models for time series and forecasting中小学PPT教学课件
建筑施工计算手册(第二版)
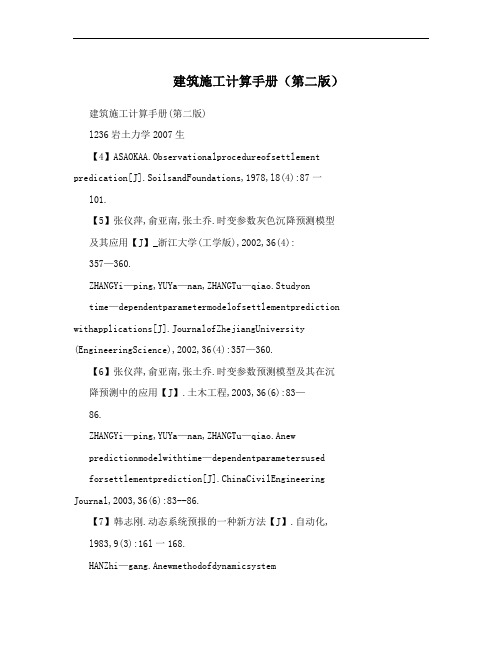
建筑施工计算手册(第二版)建筑施工计算手册(第二版)l236岩土力学2007生【4】ASAOKAA.Observationalprocedureofsettlement predication[J].SoilsandFoundations,1978,l8(4):87一l01.【5】张仪萍,俞亚南,张土乔.时变参数灰色沉降预测模型及其应用【J】_浙江大学(工学版),2002,36(4):357—360.ZHANGYi—ping,YUYa—nan,ZHANGTu—qiao.Studyontime—dependentparametermodelofsettlementprediction withapplications[J].JournalofZhejiangUniversity (EngineeringScience),2002,36(4):357—360.【6】张仪萍,俞亚南,张土乔.时变参数预测模型及其在沉降预测中的应用【J】.土木工程,2003,36(6):83—86.ZHANGYi—ping,YUYa—nan,ZHANGTu—qiao.Anewpredictionmodelwithtime—dependentparametersused forsettlementprediction[J].ChinaCivilEngineering Journal,2003,36(6):83--86.【7】韩志刚.动态系统预报的一种新方法【J】.自动化, l983,9(3):16l一168.HANZhi—gang.Anewmethodofdynamicsystemprediction[J].ACTAAutomaticaSinica,1983,9(3): l6l—l68.【8】刘国林.顾及多因素影响的双线性变形模型及其动态参数估计【J】_测绘,1995,24(3):l83—191.LIUGuo—lin.Bilineardeformationmodelsinconsiderationofvariousfactorsanddynamicparametersestimation[J].ACTAGeodaeticaetCartographica Sinica,1995,24(3):183—191.【9】余天堂,王振波.基于多层递阶方法的边坡位移预测【J】岩上力学,2003,24(3):442--444.YUTian—tang,WANGZhen—bo.Predictionofslopedisplacementswithmulti—layerrecursivemethod[J].Rock andSoilMechanirs,2003,24(3):442,444.【l0】张仪萍,王士金,张土乔.沉降预测的多层递阶时问序列模型研究【J】.浙江大学(工学版),2005,39(7):983——986.ZHANGYi—ping,WANGShi-jin,ZHANGTu—qiao.Studyonmulti—layerrecursivetimeseriesmodelforsettlementprediction[J].JournalofZhejiangUniversity (EngineeringScience),2005,39(7):983--986. [1IJ姜继忱.关于一类递推算法的进一步探讨fJ1.黑龙江大学(自然科学版),1982,(1):20—29.【l2】吕培印,杨锦军,陈伟.时间序列分析法预报建筑物地基沉降『J】.辽宁工程学院,1996,16(4):27--30.LUPei—yin,YANGJin-jun,CHENWei.Timeseries methodforforecastingsettlementofbuildingfoundation[J].JournalofLiaoningInstituteof Technology,1996,l6(4):27—30.【l3】徐浩峰,应宏伟,朱向荣.时序分析预报基坑周围建筑物沉降【J】_建筑技术,2003,34(2):l09—110.XUHao—feng,YINGHong—wei,ZHUXiang—rong.Time—seriesmethodforforecastingsettlementofbuilding besidefoundationpits[J].ArchitectureTechnology,2003,34(2):109—110.建筑旅工计算手册(第二版)建筑科学类/江正荣N~:/4,16开/l000:~-W800页/精装/估价:88.~/2007年5月出版/ISBN:978—7—112—09l44—7本手册主要介绍工业与民用建筑施工经常遇到的各类有关施工计算问题.全书共有22章,主要包括:土方工程,基坑支护工程,地下水控制工程,地基处理工程,地基与基础工程,砌体与墙体工程,脚手架工程等,基本覆盖了建筑施工计算的主要应用领域.书中对每项计算公式均有详细的说明或推导,附有必要的图表资料,参考数据以及大量的典型计算实例.书后的两个附录是施工常用计算数据和施工常用结构计算用表及公式.读者对象:施工技术人员,管理人员,土建设计人员.(摘自新华书目报?科技新书目)。
holt-winter-multiplicative法 -回复
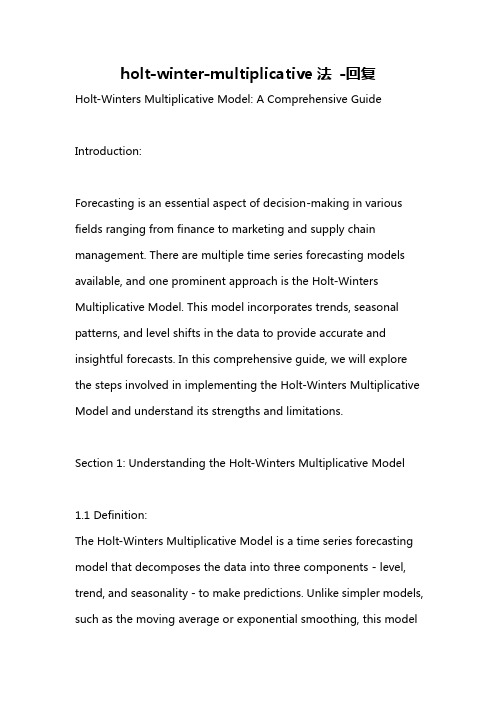
holt-winter-multiplicative法-回复Holt-Winters Multiplicative Model: A Comprehensive GuideIntroduction:Forecasting is an essential aspect of decision-making in various fields ranging from finance to marketing and supply chain management. There are multiple time series forecasting models available, and one prominent approach is the Holt-Winters Multiplicative Model. This model incorporates trends, seasonal patterns, and level shifts in the data to provide accurate and insightful forecasts. In this comprehensive guide, we will explore the steps involved in implementing the Holt-Winters Multiplicative Model and understand its strengths and limitations.Section 1: Understanding the Holt-Winters Multiplicative Model1.1 Definition:The Holt-Winters Multiplicative Model is a time series forecasting model that decomposes the data into three components - level, trend, and seasonality - to make predictions. Unlike simpler models, such as the moving average or exponential smoothing, this modelallows for dynamic adjustment of these three components, enabling accurate forecasting.1.2 Characteristics:The Holt-Winters Multiplicative Model is widely used due to its flexibility and ability to capture nonlinear trends and seasonal patterns in the data. It is particularly effective when the data exhibits a changing trend and fluctuating patterns. Moreover, this model provides robust forecasts by incorporating both short-term and long-term dependencies.Section 2: Implementing the Holt-Winters Multiplicative Model2.1 Data Preprocessing:Before implementing the Holt-Winters Multiplicative Model, it is essential to preprocess the data. This involves identifying and handling missing values, outliers, and other anomalies. Additionally, the time series data should be transformed if it exhibitsnon-constant variance or non-linear relationships.2.2 Model Initialization:The Holt-Winters Multiplicative Model requires initializing the threecomponents - level, trend, and seasonality. This can be done using various methods, such as simple averages, linear regression, or exponential smoothing. The choice of initialization method depends on the characteristics of the data and the analyst's expertise.2.3 Parameter Estimation:Once the model is initialized, the next step is to estimate the parameters. This involves finding the optimal values for the smoothing constants (alpha, beta, and gamma) and the length of the seasonal period. Parameter estimation can be performed using iterative optimization techniques, such as least squares or maximum likelihood estimation.2.4 Model Fitting and Forecasting:After estimating the parameters, the Holt-Winters Multiplicative Model is fitted to the data. This involves updating the level, trend, and seasonality components iteratively using the chosen optimization technique. Once the model is fitted, it can be used to forecast future values by extrapolating the trend and seasonal patterns.Section 3: Evaluating and Interpreting Holt-Winters Multiplicative Model3.1 Model Evaluation:To assess the performance of the Holt-Winters Multiplicative Model, various evaluation metrics can be employed. These include mean absolute error (MAE), mean squared error (MSE), root mean squared error (RMSE), and mean absolute percentage error (MAPE). Comparison with alternative models and techniques is also crucial to gauge the model's accuracy.3.2 Interpretation:Interpreting the results of the Holt-Winters Multiplicative Model is essential for decision-making. By analyzing the estimated components - level, trend, and seasonality - one can gain insights into the direction and magnitude of changes in the time series. This information can be leveraged to understand the underlying factors driving the data and make informed predictions.Section 4: Strengths and Limitations of Holt-Winters Multiplicative Model4.1 Strengths:The Holt-Winters Multiplicative Model has several strengths. It captures both trend and seasonal patterns, providing accurate forecasts for complex time series data. Additionally, it allows for flexible adjustment of the three components, enabling dynamic adaptation to changing patterns. Moreover, this model accommodates both short-term and long-term dependencies, enhancing its forecasting capabilities.4.2 Limitations:Despite its strengths, the Holt-Winters Multiplicative Model has some limitations. It assumes that the components - level, trend, and seasonality - are multiplicative, which may not always hold true in practice. Additionally, this model requires historical data that demonstrate stable patterns, making it less suitable for highly volatile or irregular time series. Moreover, this model has limited explanatory capabilities, focusing primarily on forecasting rather than detailed causal analysis.Conclusion:The Holt-Winters Multiplicative Model is a powerful forecastingapproach that incorporates trends, seasonality, and level shifts in the data. By understanding its characteristics, implementing the model step-by-step, and evaluating its performance, analysts can leverage this technique to make accurate predictions. However, it is essential to be aware of the model's limitations and interpret the results cautiously. With its ability to capture complex patterns, the Holt-Winters Multiplicative Model offers valuable insights for decision-making in various industries.。
时间序列与arima模型的关系

英文回复:Time—series data are observations or records over time that are important for analysing and predicting future trends,cyclicality and regularity。
As amon statistical method, time—series analysis is aimed at effectively predicting future developments through in—depth analysis of historical data。
For time series analysis, there are many models and methods,of which the ARIMA model is an effective one。
At this critical point, we should pursue an approach that closely integrates practical, integrated and scientific decision—making and promotes continuous innovation in time—series analysis theory and methodology to better serve our countries and peoples。
时间序列数据是指各种数据随着时间的推移所呈现出的观测结果或记录,其对于分析和预测未来的趋势、周期性和规律性具有重要意义。
时间序列分析作为一种常见的统计方法,通过对历史数据的深入剖析,旨在有效预测未来的发展走势。
针对时间序列分析,存在多种模型和方法,其中ARIMA模型为行之有效的一种。
值此关键节点,我们应坚持紧密结合实际、统筹兼顾、科学决策的方针,推动时间序列分析理论与方法的不断创新,以更好地服务于我们的国家和人民大众。
数据、模型与决策(运筹学)课后习题和案例答案013
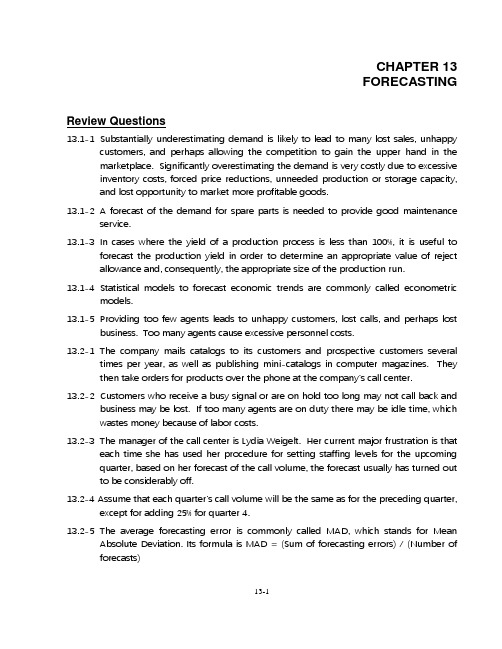
CHAPTER 13FORECASTINGReview Questions13.1-1 Substantially underestimating demand is likely to lead to many lost sales, unhappycustomers, and perhaps allowing the competition to gain the upper hand in the marketplace. Significantly overestimating the demand is very costly due to excessive inventory costs, forced price reductions, unneeded production or storage capacity, and lost opportunity to market more profitable goods.13.1-2 A forecast of the demand for spare parts is needed to provide good maintenanceservice.13.1-3 In cases where the yield of a production process is less than 100%, it is useful toforecast the production yield in order to determine an appropriate value of reject allowance and, consequently, the appropriate size of the production run.13.1-4 Statistical models to forecast economic trends are commonly called econometricmodels.13.1-5 Providing too few agents leads to unhappy customers, lost calls, and perhaps lostbusiness. Too many agents cause excessive personnel costs.13.2-1 The company mails catalogs to its customers and prospective customers severaltimes per year, as well as publishing mini-catalogs in computer magazines. They then take orders for products over the phone at the company’s call center.13.2-2 Customers who receive a busy signal or are on hold too long may not call back andbusiness may be lost. If too many agents are on duty there may be idle time, which wastes money because of labor costs.13.2-3 The manager of the call center is Lydia Weigelt. Her current major frustration is thateach time she has used her procedure for setting staffing levels for the upcoming quarter, based on her forecast of the call volume, the forecast usually has turned out to be considerably off.13.2-4 Assume that each quarter’s cal l volume will be the same as for the preceding quarter,except for adding 25% for quarter 4.13.2-5 The average forecasting error is commonly called MAD, which stands for MeanAbsolute Deviation. Its formula is MAD = (Sum of forecasting errors) / (Number of forecasts)13.2-6 MSE is the mean square error. Its formula is (Sum of square of forecasting errors) /(Number of forecasts).13.2-7 A time series is a series of observations over time of some quantity of interest.13.3-1 In general, the seasonal factor for any period of a year measures how that periodcompares to the overall average for an entire year.13.3-2 Seasonally adjusted call volume = (Actual call volume) / (Seasonal factor).13.3-3 Actual forecast = (Seasonal factor)(Seasonally adjusted forecast)13.3-4 The last-value forecasting method sometimes is called the naive method becausestatisticians consider it naive to use just a sample size of one when additional relevant data are available.13.3-5 Conditions affecting the CCW call volume were changing significantly over the pastthree years.13.3-6 Rather than using old data that may no longer be relevant, this method averages thedata for only the most recent periods.13.3-7 This method modifies the moving-average method by placing the greatest weighton the last value in the time series and then progressively smaller weights on the older values.13.3-8 A small value is appropriate if conditions are remaining relatively stable. A largervalue is needed if significant changes in the conditions are occurring relatively frequently.13.3-9 Forecast = α(Last Value) + (1 –α)(Last forecast). Estimated trend is added to thisformula when using exponential smoothing with trend.13.3-10 T he one big factor that drives total sales up or down is whether there are any hotnew products being offered.13.4-1 CB Predictor uses the raw data to provide the best fit for all these inputs as well asthe forecasts.13.4-2 Each piece of data should have only a 5% chance of falling below the lower line and a5% chance of rising above the upper line.13.5-1 The next value that will occur in a time series is a random variable.13.5-2 The goal of time series forecasting methods is to estimate the mean of theunderlying probability distribution of the next value of the time series as closely as possible.13.5-3 No, the probability distribution is not the same for every quarter.13.5-4 Each of the forecasting methods, except for the last-value method, placed at leastsome weight on the observations from Year 1 to estimate the mean for each quarter in Year 2. These observations, however, provide a poor basis for estimating the mean of the Year 2 distribution.13.5-5 A time series is said to be stable if its underlying probability distribution usuallyremains the same from one time period to the next. A time series is unstable if both frequent and sizable shifts in the distribution tend to occur.13.5-6 Since sales drive call volume, the forecasting process should begin by forecastingsales.13.5-7 The major components are the relatively stable market base of numerous small-niche products and each of a few major new products.13.6-1 Causal forecasting obtains a forecast of the quantity of interest by relating it directlyto one or more other quantities that drive the quantity of interest.13.6-2 The dependent variable is call volume and the independent variable is sales.13.6-3 When doing causal forecasting with a single independent variable, linear regressioninvolves approximating the relationship between the dependent variable and the independent variable by a straight line.13.6-4 In general, the equation for the linear regression line has the form y = a + bx. Ifthere is more than one independent variable, then this regression equation has a term, a constant times the variable, added on the right-hand side for each of these variables.13.6-5 The procedure used to obtain a and b is called the method of least squares.13.6-6 The new procedure gives a MAD value of only 120 compared with the old MADvalue of 400 with the 25% rule.13.7-1 Statistical forecasting methods cannot be used if no data are available, or if the dataare not representative of current conditions.13.7-2 Even when good data are available, some managers prefer a judgmental methodinstead of a formal statistical method. In many other cases, a combination of the two may be used.13.7-3 The jury of executive opinion method involves a small group of high-level managerswho pool their best judgment to collectively make a forecast rather than just the opinion of a single manager.13.7-4 The sales force composite method begins with each salesperson providing anestimate of what sales will be in his or her region.13.7-5 A consumer market survey is helpful for designing new products and then indeveloping the initial forecasts of their sales. It is also helpful for planning a marketing campaign.13.7-6 The Delphi method normally is used only at the highest levels of a corporation orgovernment to develop long-range forecasts of broad trends.13.8-1 Generally speaking, judgmental forecasting methods are somewhat more widelyused than statistical methods.13.8-2 Among the judgmental methods, the most popular is a jury of executive opinion.Manager’s opinion is a close second.13.8-3 The survey indicates that the moving-average method and linear regression are themost widely used statistical forecasting methods.Problems13.1 a) Forecast = last value = 39b) Forecast = average of all data to date = (5 + 17 + 29 + 41 + 39) / 5 = 131 / 5 =26c) Forecast = average of last 3 values = (29 + 41 + 39) / 3 = 109 / 3 = 36d) It appears as if demand is rising so the average forecasting method seemsinappropriate because it uses older, out-of-date data.13.2 a) Forecast = last value = 13b) Forecast = average of all data to date = (15 + 18 + 12 + 17 + 13) / 5 = 75 / 5 =15c) Forecast = average of last 3 values = (12 + 17 + 13) / 3 = 42 / 3 = 14d) The averaging method seems best since all five months of data are relevant indetermining the forecast of sales for next month and the data appears relativelystable.13.3MAD = (Sum of forecasting errors) / (Number of forecasts) = (18 + 15 + 8 + 19) / 4 = 60 / 4 = 15 MSE = (Sum of squares of forecasting errors) / (Number of forecasts) = (182 + 152 + 82 + 192) / 4 = 974 / 4 = 243.513.4 a) Method 1 MAD = (258 + 499 + 560 + 809 + 609) / 5 = 2,735 / 5 = 547Method 2 MAD = (374 + 471 + 293 + 906 + 396) / 5 = 2,440 / 5 = 488Method 1 MSE = (2582 + 4992 + 5602 + 8092 + 6092) / 5 = 1,654,527 / 5 = 330,905Method 2 MSE = (3742 + 4712 + 2932 + 9062 + 3962) / 5 = 1,425,218 / 5 = 285,044Method 2 gives a lower MAD and MSE.b) She can use the older data to calculate more forecasting errors and compareMAD for a longer time span. She can also use the older data to forecast theprevious five months to see how the methods compare. This may make her feelmore comfortable with her decision.13.5 a)b)c)d)13.6 a)b)This progression indicatesthat the state’s economy is improving with the unemployment rate decreasing from 8% to 7% (seasonally adjusted) over the four quarters.13.7 a)b) Seasonally adjusted value for Y3(Q4)=28/1.04=27,Actual forecast for Y4(Q1) = (27)(0.84) = 23.c) Y4(Q1) = 23 as shown in partb Seasonally adjusted value for Y4(Q1) = 23 / 0.84 = 27 Actual forecast for Y4(Q2) = (27)(0.92) = 25Seasonally adjusted value for Y4(Q2) = 25 / 0.92 = 27 Actual forecast for Y4(Q3) = (27)(1.20) = 33Seasonally adjusted value for Y4(Q3) = 33/1.20 = 27Actual forecast for Y4(Q4) = (27)(1.04) = 28d)13.8 Forecast = 2,083 – (1,945 / 4) + (1,977 / 4) = 2,09113.9 Forecast = 782 – (805 / 3) + (793 / 3) = 77813.10 Forecast = 1,551 – (1,632 / 10) + (1,532 / 10) = 1,54113.11 Forecast(α) = α(last value) + (1 –α)(last forecast)Forecast(0.1) = (0.1)(792) + (1 –0.1)(782) = 783 Forecast(0.3) = (0.3)(792) + (1 –0.3)(782) = 785 Forecast(0.5) = (0.5)(792) + (1 – 0.5)(782) = 78713.12 Forecast(α) = α(last value) + (1 –α)(last forecast)Forecast(0.1) = (0.1)(1,973) + (1 –0.1)(2,083) = 2,072 Forecast(0.3) = (0.3)(1,973) + (1 –0.3)(2,083) = 2,050 Forecast(0.5) = (0.5)(1,973) + (1 – 0.5)(2,083) = 2,02813.13 a) Forecast(year 1) = initial estimate = 5000Forecast(year 2) = α(last value) + (1 –α)(last forecast)= (0.25)(4,600) + (1 –0.25)(5,000) = 4,900 Forecast(year 3) = (0.25)(5,300) + (1 – 0.25)(4,900) = 5,000b) MAD = (400 + 400 + 1,000) / 3 = 600MSE = (4002 + 4002 + 1,0002) / 3 = 440,000c) Forecast(next year) = (0.25)(6,000) + (1 – 0.25)(5,000) = 5,25013.14 Forecast = α(last value) + (1 –α)(last forecast) + Estimated trendEstimated trend = β(Latest trend) + (1 –β)(Latest estimate of trend) Latest trend = α(Last value – Next-to-last value) + (1 –α)(Last forecast – Next-to-last forecast)Forecast(year 1) = Initial average + Initial trend = 3,900 + 700 = 4,600Forecast (year 2) = (0.25)(4,600) + (1 –0.25)(4,600)+(0.25)[(0.25)(4,600 –3900) + (1 –0.25)(4,600 –3,900)] + (1 –0.25)(700) = 5,300Forecast (year 3) = (0.25)(5,300) + (1 – 0.25)(5,300) + (0.25)[(0.25)(5,300 – 4,600) + (1 – 0.25)(5,300 – 4,600)]+(1 – 0.25)(700) = 6,00013.15 Forecast = α(last value) + (1 –α)(last forecast) + Estimated trendEstimated trend = β(Latest trend) + (1 –β)(Latest estimate of trend) Latest trend = α(Last value – Next-to-last value) + (1 –α)(Last forecast – Next-to-last forecast)Forecast = (0.2)(550) + (1 – 0.2)(540) + (0.3)[(0.2)(550 – 535) + (1 – 0.2)(540 –530)] + (1 – 0.3)(10) = 55213.16 Forecast = α(last value) + (1 –α)(last forecast) + Estimated trendEstimated trend = β(Latest trend) + (1 –β)(Latest estimate of trend) Latest trend = α(Last value – Next-to-last value) + (1 –α)(Last forecast – Next-to-last forecast)Forecast = (0.1)(4,935) + (1 – 0.1)(4,975) + (0.2)[(0.1)(4,935 – 4,655) + (1 – 0.1) (4,975 – 4720)] + (1 – 0.2)(240) = 5,21513.17 a) Since sales are relatively stable, the averaging method would be appropriate forforecasting future sales. This method uses a larger sample size than the last-valuemethod, which should make it more accurate and since the older data is stillrelevant, it should not be excluded, as would be the case in the moving-averagemethod.b)c)d)e) Considering the MAD values (5.2, 3.0, and 3.9, respectively), the averagingmethod is the best one to use.f) Considering the MSE values (30.6, 11.1, and 17.4, respectively), the averagingmethod is the best one to use.g) Unless there is reason to believe that sales will not continue to be relatively stable,the averaging method should be the most accurate in the future as well.13.18 Using the template for exponential smoothing, with an initial estimate of 24, thefollowing forecast errors were obtained for various values of the smoothing constant α:use.13.19 a) Answers will vary. Averaging or Moving Average appear to do a better job thanLast Value.b) For Last Value, a change in April will only affect the May forecast.For Averaging, a change in April will affect all forecasts after April.For Moving Average, a change in April will affect the May, June, and July forecast.c) Answers will vary. Averaging or Moving Average appear to do a slightly better jobthan Last Value.d) Answers will vary. Averaging or Moving Average appear to do a slightly better jobthan Last Value.13.20 a) Since the sales level is shifting significantly from month to month, and there is noconsistent trend, the last-value method seems like it will perform well. Theaveraging method will not do as well because it places too much weight on olddata. The moving-average method will be better than the averaging method butwill lag any short-term trends. The exponential smoothing method will also lagtrends by placing too much weight on old data. Exponential smoothing withtrend will likely not do well because the trend is not consistent.b)Comparing MAD values (5.3, 10.0, and 8.1, respectively), the last-value method is the best to use of these three options.Comparing MSE values (36.2, 131.4, and 84.3, respectively), the last-value method is the best to use of these three options.c) Using the template for exponential smoothing, with an initial estimate of 120, thefollowing forecast errors were obtained for various values of the smoothingconstant α:constant is appropriate.d) Using the template for exponential smoothing with trend, using initial estimates of120 for the average value and 10 for the trend, the following forecast errors wereobtained for various values of the smoothing constants α and β:constants is appropriate.e) Management should use the last-value method to forecast sales. Using thismethod the forecast for January of the new year will be 166. Exponentialsmoothing with trend with high smoothing constants (e.g., α = 0.5 and β = 0.5)also works well. With this method, the forecast for January of the new year will be165.13.21 a) Shift in total sales may be due to the release of new products on top of a stableproduct base, as was seen in the CCW case study.b) Forecasting might be improved by breaking down total sales into stable and newproducts. Exponential smoothing with a relatively small smoothing constant canbe used for the stable product base. Exponential smoothing with trend, with arelatively large smoothing constant, can be used for forecasting sales of each newproduct.c) Managerial judgment is needed to provide the initial estimate of anticipated salesin the first month for new products. In addition, a manger should check theexponential smoothing forecasts and make any adjustments that may benecessary based on knowledge of the marketplace.13.22 a) Answers will vary. Last value seems to do the best, with exponential smoothingwith trend a close second.b) For last value, a change in April will only affect the May forecast.For averaging, a change in April will affect all forecasts after April.For moving average, a change in April will affect the May, June, and July forecast.For exponential smoothing, a change in April will affect all forecasts after April.For exponential smoothing with trend, a change in April will affect all forecastsafter April.c) Answers will vary. last value or exponential smoothing seem to do better than theaveraging or moving average.d) Answers will vary. last value or exponential smoothing seem to do better than theaveraging or moving average.13.23 a) Using the template for exponential smoothing, with an initial estimate of 50, thefollowing MAD values were obtained for various values of the smoothing constantα:Choose αb) Using the template for exponential smoothing, with an initial estimate of 50, thefollowing MAD values were obtained for various values of the smoothing constantα:Choose αc) Using the template for exponential smoothing, with an initial estimate of 50, thefollowing MAD values were obtained for various values of the smoothing constantα:13.24 a)b)Forecast = 51.c) Forecast = 54.13.25 a) Using the template for exponential smoothing with trend, with an initial estimatesof 50 for the average and 2 for the trend and α = 0.2, the following MAD values were obtained for various values of the smoothing constant β:Choose β = 0.1b) Using the template for exponential smoothing with trend, with an initial estimatesof 50 for the average and 2 for the trend and α = 0.2, the following MAD valueswere obtained for various values of the smoothing constant β:Choose β = 0.1c) Using the template for exponential smoothing with trend, with an initial estimatesof 50 for the average and 2 for the trend and α = 0.2, the following MAD valueswere obtained for various values of the smoothing constant β:13.26 a)b)0.582. Forecast = 74.c) = 0.999. Forecast = 79.13.27 a) The time series is not stable enough for the moving-average method. Thereappears to be an upward trend.b)c)d)e) Based on the MAD and MSE values, exponential smoothing with trend should beused in the future.β = 0.999.f)For exponential smoothing, the forecasts typically lie below the demands.For exponential smoothing with trend, the forecasts are at about the same level as demand (perhaps slightly above).This would indicate that exponential smoothing with trend is the best method to usehereafter.13.2913.30 a)factors:b)c) Winter = (49)(0.550) = 27Spring = (49)(1.027) = 50Summer = (49)(1.519) = 74Fall = (49)(0.904) = 44d)e)f)g) The exponential smoothing method results in the lowest MAD value (1.42) and thelowest MSE value (2.75).13.31 a)b)c)d)e)f)g) The last-value method with seasonality has the lowest MAD and MSE value. Usingthis method, the forecast for Q1 is 23 houses.h) Forecast(Q2) = (27)(0.92) = 25Forecast(Q3) = (27)(1.2) = 32Forecast(Q4) = (27)(1.04) = 2813.32 a)b) The moving-average method with seasonality has the lowest MAD value. Using13.33 a)b)c)d) Exponential smoothing with trend should be used.e) The best values for the smoothing constants are α = 0.3, β = 0.3, and γ = 0.001.C28:C38 below.13.34 a)b)c)d)e) Moving average results in the best MAD value (13.30) and the best MSE value(249.09).f)MAD = 14.17g) Moving average performed better than the average of all three so it should beused next year.h) The best method is exponential smoothing with seasonality and trend, using13.35 a)••••••••••0100200300400500600012345678910S a l e sMonthb)c)••••••••••0100200300400500600012345678910S a l e sMonthd) y = 410.33 + (17.63)(11) = 604 e) y = 410.33 + (17.63)(20) = 763f) The average growth in sales per month is 17.63.13.36 a)•••01000200030004000500060000123A p p l i c a t i o n sYearb)•••01000200030004000500060000123A p p l i c a t i o n sYearc)d) y (year 4) = 3,900+ (700)(4) = 6,700 y (year 5) = 3,900 + (700)(5) = 7,400 y (year 6) = 3,900 + (700)(6) = 8,100 y (year 7) = 3,900 + (700)(7) =8,800y (year 8) = 3,900 + (700)(8) = 9,500e) It does not make sense to use the forecast obtained earlier of 9,500. Therelationship between the variable has changed and, thus, the linear regression that was used is no longer appropriate.f)•••••••0100020003000400050006000700001234567A p p l i c a t i o n sYeary =5,229 +92.9x y =5,229+(92.9)(8)=5,971the forecast that it provides for year 8 is not likely to be accurate. It does not make sense to continue to use a linear regression line when changing conditions cause a large shift in the underlying trend in the data.g)Causal forecasting takes all the data into account, even the data from before changing conditions cause a shift. Exponential smoothing with trend adjusts to shifts in the underlying trend by placing more emphasis on the recent data.13.37 a)••••••••••50100150200250300350400450500012345678910A n n u a l D e m a n dYearb)c)••••••••••50100150200250300350400450500012345678910A n n u a l D e m a n dYeard) y = 380 + (8.15)(11) = 470 e) y = 380 = (8.15)(15) = 503f) The average growth per year is 8.15 tons.13.38 a) The amount of advertising is the independent variable and sales is the dependentvariable.b)•••••0510*******100200300400500S a l e s (t h o u s a n d s o f p a s s e n g e r s )Amount of Advertising ($1,000s)c)•••••0510*******100200300400500S a l e s (t h o u s a n d s o f p a s s e n g e r s )Amount of Advertising ($1,000s)d) y = 8.71 + (0.031)(300) = 18,000 passengers e) 22 = 8.71 + (0.031)(x ) x = $429,000f) An increase of 31 passengers can be attained.13.39 a) If the sales change from 16 to 19 when the amount of advertising is 225, then thelinear regression line shifts below this point (the line actually shifts up, but not as much as the data point has shifted up).b) If the sales change from 23 to 26 when the amount of advertising is 450, then the linear regression line shifts below this point (the line actually shifts up, but not as much as the data point has shifted up).c) If the sales change from 20 to 23 when the amount of advertising is 350, then the linear regression line shifts below this point (the line actually shifts up, but not as much as the data point has shifted up).13.40 a) The number of flying hours is the independent variable and the number of wingflaps needed is the dependent variable.b)••••••024*********100200W i n g F l a p s N e e d e dFlying Hours (thousands)c)d)••••••024*********100200W i n g F l a p s N e e d e dFlying Hours (thousands)e) y = -3.38 + (0.093)(150) = 11f) y = -3.38 + (0.093)(200) = 1513.41 Joe should use the linear regression line y = –9.95 + 0.10x to develop a forecast forCase13.1 a) We need to forecast the call volume for each day separately.1) To obtain the seasonally adjusted call volume for the past 13 weeks, we firsthave to determine the seasonal factors. Because call volumes follow seasonalpatterns within the week, we have to calculate a seasonal factor for Monday,Tuesday, Wednesday, Thursday, and Friday. We use the Template for SeasonalFactors. The 0 values for holidays should not factor into the average. Leaving themblank (rather than 0) accomplishes this. (A blank value does not factor into theAVERAGE function in Excel that is used to calculate the seasonal values.) Using thistemplate (shown on the following page, the seasonal factors for Monday, Tuesday,Wednesday, Thursday, and Friday are 1.238, 1.131, 0.999, 0.850, and 0.762,respectively.2) To forecast the call volume for the next week using the last-value forecasting method, we need to use the Last Value with Seasonality template. To forecast the next week, we need only start with the last Friday value since the Last Value method only looks at the previous day.The forecasted call volume for the next week is 5,045 calls: 1,254 calls are received on Monday, 1,148 calls are received on Tuesday, 1,012 calls are received on Wednesday, 860 calls are received on Thursday, and 771 calls are received on Friday.3) To forecast the call volume for the next week using the averaging forecasting method, we need to use the Averaging with Seasonality template.The forecasted call volume for the next week is 4,712 calls: 1,171 calls are received on Monday, 1,071 calls are received on Tuesday, 945 calls are received on Wednesday, 804 calls are received on Thursday, and 721 calls are received onFriday.4) To forecast the call volume for the next week using the moving-average forecasting method, we need to use the Moving Averaging with Seasonality template. Since only the past 5 days are used in the forecast, we start with Monday of the last week to forecast through Friday of the next week.The forecasted call volume for the next week is 4,124 calls: 985 calls are received on Monday, 914 calls are received on Tuesday, 835 calls are received on Wednesday, 732 calls are received on Thursday, and 658 calls are received on Friday.5) To forecast the call volume for the next week using the exponential smoothing forecasting method, we need to use the Exponential with Seasonality template. We start with the initial estimate of 1,125 calls (the average number of calls on non-holidays during the previous 13 weeks).The forecasted call volume for the next week is 4,322 calls: 1,074 calls are received on Monday, 982 calls are received on Tuesday, 867 calls are received onWednesday, 737 calls are received on Thursday, and 661 calls are received on Friday.b) To obtain the mean absolute deviation for each forecasting method, we simplyneed to subtract the true call volume from the forecasted call volume for each day in the sixth week. We then need to take the absolute value of the five differences.Finally, we need to take the average of these five absolute values to obtain the mean absolute deviation.1) The spreadsheet for the calculation of the mean absolute deviation for the last-value forecasting method follows.This method is the least effective of the four methods because this method depends heavily upon the average seasonality factors. If the average seasonality factors are not the true seasonality factors for week 6, a large error will appear because the average seasonality factors are used to transform the Friday call volume in week 5 to forecasts for all call volumes in week 6. We calculated in part(a) that the call volume for Friday is 0.762 times lower than the overall average callvolume. In week 6, however, the call volume for Friday is only 0.83 times lower than the average call volume over the week. Also, we calculated that the call volume for Monday is 1.34 times higher than the overall average call volume. In Week 6, however, the call volume for Monday is only 1.21 times higher than the average call volume over the week. These differences introduce a large error.。
T.W. ANDERSON (1971). The Statistical Analysis of Time Series. Series in Probability and Ma

425 BibliographyH.A KAIKE(1974).Markovian representation of stochastic processes and its application to the analysis of autoregressive moving average processes.Annals Institute Statistical Mathematics,vol.26,pp.363-387. B.D.O.A NDERSON and J.B.M OORE(1979).Optimal rmation and System Sciences Series, Prentice Hall,Englewood Cliffs,NJ.T.W.A NDERSON(1971).The Statistical Analysis of Time Series.Series in Probability and Mathematical Statistics,Wiley,New York.R.A NDRE-O BRECHT(1988).A new statistical approach for the automatic segmentation of continuous speech signals.IEEE Trans.Acoustics,Speech,Signal Processing,vol.ASSP-36,no1,pp.29-40.R.A NDRE-O BRECHT(1990).Reconnaissance automatique de parole`a partir de segments acoustiques et de mod`e les de Markov cach´e s.Proc.Journ´e es Etude de la Parole,Montr´e al,May1990(in French).R.A NDRE-O BRECHT and H.Y.S U(1988).Three acoustic labellings for phoneme based continuous speech recognition.Proc.Speech’88,Edinburgh,UK,pp.943-950.U.A PPEL and A.VON B RANDT(1983).Adaptive sequential segmentation of piecewise stationary time rmation Sciences,vol.29,no1,pp.27-56.L.A.A ROIAN and H.L EVENE(1950).The effectiveness of quality control procedures.Jal American Statis-tical Association,vol.45,pp.520-529.K.J.A STR¨OM and B.W ITTENMARK(1984).Computer Controlled Systems:Theory and rma-tion and System Sciences Series,Prentice Hall,Englewood Cliffs,NJ.M.B AGSHAW and R.A.J OHNSON(1975a).The effect of serial correlation on the performance of CUSUM tests-Part II.Technometrics,vol.17,no1,pp.73-80.M.B AGSHAW and R.A.J OHNSON(1975b).The influence of reference values and estimated variance on the ARL of CUSUM tests.Jal Royal Statistical Society,vol.37(B),no3,pp.413-420.M.B AGSHAW and R.A.J OHNSON(1977).Sequential procedures for detecting parameter changes in a time-series model.Jal American Statistical Association,vol.72,no359,pp.593-597.R.K.B ANSAL and P.P APANTONI-K AZAKOS(1986).An algorithm for detecting a change in a stochastic process.IEEE rmation Theory,vol.IT-32,no2,pp.227-235.G.A.B ARNARD(1959).Control charts and stochastic processes.Jal Royal Statistical Society,vol.B.21, pp.239-271.A.E.B ASHARINOV andB.S.F LEISHMAN(1962).Methods of the statistical sequential analysis and their radiotechnical applications.Sovetskoe Radio,Moscow(in Russian).M.B ASSEVILLE(1978).D´e viations par rapport au maximum:formules d’arrˆe t et martingales associ´e es. Compte-rendus du S´e minaire de Probabilit´e s,Universit´e de Rennes I.M.B ASSEVILLE(1981).Edge detection using sequential methods for change in level-Part II:Sequential detection of change in mean.IEEE Trans.Acoustics,Speech,Signal Processing,vol.ASSP-29,no1,pp.32-50.426B IBLIOGRAPHY M.B ASSEVILLE(1982).A survey of statistical failure detection techniques.In Contribution`a la D´e tectionS´e quentielle de Ruptures de Mod`e les Statistiques,Th`e se d’Etat,Universit´e de Rennes I,France(in English). M.B ASSEVILLE(1986).The two-models approach for the on-line detection of changes in AR processes. In Detection of Abrupt Changes in Signals and Dynamical Systems(M.Basseville,A.Benveniste,eds.). Lecture Notes in Control and Information Sciences,LNCIS77,Springer,New York,pp.169-215.M.B ASSEVILLE(1988).Detecting changes in signals and systems-A survey.Automatica,vol.24,pp.309-326.M.B ASSEVILLE(1989).Distance measures for signal processing and pattern recognition.Signal Process-ing,vol.18,pp.349-369.M.B ASSEVILLE and A.B ENVENISTE(1983a).Design and comparative study of some sequential jump detection algorithms for digital signals.IEEE Trans.Acoustics,Speech,Signal Processing,vol.ASSP-31, no3,pp.521-535.M.B ASSEVILLE and A.B ENVENISTE(1983b).Sequential detection of abrupt changes in spectral charac-teristics of digital signals.IEEE rmation Theory,vol.IT-29,no5,pp.709-724.M.B ASSEVILLE and A.B ENVENISTE,eds.(1986).Detection of Abrupt Changes in Signals and Dynamical Systems.Lecture Notes in Control and Information Sciences,LNCIS77,Springer,New York.M.B ASSEVILLE and I.N IKIFOROV(1991).A unified framework for statistical change detection.Proc.30th IEEE Conference on Decision and Control,Brighton,UK.M.B ASSEVILLE,B.E SPIAU and J.G ASNIER(1981).Edge detection using sequential methods for change in level-Part I:A sequential edge detection algorithm.IEEE Trans.Acoustics,Speech,Signal Processing, vol.ASSP-29,no1,pp.24-31.M.B ASSEVILLE, A.B ENVENISTE and G.M OUSTAKIDES(1986).Detection and diagnosis of abrupt changes in modal characteristics of nonstationary digital signals.IEEE rmation Theory,vol.IT-32,no3,pp.412-417.M.B ASSEVILLE,A.B ENVENISTE,G.M OUSTAKIDES and A.R OUG´E E(1987a).Detection and diagnosis of changes in the eigenstructure of nonstationary multivariable systems.Automatica,vol.23,no3,pp.479-489. M.B ASSEVILLE,A.B ENVENISTE,G.M OUSTAKIDES and A.R OUG´E E(1987b).Optimal sensor location for detecting changes in dynamical behavior.IEEE Trans.Automatic Control,vol.AC-32,no12,pp.1067-1075.M.B ASSEVILLE,A.B ENVENISTE,B.G ACH-D EVAUCHELLE,M.G OURSAT,D.B ONNECASE,P.D OREY, M.P REVOSTO and M.O LAGNON(1993).Damage monitoring in vibration mechanics:issues in diagnos-tics and predictive maintenance.Mechanical Systems and Signal Processing,vol.7,no5,pp.401-423.R.V.B EARD(1971).Failure Accommodation in Linear Systems through Self-reorganization.Ph.D.Thesis, Dept.Aeronautics and Astronautics,MIT,Cambridge,MA.A.B ENVENISTE and J.J.F UCHS(1985).Single sample modal identification of a nonstationary stochastic process.IEEE Trans.Automatic Control,vol.AC-30,no1,pp.66-74.A.B ENVENISTE,M.B ASSEVILLE and G.M OUSTAKIDES(1987).The asymptotic local approach to change detection and model validation.IEEE Trans.Automatic Control,vol.AC-32,no7,pp.583-592.A.B ENVENISTE,M.M ETIVIER and P.P RIOURET(1990).Adaptive Algorithms and Stochastic Approxima-tions.Series on Applications of Mathematics,(A.V.Balakrishnan,I.Karatzas,M.Yor,eds.).Springer,New York.A.B ENVENISTE,M.B ASSEVILLE,L.E L G HAOUI,R.N IKOUKHAH and A.S.W ILLSKY(1992).An optimum robust approach to statistical failure detection and identification.IFAC World Conference,Sydney, July1993.B IBLIOGRAPHY427 R.H.B ERK(1973).Some asymptotic aspects of sequential analysis.Annals Statistics,vol.1,no6,pp.1126-1138.R.H.B ERK(1975).Locally most powerful sequential test.Annals Statistics,vol.3,no2,pp.373-381.P.B ILLINGSLEY(1968).Convergence of Probability Measures.Wiley,New York.A.F.B ISSELL(1969).Cusum techniques for quality control.Applied Statistics,vol.18,pp.1-30.M.E.B IVAIKOV(1991).Control of the sample size for recursive estimation of parameters subject to abrupt changes.Automation and Remote Control,no9,pp.96-103.R.E.B LAHUT(1987).Principles and Practice of Information Theory.Addison-Wesley,Reading,MA.I.F.B LAKE and W.C.L INDSEY(1973).Level-crossing problems for random processes.IEEE r-mation Theory,vol.IT-19,no3,pp.295-315.G.B ODENSTEIN and H.M.P RAETORIUS(1977).Feature extraction from the encephalogram by adaptive segmentation.Proc.IEEE,vol.65,pp.642-652.T.B OHLIN(1977).Analysis of EEG signals with changing spectra using a short word Kalman estimator. Mathematical Biosciences,vol.35,pp.221-259.W.B¨OHM and P.H ACKL(1990).Improved bounds for the average run length of control charts based on finite weighted sums.Annals Statistics,vol.18,no4,pp.1895-1899.T.B OJDECKI and J.H OSZA(1984).On a generalized disorder problem.Stochastic Processes and their Applications,vol.18,pp.349-359.L.I.B ORODKIN and V.V.M OTTL’(1976).Algorithm forfinding the jump times of random process equation parameters.Automation and Remote Control,vol.37,no6,Part1,pp.23-32.A.A.B OROVKOV(1984).Theory of Mathematical Statistics-Estimation and Hypotheses Testing,Naouka, Moscow(in Russian).Translated in French under the title Statistique Math´e matique-Estimation et Tests d’Hypoth`e ses,Mir,Paris,1987.G.E.P.B OX and G.M.J ENKINS(1970).Time Series Analysis,Forecasting and Control.Series in Time Series Analysis,Holden-Day,San Francisco.A.VON B RANDT(1983).Detecting and estimating parameters jumps using ladder algorithms and likelihood ratio test.Proc.ICASSP,Boston,MA,pp.1017-1020.A.VON B RANDT(1984).Modellierung von Signalen mit Sprunghaft Ver¨a nderlichem Leistungsspektrum durch Adaptive Segmentierung.Doctor-Engineer Dissertation,M¨u nchen,RFA(in German).S.B RAUN,ed.(1986).Mechanical Signature Analysis-Theory and Applications.Academic Press,London. L.B REIMAN(1968).Probability.Series in Statistics,Addison-Wesley,Reading,MA.G.S.B RITOV and L.A.M IRONOVSKI(1972).Diagnostics of linear systems of automatic regulation.Tekh. Kibernetics,vol.1,pp.76-83.B.E.B RODSKIY and B.S.D ARKHOVSKIY(1992).Nonparametric Methods in Change-point Problems. Kluwer Academic,Boston.L.D.B ROEMELING(1982).Jal Econometrics,vol.19,Special issue on structural change in Econometrics. L.D.B ROEMELING and H.T SURUMI(1987).Econometrics and Structural Change.Dekker,New York. D.B ROOK and D.A.E VANS(1972).An approach to the probability distribution of Cusum run length. Biometrika,vol.59,pp.539-550.J.B RUNET,D.J AUME,M.L ABARR`E RE,A.R AULT and M.V ERG´E(1990).D´e tection et Diagnostic de Pannes.Trait´e des Nouvelles Technologies,S´e rie Diagnostic et Maintenance,Herm`e s,Paris(in French).428B IBLIOGRAPHY S.P.B RUZZONE and M.K AVEH(1984).Information tradeoffs in using the sample autocorrelation function in ARMA parameter estimation.IEEE Trans.Acoustics,Speech,Signal Processing,vol.ASSP-32,no4, pp.701-715.A.K.C AGLAYAN(1980).Necessary and sufficient conditions for detectability of jumps in linear systems. IEEE Trans.Automatic Control,vol.AC-25,no4,pp.833-834.A.K.C AGLAYAN and R.E.L ANCRAFT(1983).Reinitialization issues in fault tolerant systems.Proc.Amer-ican Control Conf.,pp.952-955.A.K.C AGLAYAN,S.M.A LLEN and K.W EHMULLER(1988).Evaluation of a second generation reconfigu-ration strategy for aircraftflight control systems subjected to actuator failure/surface damage.Proc.National Aerospace and Electronic Conference,Dayton,OH.P.E.C AINES(1988).Linear Stochastic Systems.Series in Probability and Mathematical Statistics,Wiley, New York.M.J.C HEN and J.P.N ORTON(1987).Estimation techniques for tracking rapid parameter changes.Intern. Jal Control,vol.45,no4,pp.1387-1398.W.K.C HIU(1974).The economic design of cusum charts for controlling normal mean.Applied Statistics, vol.23,no3,pp.420-433.E.Y.C HOW(1980).A Failure Detection System Design Methodology.Ph.D.Thesis,M.I.T.,L.I.D.S.,Cam-bridge,MA.E.Y.C HOW and A.S.W ILLSKY(1984).Analytical redundancy and the design of robust failure detection systems.IEEE Trans.Automatic Control,vol.AC-29,no3,pp.689-691.Y.S.C HOW,H.R OBBINS and D.S IEGMUND(1971).Great Expectations:The Theory of Optimal Stop-ping.Houghton-Mifflin,Boston.R.N.C LARK,D.C.F OSTH and V.M.W ALTON(1975).Detection of instrument malfunctions in control systems.IEEE Trans.Aerospace Electronic Systems,vol.AES-11,pp.465-473.A.C OHEN(1987).Biomedical Signal Processing-vol.1:Time and Frequency Domain Analysis;vol.2: Compression and Automatic Recognition.CRC Press,Boca Raton,FL.J.C ORGE and F.P UECH(1986).Analyse du rythme cardiaque foetal par des m´e thodes de d´e tection de ruptures.Proc.7th INRIA Int.Conf.Analysis and optimization of Systems.Antibes,FR(in French).D.R.C OX and D.V.H INKLEY(1986).Theoretical Statistics.Chapman and Hall,New York.D.R.C OX and H.D.M ILLER(1965).The Theory of Stochastic Processes.Wiley,New York.S.V.C ROWDER(1987).A simple method for studying run-length distributions of exponentially weighted moving average charts.Technometrics,vol.29,no4,pp.401-407.H.C S¨ORG¨O and L.H ORV´ATH(1988).Nonparametric methods for change point problems.In Handbook of Statistics(P.R.Krishnaiah,C.R.Rao,eds.),vol.7,Elsevier,New York,pp.403-425.R.B.D AVIES(1973).Asymptotic inference in stationary gaussian time series.Advances Applied Probability, vol.5,no3,pp.469-497.J.C.D ECKERT,M.N.D ESAI,J.J.D EYST and A.S.W ILLSKY(1977).F-8DFBW sensor failure identification using analytical redundancy.IEEE Trans.Automatic Control,vol.AC-22,no5,pp.795-803.M.H.D E G ROOT(1970).Optimal Statistical Decisions.Series in Probability and Statistics,McGraw-Hill, New York.J.D ESHAYES and D.P ICARD(1979).Tests de ruptures dans un mod`e pte-Rendus de l’Acad´e mie des Sciences,vol.288,Ser.A,pp.563-566(in French).B IBLIOGRAPHY429 J.D ESHAYES and D.P ICARD(1983).Ruptures de Mod`e les en Statistique.Th`e ses d’Etat,Universit´e deParis-Sud,Orsay,France(in French).J.D ESHAYES and D.P ICARD(1986).Off-line statistical analysis of change-point models using non para-metric and likelihood methods.In Detection of Abrupt Changes in Signals and Dynamical Systems(M. Basseville,A.Benveniste,eds.).Lecture Notes in Control and Information Sciences,LNCIS77,Springer, New York,pp.103-168.B.D EVAUCHELLE-G ACH(1991).Diagnostic M´e canique des Fatigues sur les Structures Soumises`a des Vibrations en Ambiance de Travail.Th`e se de l’Universit´e Paris IX Dauphine(in French).B.D EVAUCHELLE-G ACH,M.B ASSEVILLE and A.B ENVENISTE(1991).Diagnosing mechanical changes in vibrating systems.Proc.SAFEPROCESS’91,Baden-Baden,FRG,pp.85-89.R.D I F RANCESCO(1990).Real-time speech segmentation using pitch and convexity jump models:applica-tion to variable rate speech coding.IEEE Trans.Acoustics,Speech,Signal Processing,vol.ASSP-38,no5, pp.741-748.X.D ING and P.M.F RANK(1990).Fault detection via factorization approach.Systems and Control Letters, vol.14,pp.431-436.J.L.D OOB(1953).Stochastic Processes.Wiley,New York.V.D RAGALIN(1988).Asymptotic solutions in detecting a change in distribution under an unknown param-eter.Statistical Problems of Control,Issue83,Vilnius,pp.45-52.B.D UBUISSON(1990).Diagnostic et Reconnaissance des Formes.Trait´e des Nouvelles Technologies,S´e rie Diagnostic et Maintenance,Herm`e s,Paris(in French).A.J.D UNCAN(1986).Quality Control and Industrial Statistics,5th edition.Richard D.Irwin,Inc.,Home-wood,IL.J.D URBIN(1971).Boundary-crossing probabilities for the Brownian motion and Poisson processes and techniques for computing the power of the Kolmogorov-Smirnov test.Jal Applied Probability,vol.8,pp.431-453.J.D URBIN(1985).Thefirst passage density of the crossing of a continuous Gaussian process to a general boundary.Jal Applied Probability,vol.22,no1,pp.99-122.A.E MAMI-N AEINI,M.M.A KHTER and S.M.R OCK(1988).Effect of model uncertainty on failure detec-tion:the threshold selector.IEEE Trans.Automatic Control,vol.AC-33,no12,pp.1106-1115.J.D.E SARY,F.P ROSCHAN and D.W.W ALKUP(1967).Association of random variables with applications. Annals Mathematical Statistics,vol.38,pp.1466-1474.W.D.E WAN and K.W.K EMP(1960).Sampling inspection of continuous processes with no autocorrelation between successive results.Biometrika,vol.47,pp.263-280.G.F AVIER and A.S MOLDERS(1984).Adaptive smoother-predictors for tracking maneuvering targets.Proc. 23rd Conf.Decision and Control,Las Vegas,NV,pp.831-836.W.F ELLER(1966).An Introduction to Probability Theory and Its Applications,vol.2.Series in Probability and Mathematical Statistics,Wiley,New York.R.A.F ISHER(1925).Theory of statistical estimation.Proc.Cambridge Philosophical Society,vol.22, pp.700-725.M.F ISHMAN(1988).Optimization of the algorithm for the detection of a disorder,based on the statistic of exponential smoothing.In Statistical Problems of Control,Issue83,Vilnius,pp.146-151.R.F LETCHER(1980).Practical Methods of Optimization,2volumes.Wiley,New York.P.M.F RANK(1990).Fault diagnosis in dynamic systems using analytical and knowledge based redundancy -A survey and new results.Automatica,vol.26,pp.459-474.430B IBLIOGRAPHY P.M.F RANK(1991).Enhancement of robustness in observer-based fault detection.Proc.SAFEPRO-CESS’91,Baden-Baden,FRG,pp.275-287.P.M.F RANK and J.W¨UNNENBERG(1989).Robust fault diagnosis using unknown input observer schemes. In Fault Diagnosis in Dynamic Systems-Theory and Application(R.Patton,P.Frank,R.Clark,eds.). International Series in Systems and Control Engineering,Prentice Hall International,London,UK,pp.47-98.K.F UKUNAGA(1990).Introduction to Statistical Pattern Recognition,2d ed.Academic Press,New York. S.I.G ASS(1958).Linear Programming:Methods and Applications.McGraw Hill,New York.W.G E and C.Z.F ANG(1989).Extended robust observation approach for failure isolation.Int.Jal Control, vol.49,no5,pp.1537-1553.W.G ERSCH(1986).Two applications of parametric time series modeling methods.In Mechanical Signature Analysis-Theory and Applications(S.Braun,ed.),chap.10.Academic Press,London.J.J.G ERTLER(1988).Survey of model-based failure detection and isolation in complex plants.IEEE Control Systems Magazine,vol.8,no6,pp.3-11.J.J.G ERTLER(1991).Analytical redundancy methods in fault detection and isolation.Proc.SAFEPRO-CESS’91,Baden-Baden,FRG,pp.9-22.B.K.G HOSH(1970).Sequential Tests of Statistical Hypotheses.Addison-Wesley,Cambridge,MA.I.N.G IBRA(1975).Recent developments in control charts techniques.Jal Quality Technology,vol.7, pp.183-192.J.P.G ILMORE and R.A.M C K ERN(1972).A redundant strapdown inertial reference unit(SIRU).Jal Space-craft,vol.9,pp.39-47.M.A.G IRSHICK and H.R UBIN(1952).A Bayes approach to a quality control model.Annals Mathematical Statistics,vol.23,pp.114-125.A.L.G OEL and S.M.W U(1971).Determination of the ARL and a contour nomogram for CUSUM charts to control normal mean.Technometrics,vol.13,no2,pp.221-230.P.L.G OLDSMITH and H.W HITFIELD(1961).Average run lengths in cumulative chart quality control schemes.Technometrics,vol.3,pp.11-20.G.C.G OODWIN and K.S.S IN(1984).Adaptive Filtering,Prediction and rmation and System Sciences Series,Prentice Hall,Englewood Cliffs,NJ.R.M.G RAY and L.D.D AVISSON(1986).Random Processes:a Mathematical Approach for Engineers. Information and System Sciences Series,Prentice Hall,Englewood Cliffs,NJ.C.G UEGUEN and L.L.S CHARF(1980).Exact maximum likelihood identification for ARMA models:a signal processing perspective.Proc.1st EUSIPCO,Lausanne.D.E.G USTAFSON, A.S.W ILLSKY,J.Y.W ANG,M.C.L ANCASTER and J.H.T RIEBWASSER(1978). ECG/VCG rhythm diagnosis using statistical signal analysis.Part I:Identification of persistent rhythms. Part II:Identification of transient rhythms.IEEE Trans.Biomedical Engineering,vol.BME-25,pp.344-353 and353-361.F.G USTAFSSON(1991).Optimal segmentation of linear regression parameters.Proc.IFAC/IFORS Symp. Identification and System Parameter Estimation,Budapest,pp.225-229.T.H¨AGGLUND(1983).New Estimation Techniques for Adaptive Control.Ph.D.Thesis,Lund Institute of Technology,Lund,Sweden.T.H¨AGGLUND(1984).Adaptive control of systems subject to large parameter changes.Proc.IFAC9th World Congress,Budapest.B IBLIOGRAPHY431 P.H ALL and C.C.H EYDE(1980).Martingale Limit Theory and its Application.Probability and Mathemat-ical Statistics,a Series of Monographs and Textbooks,Academic Press,New York.W.J.H ALL,R.A.W IJSMAN and J.K.G HOSH(1965).The relationship between sufficiency and invariance with applications in sequential analysis.Ann.Math.Statist.,vol.36,pp.576-614.E.J.H ANNAN and M.D EISTLER(1988).The Statistical Theory of Linear Systems.Series in Probability and Mathematical Statistics,Wiley,New York.J.D.H EALY(1987).A note on multivariate CuSum procedures.Technometrics,vol.29,pp.402-412.D.M.H IMMELBLAU(1970).Process Analysis by Statistical Methods.Wiley,New York.D.M.H IMMELBLAU(1978).Fault Detection and Diagnosis in Chemical and Petrochemical Processes. Chemical Engineering Monographs,vol.8,Elsevier,Amsterdam.W.G.S.H INES(1976a).A simple monitor of a system with sudden parameter changes.IEEE r-mation Theory,vol.IT-22,no2,pp.210-216.W.G.S.H INES(1976b).Improving a simple monitor of a system with sudden parameter changes.IEEE rmation Theory,vol.IT-22,no4,pp.496-499.D.V.H INKLEY(1969).Inference about the intersection in two-phase regression.Biometrika,vol.56,no3, pp.495-504.D.V.H INKLEY(1970).Inference about the change point in a sequence of random variables.Biometrika, vol.57,no1,pp.1-17.D.V.H INKLEY(1971).Inference about the change point from cumulative sum-tests.Biometrika,vol.58, no3,pp.509-523.D.V.H INKLEY(1971).Inference in two-phase regression.Jal American Statistical Association,vol.66, no336,pp.736-743.J.R.H UDDLE(1983).Inertial navigation system error-model considerations in Kalmanfiltering applica-tions.In Control and Dynamic Systems(C.T.Leondes,ed.),Academic Press,New York,pp.293-339.J.S.H UNTER(1986).The exponentially weighted moving average.Jal Quality Technology,vol.18,pp.203-210.I.A.I BRAGIMOV and R.Z.K HASMINSKII(1981).Statistical Estimation-Asymptotic Theory.Applications of Mathematics Series,vol.16.Springer,New York.R.I SERMANN(1984).Process fault detection based on modeling and estimation methods-A survey.Auto-matica,vol.20,pp.387-404.N.I SHII,A.I WATA and N.S UZUMURA(1979).Segmentation of nonstationary time series.Int.Jal Systems Sciences,vol.10,pp.883-894.J.E.J ACKSON and R.A.B RADLEY(1961).Sequential and tests.Annals Mathematical Statistics, vol.32,pp.1063-1077.B.J AMES,K.L.J AMES and D.S IEGMUND(1988).Conditional boundary crossing probabilities with appli-cations to change-point problems.Annals Probability,vol.16,pp.825-839.M.K.J EERAGE(1990).Reliability analysis of fault-tolerant IMU architectures with redundant inertial sen-sors.IEEE Trans.Aerospace and Electronic Systems,vol.AES-5,no.7,pp.23-27.N.L.J OHNSON(1961).A simple theoretical approach to cumulative sum control charts.Jal American Sta-tistical Association,vol.56,pp.835-840.N.L.J OHNSON and F.C.L EONE(1962).Cumulative sum control charts:mathematical principles applied to their construction and use.Parts I,II,III.Industrial Quality Control,vol.18,pp.15-21;vol.19,pp.29-36; vol.20,pp.22-28.432B IBLIOGRAPHY R.A.J OHNSON and M.B AGSHAW(1974).The effect of serial correlation on the performance of CUSUM tests-Part I.Technometrics,vol.16,no.1,pp.103-112.H.L.J ONES(1973).Failure Detection in Linear Systems.Ph.D.Thesis,Dept.Aeronautics and Astronautics, MIT,Cambridge,MA.R.H.J ONES,D.H.C ROWELL and L.E.K APUNIAI(1970).Change detection model for serially correlated multivariate data.Biometrics,vol.26,no2,pp.269-280.M.J URGUTIS(1984).Comparison of the statistical properties of the estimates of the change times in an autoregressive process.In Statistical Problems of Control,Issue65,Vilnius,pp.234-243(in Russian).T.K AILATH(1980).Linear rmation and System Sciences Series,Prentice Hall,Englewood Cliffs,NJ.L.V.K ANTOROVICH and V.I.K RILOV(1958).Approximate Methods of Higher Analysis.Interscience,New York.S.K ARLIN and H.M.T AYLOR(1975).A First Course in Stochastic Processes,2d ed.Academic Press,New York.S.K ARLIN and H.M.T AYLOR(1981).A Second Course in Stochastic Processes.Academic Press,New York.D.K AZAKOS and P.P APANTONI-K AZAKOS(1980).Spectral distance measures between gaussian pro-cesses.IEEE Trans.Automatic Control,vol.AC-25,no5,pp.950-959.K.W.K EMP(1958).Formula for calculating the operating characteristic and average sample number of some sequential tests.Jal Royal Statistical Society,vol.B-20,no2,pp.379-386.K.W.K EMP(1961).The average run length of the cumulative sum chart when a V-mask is used.Jal Royal Statistical Society,vol.B-23,pp.149-153.K.W.K EMP(1967a).Formal expressions which can be used for the determination of operating character-istics and average sample number of a simple sequential test.Jal Royal Statistical Society,vol.B-29,no2, pp.248-262.K.W.K EMP(1967b).A simple procedure for determining upper and lower limits for the average sample run length of a cumulative sum scheme.Jal Royal Statistical Society,vol.B-29,no2,pp.263-265.D.P.K ENNEDY(1976).Some martingales related to cumulative sum tests and single server queues.Stochas-tic Processes and Appl.,vol.4,pp.261-269.T.H.K ERR(1980).Statistical analysis of two-ellipsoid overlap test for real time failure detection.IEEE Trans.Automatic Control,vol.AC-25,no4,pp.762-772.T.H.K ERR(1982).False alarm and correct detection probabilities over a time interval for restricted classes of failure detection algorithms.IEEE rmation Theory,vol.IT-24,pp.619-631.T.H.K ERR(1987).Decentralizedfiltering and redundancy management for multisensor navigation.IEEE Trans.Aerospace and Electronic systems,vol.AES-23,pp.83-119.Minor corrections on p.412and p.599 (May and July issues,respectively).R.A.K HAN(1978).Wald’s approximations to the average run length in cusum procedures.Jal Statistical Planning and Inference,vol.2,no1,pp.63-77.R.A.K HAN(1979).Somefirst passage problems related to cusum procedures.Stochastic Processes and Applications,vol.9,no2,pp.207-215.R.A.K HAN(1981).A note on Page’s two-sided cumulative sum procedures.Biometrika,vol.68,no3, pp.717-719.B IBLIOGRAPHY433 V.K IREICHIKOV,V.M ANGUSHEV and I.N IKIFOROV(1990).Investigation and application of CUSUM algorithms to monitoring of sensors.In Statistical Problems of Control,Issue89,Vilnius,pp.124-130(in Russian).G.K ITAGAWA and W.G ERSCH(1985).A smoothness prior time-varying AR coefficient modeling of non-stationary covariance time series.IEEE Trans.Automatic Control,vol.AC-30,no1,pp.48-56.N.K LIGIENE(1980).Probabilities of deviations of the change point estimate in statistical models.In Sta-tistical Problems of Control,Issue83,Vilnius,pp.80-86(in Russian).N.K LIGIENE and L.T ELKSNYS(1983).Methods of detecting instants of change of random process prop-erties.Automation and Remote Control,vol.44,no10,Part II,pp.1241-1283.J.K ORN,S.W.G ULLY and A.S.W ILLSKY(1982).Application of the generalized likelihood ratio algorithm to maneuver detection and estimation.Proc.American Control Conf.,Arlington,V A,pp.792-798.P.R.K RISHNAIAH and B.Q.M IAO(1988).Review about estimation of change points.In Handbook of Statistics(P.R.Krishnaiah,C.R.Rao,eds.),vol.7,Elsevier,New York,pp.375-402.P.K UDVA,N.V ISWANADHAM and A.R AMAKRISHNAN(1980).Observers for linear systems with unknown inputs.IEEE Trans.Automatic Control,vol.AC-25,no1,pp.113-115.S.K ULLBACK(1959).Information Theory and Statistics.Wiley,New York(also Dover,New York,1968). K.K UMAMARU,S.S AGARA and T.S¨ODERSTR¨OM(1989).Some statistical methods for fault diagnosis for dynamical systems.In Fault Diagnosis in Dynamic Systems-Theory and Application(R.Patton,P.Frank,R. Clark,eds.).International Series in Systems and Control Engineering,Prentice Hall International,London, UK,pp.439-476.A.K USHNIR,I.N IKIFOROV and I.S AVIN(1983).Statistical adaptive algorithms for automatic detection of seismic signals-Part I:One-dimensional case.In Earthquake Prediction and the Study of the Earth Structure,Naouka,Moscow(Computational Seismology,vol.15),pp.154-159(in Russian).L.L ADELLI(1990).Diffusion approximation for a pseudo-likelihood test process with application to de-tection of change in stochastic system.Stochastics and Stochastics Reports,vol.32,pp.1-25.T.L.L A¨I(1974).Control charts based on weighted sums.Annals Statistics,vol.2,no1,pp.134-147.T.L.L A¨I(1981).Asymptotic optimality of invariant sequential probability ratio tests.Annals Statistics, vol.9,no2,pp.318-333.D.G.L AINIOTIS(1971).Joint detection,estimation,and system identifirmation and Control, vol.19,pp.75-92.M.R.L EADBETTER,G.L INDGREN and H.R OOTZEN(1983).Extremes and Related Properties of Random Sequences and Processes.Series in Statistics,Springer,New York.L.L E C AM(1960).Locally asymptotically normal families of distributions.Univ.California Publications in Statistics,vol.3,pp.37-98.L.L E C AM(1986).Asymptotic Methods in Statistical Decision Theory.Series in Statistics,Springer,New York.E.L.L EHMANN(1986).Testing Statistical Hypotheses,2d ed.Wiley,New York.J.P.L EHOCZKY(1977).Formulas for stopped diffusion processes with stopping times based on the maxi-mum.Annals Probability,vol.5,no4,pp.601-607.H.R.L ERCHE(1980).Boundary Crossing of Brownian Motion.Lecture Notes in Statistics,vol.40,Springer, New York.L.L JUNG(1987).System Identification-Theory for the rmation and System Sciences Series, Prentice Hall,Englewood Cliffs,NJ.。
acf和adf代码

ACF and ADF CodesIntroductionIn time series analysis, two commonly used models for forecasting are the autoregressive conditional heteroskedasticity (ARCH) model and the autoregressive integrated moving average (ARIMA) model. These models are widely used in finance, economics, and other fields to predict future values of a time series based on its past behavior. In this article, we will explore the ACF (Autocorrelation Function) and ADF (Augmented Dickey-Fuller) codes, which are essential tools for analyzing and diagnosing time series data.Autocorrelation Function (ACF)The ACF measures the correlation between a time series and its lagged values. It helps us understand the relationship between the current value of a series and its past values at different lags. The ACF is a crucial tool for identifying patterns, seasonality, and dependencies in time series data.The ACF code calculates the correlation coefficient for each lag and plots it against the lag. The code snippet below demonstrates how to calculate and plot the ACF for a given time series using Python:import pandas as pdimport matplotlib.pyplot as pltfrom statsmodels.graphics.tsaplots import plot_acf# Load the time series datadata = pd.read_csv('time_series_data.csv')# Calculate and plot the ACFplot_acf(data, lags=20)plt.show()In the code above, we first load the time series data from a CSV file using the pandas library. Then, we use the plot_acf function from the statsmodels.graphics.tsaplots module to calculate and plot the ACF. The lags parameter specifies the number of lags to include in the plot. Finally, we use plt.show() to display the plot.The ACF plot helps us identify significant lags in the time series data. If a lag has a correlation coefficient close to 1 or -1, it indicates a strong positive or negative correlation, respectively. On the other hand, if the correlation coefficient is close to 0, it suggests no correlation.Augmented Dickey-Fuller (ADF) TestThe ADF test is a statistical test used to determine if a time series is stationary or not. Stationarity is a key assumption in many time series models, including ARIMA. A stationary time series has constant mean and variance over time, and its properties do not depend on the specifictime period.The ADF code allows us to perform the ADF test on a given time series to check for stationarity. The following code snippet demonstrates how to perform the ADF test using Python:import pandas as pdfrom statsmodels.tsa.stattools import adfuller# Load the time series datadata = pd.read_csv('time_series_data.csv')# Perform the ADF testresult = adfuller(data)# Extract and print the test statisticsprint('ADF Statistic:', result[0])print('p-value:', result[1])print('Critical Values:', result[4])In the code above, we first load the time series data from a CSV file using the pandas library. Then, we use the adfuller function from the statsmodels.tsa.stattools module to perform the ADF test. The function returns a tuple containing the test statistics, p-value, and critical values.The ADF Statistic is the test statistic of the ADF test. The p-value represents the probability that the null hypothesis of non-stationarity is true. If the p-value is less than a chosen significance level (e.g., 0.05), we can reject the null hypothesis and conclude that the time series is stationary. The Critical Values represent the threshold values for different significance levels.ConclusionThe ACF and ADF codes are essential tools for analyzing and diagnosing time series data. The ACF helps us understand the correlation between a time series and its lagged values, while the ADF test allows us to check for stationarity. By using these codes, we can gain insights into the patterns, dependencies, and stationarity of time series data, which are crucial for accurate forecasting and decision-making in various fields.。
计量经济学Test-bank-questions-Chapter-5
Multiple Choice Test Bank Questions No Feedback – Chapter 5 Correct answers denoted by an asterisk.1. Consider the following model estimated for a time seriesy t = 0.3 + 0.5 y t-1 - 0.4εt-1 +εtwhere εt is a zero mean error process.What is the (unconditional) mean of the series, y t ?(a) * 0.6(b) 0.3(c) 0.0(d) 0.42. Consider the following single exponential smoothing model:S t = α X t + (1-α) S t-1You are given the following data:αˆ=0.1, X t=0.5,S t-1=0.2If we believe that the true DGP can be approximated by the exponential smoothing model, what would be an appropriate 2-step ahead forecast for X? (i.e. a forecast of X t+2 made at time t)(a) 0.2(b) * 0.23(c) 0.5(d) There is insufficient information given in the question to form more than a one step ahead forecast.3. Consider the following MA(3) process.y t = 0.1 + 0.4u t-1 + 0.2u t-2– 0.1u t-3 + u tWhat is the optimal forecast for y t, 3 steps into the future (i.e. for time t+2 if all information until time t-1 is available), if you have the following data?u t-1 = 0.3; u t-2 = -0.6; u t-3 = -0.3(a)0.4(b)0.0(c)* 0.07(d)–0.14. Which of the following sets of characteristics would usually best describe an autoregressive process of order 3 (i.e. an AR(3))?(a) * A slowly decaying acf, and a pacf with 3 significant spikes(b) A slowly decaying pacf and an acf with 3 significant spikes(c) A slowly decaying acf and pacf(d) An acf and a pacf with 3 significant spikes5. A process, x t, which has a constant mean and variance, and zero autocovariance for all non-zero lags is best described as(a) * A white noise process(b) A covariance stationary process(c) An autocorrelated process(d) A moving average process6. Which of the following conditions must hold for the autoregressive part of an ARMA model to be stationary?(a) * All roots of the characteristic equation must lie outside the unit circle(b) All roots of the characteristic equation must lie inside the unit circle(c) All roots must be smaller than unity(d) At least one of the roots must be bigger than one in absolute value.7. Which of the following statements are true concerning time-series forecasting?(i) All time-series forecasting methods are essentially extrapolative.(ii) Forecasting models are prone to perform poorly following a structural break in a series.(iii) Forecasting accuracy often declines with prediction horizon.(iv) The mean squared errors of forecasts are usually very highly correlated with the profitability of employing those forecasts in a trading strategy.(a) (i), (ii), (iii), and (iv)(b) * (i), (ii) and (iii) only(c) (ii), (iii) only(d) (ii) and (iv) only8. If a series, y t, follows a random walk (with no drift), what is the optimal 1-step ahead forecast for y?(a)* The current value of y.(b)Zero.(c)The historical unweighted average of y.(d)An exponentially weighted average of previous values of y.9. Consider a series that follows an MA(1) with zero mean and a moving average coefficient of 0.4. What is the value of the autocorrelation function at lag 1?(a)0.4(b)1(c)*0.34(d)It is not possible to determine the value of the autocovariances without knowing thedisturbance variance.10. Which of the following statements are true?(i)An MA(q) can be expressed as an AR(infinity) if it is invertible(ii)An AR(p) can be written as an MA(infinity) if it is stationary(iii)The (unconditional) mean of an ARMA process will depend only on the intercept and on the AR coefficients and not on the MA coefficients(iv) A random walk series will have zero pacf except at lag 1(a)(ii) and (iv) only(b)(i) and (iii) only(c)(i), (ii), and (iii) only(d)* (i), (ii), (iii), and (iv).11. Consider the following picture and suggest the model from the following list that best characterises the process:(a)An AR(1)(b)An AR(2)(c)* An ARMA(1,1)(d)An MA(3)The acf is clearly declining very slowly in this case, which is consistent with their being an autoregressive part to the appropriate model. The pacf is clearly significant for lags one and two, but the question is does it them become insignificant for lags 2 and 4,indicating an AR(2) process, or does it remain significant, which would be more consistent with a mixed ARMA process? Well, given the huge size of the sample that gave rise to this acf and pacf, even a pacf value of 0.001 would still be statistically significant. Thus an ARMA process is the most likely candidate, although note that it would not be possible to tell from the acf and pacf which model from the ARMA family was more appropriate. The DGP for the data that generated this plot was y_t = 0.9 y_(t-1) – 0.3 u_(t-1) + u_t.12. Which of the following models can be estimated using ordinary least squares?(i)An AR(1)(ii) An ARMA(2,0)(iii) An MA(1)(iv) An ARMA(1,1)(a)(i) only(b)* (i) and (ii) only(c)(i), (ii), and (iii) only(d)(i), (ii), (iii), and (iv).13. If a series, y, is described as “mea n-reverting”, which model from the following list is likely to produce the best long-term forecasts for that series y?(a)A random walk(b)* The long term mean of the series(c)A model from the ARMA family(d)A random walk with drift14. Consider the following AR(2) model. What is the optimal 2-step ahead forecast for y if all information available is up to and including time t, if the values of y at time t, t-1 and t-2 are –0.3, 0.4 and –0.1 respectively, and the value of u at time t-1 is 0.3?y t = -0.1 + 0.75y t-1 - 0.125y t-2 + u t(a)-0.1(b)0.27(c)* -0.34(d)0.3015. What is the optimal three-step ahead forecast from the AR(2) model given in question 14?(a)-0.1(b)0.27(c)-0.34(d)* -0.3116. Suppose you had to guess at the most likely value of a one hundred step-ahead forecast for the AR(2) model given in question 14 – what would your forecast be?(a)-0.1(b)0.7(c)* –0.27(d)0.75。
Models for Time Series and ForecastingPPT
to accompany
Introduction to Business Statistics
fourth edition, by Ronald M. Weiers
Presentation by Priscilla Chaffe-Stengel
© 2002 The Wadsworth Group
? = b0 + b1x y • Linear: ? = b + b x + b x2 • Quadratic: y 0 1 2
Trend Equations
? = the trend line estimate of y y x = time period b0, b1, and b2 are coefficients that are selected to minimize the deviations between the ? and the actual data values trend estimates y y for the past time periods. Regression methods are used to determine the best values for the coefficients.
• Trend equation • Moving average • Exponential smoothing
ቤተ መጻሕፍቲ ባይዱ
• Seasonal index • Ratio to moving average method • Deseasonalizing • MAD criterion • MSE criterion • Constructing an index using the CPI • Shifting the base of an index
Principles of Marketing Engineering - Ch5 - Forecasting
A study by Van den Bulte and Stremersch (2004) suggests an average value of 0.03 for p and an average value of 0.42 for q, The average was taken across a couple of hundred categories.
© CUMT2011 Principles Chapter 6: Forecasting - 14
6 巴斯模型的参数估计方法
(1)以历史销售数据(Past /Historical
Data)为依据校准模型
线性回归 非线性最小二乘法
(2)以主观判断为依据校准模型
相似产品进行类比(Analogy) 市场调查
N (t ) n(t ) [ N N (t )] p q N N(t) : Cumulative number of adopters until time t.
© CUMT2011 Principles Chapter 6: Forecasting - 9
3 巴斯(Bass)模型参数
This chapter focuses mainly on new
product forecasting
Bass diffusion model(巴斯扩散模型)
© CUMT2011 Principles Chapter 6: Forecasting - 4
第二节 新产品预测的Bass 模型
© CUMT2011 Principles Chapter 6: Forecasting - 16
6 Generalized Bass Model
【免费下载】Wiley Series in Probability and Statistics
Wiley Series in Probability and Statistics1. A Course in Time Series Analysis2. A Primer on Experiments with Mixtures3. A Probabilistic Analysis of the Sacco and Vanzetti Evidence4. A User's Guide to Principal Components5. A Weak Convergence Approach to the Theory of Large Deviations6.Accelerated Testing: Statistical Models, Test Plans, and Data Analysis7.Advanced Calculus with Applications in Statistics, Second Edition8.Alternative Methods of Regression9.An Elementary Introduction to Statistical Learning Theory10.An Introduction to Categorical Data Analysis, Second Edition11.An Introduction to Probability and Statistics, Second Edition12.An Introduction to Regression Graphics13.Analysis of Financial Time Series14.Analysis of Financial Time Series, Second Edition15.Analysis of Financial Time Series, Third Edition, Third Edition16.Analysis of Ordinal Categorical Data, Second Edition17.Analyzing Microarray Gene Expression Data18.Applications of Statistics to Industrial Experimentation19.Applied Bayesian Modeling and Causal Inference from Incomplete-DataPerspectives: An Essential Journey with Donald Rubin's Statistical Family20.Applied Bayesian Modelling21.Applied Life Data Analysis22.Applied Linear Regression, Third Edition23.Applied MANOVA and Discriminant Analysis, Second Edition24.Applied Multiway Data Analysis25.Applied Regression Including Computing and Graphics26.Applied Spatial Statistics for Public Health Data27.Applied Survival Analysis: Regression Modeling of Time-to-Event Data, SecondEdition28.Approximate Dynamic Programming: Solving the Curses of Dimensionality29.Approximate Dynamic Programming: Solving the Curses of Dimensionality, SecondEdition30.Approximation Theorems of Mathematical Statistics31.Aspects of Multivariate Statistical Theory32.Aspects of Statistical Inference33.Batch Effects and Noise in Microarray Experiments: Sources and Solutions34.Bayes Linear Statistics: Theory and Methods35.Bayesian Analysis for the Social Sciences36.Bayesian Analysis of Stochastic Process Models37.Bayesian Methods and Ethics in a Clinical Trial Design38.Bayesian Models for Categorical Data39.Bayesian Networks: An Introduction40.Bayesian Statistical Modelling, Second Edition41.Bayesian Statistics and Marketing42.Bayesian Theory43.Bias and Causation44.Biostatistical Methods in Epidemiology45.Biostatistical Methods: The Assessment of Relative Risks46.Biostatistical Methods: The Assessment of Relative Risks, Second Edition47.Biostatistics: A Methodology for the Health Sciences, Second Edition48.Bootstrap Methods: A Guide for Practitioners and Researchers, Second Edition49.Business Survey Methods50.Case Studies in Reliability and Maintenance51.Categorical Data Analysis, Second Edition52.Causality: Statistical Perspectives and Applications53.Clinical Trial Design: Bayesian and Frequentist Adaptive Methods54.Clinical Trials: A Methodologic Perspective, Second Edition55.Cluster Analysis, 5th Editionbinatorial Methods in Discrete Distributionsparative Statistical Inference, Third Edition58.Constrained Statistical Inference: Inequality, Order, and Shape Restrictions59.Contemporary Bayesian Econometrics and Statistics60.Continuous Multivariate Distributions: Models and Applications, Volume 1,Second Edition61.Convergence of Probability Measures, Second Edition62.Counting Processes and Survival Analysis63.Decision Theory64.Design and Analysis of Clinical Trials: Concepts and Methodologies, SecondEdition65.Design and Analysis of Experiments: Advanced Experimental Design, Volume 266.Design and Analysis of Experiments: Introduction to Experimental Design, Volume1, Second Edition67.Design and Analysis of Experiments: Special Designs and Applications, Volume 368.Directional Statistics69.Dirichlet and Related Distributions: Theory, Methods and Applications70.Discrete Distributions: Applications in the Health Sciences71.Discriminant Analysis and Statistical Pattern Recognition72.Empirical Model Building73.Empirical Model Building: Data, Models, and Reality, Second Edition74.Environmental Statistics: Methods and Applications75.Exploration and Analysis of DNA Microarray and Protein Array Data76.Exploratory Data Mining and Data Cleaning77.Exploring Data Tables, Trends, and Shapes78.Financial Derivatives in Theory and Practice79.Finding Groups in Data: An Introduction to Cluster Analysis80.Finite Mixture Models81.Flowgraph Models for Multistate Time-to-Event Data82.Forecasting with Dynamic Regression Models83.Forecasting with Univariate Box-Jenkins Models: Concepts and Cases84.Fourier Analysis of Time Series: An Introduction, Second Edition85.Fractal-Based Point Processes86.Fractional Factorial Plans87.Fundamentals of Exploratory Analysis of Variance88.Generalized Least Squares89.Generalized Linear Models: With Applications in Engineering and the Sciences,Second Edition90.Generalized, Linear, and Mixed Models91.Geometrical Foundations of Asymptotic Inference92.Geostatistics: Modeling Spatial Uncertainty93.Geostatistics: Modeling Spatial Uncertainty, Second Edition94.High-Dimensional Covariance Estimation95.Hilbert Space Methods in Probability and Statistical Inference96.History of Probability and Statistics and Their Applications before 175097.Image Processing and Jump Regression Analysis98.Inference and Prediction in Large Dimensions99.Introduction to Nonparametric Regression100.Introduction to Statistical Time Series, Second Edition101.Introductory Stochastic Analysis for Finance and Insurancetent Class and Latent Transition Analysis: With Applications in the Social, Behavioral, and Health Sciencestent Curve Models: A Structural Equation Perspectivetent Variable Models and Factor Analysis: A Unified Approach, 3rd Edition105.Leading Personalities in Statistical Sciences: From the Seventeenth Century to the Present106.Lévy Processes in Finance: Pricing Financial Derivatives107.Linear Models: The Theory and Application of Analysis of Variance108.Linear Statistical Inference and its Applications: Second Editon109.Linear Statistical Models110.LISP-STAT: An Object-Oriented Environment for Statistical Computing and Dynamic Graphics111.Longitudinal Data Analysis112.Long-Memory Time Series: Theory and Methods113.Loss Distributions114.Loss Models: From Data to Decisions, Third Edition115.Management of Data in Clinical Trials, Second Edition116.Markov Decision Processes: Discrete Stochastic Dynamic Programming117.Markov Processes and Applications118.Markov Processes: Characterization and Convergence119.Mathematics of Chance120.Measurement Error Models121.Measurement Errors in Surveys122.Meta Analysis: A Guide to Calibrating and Combining Statistical Evidence 123.Methods and Applications of Linear Models: Regression and the Analysis of Variance124.Methods for Statistical Data Analysis of Multivariate Observations, Second Edition125.Methods of Multivariate Analysis, Second Edition126.Methods of Multivariate Analysis, Third Edition127.Mixed Models: Theory and Applications128.Mixtures: Estimation and Applications129.Modelling Under Risk and Uncertainty: An Introduction to Statistical, Phenomenological and Computational Methods130.Models for Investors in Real World Markets131.Models for Probability and Statistical Inference: Theory and Applications 132.Modern Applied U-Statistics133.Modern Experimental Design134.Modes of Parametric Statistical Inference135.Multilevel Statistical Models, 4th Edition136.Multiple Comparison Procedures137.Multiple Imputation for Nonresponse in Surveys138.Multiple Time Series139.Multistate Systems Reliability Theory with Applications140.Multivariable Model-Building: A pragmatic approach to regression analysis based on fractional polynomials for modelling continuous variables141.Multivariate Density Estimation: Theory, Practice, and Visualization142.Multivariate Observations143.Multivariate Statistics: High-Dimensional and Large-Sample Approximations 144.Nonlinear Regression145.Nonlinear Regression Analysis and Its Applications146.Nonlinear Statistical Models147.Nonparametric Analysis of Univariate Heavy-Tailed Data: Research and Practice148.Nonparametric Regression Methods for Longitudinal Data Analysis: Mixed-Effects Modeling Approaches149.Nonparametric Statistics with Applications to Science and Engineering 150.Numerical Issues in Statistical Computing for the Social Scientist151.Operational Risk: Modeling Analytics152.Optimal Learning153.Order Statistics, Third Edition154.Periodically Correlated Random Sequences: Spectral Theory and Practice 155.Permutation Tests for Complex Data: Theory, Applications and Software 156.Planning and Analysis of Observational Studies157.Planning, Construction, and Statistical Analysis of Comparative Experiments158.Precedence-Type Tests and Applications159.Preparing for the Worst: Incorporating Downside Risk in Stock Market Investments160.Probability and Finance: It's Only a Game!161.Probability: A Survey of the Mathematical Theory, Second Edition162.Quantitative Methods in Population Health: Extensions of Ordinary Regression163.Random Data: Analysis and Measurement Procedures, Fourth Edition164.Random Graphs for Statistical Pattern Recognition165.Randomization in Clinical Trials: Theory and Practice166.Recent Advances in Quantitative Methods in Cancer and Human Health Risk Assessment167.Records168.Regression Analysis by Example, Fourth Edition169.Regression Diagnostics: Identifying Influential Data and Sources of Collinearity170.Regression Graphics: Ideas for Studying Regressions Through Graphics171.Regression Models for Time Series Analysis172.Regression with Social Data: Modeling Continuous and Limited Response Variables173.Reliability and Risk: A Bayesian Perspective174.Reliability: Modeling, Prediction, and Optimization175.Response Surfaces, Mixtures, and Ridge Analyses, Second Edition176.Robust Estimation & Testing177.Robust Methods in Biostatistics178.Robust Regression and Outlier Detection179.Robust Statistics180.Robust Statistics, Second Edition181.Robust Statistics: Theory and Methods182.Runs and Scans with Applications183.Sampling, Third Edition184.Sensitivity Analysis in Linear Regression185.Sequential Estimation186.Shape & Shape Theory187.Simulation and the Monte Carlo Method188.Simulation and the Monte Carlo Method, Second Edition189.Simulation and the Monte Carlo Method: Solutions Manual to Accompany, Second Edition190.Simulation: A Modeler's Approach191.Smoothing and Regression: Approaches, Computation, and Application192.Smoothing of Multivariate Data: Density Estimation and Visualization193.Spatial Statistics194.Spatial Statistics and Spatio-Temporal Data: Covariance Functions and Directional Properties195.Spatial Tessellations: Concepts and Applications of Voronoi Diagrams, Second Edition196.Stage-Wise Adaptive Designs197.Statistical Advances in the Biomedical Sciences: Clinical Trials, Epidemiology, Survival Analysis, and Bioinformatics198.Statistical Analysis of Profile Monitoring199.Statistical Design and Analysis of Experiments: With Applications to Engineering and Science, Second Edition200.Statistical Factor Analysis and Related Methods: Theory and Applications 201.Statistical Inference for Fractional Diffusion Processes202.Statistical Meta-Analysis with Applications203.Statistical Methods for Comparative Studies: Techniques for Bias Reduction 204.Statistical Methods for Forecasting205.Statistical Methods for Quality Improvement, Third Edition206.Statistical Methods for Rates and Proportions, Third Edition207.Statistical Methods for Survival Data Analysis, Third Edition208.Statistical Methods for the Analysis of Biomedical Data, Second Edition 209.Statistical Methods in Diagnostic Medicine210.Statistical Methods in Diagnostic Medicine, Second Edition211.Statistical Methods in Engineering and Quality Assurance212.Statistical Methods in Spatial Epidemiology, Second Edition213.Statistical Modeling by Wavelets214.Statistical Models and Methods for Lifetime Data, Second Edition215.Statistical Rules of Thumb, Second Edition216.Statistical Size Distributions in Economics and Actuarial Sciences217.Statistical Texts for Mixed Linear Models218.Statistical Tolerance Regions: Theory, Applications, and Computation219.Statistics for Imaging, Optics, and Photonics220.Statistics for Research, Third Edition221.Statistics of Extremes: Theory and Applications222.Statistics: A Biomedical Introduction223.Stochastic Dynamic Programming and the Control of Queueing Systems224.Stochastic Processes for Insurance & Finance225.Stochastic Simulation226.Structural Equation Modeling: A Bayesian Approach227.Subjective and Objective Bayesian Statistics: Principles, Models, and Applications, Second Edition228.Survey Errors and Survey Costs229.System Reliability Theory: Models, Statistical Methods, and Applications, Second Edition230.The Analysis of Covariance and Alternatives: Statistical Methods for Experiments, Quasi-Experiments, and Single-Case Studies, Second Edition231.The Construction of Optimal Stated Choice Experiments: Theory and Methods 232.The EM Algorithm and Extensions, Second Edition233.The Statistical Analysis of Failure Time Data, Second Edition234.The Subjectivity of Scientists and the Bayesian Approach235.The Theory of Measures and Integration236.The Theory of Response-Adaptive Randomization in Clinical Trials237.Theory of Preliminary Test and Stein-Type Estimation With Applications 238.Time Series Analysis and Forecasting by Example239.Time Series: Applications to Finance with R and S-Plus, Second Edition 240.Uncertainty Analysis with High Dimensional Dependence Modelling241.Univariate Discrete Distributions, Third Editioning the Weibull Distribution: Reliability, Modeling, and Inference243.Variance Components244.Variations on Split Plot and Split Block Experiment Designs245.Visual Statistics: Seeing Data with Dynamic Interactive Graphics246.Weibull Models。
- 1、下载文档前请自行甄别文档内容的完整性,平台不提供额外的编辑、内容补充、找答案等附加服务。
- 2、"仅部分预览"的文档,不可在线预览部分如存在完整性等问题,可反馈申请退款(可完整预览的文档不适用该条件!)。
- 3、如文档侵犯您的权益,请联系客服反馈,我们会尽快为您处理(人工客服工作时间:9:00-18:30)。
Chapter 18 - Key Terms
• Time series
• Classical time series model
– Trend value – Cyclical component – Seasonal component – Irregular component
• Trend equation
• Shifting the base of an index
© 2002 The Wadsworth Group
Classical Time Series Model
y=T•C•S•I
where y = observed value of the time series variable
T = trend component, which reflects the general tendency of the time series without fluctuations
• Use MAD and MSE criteria to compare how well equations fit data.
• Use index numbers to compare business or economic measures over time.
© 2002 The Wadsworth Group
• Fit a linear or quadratic trend equation to a time series.
• Smooth a time series with the centered moving average and exponential smoothing techniques.
• Moving average
• Exponential smoothing
• Seasonal index
• Ratio to moving average method
• Deseasonalizing
• MAD criterion
• MSE criterion
• Constructing an index using the CPI
© 2002 The Wadsworth Group
Smoothing Techniques
• Smoothing techniques - dampen the impacts of fluctuation in a time series, thereby providing a better view of the trend and (possibly) the cyclical components.
© 2002 The Wadsworth Group
Chapter 18 - Learning Objectives
• Describe the trend, cyclical, seasonal, and irregular components of the time series model.
CHAPTER 18 Models for Time Series and
Forecasting
to accompany
Introduction to Business Statistics
fourth edition, by Ronald M. Weiers
Presentation by Priscilla Chaffe-Stengel Donald N. Stengel
I = irregular component, which reflects fluctuations that
are not systematic
© 2002 The Wadsworth Group
Trend Equations
• Linear: y? = b0 + b1x • Quadratic: y? = b0 + b1x + b2x2
• Moving average - a technique that replaces a data value with the average of that data value and neighboring data values.
• Exponential smoothing - a technique that replaces a data value with a weighted average of the actual data value and the value resulting from exponential smoothing for the previous time period.
• Determine seasonal indexes and use them to compensate for the seasonal effects in a time series.
• Use the trend extrapolation and exponential smoothing forecast methods to estimate a future value.
y?= the trend line estimate of y
x = time period
b0, b1, and b2 are coefficients that are selected to minimize the deviations between the trend estimates y? and the actual data values y for the past time periods. Regression methods are used to determine the best values for the coefficients.
C = cyclical component, which reflects systematic fluctuations that are not calendar-related, such as business cycles
S = seasonal component, which reflects systematic fluctuations that are calendar-related, such as the day of the week or the month of the year