Oracle大数据平台最佳实践
大数据日志分析实验报告
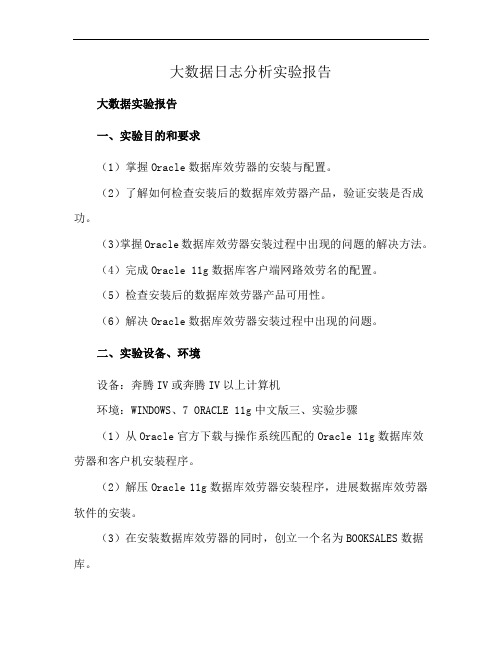
大数据日志分析实验报告大数据实验报告一、实验目的和要求(1)掌握Oracle数据库效劳器的安装与配置。
(2)了解如何检查安装后的数据库效劳器产品,验证安装是否成功。
(3)掌握Oracle数据库效劳器安装过程中出现的问题的解决方法。
(4)完成Oracle 11g数据库客户端网路效劳名的配置。
(5)检查安装后的数据库效劳器产品可用性。
(6)解决Oracle数据库效劳器安装过程中出现的问题。
二、实验设备、环境设备:奔腾IV或奔腾IV以上计算机环境:WINDOWS、7 ORACLE 11g中文版三、实验步骤(1)从Oracle官方下载与操作系统匹配的Oracle 11g数据库效劳器和客户机安装程序。
(2)解压Oracle 11g数据库效劳器安装程序,进展数据库效劳器软件的安装。
(3)在安装数据库效劳器的同时,创立一个名为BOOKSALES数据库。
(4)安装完数据库效劳器程序后,解压客户机程序,并进展客户机的安装。
(5)安装完客户机程序后,启动客户机的“Net Configuration Assistant",进展本地NET效劳名配置,将数据库效劳器中的BOOKSALES 数据库配置到客户端。
(6)启动OEM管理工具,登录、查看、操作BOOKSALES数据库。
(7)启动SQL Plus工具,分别以SYS用户和SYSTEM用户登录BOOKSALES数据库。
三、实验步骤(1)向BOOKSALES数据库的USERS表空间添加一个大小为10MB的数据文件users02(2)向BOOKSALES数据库的TEMP表空间添加一个大小为10MB的临时数据文件temp02.(3)向BOOKSALES数据库的间中添加一个可以自动扩展的数据文件user03大小5M,每次扩展IM,最大容量为100M.(4)取消BOOKSALES数据库数据文件user03.的自动扩展。
(5)将BOOKSALES数据库数据文件users02.更名为users002.(6)查询BOOKSALES数据库当前所有的数据文件的详细信息。
oracle数据库性能调优
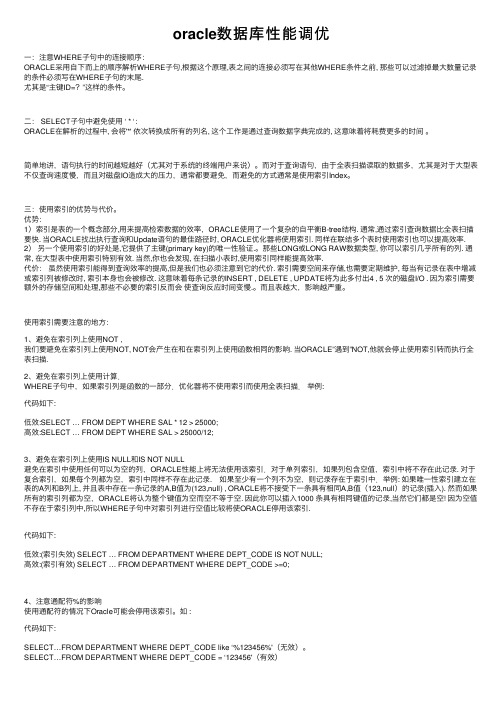
oracle数据库性能调优⼀:注意WHERE⼦句中的连接顺序:ORACLE采⽤⾃下⽽上的顺序解析WHERE⼦句,根据这个原理,表之间的连接必须写在其他WHERE条件之前, 那些可以过滤掉最⼤数量记录的条件必须写在WHERE⼦句的末尾.尤其是“主键ID=?”这样的条件。
⼆: SELECT⼦句中避免使⽤ ‘ * ‘:ORACLE在解析的过程中, 会将'*' 依次转换成所有的列名, 这个⼯作是通过查询数据字典完成的, 这意味着将耗费更多的时间。
简单地讲,语句执⾏的时间越短越好(尤其对于系统的终端⽤户来说)。
⽽对于查询语句,由于全表扫描读取的数据多,尤其是对于⼤型表不仅查询速度慢,⽽且对磁盘IO造成⼤的压⼒,通常都要避免,⽽避免的⽅式通常是使⽤索引Index。
三:使⽤索引的优势与代价。
优势:1)索引是表的⼀个概念部分,⽤来提⾼检索数据的效率,ORACLE使⽤了⼀个复杂的⾃平衡B-tree结构. 通常,通过索引查询数据⽐全表扫描要快. 当ORACLE找出执⾏查询和Update语句的最佳路径时, ORACLE优化器将使⽤索引. 同样在联结多个表时使⽤索引也可以提⾼效率. 2)另⼀个使⽤索引的好处是,它提供了主键(primary key)的唯⼀性验证.。
那些LONG或LONG RAW数据类型, 你可以索引⼏乎所有的列. 通常, 在⼤型表中使⽤索引特别有效. 当然,你也会发现, 在扫描⼩表时,使⽤索引同样能提⾼效率.代价:虽然使⽤索引能得到查询效率的提⾼,但是我们也必须注意到它的代价. 索引需要空间来存储,也需要定期维护, 每当有记录在表中增减或索引列被修改时, 索引本⾝也会被修改. 这意味着每条记录的INSERT , DELETE , UPDATE将为此多付出4 , 5 次的磁盘I/O . 因为索引需要额外的存储空间和处理,那些不必要的索引反⽽会使查询反应时间变慢.。
⽽且表越⼤,影响越严重。
使⽤索引需要注意的地⽅:1、避免在索引列上使⽤NOT , 我们要避免在索引列上使⽤NOT, NOT会产⽣在和在索引列上使⽤函数相同的影响. 当ORACLE”遇到”NOT,他就会停⽌使⽤索引转⽽执⾏全表扫描.2、避免在索引列上使⽤计算.WHERE⼦句中,如果索引列是函数的⼀部分.优化器将不使⽤索引⽽使⽤全表扫描.举例:代码如下:低效:SELECT … FROM DEPT WHERE SAL * 12 > 25000;⾼效:SELECT … FROM DEPT WHERE SAL > 25000/12;3、避免在索引列上使⽤IS NULL和IS NOT NULL避免在索引中使⽤任何可以为空的列,ORACLE性能上将⽆法使⽤该索引.对于单列索引,如果列包含空值,索引中将不存在此记录. 对于复合索引,如果每个列都为空,索引中同样不存在此记录. 如果⾄少有⼀个列不为空,则记录存在于索引中.举例: 如果唯⼀性索引建⽴在表的A列和B列上, 并且表中存在⼀条记录的A,B值为(123,null) , ORACLE将不接受下⼀条具有相同A,B值(123,null)的记录(插⼊). 然⽽如果所有的索引列都为空,ORACLE将认为整个键值为空⽽空不等于空. 因此你可以插⼊1000 条具有相同键值的记录,当然它们都是空! 因为空值不存在于索引列中,所以WHERE⼦句中对索引列进⾏空值⽐较将使ORACLE停⽤该索引.代码如下:低效:(索引失效) SELECT … FROM DEPARTMENT WHERE DEPT_CODE IS NOT NULL;⾼效:(索引有效) SELECT … FROM DEPARTMENT WHERE DEPT_CODE >=0;4、注意通配符%的影响使⽤通配符的情况下Oracle可能会停⽤该索引。
Oracle Exadata数据库一体机极致性能和最佳实践

Exadata 采用更高的单路带宽
- InfiniBand提供40Gb/s的带宽,比高端阵列 的光纤通道技术快8倍
Exadata 提供更高的IOPS
- 智能Exadata Smart Flash Cache技术处理更 多的IOPS
• 冗余40Gb/s 交换机 • 统一的服务器和存储网络
• 5.3 TB PCI 闪存 • 跨服务器进行存储镜像
© 2010 Oracle Corporation – Oracle Confidential
CONFIDENTIAL – ORACLE HIGHLY RESTRICTED
6
– 6–
Exadata X2 体系架构
CONFIDENTIAL – ORACLE HIGHLY RESTRICTED
4
前所未有的交钥匙方案:
Sun Oracle Exadata X2数据库机
• 完整的, 预配置的, 严格测 试的系统提供极限性能 • 随付即用的系统 • 高性能、高可用性
© 2010 Oracle Corporation – Oracle Confidential
Copyright © 2009, Oracle Corporation and/or its affiliates
CONFIDENTIAL – ORACLE HIGHLY RESTRICTED
8
– 8–
Exadata数据库一体机创新的技术架构
集中管理平台
三层架构/两层应用 架构
客户端 避免单点故障
智能存储层 1M IOPS/机架
Exadata Cell
Exadata Cell
Oracle数据集成方案
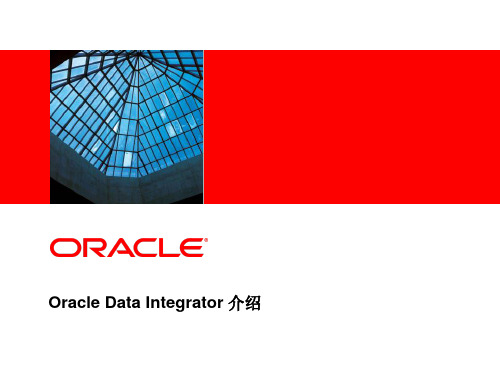
Oracle BI Suite EE
Interactive Dashboards
Publisher
Oracle BI Presentation Server
Oracle BI Server
Delivers
Oracle BI Enterprise Data
Warehouse
Bulk E-LT
Oracle Data Integrator
ERPBiblioteka CRM业务系统ODI是一个ETL工具
数据整合
数据源 抽取管理
ETL 作业调度
信息发现与管理
信息共享
数据存储管理
信息模型
数据展现
数据库管理
元数据管理
安全访问控制
用户
业务系统 业务系统
业务系统 业务系统
Reconciliation Standardization & Transform
抽取转换
ETL 解决方案
数据集成
Message
Id Name
City
Duplicated Record
001 John Doe New York
Duplicated Record
022 John Doe Boston
Invalid City Reference 230 Albert Fresh Maris
• 数据完整性防火墙 • 审计,清洗和回收
可执行代码
• 120多个知识模块(非黑 盒的)
✓ 开发和利用最佳实践 ✓ 简化管理工作 ✓ 减少拥有成本
• 客制化和扩展性
热插拔的知识模块架构
Reverse Engineer Metadata
Journalize Read from CDC
一体化方案解决大数据处理的两个难题
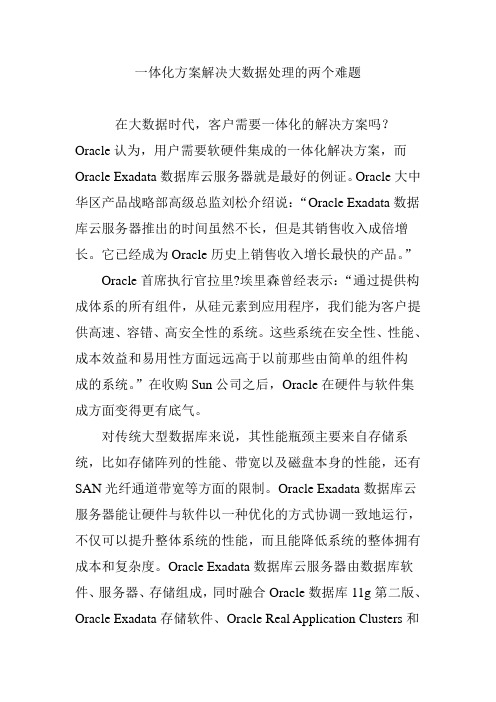
一体化方案解决大数据处理的两个难题在大数据时代,客户需要一体化的解决方案吗?Oracle认为,用户需要软硬件集成的一体化解决方案,而Oracle Exadata数据库云服务器就是最好的例证。
Oracle大中华区产品战略部高级总监刘松介绍说:“Oracle Exadata数据库云服务器推出的时间虽然不长,但是其销售收入成倍增长。
它已经成为Oracle历史上销售收入增长最快的产品。
”Oracle首席执行官拉里?埃里森曾经表示:“通过提供构成体系的所有组件,从硅元素到应用程序,我们能为客户提供高速、容错、高安全性的系统。
这些系统在安全性、性能、成本效益和易用性方面远远高于以前那些由简单的组件构成的系统。
”在收购Sun公司之后,Oracle在硬件与软件集成方面变得更有底气。
对传统大型数据库来说,其性能瓶颈主要来自存储系统,比如存储阵列的性能、带宽以及磁盘本身的性能,还有SAN光纤通道带宽等方面的限制。
Oracle Exadata数据库云服务器能让硬件与软件以一种优化的方式协调一致地运行,不仅可以提升整体系统的性能,而且能降低系统的整体拥有成本和复杂度。
Oracle Exadata数据库云服务器由数据库软件、服务器、存储组成,同时融合Oracle数据库11g第二版、Oracle Exadata存储软件、Oracle Real Application Clusters和Sun FlashFire技术,并采用40Gb InfiniBand内部连接,因此更适合数据仓库和高速联机交易处理等应用。
大数据对数据存储和智能分析都提出了更高的要求。
Oracle Exadata数据库云服务器将存储的软硬件与数据仓库软件有机地结合在一起,通过预调试,优化了系统的整体性能,方便用户部署和使用。
刘松认为,Oracle Exadata数据库云服务器的出现也简化了数据中心架构。
现在,客户只要使用一台Oracle Exadata数据库云服务器加一台Exalogic Elastic Cloud(Oracle即将推出的中间件云服务器),就可以取代原来数量众多的应用服务器和数据库服务器。
Oracle数据库性能优化与案例分析
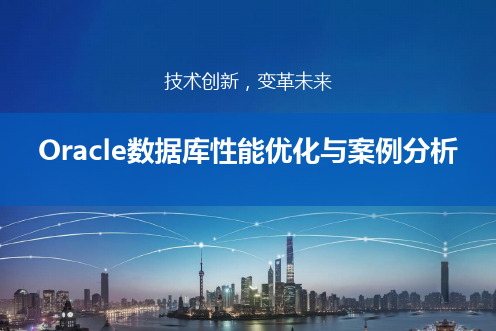
Oracle数据库性能优化与案例分析
性能优化探讨
• 原因:为什么? • 慢(响应时间) • 慢(吞吐量)
性能优化探讨
• 目的:为了什么? • 快(响应时间) • 快(吞吐量)
性能优化之案例分析
• 案例之方法论 • 案例之登录访问 • 案例之资源 • 案例之锁
性能优化方法论发展
• 登录输入指标测量 • Logons:= EndSnap. logons cumulative– StartSnap. logons
cumulative。 • Logons Per Second:= Logons / TimeInterval
案例之登录访问
登录输出指标测量:
Logon Response Time:= Network Response Time * 10 + Native TCP Logon :=Network Response Time * 10 + Listener Response Time + Native IPC Logon Time 。
案例之登录访问
• 例:
•
某医院HIS业务系统的账户登录操作异常缓慢,部分情况下
甚至会出现长时间的卡壳情况,业务影响主要发生在每天早上
的上班时刻。
案例之登录访问
优化过程: • 账户登录过程一般涉及到在账户表格以及对应日志表格上的冲
突,比如Buffer busy waits或者TX lock。AWR未体现该特征。 • AWR报告显示connection management call elapsed time时间偏长
成功率:98% 高 失败率:2% 低
失败人数:500*2%=10
Oracle的SiebelCRM解决方案
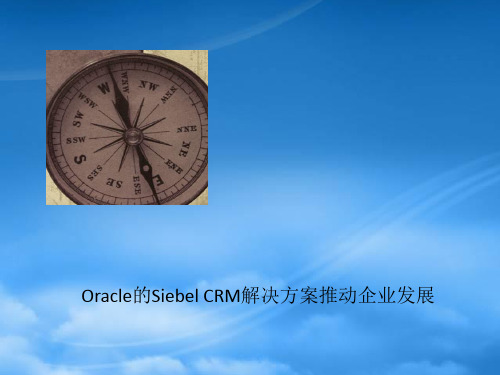
Oracle CRM:全球最好的客户关系管理系统
跨行业解决方案
商业智能
客户数据整合
商业流程整合
套装
行业解决方案
托管式
定制
交付选择
最多的成功案例 最佳的销售和最多的实施最佳的客户结果跨行业最多的客户成功
为什么 Oracle 是最佳的合作伙伴
最强功能的软件
最有力的专家意见
洞察力驱动升等销售和交叉销售
洞察力驱动的销售绩效
行业特定的线索到签单流程
GARTNER 2004 CRM
评估象限 地位
2004 IDC 营销和销售联合CMO奖
2004 Gartner 评估象限和地位
METASpectrum 2004 CRM 应用套件评估
行业荣誉
“The real battle is for the number two spot.”—Liz Roche, META
现场服务
B2B CRM
客户服务 & 支持
B2C CRM
销售
Oracle/Siebel
Oracle/Siebel
Oracle/Siebel
Oracle/Siebel
Oracle/Siebel
Oracle/Siebel
CDI
最全面的应用功能
公认产品-分析师
划算的全面CRM,可被业务用户配置
Shared service offering共享服务部署迅速内置分析和CRM最佳实践
On Premise
Private Hosted
On Premise & Hosted Combined
Shared Hosting
大数据成功案例
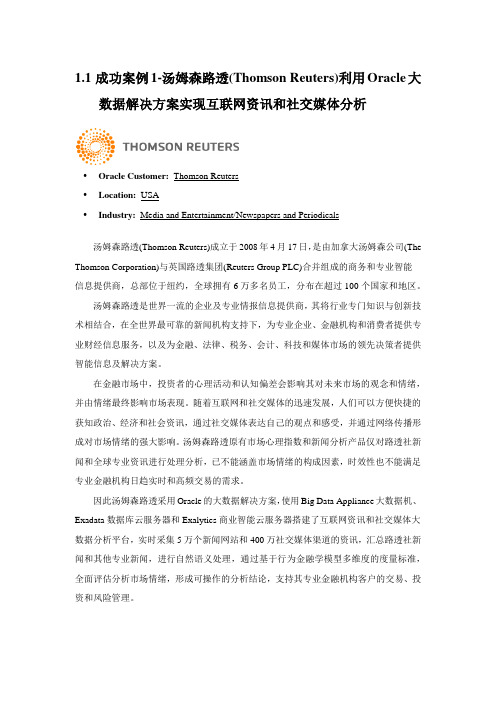
1.1成功案例1-汤姆森路透(Thomson Reuters)利用Oracle大数据解决方案实现互联网资讯和社交媒体分析•Oracle Customer: Thomson Reuters•Location: USA•Industry: Media and Entertainment/Newspapers and Periodicals汤姆森路透(Thomson Reuters)成立于2008年4月17日,是由加拿大汤姆森公司(The Thomson Corporation)与英国路透集团(Reuters Group PLC)合并组成的商务和专业智能信息提供商,总部位于纽约,全球拥有6万多名员工,分布在超过100个国家和地区。
汤姆森路透是世界一流的企业及专业情报信息提供商,其将行业专门知识与创新技术相结合,在全世界最可靠的新闻机构支持下,为专业企业、金融机构和消费者提供专业财经信息服务,以及为金融、法律、税务、会计、科技和媒体市场的领先决策者提供智能信息及解决方案。
在金融市场中,投资者的心理活动和认知偏差会影响其对未来市场的观念和情绪,并由情绪最终影响市场表现。
随着互联网和社交媒体的迅速发展,人们可以方便快捷的获知政治、经济和社会资讯,通过社交媒体表达自己的观点和感受,并通过网络传播形成对市场情绪的强大影响。
汤姆森路透原有市场心理指数和新闻分析产品仅对路透社新闻和全球专业资讯进行处理分析,已不能涵盖市场情绪的构成因素,时效性也不能满足专业金融机构日趋实时和高频交易的需求。
因此汤姆森路透采用Oracle的大数据解决方案,使用Big Data Appliance大数据机、Exadata数据库云服务器和Exalytics商业智能云服务器搭建了互联网资讯和社交媒体大数据分析平台,实时采集5万个新闻网站和400万社交媒体渠道的资讯,汇总路透社新闻和其他专业新闻,进行自然语义处理,通过基于行为金融学模型多维度的度量标准,全面评估分析市场情绪,形成可操作的分析结论,支持其专业金融机构客户的交易、投资和风险管理。
- 1、下载文档前请自行甄别文档内容的完整性,平台不提供额外的编辑、内容补充、找答案等附加服务。
- 2、"仅部分预览"的文档,不可在线预览部分如存在完整性等问题,可反馈申请退款(可完整预览的文档不适用该条件!)。
- 3、如文档侵犯您的权益,请联系客服反馈,我们会尽快为您处理(人工客服工作时间:9:00-18:30)。
Colin Cunningham, IntelKumaran Siva, IntelSandeep Mahajan, Oracle03-Oct-20174:45 p.m. -5:30 p.m. | Moscone West -Room 3020Exploring New SSD Usage Models to Accelerate Cloud Performance –03-Oct-2017, 3:45 -4:30PM, Moscone West –Room 30201.10 min –ScottOracle Big Data solution2.15 min –DanielNVMe and NVMEoF, SPDK3.15 min –Sunil, Case StudyApache Spark and TeraSort4. 5 min -QA Best Practices for Big Data in the Cloud -03-Oct-2017, 4:45 -5:30PM, Moscone West -Room 30201.10 min -SandeepOracle Big Data solution2.15 min –SivaFPGA enables new storage use cases 3.15 min –Colin, Case StudyApache Spark, Big Data Analytics 4. 5 min -QABig Data TalkOracle Safe Harbor StatementThe following is intended to outline our general product direction. It is intended for information purposes only, and may not be incorporated into any contract. It is not a commitment to deliver any material, code, or functionality, and should not be relied upon in making purchasing decisions. The development, release, and timing of any features or functionality described for Oracle’s products remains at the sole discretion of Oracle.Intel Notices & DisclaimersIntel technologies’ features and benefits depend on system configuration and may require enabled hardware, software or servic e activation. Performance varies depending on system configuration. Check with your system manufacturer or retailer or learn more at .No computer system can be absolutely secure.Tests document performance of components on a particular test, in specific systems. Differences in hardware, software, or configuration will affect actual performance. Consult other sources of information to evaluate performance as you consider your purchase. For more complete information about performance and benchmark results, visit /performance. Software and workloads used in performance tests may have been optimized for performance only on Intel microprocessors. Performance tests, such as SYSmark and MobileMark, are measured using specific computer systems, components, software, operations and functions. Any change to any of those factors may cause the results to vary. You should consult other information and performance tests to assist you in fully evaluating your contemplated purchases, including the performance of that product when combined with other products.For more complete information visit /performance.Cost reduction scenarios described are intended as examples of how a given Intel-based product, in the specified circumstances and configurations, may affect future costs and provide cost savings.Circumstances will vary.Intel does not guarantee any costs or cost reduction.Intel does not control or audit third-party benchmark data or the web sites referenced in this document. You should visit the referenced web site and confirm whether referenced data are accurate.© 2017 Intel Corporation.Intel, the Intel logo, and Intel Xeon are trademarks of Intel Corporation in the U.S. and/or other countries.*Other names and brands may be claimed as property of others.Develop & DeployIntegrate & Extend Oracle Cloud PlatformAnalyze & PredictSecure & ManageInnovate with a Comprehensive, Open, Integrated and Hybrid Cloud Platformthat is Highly Scalable, Secure and Globally AvailablePublish & EngageData Management Oracle Cloud PlatformIdentity & Security Application DevelopmentContent & Experience Systems ManagementAnalytics and Big Data HybridComprehensiveOpenIntegrated Oracle Data CenterOracle Public CloudYour Data CenterOracle Cloud at Customer Enterprise Integration Data IntegrationBuilt on High Performant Oracle Cloud InfrastructureOracle Cloud Platform Momentum14,000+OracleCustomers $1.4BillionFY17 Oracle Cloud Revenue(60% YoY Growth )3,000+Apps in theMarketplace10PaaSCategories where Leader Oracle is a Industry Cloud PlatformOracle CloudAnalystsAccording toPlatformOracle Big Data as a Service •Work with a stack you are familiar with•Maximum portability •Maximum performance–I/O tuned for Oracle Cloud–OS tuned for Oracle Cloud–Network tuned for Oracle Cloud•Elastic and Scalable–Big Data clusters are elastic on demand –Storage is scaled independently–Choose appropriate compute shapesfor your workload •Managed–Automated lifecycle management–Service monitoring via dashboards or REST APIsOracle Cloud Platform does the grunt workBig Data Cloud Service ManagementCopyright © 2017, Oracle and/or its affiliates. All rights reserved.1112FPGA Technology IntroductionMEMORY INTERFACES PARTIAL RECONFIGURATION Allows separate regionsMemory Interface PR Region 0Configurable high performance memory interfacesNetwork InterfaceHardened controllersPCIE HOST INTERFACEPCIe(e.g. Platform) Hardened + Soft host interfaceHardened PCIe controller Soft interface allows different use models and driversNETWORK INTERFACE Configurable network interfacesPR Region 1 (e.g. Application)Hard/soft interfacesMEMORY BLOCKS LOGIC ELEMENTS Thousands of 20Kb memory blocks Allows processing to stay on-chipMain programmable componentMillions of logic elements Simple logic, adders, and registers Interconnect with configurable fabricVARIABLE PRECISION DSP BLOCKS Allows FPGA to perform compute intensive functionsWhere FPGAs Fit In?FLEXIBILITY EFFICIENCYCPUGPUFPGAASIC• Balanced architecture: Good enough most workloads • Good single thread & throughput perf. • Fastest cadence• Focused on compute throughput • Many low performance threads • High memory throughput • Purpose made programming tools• Full custom pipeline • Capable of networking and compute • High memory throughput • Change cadence in months rapidly changing needs • Requires sophistication• Fixed function • High efficiency – only blocks that are needed • Change cadence in years needs stable standards • Expensive: minimum volume for viability • Requires sophistication14Potential FPGA Uses Across The DatacenterNFV Node Compute NodeStorage NodeFPGAStd NICCPU IA CPU IA CPU IA CoreCPU IA CPU IA CPU IA CoreOptics OpticsStorage NetworkingStorage Stack Media InterfaceStd NICCore CoreOptics OpticsVirtual Networking Firewall Load BalancingOptics OpticsNetworking, Security Storage Distributed App Accel NFV Node: Provides infrastructure networking functions Compute Node: Provides Infrastructure including storage networking/stack acceleration Storage Node: Offloads stack, networking, and interfaces to media HDD, SDD, NVMe Acceleration Node: Focused on high Large FPGACore CoreFPGAOptics OpticsCore CoreFPGAFPGACPU IA CPU IA CPU IA CoreApp AceleratorMachine LearningTranscodeAccelerator NodeCPU IA CPU IA CPU IA CoreCore CoreFPGA Value Proposition For Storage High Bandwidth & Low Latency Driven by NVMe technology Optimized storage stack & networking FPGA can be used to implement storage stack and networking protocol layers Rapidly evolving standards FPGA adaptable to different protocols, stacks, and host interfaces16Example FPGA For Initiator & TargetDC Networking System Integration Simplicity No Drivers Required, Config SW runs from Console FPGA Future Proof SolutionFPGA solution can change with Standards, AFU capability (IPSEC, Compression)Scalable NVMe Solution NVMeof compliant18The Analytic WorkloadWe want to execute analytics in the cloud leveraging today’s modern Hadoop framework with SparkCleanse Aggregate Transform Unify Load Analytics Reporting Machine Learning GraphStructured Data Unstructured Data Semi-Structured DataOur study will examine such an analytic pipeline over 3 phases (1) Import create, write data to HDFS (2) Load into Hive (3) Analyze data and report19Spark ResourcingSpark worker nodes live in Hadoop as data nodes The data node transforms from a physical server into a virtual server in our cloudSpark Tunings: Number of Spark Executors Cores per Executor Memory per executor Executor Memory Overhead Partitions, parallelism, … etc., etc.Top diagram from /docs/latest/img/cluster-overview.pngE1 E25 VCPUs 27GB memory 3GB memoryOverheaddeployment example having 3 Spark executors on each 8-core/16-VCPU virtual machine…E3 vm1 vm2 vmn20VCPUs used: 15/16 Memory Used: 99GB/120GBBig Data Run—Hard Drive vs Local NVMePlatform2S Intel Xeon E5-2699 v4768 GB DRAM. 10Gbps Ethernet.2S Intel Xeon E5-2699 v4768 GB DRAM. 10Gbps Ethernet.Storage7 x Hard Drives (2TB, 7200 RPM) 2 x Intel NAND NVMeOS/ Hypervisor Centos7.2 /KVMCentos7.2 /KVMBig Data SW Hortonworks Data Platform 2.4Hortonworks Data Platform 2.4Big Data Cluster18 Datanode VMs (16 VCPUs120 GB memory)2 Namenode VMs (4 VCPUs 30 GB memory)18 Datanode VMs (16 VCPUs,120 GB memory)2 Namenode VMs (4 VCPUs 30GB memory)Server 1Server 2Server 3Server 4ToRServer 1Server 2Server 3Server 4ToRBroadwell+ HDD Broadwell+ NVMeIO service time likewise suffersBig Data Performance Obstacle: Local Hard Drives Input/OutputLocal HDD leaves CPU waiting for dataImport and Load Phase most IO-intensive and stand to gain from new storage technologiesNVMe Impact to BDaaS2.5x acceleration of Import phaseachieved by replacing hard driveswith NVMe SSD on the same cluster2.5xIO wait is virtually extinguished withthe adoption of Intel NVMe SSDsBig Data Run—Storage Area Network vs Local NVMePlatform2S Intel Xeon E5-2699 v4768 GB DRAM. 10Gbps Ethernet.2S Intel Xeon E5-2699 v4768 GB DRAM. 10Gbps Ethernet.Storage Enterprise Storage Area Network 2 x Intel NAND NVMeOS/ Hypervisor OEL 6.6 /XenOEL 6.6 /XenBig Data SW Hortonworks Data Platform 2.4Hortonworks Data Platform 2.4Big Data Cluster18 Datanode VMs (16 VCPUs120 GB memory)2 Namenode VMs (4 VCPUs 30 GB memory)18 Datanode VMs (16 VCPUs,120 GB memory)2 Namenode VMs (4 VCPUs 30GB memory)Server 1Server 2Server 3Server 4ToRServer 1Server 2Server 3Server 4ToRBroadwell + SAN Broadwell+ NVMeTraditional SANSharedStorageNVMe here yields a 1.3x acceleration in time to deliver our data into HDFSBig Data Performance Obstacle:NetworkIO performance suffers if storage over network tests capacity. Local NVMe reduces congestionAt left our 10GbE NIC limited IO when using a SAN appliance. Local NVMe gives Hadoop data nodes a faster path to data1.3x45 min 34 mingap = HDFS write over the networkAnalytics Scale with Intel Xeon Scalable Processors Analytics need compute. Machine Learning and SQL ranat 1.37x the velocity over the prior generation usingXeon Platinum•Skylake has up to 28 cores per socket•Broadwell has up to 22 cores per socket•Skylake microarchitecture improvements:•improved cache hierarchy for cloud workloads• 1.5X memory bandwidthIntel Xeon Scalable Family lets you do more analytics inevery serverCustomers build and use Big Data platforms to enhance and expedite decision making, derive insights and take actionShorten the time to move and process data and expand opportunity for impactful analysis Intel NVMe and Xeon Scalable Processors do just that. Leaving you time to innovate —”Go off and do something wonderful”Hortonworks Data Platform 2.4 on CentOS 7.2 with KVM data node VMs (16VCPU/120GB RAM)2 name node VMs (4VCPU/30GB RAM) running executing 3TB data set on analytics workflow as:(1)4 x 2S Intel Xeon E5-2699v4 with 7x 1.8TB SATA HDDs (2)4 x 2S Intel Xeon E5-2699v4 with 2x 1.5TB P3700 NVMe(3)4 x 2S Intel Xeon SP 8170 with 2x 1.5 TB Intel P4600 NVMe (3D NAND)Raise Analytic Productivity withIntel NVMe and Xeon Scalable Processors33%53%49%% of time doing AnalyticsBig Data Performance Summary•Intel NVMe SSDs populate HDFS faster• 2.5x faster than with hard drives by removing the IO bottleneck• 1.3x faster than with a storage area network by relieving network congestion •Skylake accelerates Machine Learning and SQL by 1.37x•Do more Analytics with Intel NVMe and Xeon Scalable Processors •53% of time in big data pipeline doing analytics, +20% over the previous generationOracle and IntelCustomers adopting both Hadoop and cloud services at a rapid rate Oracle and Intel cloud partnership has extended to optimize Big Data You can do it yourself but Oracle has made Big Data simpleUp to 3.5X faster performance on Oracle BDCSCE over DIY HadoopBoth clusters utilized 18 data node VMs (16VCPU and 120GB RAM) and 2 name node VMs (4VCPU and 30GB RAM), on 4 x 2S Intel Xeon E5-2699 v4 running either Oracle Big Data Cloud Services Compute Edition or DIY (Hortonworks Data Platform 2.4 on CentOS 7.2 with KVM, with 7x2.0TB 7200RPM drives per system )0.511.522.533.54TeraSortAnalytics workloadPerformance onOracle Big Data Cloud ServiceDIY Hadoop with HDD Oracle Big Data Cloud Service Compute Edition3.5X2X。