Corpus-based and Knowledge-based Measures of Text Semantic Similarity
A New Academic Word List

A New Academic Word ListAVERIL COXHEADVictoria University of WellingtonWellington, New ZealandThis article describes the development and evaluation of a new aca-demic word list (Coxhead, 1998), which was compiled from a corpus of3.5 million running words of written academic text by examining therange and frequency of words outside the first 2,000 most frequentlyoccurring words of English, as described by West (1953). The AWLcontains 570 word families that account for approximately 10.0% of thetotal words (tokens) in academic texts but only 1.4% of the total wordsin a fiction collection of the same size. This difference in coverageprovides evidence that the list contains predominantly academic words.By highlighting the words that university students meet in a wide rangeof academic texts, the AWL shows learners with academic goals whichwords are most worth studying. The list also provides a useful basis forfurther research into the nature of academic vocabulary.O ne of the most challenging aspects of vocabulary learning and teaching in English for academic purposes (EAP) programmes is making principled decisions about which words are worth focusing on during valuable class and independent study time. Academic vocabulary causes a great deal of difficulty for learners (Cohen, Glasman, Rosenbaum-Cohen, Ferrara, & Fine, 1988) because students are generally not as familiar with it as they are with technical vocabulary in their own fields and because academic lexical items occur with lower frequency than general-service vocabulary items do (Worthington & Nation, 1996; Xue & Nation, 1984).The General Service List (GSL) (West, 1953), developed from a corpus of 5 million words with the needs of ESL/EFL learners in mind, contains the most widely useful 2,000 word families in English. West used a variety of criteria to select these words, including frequency, ease of learning, coverage of useful concepts, and stylistic level (pp. ix–x). The GSL has been criticised for its size (Engels, 1968), age (Richards, 1974), and need for revision (Hwang, 1989). Despite these criticisms, the GSL covers up to 90% of fiction texts (Hirsh, 1993), up to 75% of nonfiction texts (Hwang, 1989), and up to 76% of the Academic Corpus (Coxhead,213 TESOL QUARTERLY Vol. 34, No. 2, Summer 20001998), the corpus of written academic English compiled for this study. There has been no comparable replacement for the GSL up to now.Academic words (e.g., substitute, underlie, establish, inherent) are not highly salient in academic texts, as they are supportive of but not central to the topics of the texts in which they occur. A variety of word lists have been compiled either by hand or by computer to identify the most useful words in an academic vocabulary. Campion and Elley (1971) and Praninskas (1972) based their lists on corpora and identified words that occurred across a range of texts whereas Lynn (1973) and Ghadessy (1979) compiled word lists by tracking student annotations above words in textbooks. All four studies were developed without the help of computers. Xue and Nation (1984) created the University Word List (UWL) by editing and combining the four lists mentioned above. The UWL has been widely used by learners, teachers, course designers, and researchers. However, as an amalgam of the four different studies, it lacked consistent selection principles and had many of the weaknesses of the prior work. The corpora on which the studies were based were small and did not contain a wide and balanced range of topics.An academic word list should play a crucial role in setting vocabulary goals for language courses, guiding learners in their independent study, and informing course and material designers in selecting texts and developing learning activities. However, given the problems with cur-rently available academic vocabulary lists, there is a need for a new academic word list based on data gathered from a large, well-designed corpus of academic English. The ideal word list would be divided into smaller, frequency-based sublists to aid in the sequencing of teaching and in materials development. A word list based on the occurrence of word families in a corpus of texts representing a variety of academic registers can provide information about how words are actually used (Biber, Conrad, & Reppen, 1994).The research reported in this article drew upon principles from corpus linguistics (Biber, Conrad, & Reppen, 1998; Kennedy, 1998) to develop and evaluate a new academic word list. After discussing issues that arise in the creation of a word list through a corpus-based study, I describe the methods used in compiling the Academic Corpus and in developing the AWL. The next section examines the coverage of the AWL relative to the complete Academic Corpus and to its four discipline-specific subcorpora. To evaluate the AWL, I discuss its coverage of (a) the Academic Corpus along with the GSL (West, 1953), (b) a second collection of academic texts, and (c) a collection of fiction texts, and compare it with the UWL(Xue & Nation, 1984). In concluding, I discuss the list’s implications for teaching and for materials and course design, and I outline future research needs.214TESOL QUARTERLYTHE DEVELOPMENT OF ACADEMIC CORPORAAND WORD LISTSTeachers and materials developers who work with vocabulary lists often assume that frequently occurring words and those which occur in many different kinds of texts may be more useful for language learners to study than infrequently occurring words and those whose occurrences are largely restricted to a particular text or type of text (Nation, in press; West, 1953). Given the assumption that frequency and coverage are important criteria for selecting vocabulary, a corpus, or collection of texts, is a valuable source of empirical information that can be used to examine the language in depth (Biber, Conrad, & Reppen, 1994). However, exactly how a corpus should be developed is not clear cut. Issues that arise include the representativeness of the texts of interest to the researcher (Biber, 1993), the organization of the corpus, its size (Biber, 1993; Sinclair, 1991), and the criteria used for word selection. RepresentationResearch in corpus linguistics (Biber, 1989) has shown that the linguistic features of texts differ across registers. Perhaps the most notable of these features is vocabulary. To describe the vocabulary of a particular register, such as academic texts, the corpus must therefore contain texts that are representative of the varieties of texts they are intended to reflect (Atkins, Clear, & Ostler, 1992; Biber, 1993; Sinclair, 1991). Sinclair (1991) warns that a corpus should contain texts whose sizes and shapes accurately reflect the texts they represent. If long texts are included in a corpus, “peculiarities of an individual style or topic occasionally show through” (p. 19), particularly through the vocabulary. Making use of a variety of short texts allows more variation in vocabulary (Sutarsyah, Nation, & Kennedy, 1994). Inclusion of texts written by a variety of writers helps neutralise bias that may result from the idiosyn-cratic style of one writer (Atkins et al., 1992; Sinclair, 1991) and increases the number of lexical items in the corpus (Sutarsyah et al., 1994).Scholars who have compiled corpora have attempted to include a variety of academic texts. Campion and Elley’s (1971) corpus consisted of 23 textbooks, 19 lectures published in journals, and a selection of university examination papers. Praninskas (1972) used a corpus of 10first-year, university-level arts and sciences textbooks that were required reading at the American University of Beirut.Lynn (1973) and Ghadessy (1979) both focussed on textbooks used in their universities. Lynn’s corpus included 52 textbooks and 4 classroom handouts from 50 A NEW ACADEMIC WORD LIST215students of accounting, business administration, and economics from which 10,000 annotations were collected by hand. The resulting list contained 197 word families arranged from those occurring the most frequently (39 times) to those occurring the least frequently. Words occurring fewer than 10 times were omitted from the list (p. 26). Ghadessy compiled a corpus of 20 textbooks from three disciplines (chemistry, biology, and physics). Words that students had glossed were recorded by hand, and the final list of 795 items was then arranged in alphabetical order (p. 27). Relative to this prior work, the corpus compiled for the present study considerably expands the representation of academic writing in part by including a variety of academic sources besides textbooks.OrganizationA register such as academic texts encompasses a variety of subregisters. An academic word list should contain an even-handed selection of words that appear across the various subject areas covered by the texts contained within the corpus. Organizing the corpus into coherent sections of equal size allows the researcher to measure the range of occurrence of the academic vocabulary across the different disciplines and subject areas of the corpus. Campion and Elley (1971) created a corpus with 19 academic subject areas, selecting words occurring outside of the first 5,000 words of Thorndike and Lorge’s (1944) list and excluding words encountered in only one discipline (p. 7). The corpus for the present study involved 28 subject areas organised into 7 general areas within each of four disciplines: arts, commerce, law, and science. SizeA corpus designed for the study of academic vocabulary should be large enough to ensure a reasonable number of occurrences of academic words. According to Sinclair (1991), a corpus should include millions of running words (tokens) to ensure that a very large sample of language is available (p. 18).1 The exact amount of language required, of course, depends on the purpose and use of the research; however, in general more language means that more information can be gathered about lexical items and more words in context can be examined in depth.1The term running words (or tokens) refers to the total number of word forms in a text, whereas the term individual words (types) refers to each different word in a text, irrespective of how many times it occurs.216TESOL QUARTERLYIn the past, researchers attempted to work with academic corpora by hand, which limited the numbers of words they could analyze.Campion and Elley (1971), in their corpus of 301,800 running words, analysed 234,000 words in textbooks, 57,000 words from articles in journals, and 10,800 words in a number of examination papers (p. 4). Praninskas’s (1972) corpus consisted of approximately 272,000 running words (p. 8), Lynn (1973) examined 52 books and 4 classroom handouts (p. 26), and Ghadessy (1979) compiled a corpus of 478,700 running words. Praninskas (1972) included a criterion of range in her list and selected words that were outside the GSL (West, 1953).In the current study, the original target was to gather 4.0 million words; however, time pressures and lack of available texts limited the corpus to approximately 3.5 million running words. The decision about size was based on an arbitrary criterion relating to the number of occurrences necessary to qualify a word for inclusion in the word list: If the corpus contained at least 100 occurrences of a word family, allowing on average at least 25 occurrences in each of the four sections of the corpus, the word was included. Study of data from the Brown Corpus (Francis & Kucera, 1982) indicated that a corpus of around 3.5 million words would be needed to identify 100 occurrences of a word family. Word SelectionAn important issue in the development of word lists is the criteria for word selection, as different criteria can lead to different results. Re-searchers have used two methods of selection for academic word lists. As mentioned, Lynn (1973) and Ghadessy (1979) selected words that learners had annotated regularly in their textbooks, believing that the annotation signalled difficulty in learning or understanding those words during reading. Campion and Elley (1971) selected words based on their occurrence in 3 or more of 19 subject areas and then applied criteria, including the degree of familiarity to native speakers. However, the number of running words in the complete corpus was too small for many words to meet the initial criterion. Praninskas (1972) also included a criterion of range in her list; however, the range of subject areas and number of running words was also small, resulting in a small list without much variety in the words.Another issue that arises in developing word lists is defining what to count as a word. The problem is that lexical items that may be morphologically distinct from one another are, in fact, strongly enough related that they should be considered to represent a single lexical item. To address this issue, word lists for learners of English generally group words into families (West, 1953; Xue & Nation, 1984). This solution is A NEW ACADEMIC WORD LIST217supported by evidence suggesting that word families are an important unit in the mental lexicon (Nagy, Anderson, Schommer, Scott, & Stallman, 1989, p. 262). Comprehending regularly inflected or derived members of a family does not require much more effort by learners if they know the base word and if they have control of basic word-building processes (Bauer & Nation, 1993, p. 253). In the present study, therefore, words were defined through the unit of the word family, as illustrated in Table 1.For the creation of the AWL, a word family was defined as a stem plus all closely related affixed forms, as defined by Level 6 of Bauer and Nation’s (1993) scale. The Level 6 definition of affix includes all inflections and the most frequent, productive, and regular prefixes and suffixes (p. 255). It includes only affixes that can be added to stems that can stand as free forms (e.g., specify and special are not in the same word family because spec is not a free form).Research QuestionsThe purpose of the research described here was to develop and evaluate a new academic word list on the basis of a larger, more principled corpus than had been used in previous research. Two questions framed the description of the AWL:1.Which lexical items occur frequently and uniformly across a widerange of academic material but are not among the first 2,000 words of English as given in the GSL (West, 1953)?2.D o the lexical items occur with different frequencies in arts, com-merce, law, and science texts?TABLE 1Sample Word Families From the Academic Word Listconcept legislate indicateconception legislated indicatedconcepts legislates indicatesconceptual legislating indicatingconceptualisation legislation indicationconceptualise legislative indicationsconceptualised legislator indicativeconceptualises legislators indicatorconceptualising legislature indicatorsconceptuallyNote. Words in italics are the most frequent form in that family occurring in the Academic Corpus.218TESOL QUARTERLYThe evaluation of the AWL considered the following questions:3.What percentage of the words in the Academic Corpus does the AWLcover?4.Do the lexical items identified occur frequently in an independentcollection of academic texts?5.How frequently do the words in the AWL occur in nonacademictexts?6.How does the AWL compare with the UWL (Xue & Nation, 1984)? METHODOLOGYThe development phase of the project identified words that met the criteria for inclusion in the AWL (Research Questions 1 and 2). In the evaluation phase, I calculated the AWL’s coverage of the original corpus and compared the AWL with words found in another academic corpus, with those in a nonacademic corpus, and with another academic word list (Questions 3–6).Developing the Academic CorpusD eveloping the corpus involved collecting each text in electronic form, removing its bibliography, and counting its words. After balancing the number of short, medium-length, and long texts (see below for a discussion on the length of texts), each text was inserted into its subject-area computer file in alphabetical order according to the author’s name. Each subject-area file was then inserted into a discipline master file, in alphabetical order according to the subject. Any text that met the selection criteria but was not included in the Academic Corpus because its corresponding subject area was complete was kept aside for use in a second corpus used to test the AWL’s coverage at a later stage. The resulting corpus contained 414 academic texts by more than 400 authors, containing 3,513,330 tokens (running words) and 70,377 types (individual words) in approximately 11,666 pages of text. The corpus was divided into four subcorpora: arts, commerce, law, and science, each containing approximately 875,000 running words and each subdivided into seven subject areas (see Table 2).The corpus includes the following representative texts from the academic domain: 158 articles from academic journals, 51 edited aca-demic journal articles from the World Wide Web, 43 complete university textbooks or course books, 42 texts from the Learned and Scientific section of the Wellington Corpus of Written English (Bauer, 1993), 41 A NEW ACADEMIC WORD LIST219220TESOL QUARTERLY texts from the Learned and Scientific section of the Brown Corpus (Francis & Kucera, 1982), 33 chapters from university textbooks, 31 texts from the Learned and Scientific section of the Lancaster-Oslo/Bergen (LOB) Corpus (Johansson, 1978), 13 books from the Academic Texts section of the MicroConcord academic corpus (Murison-Bowie, 1993),and 2 university psychology laboratory manuals.The majority of the texts were written for an international audience.Sixty-four percent were sourced in New Zealand, 20% in Britain, 13% in the United States, 2% in Canada, and 1% in Australia. It is difficult to say exactly what influence the origin of the texts would have on the corpus,for even though a text was published in one country, at least some of the authors may well have come from another.The Academic Corpus was organized to allow the range of occurrence of particular words to be examined. Psychology and sociology texts were placed in the arts section on the basis of Biber’s (1989) finding that texts from the social sciences (psychology and sociology) shared syntactic characteristics with texts from the arts (p. 28). Lexical items may well pattern similarly. Placing the social science subject areas in the science section of the Academic Corpus might have introduced a bias: The psychology and sociology texts might have added lexical items that do not occur in any great number in any other subject in the science section. The presence of these items, in turn, would have suggested that science and arts texts share more academic vocabulary items than is generally true.With the exception of the small number of texts from the Brown (Francis & Kucera, 1982), LOB (Johansson, 1978), and Wellington TABLE 2Composition of the Academic Corpus DisciplineArtsCommerce Law Science Total Running 883,214879,547874,723875,846351,333wordsTexts122 107 72 113 414Subject Education Accounting Constitutional Biology areas History Economics Criminal Chemistry Linguistics Finance Family and Computer science Philosophy Industrial medicolegal Geography Politics relations International Geology Psychology Management Pure commercial Mathematics Sociology Marketing Quasi-commercial Physics Public policy Rights and remedies(Bauer, 1993) corpora, the texts in the Academic Corpus were complete. The fact that frequency of occurrence of words was only one of the criteria for selecting texts minimized any possible bias from word repetition within longer texts. To maintain a balance of long and short texts, the four main sections (and, within each section, the seven subject areas) each contained approximately equal numbers of short texts (2,000–5,000 running words), medium texts (5,000–10,000 running words), and long texts (more than 10,000 running words). The break-down of texts in the four main sections was as follows: arts—18 long, 35 medium; commerce—18 long, 37 medium; law—23 long, 22 medium; and science—19 long, 37 medium.Developing the Academic Word ListThe corpus analysis programme Range (Heatley & Nation, 1996) was used to count and sort the words in the Academic Corpus. This programme counts the frequency of words in up to 32 files at a time and records the number of files in which each word occurs (range) and the frequency of occurrence of the words in total and in each file.Words were selected for the AWL based on three criteria:1.Specialised occurrence: The word families included had to be outsidethe first 2,000 most frequently occurring words of English, as represented by West’s (1953) GSL.2.Range: A member of a word family had to occur at least 10 times ineach of the four main sections of the corpus and in 15 or more of the28 subject areas.3.Frequency: Members of a word family had to occur at least 100 times inthe Academic Corpus.Frequency was considered secondary to range because a word count based mainly on frequency would have been biased by longer texts and topic-related words. For example, the Collins COBUILD Dictionary (1995) highlights Yemeni and Lithuanian as high-frequency words, probably because the corpus on which the dictionary is based contains a large number of newspapers from the early 1990s.The conservative threshold of a frequency of 100 was applied strictly for multiple-member word families but not so stringently for word families with only one member, as single-member families operate at a disadvantage in gaining a high frequency of occurrence. In the Aca-demic Corpus, the word family with only one member that occurs the least frequently is forthcoming (80 occurrences).A NEW ACADEMIC WORD LIST221RESULTSDescriptionOccurrence of Academic WordsThe first research question asked which lexical items beyond the first 2,000 in West’s (1953) GSL occur frequently across a range of academic texts. In the Academic Corpus, 570 word families met the criteria for inclusion in the AWL (see Appendix A). Some of the most frequent word families in the AWL are analyse, concept, data, and research. Some of the least frequent are convince, notwithstanding, ongoing, persist, and whereby. Differences in Occurrence of Words Across DisciplinesThe second question was whether the lexical items selected for the AWL occur with different frequencies in arts, commerce, law, and science texts. The list appears to be slightly advantageous for commerce students, as it covers 12.0% of the commerce subcorpus. The coverage of arts and of law is very similar (9.3% and 9.4%, respectively), and the coverage of science is the lowest among the four disciplines (9.1%). The 3.0% difference between the coverage of the commerce subcorpus and the coverage of the other three subcorpora may result from the presence of key lexical items such as economic, export, finance, and income, which occur with very high frequency in commerce texts. (See Appendix B for excerpts from texts in each section of the Academic Corpus.) The words in the AWL occur in a wide range of the subject areas in the Academic Corpus. Of the 570 word families in the list, 172 occur in all 28 subject areas, and 263 (172 + 91) occur in 27 or more subject areas (see Table 3). In total, 67% of the word families in the AWL occur in 25 or more of the 28 subject areas, and 94% occur in 20 or more. EvaluationCoverage of the Academic Corpus Beyond the GSLThe AWL accounts for 10.0% of the tokens in the Academic Corpus. This coverage is more than twice that of the third 1,000 most frequent words, according to Francis and Kucera’s (1982) count, which cover 4.3% of the Brown Corpus. Taken together, the first 2,000 words in West’s (1953) GSL and the word families in the AWL account for approximately 86% of the Academic Corpus (see Table 4). Note that the AWL’s coverage of the Academic Corpus is double that of the second 222TESOL QUARTERLY1,000 words of the GSL. The AWL and the GSL combined have a total of 2,550 word families, and all but 12 of those in the GSL occur in the Academic Corpus.The AWL, the first 1,000 words of the GSL (West, 1953), and the second 1,000 words of the GSL cover the arts, commerce, and law subcorpora similarly but in very different patterns (see Table 5). The first 1,000 words of the GSL account for fewer of the word families in the commerce subcorpus than in the arts and law subcorpora, but this lower coverage of commerce is balanced by the AWL’s higher coverage of this discipline. On the other hand, the AWL’s coverage of the arts and law subcorpora is lower than its coverage of the commerce subcorpus, but the GSL’s coverage of arts and law is slightly higher than its coverage of commerce. The AWL’s coverage of the science subcorpus is 9.1%, which indicates that the list is also extremely useful for science students. The GSL, in contrast, is not quite as useful for science students as it is for arts,commerce, and law students.Subject-Area Coverage of Word Families in the Academic Word List No. of Subject areas in No. of Subject areas in word familieswhich they occurred word families which they occurred 1722820219127152058269196225918432451743235163322415Note. Total subject areas = 28; total word families = 570.TABLE 4Coverage of the Academic Corpus by the Academic Word Listand the General Service List (West, 1953)No. of word familiesCoverage of In Academic Word listAcademic Corpus (%)Total Corpus Academic Word List10.0570570General Service List First 1,000 words 71.41,0011,000Second 1,000 words4.7979968Total 86.12,5502,538Coverage of Another Academic CorpusA frequency-based word list that is derived from a particular corpus should be expected to cover that corpus well. The real test is how the list covers a different collection of similar texts. To establish whether the AWL maintains high coverage over academic texts other than those in the Academic Corpus, I compiled a second corpus of academic texts in English, using the same criteria and sources to select texts and dividing them into the same four disciplines. This corpus comprised approxi-mately 678,000 tokens (82,000 in arts, 53,000 in commerce, 143,000 in law, and 400,000 in science) representing 32,539 types of lexical items.This second corpus was made up of texts that had met the criteria for inclusion in the Academic Corpus but were not included either because they were collected too late or because the subject area they belonged to was already complete.The AWL’s coverage of the second corpus is 8.5% (see Table 6), and all 570 word families in the AWL occur in the second corpus. The GSL’s coverage of the second corpus (66.2%) is consistent with its coverage of the science section of the Academic Corpus (65.7%). The overall lower coverage of the second corpus by both the AWL and the GSL (79.1%)seems to be partly the result of the large proportion of science texts it contains.Coverage of Nonacademic TextsTo establish that the AWL is truly an academic word list rather than a general-service word list, I developed a collection of 3,763,733 running words of fiction texts. The collection consisted of 50 texts from Project Gutenberg’s () collection of texts that were written more than 50 years ago and are thus in the public domain. The Coverage of the Four Subcorpora of the Academic Corpus by the General Service List (West, 1953) and the Academic Word List (%)General Service ListAcademic First 1,000Second 1,000SubcorpusWord List words words Total Arts9.373.0 4.486.7Commerce12.071.6 5.288.8Law9.475.0 4.188.5Science 9.165.7 5.079.8。
TheApplicationofCorpus―basedLexicalChunksApproachinEnglishTeaching

TheApplicationofCorpus―basedLexicalChunksApproachinEnglishTeaching【Abstract】With the guidance of lexical chunks teaching approach,this article is to discuss the application ofcorpus-based lexical chunks approach in English teaching by making use of corpus concordance analysis.After comparing the language differences between native language corpus and target language corpus,it advocates that the emphasis of English lexical study should be put on corpus-based study of chunks,aiming to promote the fluency and authenticity of language output in English teaching.【Key words】chunks;corpus;English teachingIntroductionLexical study lies not only in the expansion of vocabulary but also in the extension of its range and depth.As for the frequently-used words,mastering the spelling、pronunciation、and meaning is only the tip of iceberg for lexical acquisition.Mainly the traditional lexical teaching ismeaning-driven in our country,therefore,in order to enlarge their vocabulary,students just memorize words andexpressions with the Chinese meaning accordingly instead ofthe interior characteristics when they occur.They neglect the depth development of vocabulary,which results in the failure of using vocabulary even after several years of English learning.In these days,more and more researchers have noticed the importance of vocabulary depth.According to Becker in 1975,to input and output language is not based on using one single word,but making use of chunks that prefabricate in the brain of human.The chunks are the smallest unit when communication takes place.The research of chunks plays a critical role in guiding foreign language teaching.So how to promote learners’language output with the help of corpus-based study of chunks?In this article,by comparing and analyzing the language output differences between native language users and target language learners,corpus concordance analysis will be used for empirical research in the language teaching,so as to promote fluent and authentic language when conducting teaching activities.1.Chunks Approach TheoryChunks namely are combination of words.The definition of chunks given by Pu Jianzhong is that:Chunk is a poly-wordsunit which is specifically structured,conveys a certain meaning,prefabricates in human’s brain and can be frequentlyused.They include idioms、expressions and collocations as well.According to Lewis,chunks usually refer to two or more words combination that are seen as wholes or chunks without further analysis.They are raw materials for language learning,which can be observed and noticed by learners to acquire the language pattern. Traditional lexical teaching places great emphasis on the pronunciation、spelling and its main usage of a new word by explaining and illustrating it.This is totally teacher-centered.Thus when reading an English article,learners often fall into a puzzle:It’s easy to understand every single word in a sentence,including its part of speech、spelling、pronunciation and meaning;when it comes to the understanding of the whole sentence combined by these words,it becomes rather difficult.Many factors are responsible for this understanding difficulty,such as several meanings of a word,the parts of speech,and one important factor lies in chunks.To some extent,being unable to recognize the chunk combined by several words,and trying to separate the chunk when making comprehension definitely hinder the understanding for the text.This comprehension difficulty and slow reading speed isdirectly or indirectly relevant to learners’ability of mastering chunks.This is also the case in English writing.That’s why Chinese learners make the phrases like “learn knowledge”、“enter society”、“reach all aims”.In the work Lexical Approach Lewis concluded that:“Language consists of grammaticalized lexis not lexicalized grammar.”This conclusion overturned the traditional concept that language consists in both grammar and lexis,thus established lexis as the foundation of language.Lexis are not separated from grammar,but are integrated into a bigger phrase---chunk under the guidance of specific grammar and semantics.Therefore,the emphasis of foreign language teaching and learning should be put on the chunks that occur frequently in language communication.By means of chunks approach,we can put the active words into the context and give a thorough consideration to the situation,then these chunks can be noticed and learned appropriately.2.Corpus and The Lexical Chunks ApproachA corpus is a collection of naturally-occurring language text,chosen to characterize a state or variety of a language.(Sinclair 1991:171)and a collection of machine-readable authentic text which is sampled to be representative of a particularlanguage or language variety.(McEnery and Wilson 2006:5).In short,a corpus is a large collection of written or spoken texts that is used for language research.With the superiority of its large capacity、authentic language materials、quick and accurate concordance,etc.corpus plays a significant role in language teaching and learning. Firstly,it’s beneficial to develop learners’intelligence when using corpus to observe and analyze language materials.Because of the large amounts of authentic examples and contexts presenting in front of learners,corpus makes learners’attention span last longer,which is helpful to enhance memory、acquire the understanding and summarize regular patterns.Secondly,the application of corpus in English teaching and learning is helpful to students’autonomous learning model,and it will activate students’language consciousness and sensitivity.The model ofdata-driven learning offers a presupposition for induction learning strategy,for effective language learning is a process of exploring the language.Corpus can promote the lexical chunks study mainly through concordance.Corpus concordance analysis is the most basic analysis means for corpus.The most common form is KWIC (Key Words in Context).After setting up the node word,thecomputer will make use of concordance tool to search for the sentences that include the node word,and present them in the form of placing the node word in the middle.This may range from several words beside the node word to the whole sentence or paragraph which contains the node word.As we can see it in this way,corpus concordance not only can assist learners with noticing the node word’s chunk model,but also provide different contexts for corresponding chunks.Hence,learners can obtain good understanding and the authentic usage of vocabulary in real situation.Learners can achieve data-driven learning by making use of corpus concordance analysis as well,which embodies students-centered learning.To select the frequently-used vocabulary from large-scale raw corpus and to acquire the most basic usage and most common collocation of them can make students truly understand and master the authentic way of using vocabulary.Therefore,after making use of the characteristics comparison analysis between native language and target language using,we can gain the analysis result and then take advantage of it to guide language learning and improve classroom teaching.In a certain degree,corpus-based study of lexical chunks can compare favorably withnatural-surroundings language learning in terms of learning effectiveness.3.The App lication of Corpus―based Lexical Chunks Approach in English Teaching3.1 Corpus-based lexical chunks approach and teachers’language teaching.According to the traditional foreign language teaching in our country,the lexical teaching is just to teach students the single word,which usually results in the separation of “wood”and “forest”.Meanwhile,there is a connection between a word and others from the aspects of pronunciation,form and meaning.This connection will be neglected if we simply learn a single word separately.Nowadays,we can get a quick access to all authentic examples of a word or a phrase by means of modern computer technology,and add up the occurring frequency of a certain word or phrase.In this case,teachers themselves can establish an accurate and overall relationship among words,and acquire the meaning and usage of every language in practical communication.Let’s take the noun “survey”for examplemonly-used dictionaries all list out the collocation of “make a (general)survey of”.But after we search for “survey”and “surveys”as the key word in the corpus,thecollocation between “make”and “survey”never occurs.The search result shows that the most frequent collocation is conduct/carry out a survey,and more passive forms are used than active forms.Traditional English textbook is grammar-structured,but the contemporary communication techniques aims for practical use when conducting language teaching.So the lexis,grammar and topics are categorized in terms of their frequency in the process of real language using.Before the use of corpus,it’s rather tough and complicated to put the frequency in order;but this job becomes easy and accurate with the assistance of corpus.For instance,in traditional teaching to modals,it’s usually thought that the order of them should be put like “can?Cmay?Cmust?Cshall?Cwill?C should?Cwould”.While after searching for them in national English corpus,we get a nearly opposite order from the above.The result indicates that,the most common modal in English is “would”rather than “can”.So if we give some consideration to the real distribution of these words when composing textbook and designing teaching plan,we can teach the most common words firstly and help students to learn and use them frequently in a nativeway.It’s better to arouse students motivation for study and connect language learning with language communication firmly.In brief,teachers can use language from native speakers’corpus as the natural materials,and conduct language teaching activities under the guidance of lexical chunks approach.The effects of this approach are embodies as the following:Firstly,lexical chunks approach can reduce the differences between the language that is taught in classroom and the language which is used by native speakers.What’s more,the using of authentic and vivid language is persuasive to arouse learners’learning interest.In the second place,with the help of corpus concordance tool,we can easily search for the chunks’examples in great detail,which paves a shortcut for key words study and research.The search result will present the contexts,which are displayed for students to observe and distinguish the target chunks and understand their typical usages as well.From the above,we can see it clearly that corpus-based lexical chunks approach enables teachers to get a quick access to the high frequency chunks,and offer students the authentic and comprehensible materials,then develop their ability to communicate in real and native English.This is a model of“observation-selection-drill”,which is different from the traditional one “illustration-practice”.This approach gives a complete consideration for the key context and abstracts the most common use and pattern of a certain word in the form of chunk.As a result,it definitely will optimize the lexical teaching.3.2 Corpus-based lexical chunks approach and students’autonomous language learning.Corpus is helpful for students to cultivate their ability of autonomous language learning,for it can be used to achieve the transfer from “learn”to “know how to learn”.During the English teaching in the past,the common phenomenon was that teachers summarized the regular patterns of the language,and students wrote them down onto their notebooks,then memorized them mechanically to “utilize”them in examination.If corpus can be fully used,students themselves can search for the examples of a certain word or a structure by making use of the corpus concordance skills,and study these examples to discover and induce the language laws by themselves.We can establish a brand-new model of autonomous language learning with the help of corpus,especially for the learning of lexical chunks.We can analyze the demands of learners according to their individual characteristicsanalysis.After doing this analysis,we can choose the language learning materials that fits the individual from the corpus,and build up a language learning pattern specifically for himself.Then the learning effectiveness will be assessed after students have the language learning finished.The autonomous learning and its result will be reflected among the table of their characteristics and learners’corpus,which is meant to make students’autonomous learning becoming closer to their demands.In this way,the learners-centered model is built and students’ability of autonomous learning will be easy to acquire.The core of implementing corpus teaching scheme is to give students’opportunity of exploring and finding the language regular patterns,and to discuss them in awell-organized class.The implementation of corpus-based lexical chunks approach requires teachers to shift their roles in teaching.A good teacher is not only a lecturer who shares knowledge but also a guide and companion who helps students with their discovery for knowledge,and gradually guide students to adapt themselves to this new study model,then make students shift from memorizing knowledge passively and mechanically to discovering and absorbing authentic and activelanguage from corpus by themselves.4.ConclusionCorpus is an expansion of existing English teaching resources.Corpus-based lexical chunks approach is beneficial both to the efficiency and effectiveness of vocabulary study,and it will do good to the promotion of vocabulary expansion and extension.Applying corpus into English lexis teaching not only offers students large amounts of authentic language materials,but also enables them to acquire knowledge with proper situations and get quick access to various collocations dynamically,which in return benefits the teacher to design data-driven learning tasks. There are two systematic projects to be done if we want to enhance corpus-based lexical chunks approach.Firstly,a further research is needed to study the corpus-based lexical chunks involved in the course requirement,in order to establish a complete learning material data.In the second place,we should reinforce the study for lexical chunks’strategies and approaches.If we want to transfer a lexical chunk from the external to the internal,make it become learners’ability,the language learner should be a skillful person who can realize and apply the law of repetition,connection and assimilation.Meanwhile,we must notice that there are different concordance offered by network corpus,so it’s necessary to use them integratedly.In addition,for the students who learn English at their beginning or just for a short time,the teacher should arrange some periods for them to get familiar with the use of corpus.By this means,students can master how to use corpus concordance,which will facilitate the review and search for the lexical chunks occurred in the textbook.References:[1]Lewis Michael.The Lexical Approach[M].Hove:Language Teaching Publication,1993.[2]Sinelair J,Renouf A.A lexical syllabus for language learning[A].Carter R&M.McCarthy(eds.).Vocabulary and Language Teaching[C].London:Longman,1998.[3]Sinclair J.Corpus,concordance,collocation[M].Oxford:Oxford University Press.1991.[4]Stubbs C.The State of the Art in CorpusLinguistics[A].K.Aijmer&B.Altenberg(eds.).English Corpus Linguistics[C].Singapore:Longman,1996.[5]Mindt D.English corpus linguistics and the foreign language teaching syllabus[A].Thomas J&M.Short(eds.).Using Corpus for Language Research.Great[C].Britain:Longman,1996.[6]濮建忠.英语词汇教学中的类联接、搭配及词块[J].外语教学与研究,2003(6).[7]扬惠中,桂诗春.中国学习者英语语料库[M].上海:上海外语教育出版社,2003.[8]卫乃兴.基于语料库和语料库驱动的词语搭配研究[J].当代语言学.2002(4).[9]王龙吟,何安平.基于语料库的外语教学与二语习得的链接[J].外语与外语教学,2005(3).[10]何安平.语料库语言学与英语教学[M].北京:外语教学与研究出版,2004.[11]武和平,王秀秀.基于网络的语料库及其在英语教学中的应用[J].电化教育研究,2002(10).[12]张超.基于语料库的词块研究在教学中的应用[J].青年与社会,2011(7).[13]徐启龙.基于网络荚语语料库的词汇中心教学法[J].上海教育科研,2010(1).[14]黎芳.基于语料库的英语词汇教学法[J].长沙大学学报,2010,24(4).【基金项目】湖北省教育科学规划研究项目――基于词块理论的大学英语口语教学有效性研究,项目编号:2014A136。
哲学社会科学自主知识体系 英语

The independent intellectual system of philosophy and social scienceThis system represents a comprehensive and autonomous framework of knowledge and understanding within the field of philosophy and social science. It is not just a compilation of theories and methodologies from various schools of thought, but a coherent and internally consistent body of knowledge that is uniquely Chinese in its perspective and approach.The construction of such a system requires a deep understanding and appreciation of Chinese culture, history, and social realities. It also involves the critical evaluation and integration of global academic resources and trends, in order to form a unique and influential voice in the international academic community.The independent intellectual system of philosophy and social science is not static but dynamic, constantly evolving and adapting to new challenges and opportunities. It is a living and breathing entity that reflects the pulse of the times and the aspirations of the people.In building this system, we aim to contribute to the advancement of human civilization, providing insights and solutions to the complex problems facing our world. By doing so, we hope to establish China as a leading force in the field of philosophy and social science, contributing to global academic discourse and human progress.。
拉康的理论——精选推荐

. 黄华,权利,身体与自我一福科与女性主义文学批评「M]北京:北京大学出版社,2005必须注意的是,自我的建构是在一种时间的辩证法中实现的。
对拉康来说,镜子阶段远不仅是幼儿的一个自然发展阶段,而且是主体发展中一个体现了时间辩证法的、先行和回溯相互交织的决定性的时刻。
“这一发展是作为时间的辩证法而经历的,它把个体的形成决定性地投射进历史之中:镜子阶段是一出戏,其内在压力迅猛地从不足冲向先行(anticipation)——对于受空间认同诱惑的主体而言,这出戏生产了从身体的破碎形象到我所说的关于身体整体性的外科整形形式的种种幻想——直到最终穿戴起异化身份的盔甲。
这副盔甲以其僵硬的结构标识出主体的精神发展。
”[2](p6)拉康认为,镜子有一个整体的功能,构建统一的自我,自我形成于镜像阶段。
来源于作者与自我分裂 6Lacan and His Fundamental Concepts of Theory of SubjectJacques Lacan(1901-80)is undoubtedly the central figure of psychoanalysis in the second half of the 20th century.He has notonly revolutionized the psychoanalytic practice but also exerted a global reinterpretation of the entire psychoanalytic theoretical edificeby employing the achievements of structural linguistics and semiotics.The reinterpretation has changed the entire field of the scientific debate.Some of his formulas such as“[T]he unconscious is structured like a language”;“[D]esire is thedesire of the Other”(Ecrites:A Selection 121,123),etc.acquire an almost iconic statuslike Einstein’s E=mc2.The least one can say about Lacan is that nobody was undisturbed and unaffected by his work:even those whopassionately oppose him have to take stance from his theory. ForLacan, psychoanalysis totally changes the way we should understand“cause”,and“reality”,which the fundamental notions like “subject’,clearly indicates that the Freudian unconscious as primordial and irrational drives is ridiculous; on the contrary, the unconscious is in aspecific way fully rational, discursive, “structured likea language”(Ecrites:A Selection 121),which entails that Lacanreduces all psychic lifeto a symbolic interplay. He proposes the triangle of Imaginary-Symbolic-Real as the three basic phases of forming the subject,the elementary matrix of the human experience.The term Imaginary is obviously similar with fiction but in Lacaniansense it is notsimply synonymous with the fictional or unreal;on the contrary,imaginary identifications can have very real effects. Thereis of course an air of unreality to the Imaginary: it traps the subjectinto alienating identifications that prevent the truth from emerging.In the Mirror Stage that is a key step to the formation of the subject,when the baby at the age from six to eighteen months glimpses its own image in a mirror or some equivalent to a mirror, itgreets the image in the mirror with great jubilation and thus identifies with it which is unreal by definition and shows somethingyet to become: an integrated unity. It is this identification that forms its unconscious, but its initial self-identification is inevitably basedon the Other; so identification with this image in the mirror means alienation of the subject. Therefore, from the beginning, the site of the subject is occupied by the other; the subject is in fact deteriorated into emptiness in essence. The unconscious which is the soul of theunconscious, while the subject becomes accordingly the other’sdesire of the subject is the desire of the other. In this sense, the unconscious is not a latent being since it is not an entity; on the contrary, it is rather a negativity, a lack of being, and a hole in chains of signifiers. Lacan calls it pre-ontological. Strictly speaking, the unconscious cannot be defined in the sense of being delimited.The Symbolic is a register that exists prior to the individual subject, into which the subject must be inserted if he/she is to be able to speak and desire. The Symbolic is often exhibited as languages, cultures, persons and surroundings around. Insertion into the Symbolic is a negative or privative process which implies recognition on the part of the child that it is not in possession of thedesire. It is also an object phallus which is the object of the mother’swith which the child attempts to identify (desire always beinga desire of and for the Other).The agency that imposes the law and deprives the child of the phallus in an act of symbolic castration isthe name of father. It is in the name of father that we recognize the symbolic function which has identified the person with the figure ofthe law. In other words, the name of father is the sign of law.Insertion into the Symbolic thus implies renunciation of the illusionof omnipotence associated with identification with the phallus, and italso introduces a split into the subject. The subject, as used by Lacan,is a deliberately ambiguous term; it refers to both the subject of a sentence and the subject of the impersonal laws of language. The subject is always divided or decentered, not simply because of the presence of the unconscious, but because of the structures of language itself. “Existence i s a product of language”(Xie Qun:24). For a subject,to be given a signifier or named is of vital importance.Thesymbolic/language decides the existence of being.The things that enter the Symbolicand are represented by language become existent. The things unnamed by language will not exist into being.By analogy,a subjectthat is represented is recognized as a being.A child has to allow itselfto be represented by language/signifier in order to be a subject.Losing the mark of a signifier,one becomes non-being.Although the Symbolic differentiates dimensions that are confusedin the Imaginary, it does not replace or transcend the Imaginary.Though every subject must be inserted into or inscribed in the Symbolic,no subject lives in the Symbolic alone, just as speech doesnot replace imagery. The two orders co-exist with a third: the Real. Itis not synonymous with external reality but equal to the left dimension that constantly resists symbolism and signification. Thetwo conceptions the Real and the reality are mutually exclusive.the daily life-world in whichWhat we experience as “reality”―we feel athome—can only stabilize itself through the exclusion or primordial repression of the traumatic Real, while the Real is often in the guiseof fantastic apparitions which forever haunt the subject. It is the threatening element that invades the subject when rifts appear in the Symbolic, and the failure to recognize or submit to the name of thefather opens up such rifts. The implication of Lacan’s theory may beworks. First, the mirror stagetwo-fold in analyzing Joseph Conrad’sinitial attempt to construct an identity as anmarks the child’sindependent entity from the Other and to locate a position in the society. Since its self-identification is based on an idealized unitaryimage, fragmentation and loss underline its experience as a subjectonce it steps into the register of Symbolic .The characters in theworks are haunted by severe loss and inner split when they experience what differentiates its prior existential conditions.Second,the implication of Lacan’stheory is found in its explanation of thecauses and destination of human desires and how they are related tobecome a subject. Lacan emphasizes that man’s d esire is theand the desire aims at the phallus, in accessible tomother’s desire,the subject, and ushers the subject into an endless desire chain. To bea subject means to fill in an ontological lack that can never actuallybe fulfilled. Lacan’s revelation of the psychic structure of the selfand its way of function offers us a tool to interpret the cause of thefrustration and disintegration experienced by Conrad’s characters his fictional works.。
2013年北京第二外国语学院英语专业语言学真题试卷_真题(含答案与解析)-交互
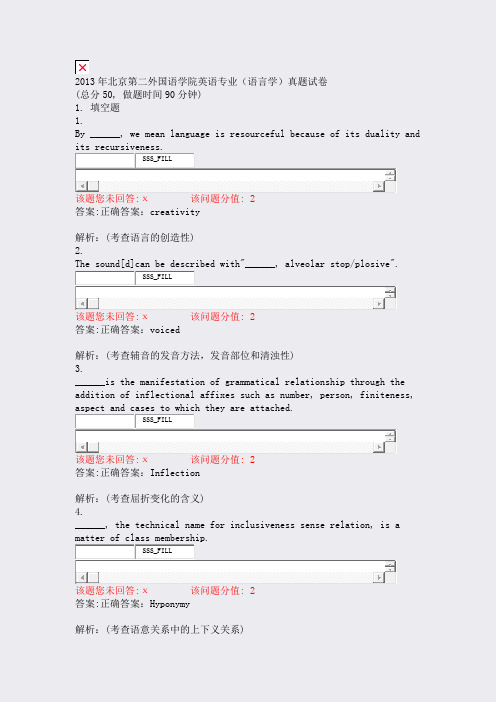
2013年北京第二外国语学院英语专业(语言学)真题试卷(总分50, 做题时间90分钟)1. 填空题1.By ______, we mean language is resourceful because of its duality and its recursiveness.SSS_FILL该题您未回答:х该问题分值: 2答案:正确答案:creativity解析:(考查语言的创造性)2.The sound[d]can be described with"______, alveolar stop/plosive".SSS_FILL该题您未回答:х该问题分值: 2答案:正确答案:voiced解析:(考查辅音的发音方法,发音部位和清浊性)3.______is the manifestation of grammatical relationship through the addition of inflectional affixes such as number, person, finiteness, aspect and cases to which they are attached.SSS_FILL该题您未回答:х该问题分值: 2答案:正确答案:Inflection解析:(考查屈折变化的含义)4.______, the technical name for inclusiveness sense relation, is a matter of class membership.SSS_FILL该题您未回答:х该问题分值: 2答案:正确答案:Hyponymy解析:(考查语意关系中的上下义关系)______is the ordinary act we perform when we speak, i. e. we move our vocal organs and produce a number of sounds, organized in a certain way and with a certain meaning.SSS_FILL该题您未回答:х该问题分值: 2答案:正确答案:The locutionary act解析:(考查言内行为的含义)2. 判断题1.As an interdisciplinary study of language and psychology, psycholinguistics has its roots in structural linguistics on the one hand, and in cognitive psychology on the other hand.SSS_SINGLE_SELA TrueB False该题您未回答:х该问题分值: 2答案:B解析:考查心理语言学的定义。
知识与技能的英语作文初一
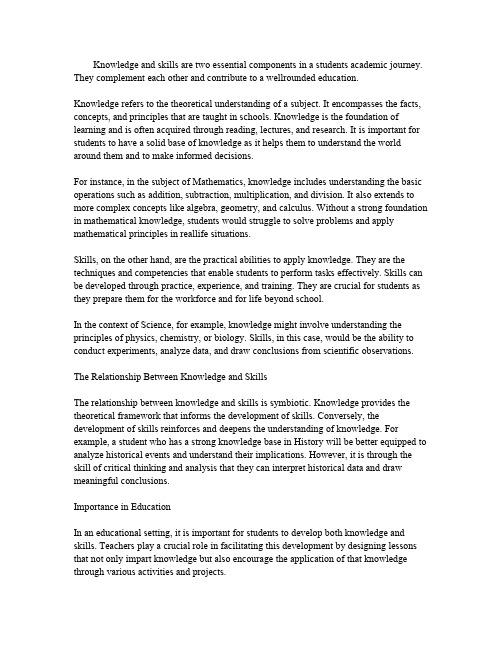
Knowledge and skills are two essential components in a students academic journey. They complement each other and contribute to a wellrounded education.Knowledge refers to the theoretical understanding of a subject.It encompasses the facts, concepts,and principles that are taught in schools.Knowledge is the foundation of learning and is often acquired through reading,lectures,and research.It is important for students to have a solid base of knowledge as it helps them to understand the world around them and to make informed decisions.For instance,in the subject of Mathematics,knowledge includes understanding the basic operations such as addition,subtraction,multiplication,and division.It also extends to more complex concepts like algebra,geometry,and calculus.Without a strong foundation in mathematical knowledge,students would struggle to solve problems and apply mathematical principles in reallife situations.Skills,on the other hand,are the practical abilities to apply knowledge.They are the techniques and competencies that enable students to perform tasks effectively.Skills can be developed through practice,experience,and training.They are crucial for students as they prepare them for the workforce and for life beyond school.In the context of Science,for example,knowledge might involve understanding the principles of physics,chemistry,or biology.Skills,in this case,would be the ability to conduct experiments,analyze data,and draw conclusions from scientific observations. The Relationship Between Knowledge and SkillsThe relationship between knowledge and skills is symbiotic.Knowledge provides the theoretical framework that informs the development of skills.Conversely,the development of skills reinforces and deepens the understanding of knowledge.For example,a student who has a strong knowledge base in History will be better equipped to analyze historical events and understand their implications.However,it is through the skill of critical thinking and analysis that they can interpret historical data and draw meaningful conclusions.Importance in EducationIn an educational setting,it is important for students to develop both knowledge and skills.Teachers play a crucial role in facilitating this development by designing lessons that not only impart knowledge but also encourage the application of that knowledge through various activities and projects.ConclusionIn conclusion,knowledge and skills are inseparable in education.They work together to create a holistic learning experience that prepares students for the challenges of the future. It is through the acquisition of both knowledge and skills that students can truly excel in their academic pursuits and become lifelong learners.。
胎儿透明隔腔、第三脑室、第四脑室的超声测量及其临床意义
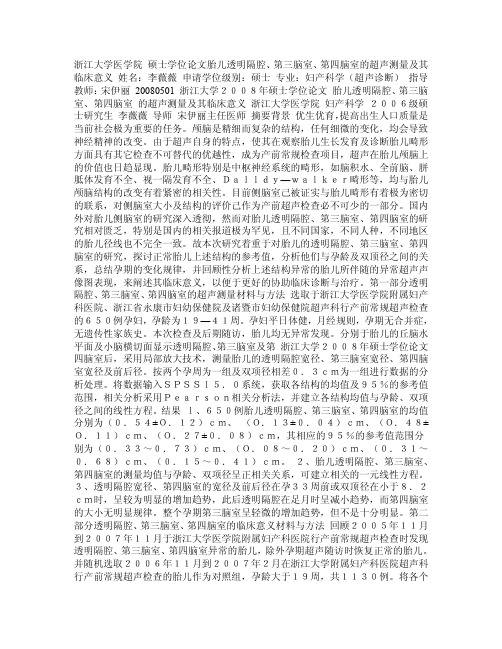
浙江大学医学院硕士学位论文胎儿透明隔腔、第三脑室、第四脑室的超声测量及其临床意义姓名:李薇薇申请学位级别:硕士专业:妇产科学(超声诊断)指导教师:宋伊丽20080501 浙江大学2008年硕士学位论文胎儿透明隔腔、第三脑室、第四脑室的超声测量及其临床意义浙江大学医学院妇产科学2006级硕士研究生李薇薇导师宋伊丽主任医师摘要背景优生优育,提高出生人口质量是当前社会极为重要的任务。
颅脑是精细而复杂的结构,任何细微的变化,均会导致神经精神的改变。
由于超声自身的特点,使其在观察胎儿生长发育及诊断胎儿畸形方面具有其它检查不可替代的优越性,成为产前常规检查项目,超声在胎儿颅脑上的价值也日趋显现。
胎儿畸形特别是中枢神经系统的畸形,如脑积水、全前脑、胼胝体发育不全、视一隔发育不全、Dalldy—walker畸形等,均与胎儿颅脑结构的改变有着紧密的相关性。
目前侧脑室己被证实与胎儿畸形有着极为密切的联系,对侧脑室大小及结构的评价己作为产前超声检查必不可少的一部分。
国内外对胎儿侧脑室的研究深入透彻,然而对胎儿透明隔腔、第三脑室、第四脑室的研究相对匮乏,特别是国内的相关报道极为罕见,且不同国家,不同人种,不同地区的胎儿径线也不完全一致。
故本次研究着重于对胎儿的透明隔腔、第三脑室、第四脑室的研究,探讨正常胎儿上述结构的参考值,分析他们与孕龄及双顶径之间的关系,总结孕期的变化规律,并回顾性分析上述结构异常的胎儿所伴随的异常超声声像图表现,来阐述其临床意义,以便于更好的协助临床诊断与治疗。
第一部分透明隔腔、第三脑室、第四脑室的超声测量材料与方法选取于浙江大学医学院附属妇产科医院、浙江省永康市妇幼保健院及诸暨市妇幼保健院超声科行产前常规超声检查的650例孕妇,孕龄为19—41周。
孕妇平日体健,月经规则,孕期无合并症,无遗传性家族史。
本次检查及后期随访,胎儿均无异常发现。
分别于胎儿的丘脑水平面及小脑横切面显示透明隔腔、第三脑室及第浙江大学2008年硕士学位论文四脑室后,采用局部放大技术,测量胎儿的透明隔腔宽径、第三脑室宽径、第四脑室宽径及前后径。
科研成果拓展人类知识边界
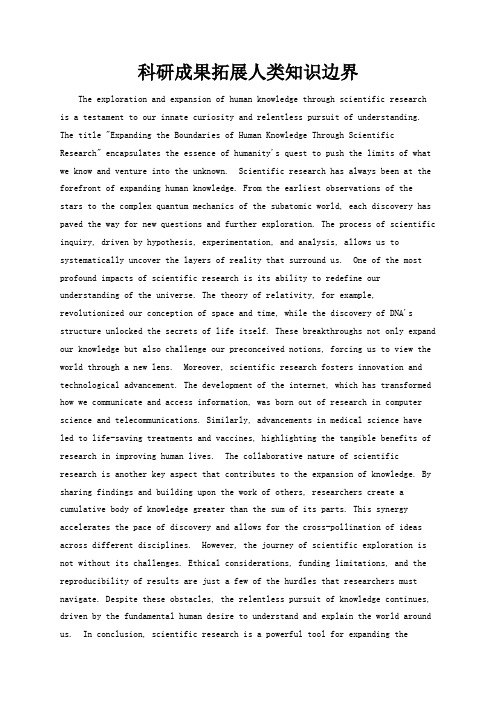
科研成果拓展人类知识边界The exploration and expansion of human knowledge through scientific research is a testament to our innate curiosity and relentless pursuit of understanding. The title "Expanding the Boundaries of Human Knowledge Through Scientific Research" encapsulates the essence of humanity's quest to push the limits of what we know and venture into the unknown. Scientific research has always been at the forefront of expanding human knowledge. From the earliest observations of the stars to the complex quantum mechanics of the subatomic world, each discovery has paved the way for new questions and further exploration. The process of scientific inquiry, driven by hypothesis, experimentation, and analysis, allows us to systematically uncover the layers of reality that surround us. One of the most profound impacts of scientific research is its ability to redefine our understanding of the universe. The theory of relativity, for example, revolutionized our conception of space and time, while the discovery of DNA's structure unlocked the secrets of life itself. These breakthroughs not only expand our knowledge but also challenge our preconceived notions, forcing us to view the world through a new lens. Moreover, scientific research fosters innovation and technological advancement. The development of the internet, which has transformed how we communicate and access information, was born out of research in computer science and telecommunications. Similarly, advancements in medical science have led to life-saving treatments and vaccines, highlighting the tangible benefits of research in improving human lives. The collaborative nature of scientific research is another key aspect that contributes to the expansion of knowledge. By sharing findings and building upon the work of others, researchers create a cumulative body of knowledge greater than the sum of its parts. This synergy accelerates the pace of discovery and allows for the cross-pollination of ideas across different disciplines. However, the journey of scientific exploration is not without its challenges. Ethical considerations, funding limitations, and the reproducibility of results are just a few of the hurdles that researchers must navigate. Despite these obstacles, the relentless pursuit of knowledge continues, driven by the fundamental human desire to understand and explain the world around us. In conclusion, scientific research is a powerful tool for expanding theboundaries of human knowledge. It is a dynamic and ever-evolving process that not only enriches our understanding of the universe but also has practicalapplications that enhance our daily lives. As we continue to explore the unknown, we honor the legacy of the countless researchers whose contributions have shaped our past and will light the way for our future. This essay reflects the spirit of scientific inquiry and the profound impact it has on expanding the horizons of human knowledge. It is a tribute to the tireless efforts of researchers and the transformative power of their discoveries. As we stand on the shoulders of giants, we look forward to the new frontiers that scientific research will unveil, continuing the noble tradition of expanding the boundaries of what it means to be human.。
- 1、下载文档前请自行甄别文档内容的完整性,平台不提供额外的编辑、内容补充、找答案等附加服务。
- 2、"仅部分预览"的文档,不可在线预览部分如存在完整性等问题,可反馈申请退款(可完整预览的文档不适用该条件!)。
- 3、如文档侵犯您的权益,请联系客服反馈,我们会尽快为您处理(人工客服工作时间:9:00-18:30)。
Corpus-based and Knowledge-based Measures of Text Semantic SimilarityRada Mihalcea and Courtney Corley Department of Computer ScienceUniversity of North Texas{rada,corley}@Carlo StrapparavaIstituto per la Ricerca Scientifica e TecnologicaITC–irststrappa@itc.itAbstractThis paper presents a method for measuring the se-mantic similarity of texts,using corpus-based andknowledge-based measures of similarity.Previous workon this problem has focused mainly on either large doc-uments(e.g.text classification,information retrieval)or individual words(e.g.synonymy tests).Given thata large fraction of the information available today,onthe Web and elsewhere,consists of short text snip-pets(e.g.abstracts of scientific documents,imaginecaptions,product descriptions),in this paper we fo-cus on measuring the semantic similarity of short texts.Through experiments performed on a paraphrase dataset,we show that the semantic similarity method out-performs methods based on simple lexical matching,re-sulting in up to13%error rate reduction with respect tothe traditional vector-based similarity metric.IntroductionMeasures of text similarity have been used for a long time in applications in natural language processing and related areas.One of the earliest applications of text similarity is perhaps the vectorial model in information retrieval,where the document most relevant to an input query is determined by ranking documents in a collection in reversed order of their similarity to the given query(Salton&Lesk1971). Text similarity has also been used for relevance feedback and text classification(Rocchio1971),word sense disam-biguation(Lesk1986;Schutze1998),and more recently for extractive summarization(Salton et al.1997),and methods for automatic evaluation of machine translation(Papineni et al.2002)or text summarization(Lin&Hovy2003).Mea-sures of text similarity were also found useful for the evalu-ation of text coherence(Lapata&Barzilay2005).With few exceptions,the typical approach tofinding the similarity between two text segments is to use a simple lex-ical matching method,and produce a similarity score based on the number of lexical units that occur in both input seg-ments.Improvements to this simple method have consid-ered stemming,stop-word removal,part-of-speech tagging, longest subsequence matching,as well as various weighting and normalization factors(Salton&Buckley1997).While Copyright c 2006,American Association for Artificial Intelli-gence().All rights reserved.successful to a certain degree,these lexical similarity meth-ods cannot always identify the semantic similarity of texts. For instance,there is an obvious similarity between the text segments I own a dog and I have an animal,but most of the current text similarity metrics will fail in identifying any kind of connection between these texts.There is a large number of word-to-word semantic simi-larity measures,using approaches that are either knowledge-based(Wu&Palmer1994;Leacock&Chodorow1998) or corpus-based(Turney2001).Such measures have been successfully applied to language processing tasks such as malapropism detection(Budanitsky&Hirst2001),word sense disambiguation(Patwardhan,Banerjee,&Pedersen 2003),and synonym identification(Turney2001).For text-based semantic similarity,perhaps the most widely used ap-proaches are the approximations obtained through query ex-pansion,as performed in information retrieval(V oorhees 1993),or the latent semantic analysis method(Landauer, Foltz,&Laham1998)that measures the similarity of texts by exploiting second-order word relations automatically ac-quired from large text collections.A related line of work consists of methods for paraphrase recognition,which typically seek to align sentences in com-parable corpora(Barzilay&Elhadad2003;Dolan,Quirk, &Brockett2004),or paraphrase generation using distribu-tional similarity applied on paths of dependency trees(Lin& Pantel2001)or using bilingual parallel corpora(Barnard& Callison-Burch2005).These methods target the identifica-tion of paraphrases in large documents,or the generation of paraphrases starting with an input text,without necessarily providing a measure of their similarity.The recently intro-duced textual entailment task(Dagan,Glickman,&Magnini 2005)is also related to some extent,however textual entail-ment targets the identification of a directional inferential re-lation between texts,which is different than textual similar-ity,and hence entailment systems are not overviewed here. In this paper,we suggest a method for measuring the semantic similarity of texts by exploiting the information that can be drawn from the similarity of the component words.Specifically,we describe two corpus-based and six knowledge-based measures of word semantic similarity,and show how they can be used to derive a text-to-text similarity metric.We show that this measure of text semantic sim-ilarity outperforms the simpler vector-based similarity ap-proach,as evaluated on a paraphrase recognition task.Text Semantic SimilarityMeasures of semantic similarity have been traditionally de-fined between words or concepts,and much less between text segments consisting of two or more words.The em-phasis on word-to-word similarity metrics is probably due to the availability of resources that specifically encode re-lations between words or concepts(e.g.WordNet),and the various testbeds that allow for their evaluation(e.g.TOEFL or SAT analogy/synonymy tests).Moreover,the derivation of a text-to-text measure of similarity starting with a word-based semantic similarity metric may not be straightforward, and consequently most of the work in this area has consid-ered mainly applications of the traditional vectorial model, occasionally extended to n-gram language models.Given two input text segments,we want to automatically derive a score that indicates their similarity at semantic level, thus going beyond the simple lexical matching methods tra-ditionally used for this task.Although we acknowledge the fact that a comprehensive metric of text semantic similarity should also take into account the structure of the text,we take afirst rough cut at this problem and attempt to model the semantic similarity of texts as a function of the semantic similarity of the component words.We do this by combining metrics of word-to-word similarity and word specificity into a formula that is a potentially good indicator of the semantic similarity of the two input texts.The following section provides details on eight different corpus-based and knowledge-based measures of word se-mantic similarity.In addition to the similarity of words, we also take into account the specificity of words,so that we can give a higher weight to a semantic matching identi-fied between two specific words(e.g.collie and sheepdog), and give less importance to the similarity measured between generic concepts(e.g.get and become).While the speci-ficity of words is already measured to some extent by their depth in the semantic hierarchy,we are reinforcing this fac-tor with a corpus-based measure of word specificity,based on distributional information learned from large corpora. The specificity of a word is determined using the in-verse document frequency(idf)introduced by Sparck-Jones (1972),defined as the total number of documents in the cor-pus divided by the total number of documents including that word.The idf measure was selected based on previous work that theoretically proved the effectiveness of this weight-ing approach(Papineni2001).In the experiments reported here,document frequency counts are derived starting with the British National Corpus–a100million words corpus of modern English including both spoken and written genres. Given a metric for word-to-word similarity and a measure of word specificity,we define the semantic similarity of two text segments T1and T2using a metric that combines the semantic similarities of each text segment in turn with re-spect to the other text segment.First,for each word w in the segment T1we try to identify the word in the segment T2 that has the highest semantic similarity(maxSim(w,T2)), according to one of the word-to-word similarity measures described in the following section.Next,the same process is applied to determine the most similar word in T1starting with words in T2.The word similarities are then weighted with the corresponding word specificity,summed up,and normalized with the length of each text segment.Finally the resulting similarity scores are combined using a simple av-erage.Note that only open-class words and cardinals can participate in this semantic matching process.As done in previous work on text similarity using vector-based models, all function words are discarded.The similarity between the input text segments T1and T2 is therefore determined using the following scoring function:sim(T1,T2)=12(w∈{T1}(maxSim(w,T2)∗id f(w))w∈{T1}id f(w)+w∈{T2}(maxSim(w,T1)∗id f(w))w∈{T2}id f(w))(1)This similarity score has a value between0and1,with a score of1indicating identical text segments,and a score of 0indicating no semantic overlap between the two segments. Note that the maximum similarity is sought only within classes of words with the same part-of-speech.The rea-son behind this decision is that most of the word-to-word knowledge-based measures cannot be applied across parts-of-speech,and consequently,for the purpose of consistency, we imposed the“same word-class”restriction to all the word-to-word similarity measures.This means that,for in-stance,the most similar word to the nounflower within the text“There are many green plants next to the house”will be sought among the nouns plant and house,and will ig-nore the words with a different part-of-speech(be,green, next).Moreover,for those parts-of-speech for which a word-to-word semantic similarity cannot be measured(e.g.some knowledge-based measures are not defined among adjec-tives or adverbs),we use instead a lexical match measure, which assigns a maxSim of1for identical occurrences of a word in the two text segments.Semantic Similarity of WordsThere is a relatively large number of word-to-word similar-ity metrics that were previously proposed in the literature, ranging from distance-oriented measures computed on se-mantic networks,to metrics based on models of distribu-tional similarity learned from large text collections.From these,we chose to focus our attention on two corpus-based metrics and six knowledge-based different metrics,selected mainly for their observed performance in other natural lan-guage processing applications.Corpus-based MeasuresCorpus-based measures of word semantic similarity try to identify the degree of similarity between words using infor-mation exclusively derived from large corpora.In the exper-iments reported here,we considered two metrics,namely: (1)pointwise mutual information(Turney2001),and(2)la-tent semantic analysis(Landauer,Foltz,&Laham1998). Pointwise Mutual Information The pointwise mutual information using data collected by information retrieval(PMI-IR)was suggested by(Turney2001)as an unsuper-vised measure for the evaluation of the semantic similarity of words.It is based on word co-occurrence using counts collected over very large corpora(e.g.the Web).Given two words w1and w2,their PMI-IR is measured as:PMI-IR(w1,w2)=log2p(w1&w2)p(w1)∗p(w2)(2)which indicates the degree of statistical dependence between w1and w2,and can be used as a measure of the seman-tic similarity of w1and w2.From the four different types of queries suggested by Turney(2001),we are using the NEAR query(co-occurrence within a ten-word window), which is a balance between accuracy(results obtained on synonymy tests)and efficiency(number of queries to be runagainst a search engine).Specifically,the following query is used to collect counts from the AltaVista search engine.p NEAR(w1&w2) hits(w1NEAR w2)W ebSize(3)With p(w i)approximated as hits(w1)/W ebSize,the fol-lowing PMI-IR measure is obtained:log2hits(w1AND w2)∗W ebSizehits(w1)∗hits(w2)(4)In a set of experiments based on TOEFL synonymy tests (Turney2001),the PMI-IR measure using the NEAR op-erator accurately identified the correct answer(out of four synonym choices)in72.5%of the cases,which exceeded by a large margin the score obtained with latent semantic analysis(64.4%),as well as the average non-English collegeapplicant(64.5%).Since Turney(2001)performed evalu-ations of synonym candidates for one word at a time,the W ebSize value was irrelevant in the ranking.In our applica-tion instead,it is not only the ranking of the synonym candi-dates that matters(for the selection of maxSim in Equation 1),but also the true value of PMI-IR,which is needed forthe overall calculation of the text-to-text similarity metric. We approximate the value of W ebSize to7x1011,which is the value used by Chklovski(2004)in co-occurrence exper-iments involving Web counts.Latent Semantic Analysis Another corpus-based mea-sure of semantic similarity is the latent semantic analysis (LSA)proposed by Landauer(1998).In LSA,term co-occurrences in a corpus are captured by means of a dimen-sionality reduction operated by a singular value decomposi-tion(SVD)on the term-by-document matrix T representing the corpus.For the experiments reported here,we run the SVD operation on the British National Corpus.SVD is a well-known operation in linear algebra,whichcan be applied to any rectangular matrix in order tofind cor-relations among its rows and columns.In our case,SVD decomposes the term-by-document matrix T into three ma-trices T=UΣk V T whereΣk is the diagonal k×k matrix containing the k singular values of T,σ1≥σ2≥...≥σk, and U and V are column-orthogonal matrices.When the three matrices are multiplied together the original term-by-document matrix is re-composed.Typically we can choose k k obtaining the approximation T UΣk V T.LSA can be viewed as a way to overcome some of the drawbacks of the standard vector space model(sparseness and high dimensionality).In fact,the LSA similarity is com-puted in a lower dimensional space,in which second-order relations among terms and texts are exploited.The similarity in the resulting vector space is then measured with the stan-dard cosine similarity.Note also that LSA yields a vector space model that allows for a homogeneous representation (and hence comparison)of words,word sets,and texts. The application of the LSA word similarity measure to text semantic similarity is done using Equation1,which roughly amounts to the pseudo-document text representa-tion for LSA computation,as described by Berry(1992).In practice,each text segment is represented in the LSA space by summing up the normalized LSA vectors of all the con-stituent words,using also a tf.idf weighting scheme. Knowledge-based MeasuresThere are a number of measures that were developed to quantify the degree to which two words are semantically re-lated using information drawn from semantic networks–see e.g.(Budanitsky&Hirst2001)for an overview.We present below several measures found to work well on the Word-Net hierarchy.All these measures assume as input a pair of concepts,and return a value indicating their semantic relat-edness.The six measures below were selected based on their observed performance in other language processing applica-tions,and for their relatively high computational efficiency. We conduct our evaluation using the following word sim-ilarity metrics:Leacock&Chodorow,Lesk,Wu&Palmer, Resnik,Lin,and Jiang&Conrath.Note that all these metrics are defined between concepts,rather than words,but they can be easily turned into a word-to-word similarity metric by selecting for any given pair of words those two meanings that lead to the highest concept-to-concept similarity1.We use the WordNet-based implementation of these metrics,as available in the WordNet::Similarity package(Patwardhan, Banerjee,&Pedersen2003).We provide below a short de-scription for each of these six metrics.The Leacock&Chodorow(Leacock&Chodorow1998) similarity is determined as:Sim lch=−loglength2∗D(5) where length is the length of the shortest path between two concepts using node-counting,and D is the maximum depth of the taxonomy.The Lesk similarity of two concepts is defined as a function of the overlap between the corresponding definitions,as pro-vided by a dictionary.It is based on an algorithm proposed by Lesk(1986)as a solution for word sense disambiguation. The application of the Lesk similarity measure is not limited to semantic networks,and it can be used in conjunction with any dictionary that provides word definitions.The Wu and Palmer(Wu&Palmer1994)similarity met-ric measures the depth of two given concepts in the Word-1This is similar to the methodology used by(McCarthy et al. 2004)tofind similarities between words and senses starting with a concept-to-concept similarity measure.Net taxonomy,and the depth of the least common subsumer (LCS),and combines thesefigures into a similarity score:Sim wup=2∗depth(LCS)depth(concept1)+depth(concept2)(6)The measure introduced by Resnik(Resnik1995)returns the information content(IC)of the LCS of two concepts:Sim res=IC(LCS)(7) where IC is defined as:IC(c)=−log P(c)(8) and P(c)is the probability of encountering an instance of concept c in a large corpus.The next measure we use in our experiments is the metric in-troduced by Lin(Lin1998),which builds on Resnik’s mea-sure of similarity,and adds a normalization factor consisting of the information content of the two input concepts:Sim lin=2∗IC(LCS)12(9)Finally,the last similarity metric considered is Jiang& Conrath(Jiang&Conrath1997):Sim jnc=112(10)Note that all the word similarity measures are normalized so that they fall within a0–1range.The normalization is done by dividing the similarity score provided by a given measure with the maximum possible score for that measure.A Walk-Through ExampleThe application of the text similarity measure is illustrated with an example.Given two text segments,as shown be-low,we want to determine a score that reflects their semantic similarity.For illustration purposes,we restrict our attention to one corpus-based measure–the PMI-IR metric imple-mented using the AltaVista NEAR operator.Text Segment1:When the defendant and his lawyer walked into the court,some of the victim supporters turned their backs on him.Text Segment2:When the defendant walked into the court-house with his attorney,the crowd turned their backs on him. Starting with each of the two text segments,and for each open-class word,we determine the most similar word in the other text segment,according to the PMI-IR similarity mea-sure.As mentioned earlier,a semantic similarity is sought only between words with the same part-of-speech.Table1 shows the word similarity scores and the word specificity (idf)starting with thefirst text segment.Next,using Equation1,we combine the word similari-ties and their corresponding specificity,and determine the semantic similarity of the two texts as0.80.This similarity score correctly identifies the paraphrase relation between the two text segments(using the same threshold of0.50as used throughout all the experiments reported in this paper).In-stead,a cosine similarity score based on the same idf weightsText1Text2maxSim idfdefendant defendant 1.00 3.93lawyer attorney0.89 2.64walked walked 1.00 1.58court courthouse0.60 1.06victims courthouse0.40 2.11supporters crowd0.40 2.15turned turned 1.000.66backs backs 1.00 2.41Table1:Word similarity scores and word specificity(idf) will result in a score of0.46,thereby failing tofind the para-phrase relation.Although there are a few words that occur in both text seg-ments(e.g.defendant,or turn),there are also words that are not identical,but closely related,wyer found similar to attorney,or supporters which is related to crowd.Unlike traditional similarity measures based on lexical matching, our metric takes into account the semantic similarity of these words,resulting in a more precise measure of text similarity.Evaluation and ResultsTo test the effectiveness of the text semantic similarity mea-sure,we use it to automatically identify if two text segments are paraphrases of each other.We use the Microsoft para-phrase corpus(Dolan,Quirk,&Brockett2004),consisting of4,076training and1,725test pairs,and determine the number of correctly identified paraphrase pairs in the cor-pus using the text semantic similarity measure as the only indicator of paraphrasing.The paraphrase pairs in this cor-pus were automatically collected from thousands of news sources on the Web over a period of18months,and were subsequently labeled by two human annotators who deter-mined if the two sentences in a pair were semantically equiv-alent or not.The agreement between the human judges who labeled the candidate paraphrase pairs in this data set was measured at approximately83%,which can be considered as an upperbound for an automatic paraphrase recognition task performed on this data set.For each candidate paraphrase pair in the test set,wefirst evaluate the text semantic similarity metric using Equation 1,and then label the candidate pair as a paraphrase if the similarity score exceeds a threshold of0.5.Note that this is an unsupervised experimental setting,and therefore the training data is not used in the experiments.BaselinesFor comparison,we also compute two baselines:(1)A ran-dom baseline created by randomly choosing a true(para-phrase)or false(not paraphrase)value for each text pair;and (2)A vector-based similarity baseline,using a cosine simi-larity measure as traditionally used in information retrieval, with tf.idf weighting.ResultsWe evaluate the results in terms of accuracy,representing the number of correctly identified true or false classifications inMetric Acc.Prec.Rec.FSemantic similarity(corpus-based)PMI-IR69.970.295.281.0LSA68.469.795.280.5Semantic similarity(knowledge-based)J&C69.372.287.179.0L&C69.572.487.079.0Lesk69.372.486.678.9Lin69.371.688.779.2W&P69.070.292.180.0Resnik69.069.096.480.4Combined70.369.697.781.3BaselinesVector-based65.471.679.575.3Random51.368.350.057.8 Table2:Text similarity for paraphrase identificationthe test data set.We also measure precision,recall and F-measure,calculated with respect to the true values in the test data.Table2shows the results obtained.Among all the individual measures of similarity,the PMI-IR measure was found to perform the best,although the difference with respect to the other measures is small.In addition to the individual measures of similarity,we also evaluate a metric that combines several similarity mea-sures into a singlefigure,using a simple average.We in-clude all similarity measures,for an overallfinal accuracy of70.3%,and an F-measure of81.3%.The improvement of the semantic similarity metrics over the vector-based cosine similarity was found to be statisti-cally significant in all the experiments,using a paired t-test (p<0.001).Discussion and ConclusionsAs it turns out,incorporating semantic information into measures of text similarity increases the likelihood of recog-nition significantly over the random baseline and over the vector-based cosine similarity baseline,as measured in a paraphrase recognition task.The best performance is achieved using a method that combines several similarity metrics into one,for an overall accuracy of70.3%,repre-senting a significant13.8%error rate reduction with respect to the vector-based cosine similarity baseline.Moreover,if we were to take into account the upperbound of83%estab-lished by the inter-annotator agreement achieved on this data set(Dolan,Quirk,&Brockett2004),the error rate reduction over the baseline appears even more significant.In addition to performance,we also tried to gain insights into the applicability of the semantic similarity measures,by finding their coverage on this data set.On average,among approximately18,000word similarities identified in this cor-pus,about14,500are due to lexical matches,and3,500are due to semantic similarities,which indicates that about20% of the relations found between text segments are based on semantics,in addition to lexical identity.Despite the differences among the various word-to-word similarity measures(corpus-based vs.knowledge-based, definitional vs.link-based),the results are surprisingly sim-ilar.To determine if the similar overall results are due to a similar behavior on the same subset of the test data(pre-sumably an“easy”subset that can be solved using measures of semantic similarity),or if the different measures cover in fact different subsets of the data,we calculated the Pearson correlation factor among all the similarity measures.As seen in Table3,there is in fact a high correlation among several of the knowledge-based measures,indicating an overlap in their behavior.Although some of these metrics are diver-gent in what they measure(e.g.Lin versus Lesk),it seems that the fact they are applied in a context lessens the differ-ences observed when applied at word level.Interestingly, the Resnik measure has a low correlation with the other knowledge-based measures,and a somehow higher correla-tion with the corpus-based metrics,which is probably due to the data-driven information content used in the Resnik mea-sure(although Lin and Jiang&Conrath also use the infor-mation content,they have an additional normalization factor that makes them behave differently).Perhaps not surprising, the corpus-based measures are only weakly correlated with the knowledge-based measures and among them,with LSA having the smallest correlation with the other metrics.An interesting example is represented by the following two text segments,where only the Resnik measure and the two corpus-based measures manage to identify the para-phrase,because of a higher similarity found between sys-tems and PC,and between technology and processor.Text Segment1:Gateway will release new Profile4systems with the new Intel technology on Wednesday.Text Segment2:Gateway’s all-in-one PC,the Profile4, also now features the new Intel processor.There are also cases where almost all the semantic simi-larity measures fail,and instead the simpler cosine similar-ity has a better performance.This is mostly the case for the negative(not paraphrase)examples in the test data,where the semantic similarities identified between words increase the overall text similarity above the threshold of0.5.For in-stance,the following text segments were falsely marked as paraphrases by all but the cosine similarity and the Resnik measure:Text Segment1:The man wasn’t on the ice,but trapped in the rapids,swaying in an eddy about250feet from the shore.Text Segment2:The man was trapped about250feet from the shore,right at the edge of the falls.The small variations between the accuracies obtained with the corpus-based and knowledge-based measures also sug-gest that both data-driven and knowledge-rich methods have their own merits,leading to a similar performance.Corpus-based methods have the advantage that no hand-made re-sources are needed and,apart form the choice of an ap-propriate and large corpus,they raise no problems related to the completeness of the resources.On the other hand, knowledge-based methods can encodefine-grained informa-tion.This difference can be observed in terms of precision and recall.In fact,while precision is generally higher with knowledge-based measures,corpus-based measures give in general better performance in recall.Although our method relies on a bag-of-words approach, as it turns out the use of measures of semantic similarity improves significantly over the traditional lexical matching。