、临床流行病学研究中心副主任、北京大学国际癌症研究院PI ,入选国家中组部海外优青项目、北京市海外高层次人才计划、北京市特聘专家、北京市优秀人才青年拔尖个人。
北京大学博士,美国哈佛医学院博士后,美国国立癌症研究所(NCI )访问研究员,归国前任美国常青藤名校布朗大学助理教授和博士生导师。
研究方向为肿瘤流行病学和分子流行病学。
在包括BMJ 、JCO 、JAMA Intern Med 、Hepatology 、JNCI 等高水平杂志在内的SCI 收录期刊上发表论文132篇。
生物材料中提取的案例
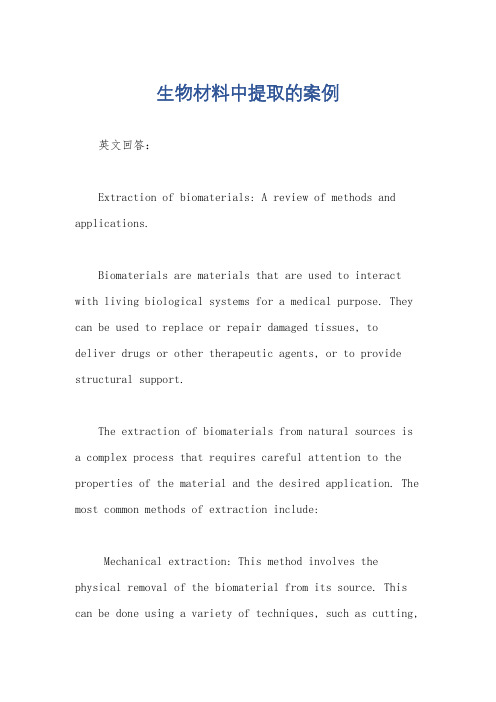
生物材料中提取的案例英文回答:Extraction of biomaterials: A review of methods and applications.Biomaterials are materials that are used to interact with living biological systems for a medical purpose. They can be used to replace or repair damaged tissues, todeliver drugs or other therapeutic agents, or to provide structural support.The extraction of biomaterials from natural sources is a complex process that requires careful attention to the properties of the material and the desired application. The most common methods of extraction include:Mechanical extraction: This method involves the physical removal of the biomaterial from its source. This can be done using a variety of techniques, such as cutting,grinding, or milling.Chemical extraction: This method involves the use of chemicals to dissolve or extract the biomaterial from its source. This can be done using a variety of chemicals, such as acids, bases, or solvents.Biological extraction: This method involves the use of biological agents, such as enzymes or bacteria, to extract the biomaterial from its source.The choice of extraction method depends on a number of factors, including the nature of the biomaterial, the desired application, and the desired properties of the extracted material.Once the biomaterial has been extracted, it can be further processed to improve its properties or to make it more suitable for a specific application. This processing may include:Purification: This process removes impurities from thebiomaterial.Sterilization: This process kills bacteria and other microorganisms that may be present in the biomaterial.Modification: This process alters the properties of the biomaterial to make it more suitable for a specific application.Biomaterials have a wide range of applications in medicine, including:Tissue engineering: Biomaterials can be used to create scaffolds for the growth of new tissues.Drug delivery: Biomaterials can be used to deliver drugs or other therapeutic agents to specific parts of the body.Structural support: Biomaterials can be used to provide structural support to damaged tissues or organs.The extraction of biomaterials from natural sources is a critical step in the development of new medical technologies. By understanding the different methods of extraction and processing, researchers can develop biomaterials that are more effective and more suitable for a wider range of applications.中文回答:生物材料提取,方法和应用综述。
- 1、下载文档前请自行甄别文档内容的完整性,平台不提供额外的编辑、内容补充、找答案等附加服务。
- 2、"仅部分预览"的文档,不可在线预览部分如存在完整性等问题,可反馈申请退款(可完整预览的文档不适用该条件!)。
- 3、如文档侵犯您的权益,请联系客服反馈,我们会尽快为您处理(人工客服工作时间:9:00-18:30)。
Relationship Extraction from Biomedical Documents usingConditional Random FieldsSaurav Sahay, Jinhan Lee, Niyant KrishnamurthiCollege of Computing, Georgia Tech.AbstractExtracting complex relationships automatically from unstructured information resources is a challenging problem. It is an important problem in this present age of abundant machine processable information as there is a need to build intelligent knowledge-aware applications for tasks such search, extraction and reasoning. We have used Conditional Random Fields (CRFs) to identify various relationships from biomedical abstracts.1. IntroductionWe look at the problem of relationship extraction as a sequence labelling and segmentation problem of observation instances. By relationship, we mean the type of association between two neighbouring entities in the domain. More explicitly, a relationship in our context is a {Concept1, Relationship, Concept2} triple. Figure 1 shows a part of the map extracted automatically from Medline abstracts related to Nuclear Cardiology.Figure 1. Map of Relationships extracted from Medline abstractsGraphical models such as Hidden Markov models (HMMs) have been most commonly applied in several bioinformatics, linguistics, modelling and recognition problem. HMMs are directed generative models that involve computation of P(y,x) whereas CRFs are undirected discriminative models that involve computation of P(y|x) (comparable to naïve bayes and logistic regression).2. Related WorkThis problem is similar to the task of named entity recognition from text and there is a lot of work in this area of language processing such as Part of Speech Tagging, Noun Phrase Chunking and Semantic Role Labelling. McCallum, Lafferty and Sutton [1-5] who invented the CRF formalism have several work using CRF to sequence and label data. Bunescu et al [6] have compared linearchain CRFs with Relational Markov Network (RMN)[7] for information extraction, the problem of identifying phrases in natural language text that refer to specific types of entities. Craven et al[8] have used relational learning methods to extract facts from text in order to construct molecular biology knowledgebases. Zhao et al [9] have described collective classification of actors, events and relationships in affiliation networks using RMNs.3. Conditional Random FieldConditional Random Fields (CRFs) are conditional probability distribution models that factorize based on an undirected model. It models the conditional distribution P(Y|X) where X is a set of input variable we observe and Y is a set of hidden variables we predict. By modeling the conditional distribution directly, it does not require to model the distribution P(X), which can includes complex dependencies. In other words, dependencies among the X do not need to be explicitly represented so that CRF can afford the use of rich global features of the input X.3.1 Linear-chain CRFA linear-chain CRF assumes a first-order Markov assumption on the dependencies among hiddenvariable y.Figure 2. Linear Chain CRFA linear-chain conditional random field takes form, where a set of real-valued feature functions isK k t k x y y f 1)},',({= and an instance-specific normalization function is )(x Z . A feature function ),,(1t t t k x y y f − could be arbitrary.∑=−=K k t t t k k x y y f x Z x y p 11)},,(exp{)(1)|(λ, ∑∑=−=y Kk t t t k k x y y f x Z 11)},,(exp{)(λ3.2 Dynamic CRFA dynamic CRF is a generalization of linear-chain CRFs that factorizes based on an undirected model whose structure and parameters are repeated. Model can have a set of hidden variables and complex interaction between hidden variables.Figure 3. Dynamic CRFA dynamic conditional random field takes form ∏∏∑∈=−=t C c K k t t t k k x y y f x Z x y p 11)},,(exp{)(1)|(λ ∑∏∏∑∈==y t C c Kk t c t k k x y f x Z 1,)},(exp{)(λInference in these models can be done using any of the inference algorithms for undirected models. Viterbi decoding is generally used for sequence labelling and marginal computation is used for parameter estimation. Exact inference is generally expensive in Dynamic models hence approximate inference techniques such as loopy Belief Propagation is applied to compute probabilities.4. MethodsWe have setup our relationship extraction task as a text classification problem where we have used rich linguistic and semantic features apart from local contextual features as feature vectors for the sentence phrases. More specifically, we have assigned features to each syntactic phrase of the sentences in biomedical abstracts. Relationships span across several phrases in a sentence. In order to capture this complex structure, we have used features from the neighbouring phrases and added them to each phrase’s set of feature.We have extracted relationships from biomedical abstracts in order to create our benchmark dataset. Our relationship extraction process is described in Figure 4. We have used NLM'sUnified Medical Language System (UMLS), a very large ontology of biomedical and health data as a dictionary for mapping biomedical terms to their Concept types and Semantic Types. We have used WordNet Lexical database for finding synonyms of verb phrases in abstracts in order to categorize them into a set of predefined relationships. The relationships are categorized as ‘affects ’, ‘causes ’, ‘exhibits ’, ‘analyzes ’, etc between the pair of concepts surrounding the verb phrases.The algorithm for relationship extraction is summarized in Figure 4[10].For each abstract AFor each sentence S in AFind occurrences of domain concept pairs in S Æ PairsFor each concept pair <C1, C2> in PairsApply verbGroup matching classify <C1,C2> into relations Æ RAdd R to Relationship Mapreturn MapFigure 4: Relation extraction algorithmExamples of some extracted and categorized concepts and relationships are as follows (Figure 5):left ventricle DIVIDED <PART_OF> regional myocardial uptakesstudy EVALUATED <MEASURES> impactprognosis population COMPRISED <CONTAINS> 16,020 consecutive patientscardioinhibitory response SHOWED <EXHIBITS> vasodepressor responseeighteen patients UNDERWENT <BRINGS_ABOUT> i-123 mibgconstant supply SUSTAIN <PREVENTS> contractile functionFigure 5: Categorized relationshipsWe have used this set of categorized relationships as labels for the phrases surrounding the concepts. For a majority of phrases, where there are no semantic relationships between surrounding concepts, we categorize them as having no relationships. Thus, we have a sparse dataset of few relationships among phrases in our training as well as test dataset.Our feature extraction algorithm is described in the following pseudo-code (Figure 6).For each phrase P in sentence SFor phrase neighborhood of {-n,+n} phrasesExtract contextual, linguistic and semantic featuresIf phrase matches in relationship R from MapLabel PhraseFeatureVector with verbGroup of RElse Label PhraseFeatureVector with type ‘None’Return PhraseFeatureVectorFigure 6: Feature extraction algorithm4.1 Choice of FeaturesThe choice of features to describe the relationship labels is extremely important to get good results for this problem. In most text classification problems, a simple ‘bag of words’ approach is taken to populate the vector space of features. These features are statistically extracted using techniques like ‘term frequency – inverse document frequency’ (TFIDF) or z-score method. These statistical features make the space of possible feature set extremely large thus requiring huge training data to come up with good decision boundaries for classification of data into the right categories.In contrast, we have used rich syntactic and semantic features for our data exploiting the rich and freely available ontologies like UMLS[11] and WordNet. We have extracted Parts of Speech information for the phrases using a Biomedical Parts of Speech tagger MedPostSKRTagger[12].The feature categories that we have used are as follows:1.Phrase2.Concept Types of phrase3.Semantic Type of phrase4. Part of Speech of phraseWe have used these features for the current as well as neighbouring phrases for capturing the rich semantics of the text to be categorized.Examples of these features are:Phrase – myocardial perfusion, Concept Type – Myocardial Perfusion, Semantic Type – Organism Attribute, Part of Speech – Noun PhrasePhrase – examined, Concept Type – Examine, Semantic Type – Finding, Part of Speech – Verb Phrase5. Experiments5.1 Recognizing a relation phraseThe aim of this experiment was to label a phrase as a relation if it defines any relationship. This binary classification experiment labelled the phrases as a ‘relation’ phrase or ‘none’. This is still a difficult classification because relationship phrases constitute less than 5% of the entire text corpus. The CRF must learn this differentiation using features which contain the POS tags, concepts, types of concepts and the actual phrases. The concepts of phrases that come in a window are specified along with their relative position. The concepts of phrases that come before the current relation phrase are coded differently than those that come after to capture the structure of concepts in a relationship.We used the Mallet CRF implementation for our tasks. The CRF performed quite well in this task, labelling most of the relation phrases correctly. Even though it had many false positives there are no false negatives and all the phrases categorized as ‘None’ had a 100% precision as seen in the table below.Label N Correct Returned P R F1None 980 966 966 1 0. 985 0.993Relation 40 40 54 0.740 1.0 0.851Testing Accuracy: 0.9861006 out of 1020 instances correctly labelledTable 1: Binary classification of relationships5.2 Segmentation of Relation PhrasesWe designed this experiment to segment each relationship triple from the entire corpus. The relationship triple consisted of simple phrases like ‘P53 gene affects cancer’. In this example‘P53 gene’ and ‘cancer’ are concepts and the experiment labels the entire phrase as a relationship.We label the relation phrases in the training data using a ‘B’ tag to indicate the start of a relationship, ‘I’ tag to indicate following phrases in the relationship and a ‘O’ tag to mark all other non relationship phrases. The model used to learn this segmentation is a factorial CRF with one of the linear CRF chains learning to label the relations and the other linear CRF to learn the segmentation.The advantage of this model which simultaneously discovers both the labels and the segmentation in comparison with an algorithm which uses the label to segment is that error does not propagate from one step to the next. The errors in the labelling step do not lead to errors in the segmentation.RPF1ReturnedLabel NCorrect(Start) 21 8 11 0.727 0.381 0.5B(Intermediate) 52 15 24 0.652 0.288 0.399I(Other) 591 582 630 0.953 0.984 0.953OTable 2: Segmentation of relationship triplesThe results of the experiment show that the system does learn to an extent to segment the relation phrases with a high precision. The low recall is mostly because of the sparse data and should improve if a larger amount of data is used to train the CRF.5.3 Relation ExtractionThe experiment uses a linear chain CRF to label each individual interesting relationship in the test corpus. The features were extracted from 100 abstracts and used for training. The features consisted of the POS tags, concepts, semantic types and the actual phrase. The noun phrase for the concepts can occur a few phrases before or after the relation verb.Label NReturnedP R F1Correct1.00.976884None 863863brings_about 2 1 1 1.0 0.5 0.6660.2750.190OTHER 21 4 8 0.5Performs 0 0 0 1.0 1.0 1.0Analyzes 2 0 0 1.0 0.0 0.0Causes 1 0 0 1.0 0.0 0.0Disrupts 0 0 0 1.0 1.0 1.0part_of 1 0 0 1.0 0.0 0.0Exhibits 2 2 2 1.0 1.0 1.0Traverses 0 0 0 1.0 1.0 1.0Uses 1 0 0 1.0 0.0 0.0location_of 0 0 0 1.0 1.0 1.0Accuracy: 0.972 Correct 870 out of 895 Joint accuracy: 0.972Table 3: Relationship labellingThe experiment extracted a few relations well from the test set. The sparseness and skew of the training data might account for it not being able to learn the low frequency relations but the relations with a larger number of examples in the training seem to work well. Another way that the efficiency of this algorithm might be hampered is if the concepts discovered in the mining stage are not general enough.6. Future WorkMany interesting experiments can be done to improve the overall efficiency of the relation discovery task of our system. Instead of giving the actual index of the relative position we can specify only if the concept came before or after a verb phrase. The NLP based relation extractor can be further improved to capture more complex relationship for training the CRF and we can use models such as skip CRF and relational markov networks to learn more complex relationships.7 ConclusionLinear chain CRF was used for labelling relations in a corpus. The experiment discovered a few relations and should perform much better with a larger training corpus. The task of binary classification of a relation and a non-relation phrase performed well with high precision and recall values. The suggestions listed in the future work can be used to further improve the experiment results.Referencesfferty, J., McCallum, A., Pereira, F.: Conditional random fields: Probabilistic models forsegmenting and labeling sequence data. In: Proc. 18th International Conf. on Machine Learning,Morgan Kaufmann, San Francisco, CA (2001) 282–2892.Dynamic Conditional Random Fields for Jointly Labeling Multiple Sequences. AndrewMcCallum, Khashayar Rohanimanesh, and Charles Sutton. In NIPS Workshop on Syntax,Semantics, and Statistics. December 2003.3.Collective Segmentation and Labeling of Distant Entities in Information Extraction. CharlesSutton and Andrew McCallum. In ICML Workshop on Statistical Relational Learning and ItsConnections to Other Fields. 2004.4.Dynamic Conditional Random Fields: Factorized Probabilistic Models for Labeling andSegmenting Sequence Data. Charles Sutton, Khashayar Rohanimanesh, and Andrew McCallum.In International Conference on Machine Learning (ICML). 2004.5.Dynamic Conditional Random Fields: Factorized Probabilistic Models for Labeling andSegmenting Sequence Data. Charles Sutton, Andrew McCallum, and Khashayar Rohanimanesh.Journal of Machine Learning Research. vol. 8. March 2007. pp. 693--723.6.Statistical Relational Learning for Natural Language Information Extraction.Razvan Bunescu and Raymond J. MooneyStatistical Relational Learning, Lise Getoor and Ben Taskar (Eds.) book, 2005.7.Benjamin Taskar, Pieter Abbeel, and D. Koller. Discriminative probabilistic models for relationaldata. In Proceedings of 18th Conference on Uncertainty in Artificial Intelligence (UAI-2002),pages 485–492, Edmonton, Canada, 2002.8.Craven, M. and Kumlien, J. 1999. Constructing Biological Knowledge Bases by ExtractingInformation from Text Sources. In Proceedings of the Seventh international Conference onintelligent Systems For Molecular Biology (August 06 - 10, 1999). T. Lengauer, R. Schneider, P.Bork, D. L. Brutlag, J. I. Glasgow, H. Mewes, and R. Zimmer, Eds. AAAI Press, 77-86.9.Bin Zhao, Prithviraj Sen, Lise Getoor, 2006. Entity and Relationship Labeling in AffiliationNetworks. ICML Workshop on Statistical Network Analysis, 200610.Domain Ontology Construction from Biomedical Text, S Sahay, B Li, EV Garcia, E Agichtein, ARam. International Conference on Artificial Intelligence (ICAI-07).11./12.L. Smith, T. Rindflesch, and W. J. Wilbur. Medpost: a part-of-speech tagger for biomedical text.Bioinformatics, 20(14), 2004.。