机器学习_Trains Data Set(火车数据集)
train validation test 划分
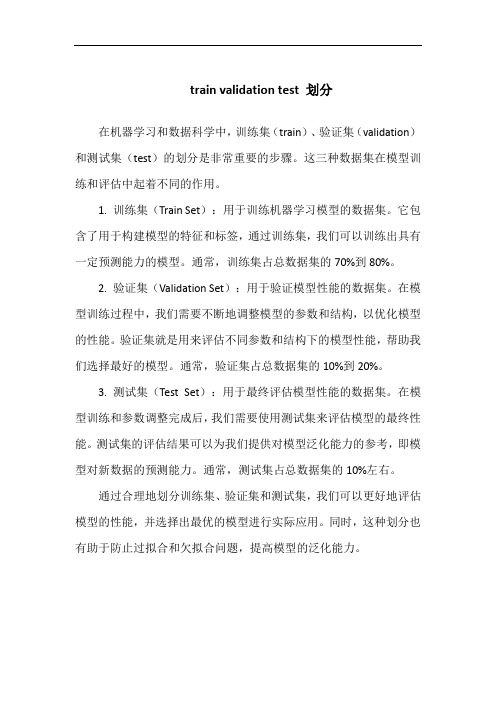
train validation test 划分
在机器学习和数据科学中,训练集(train)、验证集(validation)和测试集(test)的划分是非常重要的步骤。
这三种数据集在模型训练和评估中起着不同的作用。
1. 训练集(Train Set):用于训练机器学习模型的数据集。
它包含了用于构建模型的特征和标签,通过训练集,我们可以训练出具有一定预测能力的模型。
通常,训练集占总数据集的70%到80%。
2. 验证集(Validation Set):用于验证模型性能的数据集。
在模型训练过程中,我们需要不断地调整模型的参数和结构,以优化模型的性能。
验证集就是用来评估不同参数和结构下的模型性能,帮助我们选择最好的模型。
通常,验证集占总数据集的10%到20%。
3. 测试集(Test Set):用于最终评估模型性能的数据集。
在模型训练和参数调整完成后,我们需要使用测试集来评估模型的最终性能。
测试集的评估结果可以为我们提供对模型泛化能力的参考,即模型对新数据的预测能力。
通常,测试集占总数据集的10%左右。
通过合理地划分训练集、验证集和测试集,我们可以更好地评估模型的性能,并选择出最优的模型进行实际应用。
同时,这种划分也有助于防止过拟合和欠拟合问题,提高模型的泛化能力。
dataset用法python

dataset用法python(实用版)目录1.介绍 Dataset2.Python 中使用 Dataset 的方法3.Dataset 的优点4.结论正文1.介绍 DatasetDataset 是一个用于存储和组织数据的 Python 对象。
它可以让你以一种结构化的方式来处理数据,类似于关系型数据库中的表结构。
Dataset由一系列的列和行组成,每一行表示一个记录,每一列表示一个字段。
使用 Dataset 可以让数据处理变得更加简单和直观。
2.Python 中使用 Dataset 的方法在 Python 中,可以使用 pandas 库来创建和操作 Dataset。
以下是一些常用的方法:- import pandas as pd- df = pd.DataFrame(data) # 创建一个 DataFrame- df.append(data, ignore_index=True) # 添加数据到 DataFrame - df.drop(columns=["column_name"]) # 删除指定的列- df.dropna() # 删除包含缺失值的行- df.groupby("column_name").mean() # 按照指定列进行分组并计算平均值3.Dataset 的优点Dataset 具有以下优点:- 结构化:Dataset 以表格的形式存储数据,使得数据结构更加清晰,易于理解和操作。
- 可扩展性:Dataset 可以轻松地扩展或修改,以适应不断变化的数据需求。
- 数据处理:使用 Dataset 可以方便地进行数据处理,如筛选、排序、计算统计等。
- 代码可读性:使用 Dataset 可以提高代码的可读性,使数据处理过程更加清晰。
4.结论Dataset 是 Python 中处理数据的一种有效方式。
通过使用 pandas 库,可以轻松地创建和操作 Dataset,从而简化数据处理过程。
机器学习经典分类算法——k-近邻算法(附python实现代码及数据集)
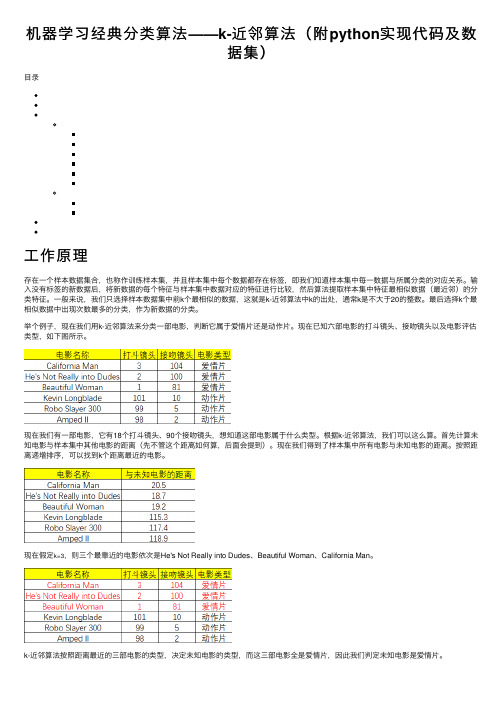
机器学习经典分类算法——k-近邻算法(附python实现代码及数据集)⽬录⼯作原理存在⼀个样本数据集合,也称作训练样本集,并且样本集中每个数据都存在标签,即我们知道样本集中每⼀数据与所属分类的对应关系。
输⼊没有标签的新数据后,将新数据的每个特征与样本集中数据对应的特征进⾏⽐较,然后算法提取样本集中特征最相似数据(最近邻)的分类特征。
⼀般来说,我们只选择样本数据集中前k个最相似的数据,这就是k-近邻算法中k的出处,通常k是不⼤于20的整数。
最后选择k个最相似数据中出现次数最多的分类,作为新数据的分类。
举个例⼦,现在我们⽤k-近邻算法来分类⼀部电影,判断它属于爱情⽚还是动作⽚。
现在已知六部电影的打⽃镜头、接吻镜头以及电影评估类型,如下图所⽰。
现在我们有⼀部电影,它有18个打⽃镜头、90个接吻镜头,想知道这部电影属于什么类型。
根据k-近邻算法,我们可以这么算。
⾸先计算未知电影与样本集中其他电影的距离(先不管这个距离如何算,后⾯会提到)。
现在我们得到了样本集中所有电影与未知电影的距离。
按照距离递增排序,可以找到k个距离最近的电影。
现在假定k=3,则三个最靠近的电影依次是He's Not Really into Dudes、Beautiful Woman、California Man。
python实现⾸先编写⼀个⽤于创建数据集和标签的函数,要注意的是该函数在实际⽤途上没有多⼤意义,仅⽤于测试代码。
def createDataSet():group = array([[1.0,1.1],[1.0,1.0],[0,0],[0,0.1]])labels = ['A','A','B','B']return group, labels然后是函数classify0(),该函数的功能是使⽤k-近邻算法将每组数据划分到某个类中,其伪代码如下:对未知类别属性的数据集中的每个点依次执⾏以下操作:(1)计算已知类别数据集中的点与当前点之间的距离;(2)按照距离递增次序排序;(3)选取与当前点距离最⼩的k个点;(4)确定前k个点所在类别的出现频率;(5)返回前k个点出现频率最⾼的类别作为当前点的预测分类。
dataset的用法
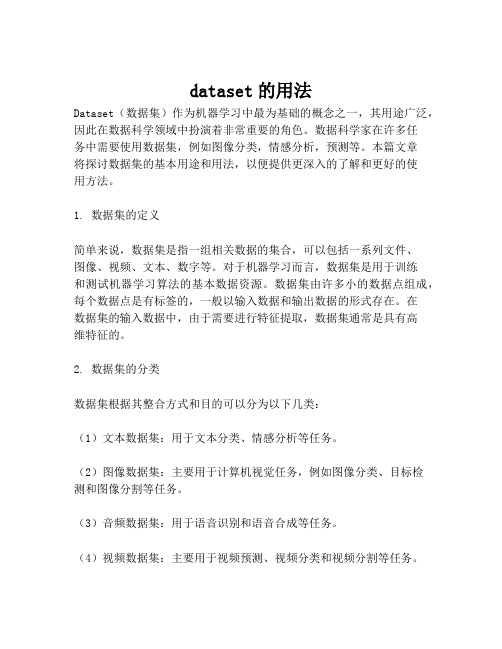
dataset的用法Dataset(数据集)作为机器学习中最为基础的概念之一,其用途广泛,因此在数据科学领域中扮演着非常重要的角色。
数据科学家在许多任务中需要使用数据集,例如图像分类,情感分析,预测等。
本篇文章将探讨数据集的基本用途和用法,以便提供更深入的了解和更好的使用方法。
1. 数据集的定义简单来说,数据集是指一组相关数据的集合,可以包括一系列文件、图像、视频、文本、数字等。
对于机器学习而言,数据集是用于训练和测试机器学习算法的基本数据资源。
数据集由许多小的数据点组成,每个数据点是有标签的,一般以输入数据和输出数据的形式存在。
在数据集的输入数据中,由于需要进行特征提取,数据集通常是具有高维特征的。
2. 数据集的分类数据集根据其整合方式和目的可以分为以下几类:(1)文本数据集:用于文本分类、情感分析等任务。
(2)图像数据集:主要用于计算机视觉任务,例如图像分类、目标检测和图像分割等任务。
(3)音频数据集:用于语音识别和语音合成等任务。
(4)视频数据集:主要用于视频预测、视频分类和视频分割等任务。
(5)时间序列数据集:由时间序列数据组成,主要用于预测和分析时间序列的趋势。
3. 数据集的用途数据集通常用于训练模型以识别数据中的特征。
在机器学习的训练中,需要使用许多数据点来训练机器学习模型。
这些数据点可能来自不同的数据集,并且通常需要进行特征提取以便进行有效的学习。
在机器学习领域,数据集用于监督式学习和非监督式学习。
在监督式学习中,数据集的输入和输出数值由人工标注注明,在非监督式学习中,数据集的输入和输出数值互相独立。
通过对数据集的分析,机器学习模型可以获得对数据点和数据集内的模式的更深入的了解。
4. 数据集的制作对于数据集的制作需要有一定的专业技能及工具支持。
制作过程通常包括以下几个步骤:(1)数据采集:数据采集是指获取数据的过程。
该过程的精度关系到后续的训练和测试效果。
数据的来源可能是从网上下载,也可以通过手动输入等方式进行数据收集。
[综]训练集(trainset)验证集(validationset)测试集(testset)
![[综]训练集(trainset)验证集(validationset)测试集(testset)](https://img.taocdn.com/s3/m/5f4b331cbb1aa8114431b90d6c85ec3a87c28bff.png)
[综]训练集(trainset)验证集(validationset)测试集(testset)在有监督(supervise)的机器学习中,数据集常被分成2~3个,即:训练集(train set) 验证集(validation set) 测试集(test set)。
⼀般需要将样本分成独⽴的三部分训练集(train set),验证集(validation set)和测试集(test set)。
其中训练集⽤来估计模型,验证集⽤来确定⽹络结构或者控制模型复杂程度的参数,⽽测试集则检验最终选择最优的模型的性能如何。
⼀个典型的划分是训练集占总样本的50%,⽽其它各占25%,三部分都是从样本中随机抽取。
样本少的时候,上⾯的划分就不合适了。
常⽤的是留少部分做测试集。
然后对其余N个样本采⽤K折交叉验证法。
就是将样本打乱,然后均匀分成K份,轮流选择其中K-1份训练,剩余的⼀份做验证,计算预测误差平⽅和,最后把K次的预测误差平⽅和再做平均作为选择最优模型结构的依据。
特别的K取N,就是留⼀法(leave one out)。
这三个名词在机器学习领域的⽂章中极其常见,但很多⼈对他们的概念并不是特别清楚,尤其是后两个经常被⼈混⽤。
Ripley,B.D(1996)在他的经典专著Pattern Recognition and Neural Networks中给出了这三个词的定义。
Training set: A set of examples used for learning, which is to fit the parameters [i.e., weights] of the classifier.Validation set: A set of examples used to tune the parameters [i.e., architecture, not weights] of a classifier, for example to choose the number of hidden units in a neural network.Test set: A set of examples used only to assess the performance [generalization] of a fully specified classifier.显然,training set是⽤来训练模型或确定模型参数的,如ANN中权值等; validation set是⽤来做模型选择(model selection),即做模型的最终优化及确定的,如ANN的结构;⽽ test set则纯粹是为了测试已经训练好的模型的推⼴能⼒。
load_dataset 用法

load_dataset 用法load_dataset 是一个用于加载数据集的函数,通常在数据科学和机器学习领域中使用。
以下是 load_dataset 的一般用法:1. 导入所需的库:```pythonimport datasets```2. 加载数据集:```pythondataset = _dataset(name="数据集名称")```在上面的代码中,你需要将 "数据集名称" 替换为你要加载的实际数据集名称。
load_dataset 函数将自动从默认的数据集存储库中下载并加载指定的数据集。
3. 对数据集进行处理:load_dataset 返回的数据集对象包含多个属性,你可以使用这些属性对数据进行进一步的处理和分析。
以下是一些常用的属性:`data`:包含数据集中的所有样本。
`target`:包含每个样本对应的标签。
`features`:包含数据集的特征名称。
`categories`:包含目标变量的类别名称。
`descriptions`:包含数据集的描述信息。
4. 使用数据集进行训练和测试:一旦你加载了数据集,就可以使用它来训练和测试机器学习模型。
以下是一个简单的示例,演示如何使用加载的数据集训练一个模型:```pythonfrom import RandomForestClassifier创建模型实例model = RandomForestClassifier()使用数据集训练模型(dataset["data"], dataset["target"])```在上面的示例中,我们使用了一个随机森林分类器作为模型实例,并使用数据集中的 "data" 和 "target" 属性来训练模型。
你可以根据自己的需求选择合适的模型和参数进行训练和测试。
机器学习_Trains Data Set(火车数据集)
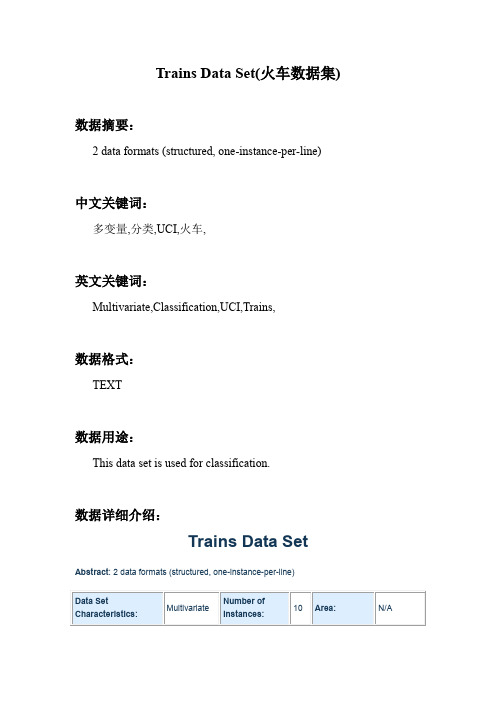
Trains Data Set(火车数据集)数据摘要:2 data formats (structured, one-instance-per-line)中文关键词:多变量,分类,UCI,火车,英文关键词:Multivariate,Classification,UCI,Trains,数据格式:TEXT数据用途:This data set is used for classification.数据详细介绍:Trains Data Set Abstract: 2 data formats (structured, one-instance-per-line)Source:Original owners:Ryszard S. Michalski (michalski '@' ) and Robert SteppDonor:GMU, Center for AI, Software Librarian, Eric E. Bloedorn (bloedorn '@' )Data Set Information:Notes:- Additional "background" knowledge is supplied that provides a partial ordering on some of the attribute values.- We are providing this dataset both in its original form and in a form similar to the more typical propositional datasets in our repository. Since the trains dataset records relations between attributes, this transformation was somewhat challenging. However, it may shed some insight on this problem for people who are more familiar with the simple one-instance-per-line dataset format.Hierarchy of values:if (cshape is one of {openrect,opentrap,ushaped,dblopnrect}then cshape is opentopif (cshape is one of {hexagon,ellipse,closedrect,jaggedtop,slopetop, engine}then cshape closedtopPrediction task: Determine concise decision rules distinguishing trains traveling east from those traveling west.Attribute Information:The following format was used for the "transformed" dataset representation as found in trains.transformed.data (one instance per line):1. Number_of_cars (integer in [3-5])2. Number_of_different_loads (integer in [1-4])3-22: 5 attributes for each of cars 2 through 5: (20 attributes total)- num_wheels (integer in [2-3])- length (short or long)- shape (closedrect, dblopnrect, ellipse, engine, hexagon, jaggedtop, openrect, opentrap, slopetop, ushaped)- num_loads (integer in [0-3])- load_shape (circlelod, hexagonlod, rectanglod, trianglod)23-32: 10 Boolean attributes describing whether 2 types of loads are on adjacent cars of the train- Rectangle_next_to_rectangle (0 if false, 1 if true)- Rectangle_next_to_triangle (0 if false, 1 if true)- Rectangle_next_to_hexagon (0 if false, 1 if true)- Rectangle_next_to_circle (0 if false, 1 if true)- Triangle_next_to_triangle (0 if false, 1 if true)- Triangle_next_to_hexagon (0 if false, 1 if true)- Triangle_next_to_circle (0 if false, 1 if true)- Hexagon_next_to_hexagon (0 if false, 1 if true)- Hexagon_next_to_circle (0 if false, 1 if true)- Circle_next_to_circle (0 if false, 1 if true)33. Class attribute (east or west)The number of cars vary between 3 and 5. Therefore, attributes referring to properties of cars that do not exist (such as the 5 attriubutes for the "5th" car when the train has fewer than 5 cars) are assigned a value of "-".Relevant Papers:R.S. Michalski and J.B. Larson "Inductive Inference of VL Decision Rules" In Proceedings of the Workshop in Pattern-Directed Inference Systems, Hawaii, May 1977.[Web Link]Stepp, R.E. and Michalski, R.S. "Conceptual Clustering: Inventing Goal-Oriented Classifications of Structured Objects" In R.S. Michalski, J.G. Carbonell, and T.M. Mitchell (Eds.) "Machine Learning: An Artificial Intelligence Approach, Volume II". Los Altos, Ca: Morgan Kaufmann.[Web Link]数据预览:点此下载完整数据集。
UCI数据库使用说明
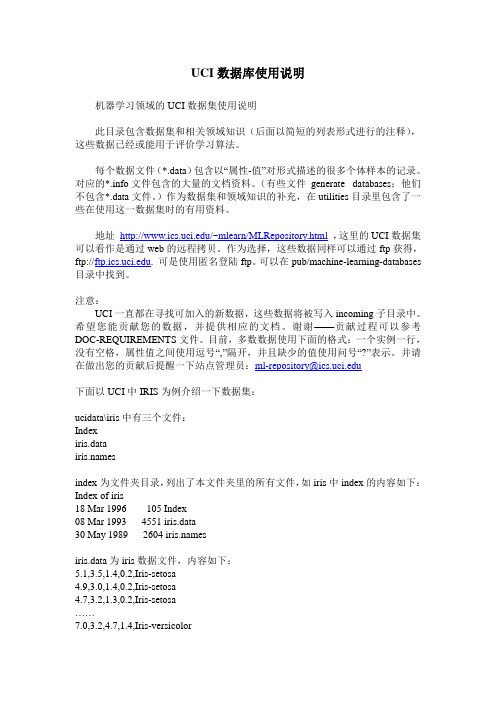
UCI数据库使用说明机器学习领域的UCI数据集使用说明此目录包含数据集和相关领域知识(后面以简短的列表形式进行的注释),这些数据已经或能用于评价学习算法。
每个数据文件(*.data)包含以“属性-值”对形式描述的很多个体样本的记录。
对应的*.info文件包含的大量的文档资料。
(有些文件_generate_ databases;他们不包含*.data文件。
)作为数据集和领域知识的补充,在utilities目录里包含了一些在使用这一数据集时的有用资料。
地址/~mlearn/MLRepository.html,这里的UCI数据集可以看作是通过web的远程拷贝。
作为选择,这些数据同样可以通过ftp获得,ftp://. 可是使用匿名登陆ftp。
可以在pub/machine-learning-databases 目录中找到。
注意:UCI一直都在寻找可加入的新数据,这些数据将被写入incoming子目录中。
希望您能贡献您的数据,并提供相应的文档。
谢谢——贡献过程可以参考DOC-REQUIREMENTS文件。
目前,多数数据使用下面的格式:一个实例一行,没有空格,属性值之间使用逗号“,”隔开,并且缺少的值使用问号“?”表示。
并请在做出您的贡献后提醒一下站点管理员:ml-repository@下面以UCI中IRIS为例介绍一下数据集:ucidata\iris中有三个文件:Indexiris.datasindex为文件夹目录,列出了本文件夹里的所有文件,如iris中index的内容如下:Index of iris18 Mar 1996 105 Index08 Mar 1993 4551 iris.data30 May 1989 2604 siris.data为iris数据文件,内容如下:5.1,3.5,1.4,0.2,Iris-setosa4.9,3.0,1.4,0.2,Iris-setosa4.7,3.2,1.3,0.2,Iris-setosa……7.0,3.2,4.7,1.4,Iris-versicolor6.4,3.2,4.5,1.5,Iris-versicolor6.9,3.1,4.9,1.5,Iris-versicolor……6.3,3.3,6.0,2.5,Iris-virginica5.8,2.7,5.1,1.9,Iris-virginica7.1,3.0,5.9,2.1,Iris-virginica……如上,属性直接以逗号隔开,中间没有空格(5.1,3.5,1.4,0.2,),最后一列为本行属性对应的值,即决策属性Iris-setosa。
- 1、下载文档前请自行甄别文档内容的完整性,平台不提供额外的编辑、内容补充、找答案等附加服务。
- 2、"仅部分预览"的文档,不可在线预览部分如存在完整性等问题,可反馈申请退款(可完整预览的文档不适用该条件!)。
- 3、如文档侵犯您的权益,请联系客服反馈,我们会尽快为您处理(人工客服工作时间:9:00-18:30)。
Trains Data Set(火车数据集)
数据摘要:
2 data formats (structured, one-instance-per-line)
中文关键词:
多变量,分类,UCI,火车,
英文关键词:
Multivariate,Classification,UCI,Trains,
数据格式:
TEXT
数据用途:
This data set is used for classification.
数据详细介绍:
Trains Data Set Abstract: 2 data formats (structured, one-instance-per-line)
Source:
Original owners:
Ryszard S. Michalski (michalski '@' ) and Robert Stepp
Donor:
GMU, Center for AI, Software Librarian, Eric E. Bloedorn (bloedorn '@' )
Data Set Information:
Notes:
- Additional "background" knowledge is supplied that provides a partial ordering on some of the attribute values.
- We are providing this dataset both in its original form and in a form similar to the more typical propositional datasets in our repository. Since the trains dataset records relations between attributes, this transformation was somewhat challenging. However, it may shed some insight on this problem for people who are more familiar with the simple one-instance-per-line dataset format.
Hierarchy of values:
if (cshape is one of {openrect,opentrap,ushaped,dblopnrect}
then cshape is opentop
if (cshape is one of {hexagon,ellipse,closedrect,jaggedtop,slopetop, engine}
then cshape closedtop
Prediction task: Determine concise decision rules distinguishing trains traveling east from those traveling west.
Attribute Information:
The following format was used for the "transformed" dataset representation as found in trains.transformed.data (one instance per line):
1. Number_of_cars (integer in [3-5])
2. Number_of_different_loads (integer in [1-4])
3-22: 5 attributes for each of cars 2 through 5: (20 attributes total)
- num_wheels (integer in [2-3])
- length (short or long)
- shape (closedrect, dblopnrect, ellipse, engine, hexagon, jaggedtop, openrect, opentrap, slopetop, ushaped)
- num_loads (integer in [0-3])
- load_shape (circlelod, hexagonlod, rectanglod, trianglod)
23-32: 10 Boolean attributes describing whether 2 types of loads are on adjacent cars of the train
- Rectangle_next_to_rectangle (0 if false, 1 if true)
- Rectangle_next_to_triangle (0 if false, 1 if true)
- Rectangle_next_to_hexagon (0 if false, 1 if true)
- Rectangle_next_to_circle (0 if false, 1 if true)
- Triangle_next_to_triangle (0 if false, 1 if true)
- Triangle_next_to_hexagon (0 if false, 1 if true)
- Triangle_next_to_circle (0 if false, 1 if true)
- Hexagon_next_to_hexagon (0 if false, 1 if true)
- Hexagon_next_to_circle (0 if false, 1 if true)
- Circle_next_to_circle (0 if false, 1 if true)
33. Class attribute (east or west)
The number of cars vary between 3 and 5. Therefore, attributes referring to properties of cars that do not exist (such as the 5 attriubutes for the "5th" car when the train has fewer than 5 cars) are assigned a value of "-".
Relevant Papers:
R.S. Michalski and J.B. Larson "Inductive Inference of VL Decision Rules" In Proceedings of the Workshop in Pattern-Directed Inference Systems, Hawaii, May 1977.
[Web Link]
Stepp, R.E. and Michalski, R.S. "Conceptual Clustering: Inventing Goal-Oriented Classifications of Structured Objects" In R.S. Michalski, J.G. Carbonell, and T.M. Mitchell (Eds.) "Machine Learning: An Artificial Intelligence Approach, Volume II". Los Altos, Ca: Morgan Kaufmann.
[Web Link]
数据预览:
点此下载完整数据集。