77个数据分析或者数据科学家面试常见问题(英)
数据分析面试题目及答案
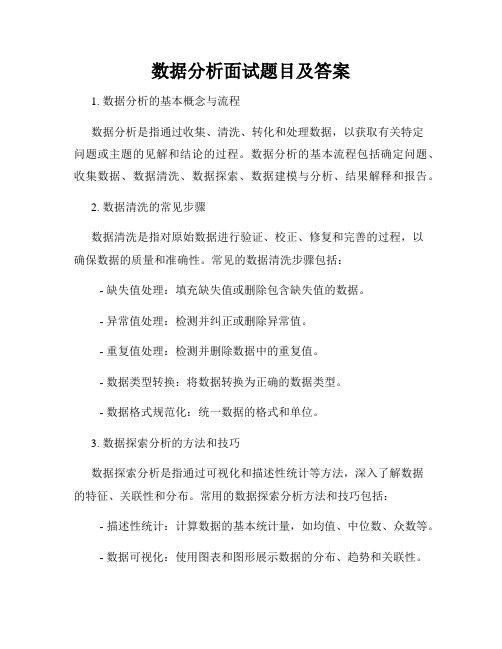
数据分析面试题目及答案1. 数据分析的基本概念与流程数据分析是指通过收集、清洗、转化和处理数据,以获取有关特定问题或主题的见解和结论的过程。
数据分析的基本流程包括确定问题、收集数据、数据清洗、数据探索、数据建模与分析、结果解释和报告。
2. 数据清洗的常见步骤数据清洗是指对原始数据进行验证、校正、修复和完善的过程,以确保数据的质量和准确性。
常见的数据清洗步骤包括:- 缺失值处理:填充缺失值或删除包含缺失值的数据。
- 异常值处理:检测并纠正或删除异常值。
- 重复值处理:检测并删除数据中的重复值。
- 数据类型转换:将数据转换为正确的数据类型。
- 数据格式规范化:统一数据的格式和单位。
3. 数据探索分析的方法和技巧数据探索分析是指通过可视化和描述性统计等方法,深入了解数据的特征、关联性和分布。
常用的数据探索分析方法和技巧包括: - 描述性统计:计算数据的基本统计量,如均值、中位数、众数等。
- 数据可视化:使用图表和图形展示数据的分布、趋势和关联性。
- 相关性分析:计算和探索数据之间的相关性,如Pearson相关系数、Spearman秩相关系数等。
- 群组分析:基于数据的特征将数据进行分类和分组。
- 时间序列分析:探索数据随时间的变化趋势和周期性。
4. 常用的数据分析工具和编程语言在数据分析中,常用的工具和编程语言有:- Microsoft Excel:适用于简单的数据分析和可视化。
- SQL:用于处理和查询结构化数据。
- Python:强大的编程语言,提供丰富的数据分析和可视化库,如NumPy、Pandas和Matplotlib。
- R语言:专门用于统计分析和数据可视化的编程语言和环境。
- Tableau:交互式数据可视化工具,可创建富有洞察力的仪表板和报告。
5. 面试常见的数据分析题目和答案示例(以下仅为示例,实际面试题目因公司和职位而异,需灵活掌握) - 请说明你对A/B测试的理解以及在数据分析中的应用。
数据分析师面试常见问题
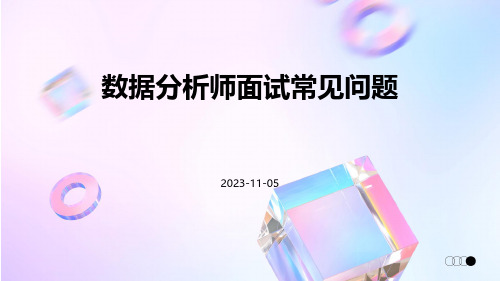
2023-11-05CATALOGUE目录•基础数学知识•数据分析工具•数据分析方法与模型•数据库与数据清洗•案例分析能力•软实力与面试技巧01基础数学知识总结词重要地位、广泛应用、数据分析核心工具详细描述统计学是数据分析的核心工具之一,广泛应用于各行各业。
在数据分析师面试中,统计学知识是必备的考察内容之一。
统计学总结词研究随机事件、概率质量函数、数学期望值详细描述概率论是研究随机事件及其概率的数学分支。
在数据分析中,概率论用于研究数据分布、预测模型、优化决策等问题。
概率论线性代数总结词矩阵运算、向量空间、特征值与特征向量详细描述线性代数是研究线性方程组、矩阵运算、向量空间等概念的数学分支。
在数据分析中,线性代数用于数据降维、矩阵分解、推荐算法等领域。
02数据分析工具了解数据库安全熟悉权限管理、数据备份等概念,能够保障数据库的安全性和稳定性。
SQL掌握基础SQL语句包括SELECT、FROM、WHERE、GROUP BY、ORDER BY等语句,能够根据需求编写简单的SQL查询语句。
了解SQL优化熟悉索引、视图、存储过程等概念,能够优化复杂的SQL 查询语句以提高性能。
掌握数据库操作熟悉数据库的创建、删除、修改等操作,能够进行数据库的管理和维护。
Python基础熟悉常用Python库如NumPy、Pandas、Matplotlib等,能够使用这些库进行数据处理和分析。
了解Python进阶知识如面向对象编程、装饰器、生成器等概念,能够提高Python编程的效率和灵活性。
掌握Python基础语法包括变量、数据类型、控制流语句、函数等概念,能够编写简单的Python程序。
1Excel基础23包括单元格格式、公式、函数、图表等概念,能够使用Excel进行基础的数据处理和分析。
掌握Excel基本操作如数据透视表、条件格式、宏等概念,能够使用这些功能提高Excel数据处理和分析的效率。
熟悉Excel高级功能熟悉VBA语法和常用函数,能够编写简单的VBA宏程序,提高Excel自动化程度。
数据分析面试常见问题
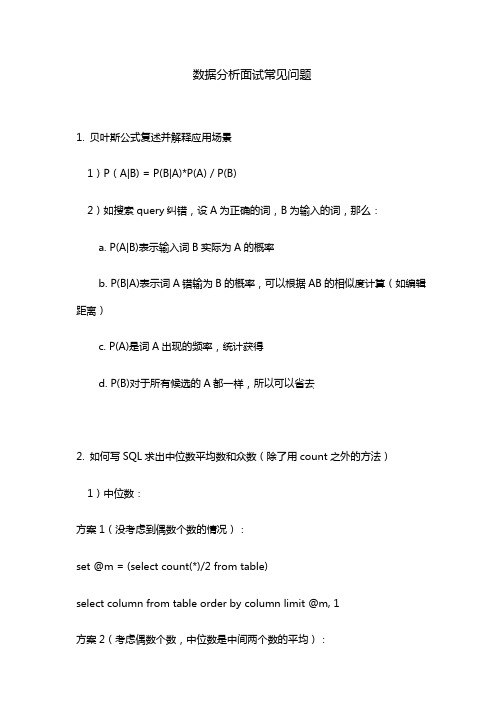
数据分析面试常见问题1. 贝叶斯公式复述并解释应用场景1)P(A|B) = P(B|A)*P(A) / P(B)2)如搜索query纠错,设A为正确的词,B为输入的词,那么:a. P(A|B)表示输入词B实际为A的概率b. P(B|A)表示词A错输为B的概率,可以根据AB的相似度计算(如编辑距离)c. P(A)是词A出现的频率,统计获得d. P(B)对于所有候选的A都一样,所以可以省去2. 如何写SQL求出中位数平均数和众数(除了用count之外的方法)1)中位数:方案1(没考虑到偶数个数的情况):set @m = (select count(*)/2 from table)select column from table order by column limit @m, 1方案2(考虑偶数个数,中位数是中间两个数的平均):set @index = -1select avg(table.column)from(select @index:=@index+1 as index, columnfrom table order by column) as twhere t.index in (floor(@index/2),ceiling(@index/2))2)平均数:select avg(distinct column) from table3)众数:select column, count(*) from table group by column order by column desc limit 1(emmm,好像用到count了)3. 学过的机器学习算法有哪些略。
4. 如何避免决策树过拟合1)限制树深2)剪枝3)限制叶节点数量4)正则化项5)增加数据6)bagging(subsample、subfeature、低维空间投影)7)数据增强(加入有杂质的数据)8)早停5. 朴素贝叶斯的理解1)推导(参考:https:///pinard/p/6069267.html)2)理解:朴素贝叶斯是在已知一些先验概率的情况下,由果索因的一种方法3)其它:朴素的意思是假设了事件相互独立6. SVM的优点1)优点:a. 能应用于非线性可分的情况b. 最后分类时由支持向量决定,复杂度取决于支持向量的数目而不是样本空间的维度,避免了维度灾难c. 具有鲁棒性:因为只使用少量支持向量,抓住关键样本,剔除冗余样本d. 高维低样本下性能好,如文本分类2)缺点:a. 模型训练复杂度高b. 难以适应多分类问题c. 核函数选择没有较好的方法论7. Kmeans的原理1)初始化k个点2)根据距离点归入k个类中3)更新k个类的类中心4)重复(2)(3),直到收敛或达到迭代次数8. 对拼多多有什么了解,为什么选择拼多多略。
数据分析面试题目
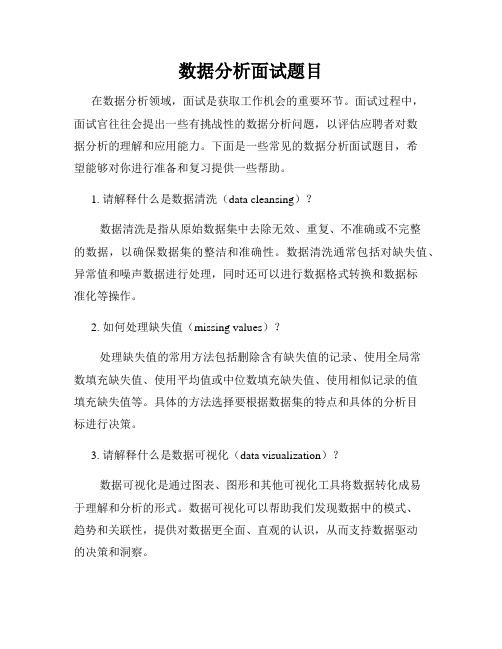
数据分析面试题目在数据分析领域,面试是获取工作机会的重要环节。
面试过程中,面试官往往会提出一些有挑战性的数据分析问题,以评估应聘者对数据分析的理解和应用能力。
下面是一些常见的数据分析面试题目,希望能够对你进行准备和复习提供一些帮助。
1. 请解释什么是数据清洗(data cleansing)?数据清洗是指从原始数据集中去除无效、重复、不准确或不完整的数据,以确保数据集的整洁和准确性。
数据清洗通常包括对缺失值、异常值和噪声数据进行处理,同时还可以进行数据格式转换和数据标准化等操作。
2. 如何处理缺失值(missing values)?处理缺失值的常用方法包括删除含有缺失值的记录、使用全局常数填充缺失值、使用平均值或中位数填充缺失值、使用相似记录的值填充缺失值等。
具体的方法选择要根据数据集的特点和具体的分析目标进行决策。
3. 请解释什么是数据可视化(data visualization)?数据可视化是通过图表、图形和其他可视化工具将数据转化成易于理解和分析的形式。
数据可视化可以帮助我们发现数据中的模式、趋势和关联性,提供对数据更全面、直观的认识,从而支持数据驱动的决策和洞察。
4. 你如何选择合适的可视化图表?选择合适的可视化图表需要考虑数据类型、分析目标和受众等因素。
例如,对于数值型数据的比较,可以选择柱状图或折线图;对于离散型数据的分布,可以选择饼图或条形图;对于时间序列数据的趋势,可以选择折线图或面积图等。
选择合适的可视化图表可以更好地展现数据的特征和模式。
5. 请说明你在数据分析项目中如何进行特征选择(feature selection)?特征选择是从原始数据中选择对分析目标有重要影响的特征。
常见的特征选择方法包括过滤法、包装法和嵌入法。
过滤法通过统计指标和相关性等方法对特征进行排序和筛选;包装法通过训练模型进行特征选择,并进行交叉验证来评估特征的重要性;嵌入法则是在模型训练过程中自动选择特征。
数据岗位招聘面试题与参考回答
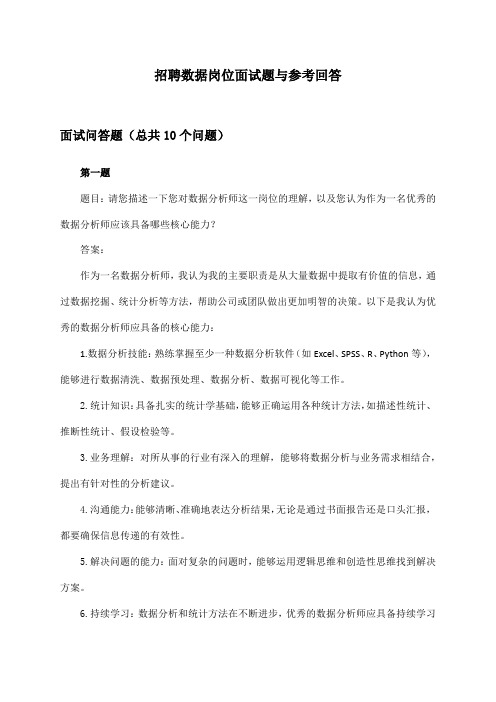
招聘数据岗位面试题与参考回答面试问答题(总共10个问题)第一题题目:请您描述一下您对数据分析师这一岗位的理解,以及您认为作为一名优秀的数据分析师应该具备哪些核心能力?答案:作为一名数据分析师,我认为我的主要职责是从大量数据中提取有价值的信息,通过数据挖掘、统计分析等方法,帮助公司或团队做出更加明智的决策。
以下是我认为优秀的数据分析师应具备的核心能力:1.数据分析技能:熟练掌握至少一种数据分析软件(如Excel、SPSS、R、Python等),能够进行数据清洗、数据预处理、数据分析、数据可视化等工作。
2.统计知识:具备扎实的统计学基础,能够正确运用各种统计方法,如描述性统计、推断性统计、假设检验等。
3.业务理解:对所从事的行业有深入的理解,能够将数据分析与业务需求相结合,提出有针对性的分析建议。
4.沟通能力:能够清晰、准确地表达分析结果,无论是通过书面报告还是口头汇报,都要确保信息传递的有效性。
5.解决问题的能力:面对复杂的问题时,能够运用逻辑思维和创造性思维找到解决方案。
6.持续学习:数据分析和统计方法在不断进步,优秀的数据分析师应具备持续学习的态度,不断更新自己的知识库。
解析:这一题旨在考察应聘者对数据分析师岗位的理解程度,以及对所需能力的自我评估。
优秀的数据分析师不仅需要具备扎实的技术能力,还需要具备良好的业务敏感度和沟通技巧。
答案中提到的各项能力都是数据分析师岗位的关键要求,通过这样的回答,面试官可以初步判断应聘者的专业背景和综合素质。
第二题题目:请描述一下您在过去的工作或项目中,如何处理过一次数据清洗的难题?您遇到了哪些挑战,又是如何克服这些挑战的?答案:在过去的一个项目中,我负责对一家大型电商平台的用户数据进行清洗和分析。
在数据清洗过程中,我遇到了以下挑战:1.数据质量问题:原始数据中存在大量的缺失值、异常值和重复数据。
2.数据格式不一致:不同来源的数据格式不统一,给数据整合带来了困难。
数据分析面试常见问题
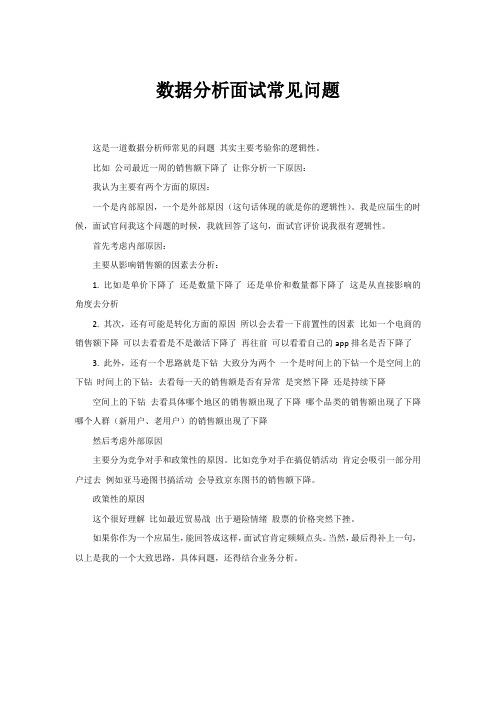
数据分析面试常见问题
这是一道数据分析师常见的问题其实主要考验你的逻辑性。
比如公司最近一周的销售额下降了让你分析一下原因:
我认为主要有两个方面的原因:
一个是内部原因,一个是外部原因(这句话体现的就是你的逻辑性)。
我是应届生的时候,面试官问我这个问题的时候,我就回答了这句,面试官评价说我很有逻辑性。
首先考虑内部原因:
主要从影响销售额的因素去分析:
1. 比如是单价下降了还是数量下降了还是单价和数量都下降了这是从直接影响的角度去分析
2. 其次,还有可能是转化方面的原因所以会去看一下前置性的因素比如一个电商的销售额下降可以去看看是不是激活下降了再往前可以看看自己的app排名是否下降了
3. 此外,还有一个思路就是下钻大致分为两个一个是时间上的下钻一个是空间上的下钻时间上的下钻:去看每一天的销售额是否有异常是突然下降还是持续下降空间上的下钻去看具体哪个地区的销售额出现了下降哪个品类的销售额出现了下降哪个人群(新用户、老用户)的销售额出现了下降
然后考虑外部原因
主要分为竞争对手和政策性的原因。
比如竞争对手在搞促销活动肯定会吸引一部分用户过去例如亚马逊图书搞活动会导致京东图书的销售额下降。
政策性的原因
这个很好理解比如最近贸易战出于避险情绪股票的价格突然下挫。
如果你作为一个应届生,能回答成这样,面试官肯定频频点头。
当然,最后得补上一句,以上是我的一个大致思路,具体问题,还得结合业务分析。
数据科学家常见面试题
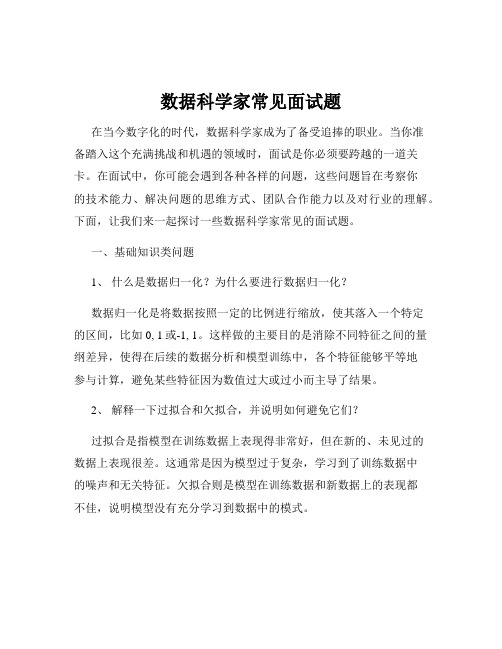
数据科学家常见面试题在当今数字化的时代,数据科学家成为了备受追捧的职业。
当你准备踏入这个充满挑战和机遇的领域时,面试是你必须要跨越的一道关卡。
在面试中,你可能会遇到各种各样的问题,这些问题旨在考察你的技术能力、解决问题的思维方式、团队合作能力以及对行业的理解。
下面,让我们来一起探讨一些数据科学家常见的面试题。
一、基础知识类问题1、什么是数据归一化?为什么要进行数据归一化?数据归一化是将数据按照一定的比例进行缩放,使其落入一个特定的区间,比如0, 1或-1, 1。
这样做的主要目的是消除不同特征之间的量纲差异,使得在后续的数据分析和模型训练中,各个特征能够平等地参与计算,避免某些特征因为数值过大或过小而主导了结果。
2、解释一下过拟合和欠拟合,并说明如何避免它们?过拟合是指模型在训练数据上表现得非常好,但在新的、未见过的数据上表现很差。
这通常是因为模型过于复杂,学习到了训练数据中的噪声和无关特征。
欠拟合则是模型在训练数据和新数据上的表现都不佳,说明模型没有充分学习到数据中的模式。
为了避免过拟合,可以采用正则化技术(如 L1 和 L2 正则化)、增加训练数据量、早停法(Early Stopping)等。
而避免欠拟合则可以尝试增加模型的复杂度,比如使用更多的特征、更深的神经网络等。
3、简单介绍一下常见的机器学习算法,如决策树、随机森林、支持向量机等,并比较它们的优缺点。
决策树是一种基于树结构的算法,易于理解和解释,但容易出现过拟合。
随机森林是由多个决策树组成的集成学习算法,具有较好的泛化能力和抗噪能力。
支持向量机在处理小样本、非线性问题上有优势,但计算复杂度较高。
二、编程和工具类问题1、你熟悉哪些编程语言用于数据科学?比如 Python、R 等,并谈谈你在项目中如何使用它们。
Python 在数据科学领域应用广泛,具有丰富的库,如 NumPy、Pandas、Scikitlearn 等。
在项目中,使用 Python 进行数据清洗、特征工程、模型训练和评估。
数据分析师常见的面试问题

数据分析师常见的面试问题(2)(总4页)--本页仅作为文档封面,使用时请直接删除即可----内页可以根据需求调整合适字体及大小--数据分析师常见的面试问题(2)这是一篇由网络搜集整理的关于数据分析师常见的面试问题集锦的文档,希望对你能有帮助。
数据分析师常见的面试问题集锦36、给出一个不符合高斯分布与不符合对数正态分布的数据案例。
给出一个分布非常混乱的数案例。
37、为什么说均方误差不是一个衡量模型的好指标你建议用哪个指标替代38、你如何证明你带来的算法改进是真的有效的与不做任何改变相比你对A/B测试熟吗39、什么是敏感性分析拥有更低的敏感性(也就是说更好的强壮性)和低的预测能力还是正好相反好你如何使用交叉验证你对于在数据集中插入噪声数据从而来检验模型的敏感性的想法如何看40、对于一下逻辑回归、决策树、神经网络。
在过去15年中这些技术做了哪些大的改进?41、除了主成分分析外你还使用其它数据降维技术吗你怎么想逐步回归你熟悉的逐步回归技术有哪些什么时候完整的数据要比降维的数据或者样本好42、你如何建议一个非参数置信区间?43、你熟悉极值理论、蒙特卡罗逻辑或者其它数理统计方法以正确的评估一个稀疏事件的发生概率?44、什么是归因分析如何识别归因与相关系数举例。
45、如何定义与衡量一个指标的预测能力?46、如何为欺诈检验得分技术发现最好的规则集你如何处理规则冗余、规则发现和二者的本质问题一个规则集的近似解决方案是否可行如何寻找一个可行的近似方案你如何决定这个解决方案足够好从而可以停止寻找另一个更好的47、如何创建一个关键字分类?48、什么是僵尸网络如何进行检测49、你有使用过API接口的经验吗什么样的API是谷歌还是亚马逊还是软件即时服务50、什么时候自己编号代码比使用数据科学者开发好的软件包更好?51、可视化使用什么工具在作图方面,你如何评价TableauRSAS在一个图中有效展现五个维度52、什么是概念验证?53、你主要与什么样的客户共事:内部、外部、销售部门/财务部门/市场部门/IT部门的人有咨询经验吗与供应商打过交道,包括供应商选择与测试。
- 1、下载文档前请自行甄别文档内容的完整性,平台不提供额外的编辑、内容补充、找答案等附加服务。
- 2、"仅部分预览"的文档,不可在线预览部分如存在完整性等问题,可反馈申请退款(可完整预览的文档不适用该条件!)。
- 3、如文档侵犯您的权益,请联系客服反馈,我们会尽快为您处理(人工客服工作时间:9:00-18:30)。
We are now at 80 questions. These are mostly open-ended questions, to assess the technical horizontal knowledge of a senior candidate for a rather high level position, e.g. director.1. What is the biggest data set that you processed, and how did you process it, what were the results?2. Tell me two success stories about your analytic or computer science projects?3. How was lift (or success) measured?4. What is: lift, KPI, robustness, model fitting, design of experiments, 80/20 rule?5. What is: collaborative filtering, n-grams, map reduce, cosine distance?6. How to optimize a web crawler to run much faster, extract better information, and better summarize data to produce cleaner databases?7. How would you come up with a solution to identify plagiarism?8. How to detect individual paid accounts shared by multiple users?9. Should click data be handled in real time? Why? In which contexts?10. What is better: good data or good models? And how do you define "good"? Is there a universal good model? Are there any models that are definitely not so good?11. What is probabilistic merging (AKA fuzzy merging)? Is it easier to handle with12. SQL or other languages? Which languages would you choose for semi-structured text data reconciliation?13. How do you handle missing data? What imputation techniques do you recommend?14. What is your favorite programming language / vendor? why?15. Tell me 3 things positive and 3 things negative about your favorite statistical software.16. Compare SAS, R, Python, Perl17. What is the curse of big data?18. Have you been involved in database design and data modeling?19. Have you been involved in dashboard creation and metric selection? What do you think about Birt?20. What features of Teradata do you like?21. You are about to send one million email (marketing campaign). How do you optimze delivery?22. How do you optimize response? Can you optimize both separately? (answer: not really)23. Toad or Brio or any other similar clients are quite inefficient to query Oracle databases. Why?24. How would you do to increase speed by a factor 10, and be able to handle far bigger outputs?How would you turn unstructured data into structured data? Is it really necessary? Is it OK to store data as flat text files rather than in an SQL-powered RDBMS?What are hash table collisions? How is it avoided? How frequently does it happen?How to make sure a mapreduce application has good load balance? What is load balance?Examples where mapreduce does not work? Examples where it works very well? What are the security issues involved with the cloud? What do you think ofEMC's solution offering an hybrid approach - both internal and external cloud - to mitigate the risks and offer other advantages (which ones)?Is it better to have 100 small hash tables or one big hash table, in memory, in terms of access speed (assuming both fit within RAM)? What do you think about in-database analytics?Why is naive Bayes so bad? How would you improve a spam detection algorithm that uses naive Bayes?Have you been working with white lists? Positive rules? (In the context of fraud or spam detection)What is star schema? Lookup tables?Can you perform logistic regression with Excel? (yes) How? (use linest on log-transformed data)? Would the result be good? (Excel has numerical issues, but it's very interactive)Have you optimized code or algorithms for speed: in SQL, Perl, C++, Python etc. How, and by how much?Is it better to spend 5 days developing a 90% accurate solution, or 10 days for 100% accuracy? Depends on the context?Define: quality assurance, six sigma, design of experiments. Give examples of good and bad designs of experiments.What are the drawbacks of general linear model? Are you familiar with alternatives (Lasso, ridge regression, boosted trees)?Do you think 50 small decision trees are better than a large one? Why?Is actuarial science not a branch of statistics (survival analysis)? If not, how so? Give examples of data that does not have a Gaussian distribution, nor log-normal. Give examples of data that has a very chaotic distribution?Why is mean square error a bad measure of model performance? What would you suggest instead?How can you prove that one improvement you've brought to an algorithm is really an improvement over not doing anything? Are you familiar with A/B testing? What is sensitivity analysis? Is it better to have low sensitivity (that is, great robustness) and low predictive power, or the other way around? How to perform good cross-validation? What do you think about the idea of injecting noise in your data set to test the sensitivity of your models?Compare logistic regression w. decision trees, neural networks. How have these technologies been vastly improved over the last 15 years?Do you know / used data reduction techniques other than PCA? What do you think of step-wise regression? What kind of step-wise techniques are you familiar with? When is full data better than reduced data or sample?How would you build non parametric confidence intervals, e.g. for scores? (see the AnalyticBridge theorem)Are you familiar either with extreme value theory, monte carlo simulations or mathematical statistics (or anything else) to correctly estimate the chance of a very rare event?What is root cause analysis? How to identify a cause vs. a correlation? Give examples.How would you define and measure the predictive power of a metric?How to detect the best rule set for a fraud detection scoring technology? How do you deal with rule redundancy, rule discovery, and the combinatorial nature of the problem (for finding optimum rule set - the one with best predictive power)? Can an approximate solution to the rule set problem be OK? How would you findan OK approximate solution? How would you decide it is good enough and stop looking for a better one?How to create a keyword taxonomy?What is a Botnet? How can it be detected?Any experience with using API's? Programming API's? Google or Amazon API's? AaaS (Analytics as a service)?When is it better to write your own code than using a data science software package?Which tools do you use for visualization? What do you think of Tableau? R? SAS? (for graphs). How to efficiently represent 5 dimension in a chart (or in a video)?What is POC (proof of concept)?What types of clients have you been working with: internal, external, sales / finance / marketing / IT people? Consulting experience? Dealing with vendors, including vendor selection and testing?Are you familiar with software life cycle? With IT project life cycle - from gathering requests to maintenance?What is a cron job?Are you a lone coder? A production guy (developer)? Or a designer (architect)? Is it better to have too many false positives, or too many false negatives?Are you familiar with pricing optimization, price elasticity, inventory management, competitive intelligence? Give examples.How does Zillow's algorithm work? (to estimate the value of any home in US) How to detect bogus reviews, or bogus Facebook accounts used for bad purposes?How would you create a new anonymous digital currency?Have you ever thought about creating a startup? Around which idea / concept? Do you think that typed login / password will disappear? How could they be replaced?Have you used time series models? Cross-correlations with time lags? Correlograms? Spectral analysis? Signal processing and filtering techniques? In which context?Which data scientists do you admire most? which startups?How did you become interested in data science?What is an efficiency curve? What are its drawbacks, and how can they be overcome?What is a recommendation engine? How does it work?What is an exact test? How and when can simulations help us when we do not use an exact test?What do you think makes a good data scientist?Do you think data science is an art or a science?What is the computational complexity of a good, fast clustering algorithm? What is a good clustering algorithm? How do you determine the number of clusters? How would you perform clustering on one million unique keywords, assuming you have 10 million data points - each one consisting of two keywords, and a metric measuring how similar these two keywords are? How would you create this 10 million data points table in the first place?Give a few examples of "best practices" in data science.What could make a chart misleading, difficult to read or interpret? What features should a useful chart have?Do you know a few "rules of thumb" used in statistical or computer science? Or in business analytics?What are your top 5 predictions for the next 20 years?How do you immediately know when statistics published in an article (e.g. newspaper) are either wrong or presented to support the author's point of view, rather than correct, comprehensive factual information on a specific subject? For instance, what do you think about the official monthly unemployment statistics regularly discussed in the press? What could make them more accurate?Testing your analytic intuition: look at these three charts. Two of them exhibit patterns. Which ones? Do you know that these charts are called scatter-plots? Are there other ways to visually represent this type of data?You design a robust non-parametric statistic (metric) to replace correlation or R square, that (1) is independent of sample size, (2) always between -1 and +1, and (3) based on rank statistics. How do you normalize for sample size? Write an algorithm that computes all permutations of n elements. How do you sample permutations (that is, generate tons of random permutations) when n is large, to estimate the asymptotic distribution for your newly created metric? You may use this asymptotic distribution for normalizing your metric. Do you think that an exact theoretical distribution might exist, and therefore, we should find it, and use it rather than wasting our time trying to estimate the asymptotic distribution using simulations?More difficult, technical question related to previous one. There is an obvious one-to-one correspondence between permutations of n elements and integers between 1 and n! Design an algorithm that encodes an integer less than n! as a permutation of n elements. What would be the reverse algorithm, used to decode a permutation and transform it back into a number? Hint: An intermediate step is to use the factorial number system representation of an integer. Feel free to check this reference online to answer the question. Even better, feel free to browse the web to find the full answer to the question (this will test the candidate's ability to quickly search online and find a solution to a problem without spending hours reinventing the wheel).。