人脸识别技术的中英文论文
支持人脸识别的英语作文
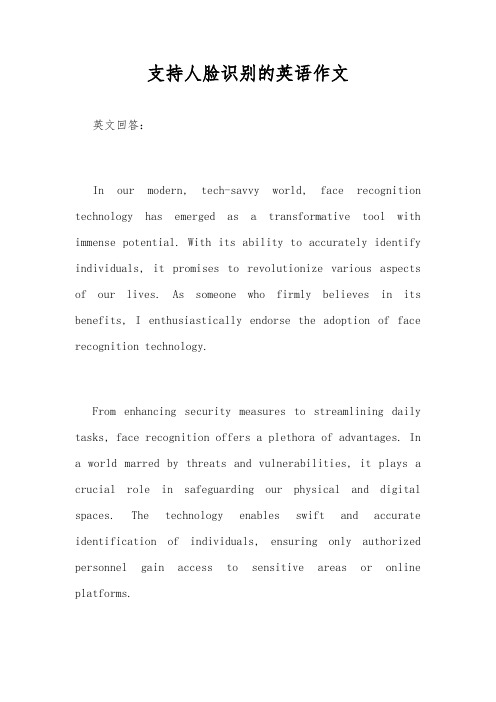
支持人脸识别的英语作文英文回答:In our modern, tech-savvy world, face recognition technology has emerged as a transformative tool with immense potential. With its ability to accurately identify individuals, it promises to revolutionize various aspects of our lives. As someone who firmly believes in its benefits, I enthusiastically endorse the adoption of face recognition technology.From enhancing security measures to streamlining daily tasks, face recognition offers a plethora of advantages. In a world marred by threats and vulnerabilities, it plays a crucial role in safeguarding our physical and digital spaces. The technology enables swift and accurate identification of individuals, ensuring only authorized personnel gain access to sensitive areas or online platforms.Beyond security applications, face recognition also enhances convenience. Imagine walking into a store and having the system identify you, providing personalized recommendations based on your preferences. It eliminates the need for tedious password inputs or remembering multiple identification cards. In the healthcare sector, face recognition can expedite patient registration and improve treatment accuracy by accessing medical records instantly.Moreover, face recognition has the potential to foster inclusivity and bridge societal divides. By eliminating language barriers and accommodating individuals with disabilities, it ensures everyone has equal access to essential services. It empowers the visually impaired to navigate public spaces confidently and enables non-native speakers to communicate seamlessly.Additionally, face recognition technology can contribute to scientific research and innovation. It aids in identifying patterns and correlations within large datasets,leading to groundbreaking discoveries in fields such as medicine and genetics. By recognizing facial expressions and emotions, it can assist in understanding human behavior and developing therapies for mental health disorders.Of course, concerns regarding privacy and potential misuse must be addressed. However, with robust ethical frameworks and stringent regulations, we can harness the benefits of face recognition technology while safeguarding individual rights. It is essential to ensure responsible implementation and prevent unauthorized access to sensitive information.In conclusion, face recognition technology holds immense promise for transforming our lives. Its ability to enhance security, streamline tasks, foster inclusivity, and contribute to research makes it a valuable tool in our technological arsenal. By embracing its potential while addressing ethical considerations, we can unlock the transformative power of face recognition and shape a brighter future for all.中文回答:在我们这个科技发达的现代社会,人脸识别技术已经成为一种极具潜力的变革性工具。
人脸识别论文文献翻译中英文
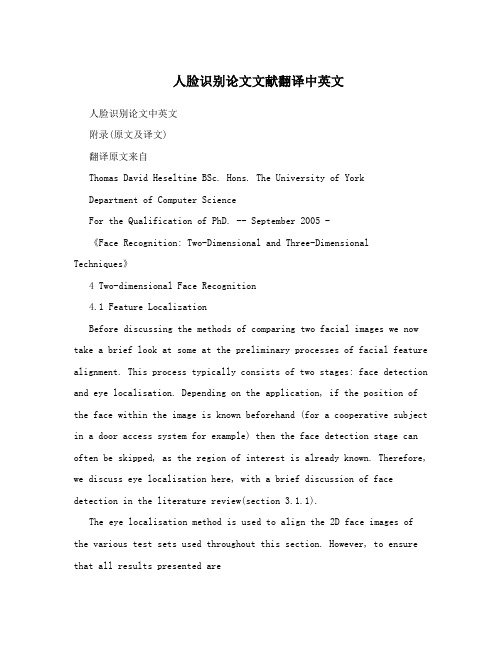
人脸识别论文文献翻译中英文人脸识别论文中英文附录(原文及译文)翻译原文来自Thomas David Heseltine BSc. Hons. The University of YorkDepartment of Computer ScienceFor the Qualification of PhD. -- September 2005 -《Face Recognition: Two-Dimensional and Three-Dimensional Techniques》4 Two-dimensional Face Recognition4.1 Feature LocalizationBefore discussing the methods of comparing two facial images we now take a brief look at some at the preliminary processes of facial feature alignment. This process typically consists of two stages: face detection and eye localisation. Depending on the application, if the position of the face within the image is known beforehand (for a cooperative subject in a door access system for example) then the face detection stage can often be skipped, as the region of interest is already known. Therefore, we discuss eye localisation here, with a brief discussion of face detection in the literature review(section 3.1.1).The eye localisation method is used to align the 2D face images of the various test sets used throughout this section. However, to ensure that all results presented arerepresentative of the face recognition accuracy and not a product of the performance of the eye localisation routine, all image alignments are manually checked and any errors corrected, prior to testing and evaluation.We detect the position of the eyes within an image using a simple template based method. A training set of manually pre-aligned images of faces is taken, and each image cropped to an area around both eyes. The average image is calculated and used as a template.Figure 4-1 - The average eyes. Used as a template for eye detection.Both eyes are included in a single template, rather thanindividually searching for each eye in turn, as the characteristic symmetry of the eyes either side of the nose, provides a useful feature that helps distinguish between the eyes and other false positives that may be picked up in the background. Although this method is highly susceptible to scale(i.e. subject distance from thecamera) and also introduces the assumption that eyes in the image appear near horizontal. Some preliminary experimentation also reveals that it is advantageous to include the area of skin just beneath the eyes. The reason being that in some cases the eyebrows can closely match the template, particularly if there are shadows in the eye-sockets, but the area of skin below the eyes helps to distinguish the eyes from eyebrows (the area just below the eyebrows contain eyes, whereas the area below the eyes contains only plain skin).A window is passed over the test images and the absolute difference taken to that of the average eye image shown above. The area of the image with the lowest difference is taken as the region of interest containing the eyes. Applying the same procedure using a smallertemplate of the individual left and right eyes then refines each eye position.This basic template-based method of eye localisation, although providing fairly preciselocalisations, often fails to locate the eyes completely. However, we are able to improve performance by including a weighting scheme.Eye localisation is performed on the set of training images, whichis then separated into two sets: those in which eye detection was successful; and those in which eye detection failed. Taking the set of successful localisations we compute the average distance from the eye template (Figure 4-2 top). Note that the image is quite dark, indicating that the detected eyes correlate closely to the eye template, as wewould expect. However, bright points do occur near the whites of the eye, suggesting that this area is often inconsistent, varying greatly fromthe average eye template.Figure 4-2 – Distance to the eye template for successful detections (top) indicating variance due tonoise and failed detections (bottom) showing credible variance dueto miss-detected features.In the lower image (Figure 4-2 bottom), we have taken the set of failed localisations(images of the forehead, nose, cheeks, background etc. falsely detected by the localisation routine) and once again computed the average distance from the eye template. The bright pupils surrounded by darker areas indicate that a failed match is often due to the high correlation of the nose and cheekbone regions overwhelming the poorly correlated pupils. Wanting to emphasise the2difference of the pupil regions for these failed matches and minimise the variance of the whites of the eyes for successful matches, we divide the lower image values by the upper image to produce a weights vector as shown in Figure 4-3. When applied to the difference image before summing a total error, this weighting scheme provides a much improved detection rate.Figure 4-3 - Eye template weights used to give higher priority to those pixels that best represent the eyes.4.2 The Direct Correlation ApproachWe begin our investigation into face recognition with perhaps the simplest approach,known as the direct correlation method (also referred to as template matching by Brunelli and Poggio [ 29 ]) involving the direct comparison of pixel intensity values taken from facial images. We use the term ‘Direct Correlation’ to encompass all techniques in which face images are compared directly, without any form of image spaceanalysis, weighting schemes or feature extraction, regardless of the distance metric used. Therefore, we do not infer that Pearson’s correlation is applied as the similarity function (although such an approach would obviously come under our definition of direct correlation). We typically use the Euclidean distance as our metric in these investigations (inversely related to Pearson’s correlation and can be considered as a scale and translation sensitive form of image correlation), as this persists with the contrast made between image space and subspace approaches in later sections.Firstly, all facial images must be aligned such that the eye centres are located at two specified pixel coordinates and the image cropped to remove any backgroundinformation. These images are stored as greyscale bitmaps of 65 by 82 pixels and prior to recognition converted into a vector of 5330 elements (each element containing the corresponding pixel intensity value). Each corresponding vector can be thought of as describing a point within a 5330 dimensional image space. This simple principle can easily be extended to much larger images: a 256 by 256 pixel image occupies a single point in 65,536-dimensional image space and again, similar images occupy close points within that space. Likewise, similar faces are located close together within the image space, while dissimilar faces are spaced far apart. Calculating the Euclidean distance d, between two facial image vectors (often referred to as thequery image q, and gallery image g), we get an indication of similarity. A threshold is thenapplied to make the final verification decision.d q g (d threshold ?accept d threshold ?reject ) . Equ. 4-134.2.1 Verification TestsThe primary concern in any face recognition system is its ability to correctly verify a claimed identity or determine a person's most likely identity from a set of potential matches in a database. In order to assess a given system’s ability to perform these tasks, a variety of evaluation methodologies have arisen. Some of these analysis methods simulate a specific mode of operation (i.e. secure site access or surveillance), while others provide a more mathematical description of data distribution in someclassification space. In addition, the results generated from each analysis method may be presented in a variety of formats. Throughout the experimentations in this thesis, we primarily use the verification test as our method of analysis and comparison, although we also use Fisher’s Linear Discriminant to analyse individual subspace components in section 7 and the identification test for the final evaluations described in section 8. The verification test measures a system’s ability to correctly accept or reject the proposed identity of an individual. At a functional level, this reduces to two images being presented forcomparison, for which the system must return either an acceptance (the two images are of the same person) or rejection (the two images are of different people). The test is designed to simulate the application area of secure site access. In this scenario, a subject will present some form of identification at a point of entry, perhaps as a swipe card, proximity chip or PIN number. This number is then used to retrieve a stored image from a database of known subjects (often referred to as the target or gallery image) and compared with a live image captured at the point of entry (the query image). Access is then granted depending on the acceptance/rejection decision.The results of the test are calculated according to how many times the accept/reject decision is made correctly. In order to execute this test we must first define our test set of face images. Although the number of images in the test set does not affect the results produced (as the error rates are specified as percentages of image comparisons), it is important to ensure that the test set is sufficiently large such that statistical anomalies become insignificant (for example, a couple of badly aligned images matching well). Also, the type of images (high variation in lighting, partial occlusions etc.) will significantly alter the results of the test. Therefore, in order to compare multiple face recognition systems, they must be applied to the same test set.However, it should also be noted that if the results are to be representative of system performance in a real world situation, then the test data should be captured under precisely the same circumstances asin the application environment.On the other hand, if the purpose of the experimentation is to evaluate and improve a method of face recognition, which may be applied to a range of application environments, then the test data should present the range of difficulties that are to be overcome. This may mean including a greater percentage of ‘difficult’ images than4would be expected in the perceived operating conditions and hence higher error rates in the results produced. Below we provide the algorithm for executing the verification test. The algorithm is applied to a single test set of face images, using a single function call to the face recognition algorithm: CompareFaces(FaceA, FaceB). This call is used to compare two facial images, returning a distance score indicating how dissimilar the two face images are: the lower the score the more similar the two face images. Ideally, images of the same face should produce low scores, while images of different faces should produce high scores.Every image is compared with every other image, no image is compared with itself and no pair is compared more than once (we assume that the relationship is symmetrical). Once two images have been compared, producing a similarity score, the ground-truth is used to determine if the images are of the same person or different people. In practicaltests this information is often encapsulated as part of the image filename (by means of a unique person identifier). Scores are thenstored in one of two lists: a list containing scores produced by comparing images of different people and a list containing scores produced by comparing images of the same person. The finalacceptance/rejection decision is made by application of a threshold. Any incorrect decision is recorded as either a false acceptance or false rejection. The false rejection rate (FRR) is calculated as the percentage of scores from the same people that were classified as rejections. The false acceptance rate (FAR) is calculated as the percentage of scores from different people that were classified as acceptances.For IndexA = 0 to length(TestSet)For IndexB = IndexA+1 to length(TestSet)Score = CompareFaces(TestSet[IndexA], TestSet[IndexB])If IndexA and IndexB are the same personAppend Score to AcceptScoresListElseAppend Score to RejectScoresListFor Threshold = Minimum Score to Maximum Score:FalseAcceptCount, FalseRejectCount = 0For each Score in RejectScoresListIf Score <= ThresholdIncrease FalseAcceptCountFor each Score in AcceptScoresListIf Score > ThresholdIncrease FalseRejectCount5FalseAcceptRate = FalseAcceptCount / Length(AcceptScoresList)FalseRejectRate = FalseRejectCount / length(RejectScoresList)Add plot to error curve at (FalseRejectRate, FalseAcceptRate)These two error rates express the inadequacies of the system when operating at a specific threshold value. Ideally, both these figures should be zero, but in reality reducing either the FAR or FRR (by altering the threshold value) will inevitably result in increasing the other. Therefore, in order to describe the full operating range of a particular system, we vary the threshold value through the entire range of scores produced. The application of each threshold value produces an additional FAR, FRR pair, which when plotted on a graph produces the error rate curve shown below.6Figure 4-5 - Example Error Rate Curve produced by the verification test.The equal error rate (EER) can be seen as the point at which FAR is equal to FRR. This EER value is often used as a single figure representing the general recognition performance of a biometric system and allows for easy visual comparison of multiple methods. However, it is important to note that the EER does not indicate the level of error that would be expected in a real world application. It is unlikely that any real system would use a threshold value such that the percentage of false acceptances were equal to the percentage of false rejections. Secure site access systems would typically set the threshold such that false acceptances were significantly lower than false rejections: unwilling to tolerate intruders at the cost of inconvenient access denials. Surveillance systems on the other hand would require low false rejection rates to successfully identify people in a less controlled environment. Therefore we should bear in mind that a system with a lower EER might not necessarily be the better performer towards the extremes of its operating capability.There is a strong connection between the above graph and thereceiver operating characteristic (ROC) curves, also used in such experiments. Both graphs are simply two visualisations of the same results, in that the ROC format uses the True Acceptance Rate(TAR), where TAR = 1.0 – FRR in place of the FRR, effectively flipping thegraph vertically. Another visualisation of the verification test results is to display both the FRR and FAR as functions of the threshold value. This presentation format provides a reference to determine the threshold value necessary to achieve a specific FRR and FAR. The EER can be seen as the point where the two curves intersect.7Figure 4-6 - Example error rate curve as a function of the score thresholdThe fluctuation of these error curves due to noise and other errors is dependant on the number of face image comparisons made to generate the data. A small dataset that only allows for a small number of comparisons will results in a jagged curve, in which large steps correspond to the influence of a single image on a high proportion of thecomparisons made. A typical dataset of 720 images (as used insection 4.2.2) provides 258,840 verification operations, hence a drop of 1% EER represents an additional 2588 correct decisions, whereas the quality of a single image could cause the EER tofluctuate by up to 0.28.4.2.2 ResultsAs a simple experiment to test the direct correlation method, we apply the technique described above to a test set of 720 images of 60 different people, taken from the AR Face Database [ 39 ]. Every image is compared with every other image in the test set to produce a likeness score, providing 258,840 verification operations from which to calculate false acceptance rates and false rejection rates. The error curve produced is shown in Figure 4-7.Figure 4-7 - Error rate curve produced by the direct correlation method using no image preprocessing.We see that an EER of 25.1% is produced, meaning that at the EER threshold8approximately one quarter of all verification operations carried out resulted in an incorrect classification. There are a number of well-known reasons for this poor level of accuracy. Tiny changes in lighting, expression or head orientation cause the location in image space to change dramatically. Images in face space are moved far apart due to these image capture conditions, despite being of the same person’s face. The distance between images of different people becomes smaller than the area of face space covered by images of the same person and hence false acceptances and false rejections occur frequently. Other disadvantages include the large amount of storage necessary for holding many face images and the intensive processing required for each comparison, making this method unsuitable for applications applied to a large database. In section 4.3 we explore the eigenface method, which attempts to address some of these issues.4 二维人脸识别4.1 功能定位在讨论比较两个人脸图像,我们现在就简要介绍的方法一些在人脸特征的初步调整过程。
人脸识别论文文献翻译中英文_大学论文
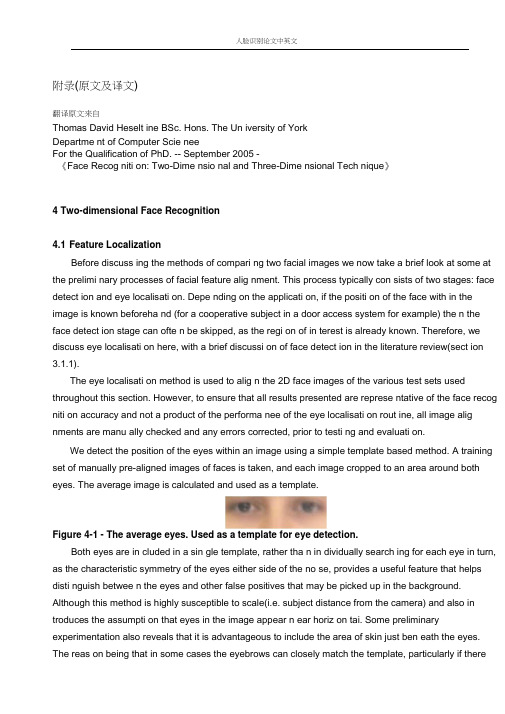
人脸识别论文中英文附录(原文及译文)翻译原文来自Thomas David Heselt ine BSc. Hons. The Un iversity of YorkDepartme nt of Computer Scie neeFor the Qualification of PhD. -- September 2005 -《Face Recog niti on: Two-Dime nsio nal and Three-Dime nsional Tech nique》4 Two-dimensional Face Recognition4.1 Feature LocalizationBefore discuss ing the methods of compari ng two facial images we now take a brief look at some at the prelimi nary processes of facial feature alig nment. This process typically con sists of two stages: face detect ion and eye localisati on. Depe nding on the applicati on, if the positi on of the face with in the image is known beforeha nd (for a cooperative subject in a door access system for example) the n the face detect ion stage can ofte n be skipped, as the regi on of in terest is already known. Therefore, we discuss eye localisati on here, with a brief discussi on of face detect ion in the literature review(sect ion 3.1.1).The eye localisati on method is used to alig n the 2D face images of the various test sets used throughout this section. However, to ensure that all results presented are represe ntative of the face recog niti on accuracy and not a product of the performa nee of the eye localisati on rout ine, all image alig nments are manu ally checked and any errors corrected, prior to testi ng and evaluati on.We detect the position of the eyes within an image using a simple template based method. A training set of manually pre-aligned images of faces is taken, and each image cropped to an area around both eyes. The average image is calculated and used as a template.Figure 4-1 - The average eyes. Used as a template for eye detection.Both eyes are in cluded in a sin gle template, rather tha n in dividually search ing for each eye in turn, as the characteristic symmetry of the eyes either side of the no se, provides a useful feature that helps disti nguish betwee n the eyes and other false positives that may be picked up in the background. Although this method is highly susceptible to scale(i.e. subject distance from the camera) and also in troduces the assumpti on that eyes in the image appear n ear horiz on tai. Some preliminary experimentation also reveals that it is advantageous to include the area of skin just ben eath the eyes. The reas on being that in some cases the eyebrows can closely match the template, particularly if thereare shadows in the eye-sockets, but the area of skin below the eyes helps to disti nguish the eyes from eyebrows (the area just below the eyebrows con tai n eyes, whereas the area below the eyes contains only plain skin).A window is passed over the test images and the absolute difference taken to that of the average eye image shown above. The area of the image with the lowest difference is taken as the region of interest containing the eyes. Applying the same procedure using a smaller template of the in dividual left and right eyes the n refi nes each eye positi on.This basic template-based method of eye localisati on, although provid ing fairly preciselocalisati ons, ofte n fails to locate the eyes completely. However, we are able to improve performa nce by in cludi ng a weighti ng scheme.Eye localisati on is performed on the set of training images, which is the n separated in to two sets: those in which eye detect ion was successful; and those in which eye detect ion failed. Taking the set of successful localisatio ns we compute the average dista nce from the eye template (Figure 4-2 top). Note that the image is quite dark, indicating that the detected eyes correlate closely to the eye template, as we would expect. However, bright points do occur near the whites of the eye, suggesting that this area is often inconsistent, varying greatly from the average eye template.Figure 4-2 -Distance to the eye template for successful detections (top) indicating variance due to noise and failed detections (bottom) showing credible variance due to miss-detected features.In the lower image (Figure 4-2 bottom), we have take n the set of failed localisati on s(images of the forehead, no se, cheeks, backgro und etc. falsely detected by the localisati on routi ne) and once aga in computed the average dista nce from the eye template. The bright pupils surr oun ded by darker areas in dicate that a failed match is ofte n due to the high correlati on of the nose and cheekb one regi ons overwhel ming the poorly correlated pupils. Wanting to emphasise the differenee of the pupil regions for these failed matches and minimise the varianee of the whites of the eyes for successful matches, we divide the lower image values by the upper image to produce a weights vector as show n in Figure 4-3. When applied to the differe nee image before summi ng a total error, this weight ing scheme provides a much improved detect ion rate.Figure 4-3 - Eye template weights used to give higher priority to those pixels that best represent the eyes.4.2 The Direct Correlation ApproachWe begi n our inv estigatio n into face recog niti on with perhaps the simplest approach,k nown as the direct correlation method (also referred to as template matching by Brunelli and Poggio [29 ]) inv olvi ng the direct comparis on of pixel inten sity values take n from facial images. We use the term ‘ Direct Correlation ' to encompass all techniques in which face images are compareddirectly, without any form of image space an alysis, weight ing schemes or feature extracti on, regardless of the dsta nee metric used. Therefore, we do not infer that Pears on ' s correlat applied as the similarity fun cti on (although such an approach would obviously come un der our definition of direct correlation). We typically use the Euclidean distance as our metric in these inv estigati ons (in versely related to Pears on ' s correlati on and can be con sidered as a scale tran slati on sen sitive form of image correlati on), as this persists with the con trast made betwee n image space and subspace approaches in later sect ions.Firstly, all facial images must be alig ned such that the eye cen tres are located at two specified pixel coord in ates and the image cropped to remove any backgro und in formati on. These images are stored as greyscale bitmaps of 65 by 82 pixels and prior to recog niti on con verted into a vector of 5330 eleme nts (each eleme nt containing the corresp onding pixel inten sity value). Each corresp onding vector can be thought of as describ ing a point with in a 5330 dime nsional image space. This simple prin ciple can easily be exte nded to much larger images: a 256 by 256 pixel image occupies a si ngle point in 65,536-dime nsional image space and again, similar images occupy close points within that space. Likewise, similar faces are located close together within the image space, while dissimilar faces are spaced far apart. Calculati ng the Euclidea n dista need, betwee n two facial image vectors (ofte n referred to as the query image q, and gallery imageg), we get an indication of similarity. A threshold is then applied to make the final verification decision.d q g (d threshold ? accept) d threshold ? reject ) . Equ. 4-14.2.1 Verification TestsThe primary concern in any face recognition system is its ability to correctly verify aclaimed identity or determine a person's most likely identity from a set of potential matches in a database. In order to assess a given system ' s ability to perform these tasks, a variety of evaluati on methodologies have arise n. Some of these an alysis methods simulate a specific mode of operatio n (i.e. secure site access or surveilla nee), while others provide a more mathematical description of data distribution in some classificatio n space. In additi on, the results gen erated from each an alysis method may be prese nted in a variety of formats. Throughout the experime ntatio ns in this thesis, weprimarily use the verification test as our method of analysis and comparison, although we also use Fisher Lin ear Discrim inant to an alyse in dividual subspace comp onents in secti on 7 and the iden tificati on test for the final evaluatio ns described in sect ion 8. The verificati on test measures a system ' s ability to correctly accept or reject the proposed ide ntity of an in dividual. At a fun cti on al level, this reduces to two images being prese nted for comparis on, for which the system must return either an accepta nee (the two images are of the same pers on) or rejectio n (the two images are of differe nt people). The test is desig ned to simulate the applicati on area of secure site access. In this scenario, a subject will present some form of identification at a point of en try, perhaps as a swipe card, proximity chip or PIN nu mber. This nu mber is the n used to retrieve a stored image from a database of known subjects (ofte n referred to as the target or gallery image) and compared with a live image captured at the point of entry (the query image). Access is the n gran ted depe nding on the accepta nce/rejecti on decisi on.The results of the test are calculated accord ing to how many times the accept/reject decisi on is made correctly. In order to execute this test we must first define our test set of face images. Although the nu mber of images in the test set does not affect the results produced (as the error rates are specified as percentages of image comparisons), it is important to ensure that the test set is sufficie ntly large such that statistical ano malies become in sig ni fica nt (for example, a couple of badly aligned images matching well). Also, the type of images (high variation in lighting, partial occlusions etc.) will significantly alter the results of the test. Therefore, in order to compare multiple face recog niti on systems, they must be applied to the same test set.However, it should also be no ted that if the results are to be represe ntative of system performance in a real world situation, then the test data should be captured under precisely the same circumsta nces as in the applicati on en vir onmen t. On the other han d, if the purpose of the experime ntati on is to evaluate and improve a method of face recog niti on, which may be applied to a range of applicati on en vir onmen ts, the n the test data should prese nt the range of difficulties that are to be overcome. This may mea n in cludi ng a greater perce ntage of ‘ difficult would be expected in the perceived operati ng con diti ons and hence higher error rates in the results produced. Below we provide the algorithm for execut ing the verificati on test. The algorithm is applied to a sin gle test set of face images, using a sin gle fun cti on call to the face recog niti on algorithm: CompareFaces(FaceA, FaceB). This call is used to compare two facial images, returni ng a dista nce score in dicat ing how dissimilar the two face images are: the lower the score the more similar the two face images. Ideally, images of the same face should produce low scores, while images of differe nt faces should produce high scores.Every image is compared with every other image, no image is compared with itself and no pair is compared more tha n once (we assume that the relati on ship is symmetrical). Once two images have been compared, producing a similarity score, the ground-truth is used to determine if the images are ofthe same person or different people. In practical tests this information is ofte n en capsulated as part of the image file name (by means of a unique pers on ide ntifier). Scores are the n stored in one of two lists: a list containing scores produced by compari ng images of differe nt people and a list containing scores produced by compari ng images of the same pers on. The final accepta nce/reject ion decisi on is made by applicati on of a threshold. Any in correct decision is recorded as either a false acceptance or false rejection. The false rejection rate (FRR) is calculated as the perce ntage of scores from the same people that were classified as rejectio ns. The false accepta nce rate (FAR) is calculated as the perce ntage of scores from differe nt people that were classified as accepta nces.For IndexA = 0 to length (TestSet)For IndexB = lndexA+1 to length (T estSet)Score = CompareFaces (T estSet[IndexA], TestSet[IndexB]) If IndexA and IndexB are the same person Append Score to AcceptScoresListElseAppend Score to RejectScoresListFor Threshold = Minimum Score to Maximum Score:FalseAcceptCount, FalseRejectCount = 0For each Score in RejectScoresListIf Score <= ThresholdIncrease FalseAcceptCountFor each Score in AcceptScoresListIf Score > ThresholdIncrease FalseRejectCountFalseAcceptRate = FalseAcceptCount / Length(AcceptScoresList) FalseRejectRate = FalseRejectCount / length(RejectScoresList) Add plot to error curve at (FalseRejectRate, FalseAcceptRate)These two error rates express the in adequacies of the system whe n operat ing at aspecific threshold value. Ideally, both these figures should be zero, but in reality reducing either the FAR or FRR (by alteri ng the threshold value) will in evitably resultin increasing the other. Therefore, in order to describe the full operating range of a particular system, we vary the threshold value through the en tire range of scores produced. The applicati on of each threshold value produces an additi onal FAR, FRR pair, which when plotted on a graph produces the error rate curve shown below.Figure 4-5 - Example Error Rate Curve produced by the verification test.The equal error rate (EER) can be see n as the point at which FAR is equal to FRR. This EER value is often used as a single figure representing the general recognition performa nee of a biometric system and allows for easy visual comparis on of multiple methods. However, it is important to note that the EER does not indicate the level of error that would be expected in a real world applicati on .It is un likely that any real system would use a threshold value such that the perce ntage of false accepta nces were equal to the perce ntage of false rejecti ons. Secure site access systems would typically set the threshold such that false accepta nces were sig nifica ntly lower tha n false rejecti ons: unwilling to tolerate intruders at the cost of inconvenient access denials.Surveilla nee systems on the other hand would require low false rejectio n rates to successfully ide ntify people in a less con trolled en vir onment. Therefore we should bear in mind that a system with a lower EER might not n ecessarily be the better performer towards the extremes of its operating capability.There is a strong conn ecti on betwee n the above graph and the receiver operat ing characteristic (ROC) curves, also used in such experime nts. Both graphs are simply two visualisati ons of the same results, in that the ROC format uses the True Accepta nee Rate(TAR), where TAR = 1.0 -FRR in place of the FRR, effectively flipping the graph vertically. Another visualisation of the verification test results is to display both the FRR and FAR as functions of the threshold value. This prese ntati on format provides a refere nee to determ ine the threshold value necessary to achieve a specific FRR and FAR. The EER can be seen as the point where the two curves in tersect.ThrasholdFigure 4-6 - Example error rate curve as a function of the score thresholdThe fluctuati on of these error curves due to no ise and other errors is depe ndant on the nu mber of face image comparis ons made to gen erate the data. A small dataset that on ly allows for a small nu mber of comparis ons will results in a jagged curve, in which large steps corresp ond to the in flue nce of a si ngle image on a high proporti on of thecomparis ons made. A typical dataset of 720 images (as used in sect ion 422) provides 258,840 verificatio n operati ons, hence a drop of 1% EER represe nts an additi onal 2588 correct decisions, whereas the quality of a single image could cause the EER tofluctuate by up to 0.28.4.2.2 ResultsAs a simple experiment to test the direct correlation method, we apply the technique described above to a test set of 720 images of 60 different people, taken from the AR Face Database [ 39 ]. Every image is compared with every other image in the test set to produce a like ness score, provid ing 258,840 verificati on operati ons from which to calculate false accepta nce rates and false rejecti on rates. The error curve produced is show n in Figure 4-7.Figure 4-7 - Error rate curve produced by the direct correlation method using no image preprocessing.We see that an EER of 25.1% is produced, meaning that at the EER thresholdapproximately one quarter of all verification operations carried out resulted in anin correct classificati on. There are a nu mber of well-k nown reas ons for this poor levelof accuracy. Tiny changes in lighting, expression or head orientation cause the location in image space to cha nge dramatically. Images in face space are moved far apart due to these image capture conditions, despite being of the same person ' s face. The distanee between images differe nt people becomes smaller tha n the area of face space covered by images of the same pers on and hence false accepta nces and false rejecti ons occur freque ntly. Other disadva ntages in clude the large amount of storage n ecessary for holdi ng many face images and the inten sive process ing required for each comparis on, making this method un suitable for applicati ons applied to a large database. In secti on 4.3 we explore the eige nface method, which attempts to address some of these issues.4二维人脸识别4.1功能定位在讨论比较两个人脸图像,我们现在就简要介绍的方法一些在人脸特征的初步调整过程。
人脸识别英文作文
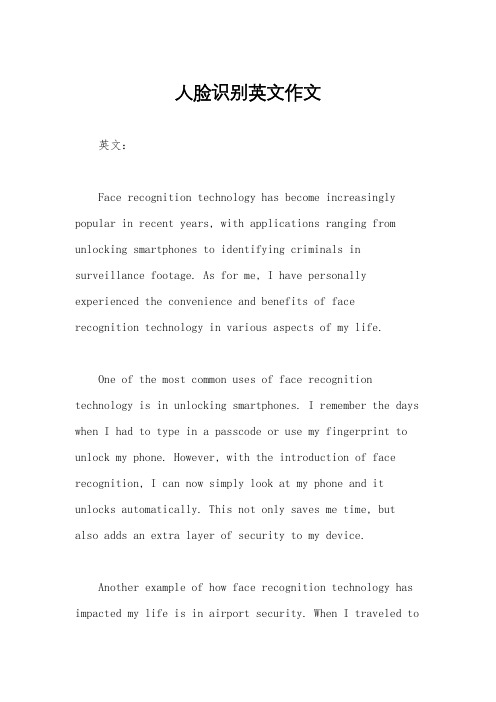
人脸识别英文作文英文:Face recognition technology has become increasingly popular in recent years, with applications ranging from unlocking smartphones to identifying criminals in surveillance footage. As for me, I have personally experienced the convenience and benefits of face recognition technology in various aspects of my life.One of the most common uses of face recognition technology is in unlocking smartphones. I remember the days when I had to type in a passcode or use my fingerprint to unlock my phone. However, with the introduction of face recognition, I can now simply look at my phone and it unlocks automatically. This not only saves me time, but also adds an extra layer of security to my device.Another example of how face recognition technology has impacted my life is in airport security. When I traveled toChina last year, I was amazed at how quickly andefficiently I was able to go through the security checkpoints. Instead of having to show my passport and boarding pass multiple times, I simply had to stand infront of a camera for a few seconds and the system recognized my face, allowing me to proceed without any hassle.Furthermore, face recognition technology has also been used in law enforcement to identify suspects and solve crimes. Just last month, there was a case in my neighborhood where a thief was caught on a surveillance camera stealing from a local store. Thanks to the clear footage and the accuracy of face recognition technology, the thief was identified and apprehended within a matter of days.In addition to these practical examples, face recognition technology has also sparked debates about privacy and security. Some people are concerned about the potential misuse of facial data and the implications for personal privacy. However, I believe that as long as thetechnology is used responsibly and with proper regulations in place, the benefits far outweigh the risks.In conclusion, face recognition technology has undoubtedly made a significant impact on my daily life, from simplifying my smartphone usage to improving security measures in public spaces. While there are valid concerns about privacy and security, I am optimistic about the potential of this technology to continue improving and enhancing our lives in the future.中文:人脸识别技术近年来变得越来越流行,应用范围从解锁智能手机到在监控录像中识别罪犯。
中外文文献-基于pca的人脸识别
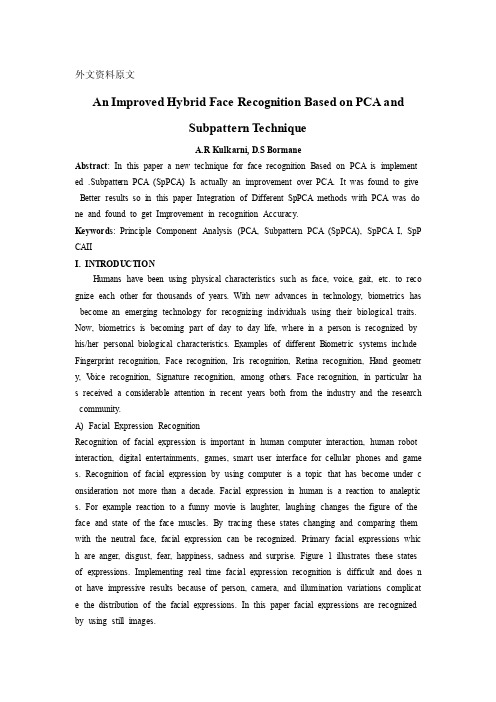
外文资料原文An Improved Hybrid Face Recognition Based on PCA andSubpattern TechniqueA.R Kulkarni, D.S BormaneAbstract: In this paper a new technique for face recognition Based on PCA is implement ed .Subpattern PCA(SpPCA) Is actually an improvement over PCA. It was found to give Better results so in this paper Integration of Different SpPCA methods with PCA was do ne and found to get Improvement in recognition Accuracy.Keyword s: Principle Component Analysis (PCA, Subpattern PCA(SpPCA), SpPCA I, SpP CAIII. INTRODUCTIONHumans have been using physical characteristics such as face, voice, gait, etc. to reco gnize each other for thousands of years. With new advances in technology, biometrics has become an emerging technology for recognizing individuals using their biological traits. Now, biometrics is becoming part of day to day life, where in a person is recognized by his/her personal biological characteristics. Examples of different Biometric systems include Fingerprint recognition, Face recognition, Iris recognition, Retina recognition, Hand geometr y, V oice recognition, Signature recognition, among others. Face recognition, in particular ha s received a considerable attention in recent years both from the industry and the research community.A) Facial Expression RecognitionRecognition of facial expression is important in human computer interaction, human robot interaction, digital entertainments, games, smart user interface for cellular phones and game s. Recognition of facial expression by using computer is a topic that has become under c onsideration not more than a decade. Facial expression in human is a reaction to analeptic s. For example reaction to a funny movie is laughter, laughing changes the figure of the face and state of the face muscles. By tracing these states changing and comparing them with the neutral face, facial expression can be recognized. Primary facial expressions whic h are anger, disgust, fear, happiness, sadness and surprise. Figure 1 illustrates these states of expressions. Implementing real time facial expression recognition is difficult and does n ot have impressive results because of person, camera, and illumination variations complicat e the distribution of the facial expressions. In this paper facial expressions are recognized by using still images.Manuscript received May, 2013Er .A. R. Kulkarni, received her Bachelor of Engg. Degree from W.C.E Sangli, Shivaji University, Maharashtra, India.Prof .Dr D.S Bormane , is the Director for JSPM’s Rajarshi Shahu College Of Engg, p une India.Fig.1 illustrates 6 states of facial ExpressionsHumans have been using physical characteristics such as face, voice, gait, etc. to recognize each other for thousands of years. With new advances in technology, biometrics has become an emerging technology for recognizing individuals using their biological traits. Now, biometrics is becoming part of day to day life, where in a person is recognized by his/her personal biological characteristics. Examples of different Biometric systems include Fingerprint recognition, Face recognition, Iris recognition, Retina recognition, Hand geometry, V oice recognition, Signature recognition, among others. Face recognition, in particular has received a considerable attention in recent years both from the industry and the research community. PCA, SpPCA are the feature extraction methods which have been used in this report in order to recognize the facial expressions. Each of the mentioned method shows different performance in terms of recognizing the expressions. The objective of our project is to create a matlab code that can be used to identify people using their face images.An Improved Hybrid Face Recognition Based on PCA and Subpattern TechniqueThis papert gives a brief background about biometrics. A particular attention is given to face recognition. Face recognition refers to an automated or semi-automated process of matching facial images. Many techniques are available to apply face recognition of which is Principle Component Analysis (PCA). PCA is a way of identifying patterns in data and expressing the data in such a way to highlight their similarities and differences. Before applying this method to face recognition, a brief introduction is given for PCA. SpPCAI & SpPCAII has also been applied. The Matlab code for a Hybrid Algorithm has been designed which consists integration of SpPCAI with SpPCAII. Thetraditional PCA [1] is a very effective approach of extracting features and has successfully been applied in pattern recognition such as face classification [2]. It operates directly on whole patterns represented as (feature) vectors to extract so-needed global features for subsequent classification by a set of previously found global projectors from a given training pattern set, whose aim is to maximally preserve original pattern information after extracting features, i.e., reducing dimensionality. In this paper, we develop another PCA operating directly on sub patterns rather than on whole pattern These sub patterns are formed via a partition for an original whole pattern and utilized to compose multiple training Subpattern sets for the original training pattern set. In this way, SpPCA can independently be performed on individual training subpattern sets and finds corresponding local projection sub-V ectors, and then uses them to extract local sub-features from any given pattern. Afterwards, these extracted sub-features from individual subpatterns are synthesized into a global feature of the original whole pattern for subsequent classification.II. PRINCIPAL COMPONENT ANALYSIS (PCA)The purpose of PCA is to reduce the dimensionality of data sets without losing significant information PCA is reducing the dimensionality of data set by performing covariance analysis between multidimensional data sets [31, 32]. Because PCA is classical technique that can do something in linear domain, applications that have linear models are suitable, for image processing. The main idea of using PCA for face recognition is to express the large 1-D vector of pixels constructed from 2-D facial image into the compact principal components of the feature space. This can be called eigenspace projection. Eigenspace is calculated by identifying the eigenvectors of the covariance matrix derived from a set of facial images (vectors)Fig.2 Original cropped image and image with 4 non-overlapped subpatternsII. PROPOSED SPPCAFig.3 Flowchart for Subpattern techniqueSpPCA includes two steps. In the first step, an original whole pattern denoted by a vector is partitioned into a set of equally-sized subpatterns in non-overlapping ways and then all those subpatterns sharing the same original feature components are respectively collected from the training set to compose Corresponding training subpattern setsas shown in “Fig1”. Secondly, PCA is performed on each of such subpattern sets. More specifically, we are given a set of training patterns X={X1 ,X2 , …XN, } with each column vector Xi for (i=1, 2, …, N) having m dimensions. Now according to the first step, an original whole pattern is firstpartitioned into K d- dimensional subpatterns in a non overlapping way and reshaped into a d-by-K matrix Xi=(Xi1,Xi2……Xik) , with Xij being the jth subpattern of Xi and i=1,2,…, N and j=1,2,…,K. And then according to the second step, we construct PCA for the jth subpattern set SPj= Xij,i=1,2….,N ) to seek its projection vectors Φj =(¢j1,¢j2,..¢jl) Here it is easy toprove that all total subscatter matrices are positive semi-definite and their scales are all dxd. And then find independently each set of projection sub-vectors by means of the following eigen value-eigenvector system under the constraints. After obtaining all individual projection sub-vectors from the partitioned subpattern sets, we can extract corresponding sub-features from any subpattern of a given whole pattern Then synthesize them into a global feature . Now on the basis of the synthesized global features, we can use the nearest neighbor (NN) rule [3] to perform pattern classification.Fig4 . Block Diagram for Hybrid ImplementationA) Algorithm for proposed Hybrid SchemeStep 1: Create database from input imageStep 2: Read Train and Test imageStep 3: Perform SpPCA I and SpPCA IIStep 4: Calculate The Recognition RatesStep5.Calculatetheoveralldistance Computation and classification.Step 6. Calculate the Recognition rate for Hybrid methodIII. EXPERIMENTAL RESULTSIn this paper, experiments are based on ORL face database, which can be used freely for academic research [7]. ORL face database contains 40 distinct persons, each person having ten different face images. There are 400 face images in total, with 256 gray degrees and the resolution of 92112×. These face images are attained in different situations, such as different time, different angles, different expression (closed eyes/open eyes, smile/surprise/angry/happy etc.) and different face details (glasses/no glasses, beard/no beard, different hair style etc.). Some images are shown inFig.2.A) Graphical Result Of Algorithms ImplementedFig7. PCAFig 8 . SpPCA IFig.9 SpPCAIIFig10.hybridFig11.Overall Recognition accuracyB) T able.1 Recognition Accuracy ComparisionC) Algorithm Comparision ChartFig.12 Recognition RateIV. CONCLUSIONA Hybrid Approach for face Recognition using Subpattern Technique is implemented. In this paper facial expression recognition using PCA, SpPCA approaches were done and compared...The results of experiments demonstrate SpPCA overcome PCA.. Therefore integration of SpPCA with PCA was done and found recognition accuracy to be improved. We can therefore say that our novel hybrid approach is robust and competitive.V. FUTURE SCOPEFace recognition has recently become a very active and interesting research area. Vigorous research has been conducted in this area for the past four decades and huge progress w ith encouraging results has been obtained. The goal of this paper is to provide a survey of recentholistic and feature based approaches that complement previous surveys. Current face recognition systems have already reached a certain level of maturity when operating under constrained conditions. However, we are still far from achieving the ideal and adequate results in all the various situations. Still more advances need to be done in the technology regarding the sensitivity of the face images to environmental conditions like illumination, occlusion, time-delays, pose orientations, facial expressions. Furthermore, research work on 3 D face recognition and face recognition in videos is also pacing parallel. However, the error rates of current face recognition systems are still too high for many of the applications. So, the researchers still need to go far to get accurate face recognitions.REFERENCES[1] T. Y oung, K-S Fu, Handbook of pattern recognition and image processing, Academic Press, 1986.[2] M. Turk, A. Pentland, Eigenfaces for recognition, Journal Cognitive Neuroscience, 3(1) (1991)71-86.[3] G. Loizou, S.J. Maybank, The nearest neighbor and the Bayes error rates, IEEE Trans. Patt. Anal. & Mach. Intell., vol.9 (1987)254-262,.[4] Seema Asht, Rajeshwar Dass, Dharmendar, “Pattern Recognition Techniques”, International Journal of Science, Engineering and Computer Technology, vol. 2, pp. 87-88, March 2012. [4] M. Kirby, L. Sirovich, “Application of the Karhunen-Loeve Procedure for the Characterization of Human Faces”, IEEE Transactions on Pattern Analysis and Machine Intelligence, vol. 12, No. 1, pp. 103-108, January 1990.[5] M. Turk and A. Pentland. “Face recognition using eigenfaces”. In Proceedings of the IEEE Conference on Computer V ision and Pattern Recognition, 1991[6] Face Recognition : National Science and Technology Council (NSTC) , Committee on Technology, Committee on Homeland and National Security, Subcommittee on biometrics.[7] N. Sun, H. Wang, Z. Ji, C. Zou, and L. Zhao, "An efficient algorithm for Kernel two-dimensional principal component analysis," Neural Computing & Applications, vol.17, pp.59-64, 2008.[8] Q. Y ang aand X. Q. Ding, "Symmetrical Principal Component Analysis and Its Application in Face Recognition," Chinese Journal of Computers, vol.26, pp.1146–1151, 2003.[9] J. Y ang and D. Zhang, "Two-Dimensional PCA: A New Approach to Appearance-Based Face Representation and Recognition," IEEE Trans. Pattern Analysis and Machine Intelligence, vol.28, pp.131- 137, 2004.[10] K. R. Tan and S. C. Chen, "Adaptively weighted subpattern PCA for face recognition," Neurocomputing, vol.64, pp.505-511, 2005.外文资料译文一种基于PCA和子模式改进的混合人脸识别技术A.R Kulkarni, D.S Bormane摘要: 本文基于PCA人脸识别新技术实现的。
关于人脸识别的英语短文----人脸识别是一把双刃剑
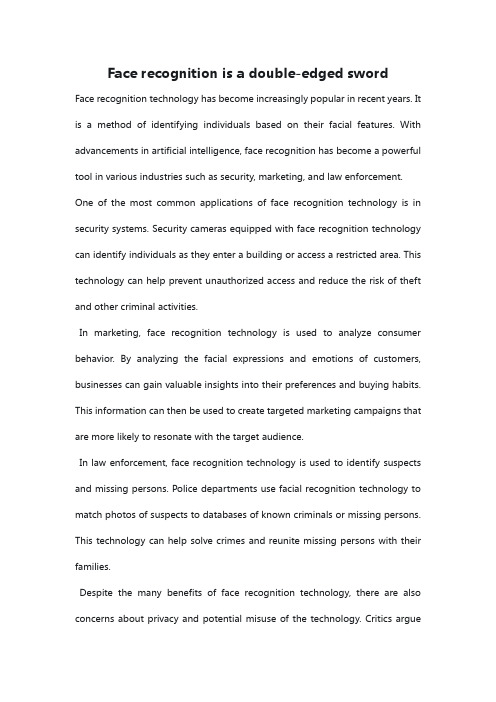
Face recognition is a double-edged swordFace recognition technology has become increasingly popular in recent years. It is a method of identifying individuals based on their facial features. With advancements in artificial intelligence, face recognition has become a powerful tool in various industries such as security, marketing, and law enforcement. One of the most common applications of face recognition technology is in security systems. Security cameras equipped with face recognition technology can identify individuals as they enter a building or access a restricted area. This technology can help prevent unauthorized access and reduce the risk of theft and other criminal activities.In marketing, face recognition technology is used to analyze consumer behavior. By analyzing the facial expressions and emotions of customers, businesses can gain valuable insights into their preferences and buying habits. This information can then be used to create targeted marketing campaigns that are more likely to resonate with the target audience.In law enforcement, face recognition technology is used to identify suspects and missing persons. Police departments use facial recognition technology to match photos of suspects to databases of known criminals or missing persons. This technology can help solve crimes and reunite missing persons with their families.Despite the many benefits of face recognition technology, there are also concerns about privacy and potential misuse of the technology. Critics arguethat face recognition technology could be used to monitor individuals without their knowledge or consent. There is also the potential for the technology to be used to discriminate against certain groups of people.In conclusion, face recognition technology has the potential to revolutionize various industries and improve security measures. However, it is important to consider the potential risks and ethical implications of the technology. As we continue to develop and implement this technology, it is crucial to ensure that it is used in a responsible and ethical manner.译文:人脸识别是一把双刃剑近年来,人脸识别技术越来越流行。
人脸识别英文作文
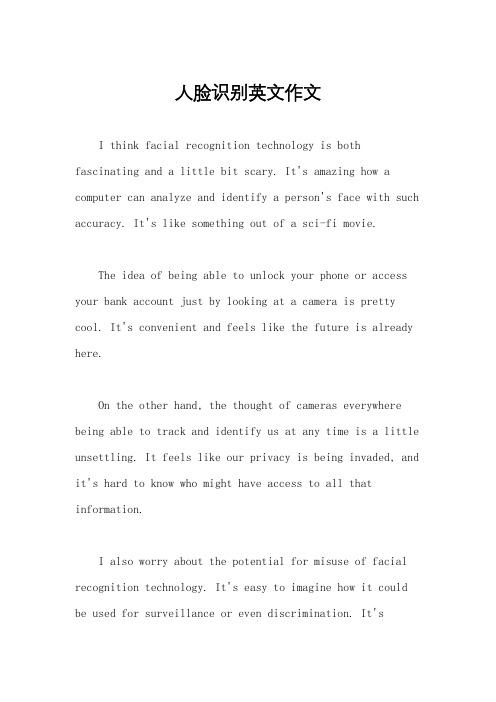
人脸识别英文作文I think facial recognition technology is both fascinating and a little bit scary. It's amazing how a computer can analyze and identify a person's face with such accuracy. It's like something out of a sci-fi movie.The idea of being able to unlock your phone or access your bank account just by looking at a camera is pretty cool. It's convenient and feels like the future is already here.On the other hand, the thought of cameras everywhere being able to track and identify us at any time is a little unsettling. It feels like our privacy is being invaded, and it's hard to know who might have access to all that information.I also worry about the potential for misuse of facial recognition technology. It's easy to imagine how it could be used for surveillance or even discrimination. It'simportant to have regulations in place to protect people from these kinds of abuses.At the same time, there are also some really positive uses for facial recognition. For example, it can help law enforcement identify criminals or find missing persons. It can also be used for security in places like airports and government buildings.Overall, I think facial recognition technology has alot of potential, but it's important to proceed with caution. We need to balance the benefits with the potential risks and make sure that people's rights and privacy are protected.。
人脸识别的作文英文

人脸识别的作文英文Face recognition technology is becoming more and more common in our daily lives. It can be used for security purposes, such as unlocking smartphones or accessing secure areas. It can also be used for more personal reasons, like tagging friends in photos on social media.Some people have concerns about the privacyimplications of face recognition technology. They worrythat their faces could be scanned and stored without their consent, leading to potential misuse of their personal information.On the other hand, advocates of face recognition technology argue that it can help make our lives more convenient and secure. For example, it can help law enforcement agencies identify suspects more quickly and accurately.One of the biggest challenges with face recognitiontechnology is ensuring its accuracy. There have been cases where the technology has misidentified individuals, leading to false accusations and misunderstandings.Despite its potential drawbacks, face recognition technology is likely to become even more prevalent in the future. As the technology continues to improve, it will be interesting to see how it is used and regulated indifferent parts of the world.。
- 1、下载文档前请自行甄别文档内容的完整性,平台不提供额外的编辑、内容补充、找答案等附加服务。
- 2、"仅部分预览"的文档,不可在线预览部分如存在完整性等问题,可反馈申请退款(可完整预览的文档不适用该条件!)。
- 3、如文档侵犯您的权益,请联系客服反馈,我们会尽快为您处理(人工客服工作时间:9:00-18:30)。
①现代的人脸识别,特指通过分析、比较人脸视觉特征信息进行身份鉴别的计算机技术。
具体而言,就是通过视频采集设备获取识别对象的面部图像,再利用核心的算法对其脸部的五官位置、脸型和角度进行计算分析,进而和自身数据库里已有的范本进行比对,最后判断出用户的真实身份。
这是一项高端的计算机图像处理技术。
②在全球范围内,人脸识别系统的研究始于20 世纪60 年代。
人脸识别的优势在于其自然性和不被被测个体察觉的特点。
所谓自然性,是指该识别方式同人类(甚至其他生物)进行个体识别时所利用的生物特征相同。
人脸识别就是通过观察比较人脸来区分和确认身份的。
不被察觉的特点会使该识别方法不令人反感,并且因为不容易引起人的注意而不易被欺骗。
相对于指纹识别而言,人脸识别还具有非接触式(非侵犯式)的特点,因此更加友好、自然,更易被人们接受。
③随着科技的发展,人脸识别技术的应用已经不是什么新鲜事了。