消防安全风险评估模型研究
火灾安全评估模型研究
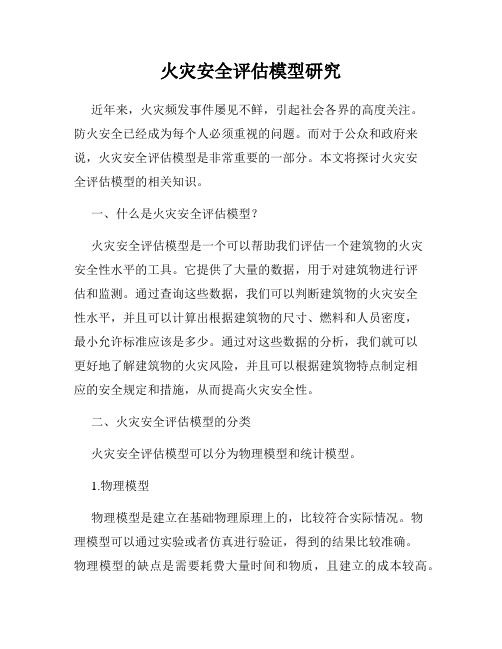
火灾安全评估模型研究近年来,火灾频发事件屡见不鲜,引起社会各界的高度关注。
防火安全已经成为每个人必须重视的问题。
而对于公众和政府来说,火灾安全评估模型是非常重要的一部分。
本文将探讨火灾安全评估模型的相关知识。
一、什么是火灾安全评估模型?火灾安全评估模型是一个可以帮助我们评估一个建筑物的火灾安全性水平的工具。
它提供了大量的数据,用于对建筑物进行评估和监测。
通过查询这些数据,我们可以判断建筑物的火灾安全性水平,并且可以计算出根据建筑物的尺寸、燃料和人员密度,最小允许标准应该是多少。
通过对这些数据的分析,我们就可以更好地了解建筑物的火灾风险,并且可以根据建筑物特点制定相应的安全规定和措施,从而提高火灾安全性。
二、火灾安全评估模型的分类火灾安全评估模型可以分为物理模型和统计模型。
1.物理模型物理模型是建立在基础物理原理上的,比较符合实际情况。
物理模型可以通过实验或者仿真进行验证,得到的结果比较准确。
物理模型的缺点是需要耗费大量时间和物质,且建立的成本较高。
2.统计模型统计模型是基于统计学和概率论,通过样本分析和研究来判断一个建筑物的火灾风险程度。
统计模型的优点是建立和比较简单,可以通过样本收集和分析来得到结果,且可以根据实际情况进行相应的调整。
但是统计模型的缺点是不能精确计算,因为建筑物的火灾风险受到很多因素的影响,这些因素往往是难以量化、难以预测和估计的,所以统计模型的计算结果只是一个大致的参考。
三、影响火灾安全评估模型的因素1.建筑物结构建筑物结构直接影响火灾扩散的速度和路径、消防措施的实施和疏散的效率。
因此,在火灾安全评估模型的建立中,要对建筑物结构进行全面、系统的分析。
2.消防设备的情况消防设备是建筑物内部防火的重要因素。
在火灾安全评估模型中,要对消防设备的类型、数量、分布等因素进行统计和分析。
3.人员行为人员行为可以影响火灾的发生和蔓延。
在火灾安全评估模型中需要考虑人员行为因素,适当调整预测结果。
消防安全风险研判分析报告

消防安全风险研判分析报告1. 引言本报告旨在分析和研判当前消防安全领域中存在的风险因素,并提供相应的解决方案和建议。
消防安全是保护人民生命财产安全的重要领域,而对于消防安全风险的准确研判和分析则是预防火灾和事故的基础工作。
2. 方法和数据来源为了全面有效地分析消防安全风险,我们采用了多种方法和数据来源。
首先,我们收集了相关的消防安全数据,包括火灾事故报告、消防监督检查报告和消防设施运行记录等。
其次,我们进行了现场调研和观察,并进行了一系列访谈,包括相关行业专家、消防设施管理人员和消防安全从业人员等。
最后,我们还分析了相关法律法规和消防安全标准等文件。
3. 消防安全风险研判分析3.1 火灾事故原因分析通过对历年来的火灾事故进行分析,我们发现以下主要原因:•电气设备故障:电气设备老化、过载、短路等问题,是引发火灾事故的主要原因之一。
•人为操作失误:由于人为操作不当,如使用明火、乱扔烟蒂等,导致火灾事故的发生。
•防火管理不力:一些单位和个人对于火灾防范意识不强,缺乏有效的防火措施,导致火灾风险增加。
•消防设施故障:一些消防设施存有隐患,如灭火器过期、消防通道被占用等,导致火灾扑灭延误。
3.2 消防安全风险评估根据上述火灾事故原因分析,我们对消防安全风险进行了评估。
我们采用综合评估模型,考虑了事故发生概率、事故后果和事故防范措施等因素,并给出了相应的风险等级。
•高风险区域:那些存在多种火灾事故原因且火灾后果严重、防范措施薄弱的区域。
•中风险区域:那些存在单一或部分火灾事故原因且火灾后果一般、防范措施较完善但仍有改进空间的区域。
•低风险区域:那些不存在或仅存在极少数火灾事故原因且火灾后果较轻、防范措施比较完善的区域。
3.3 解决方案和建议针对火灾事故原因和消防安全风险评估结果,我们提出如下解决方案和建议:•强化消防安全宣传:加强对公众的消防安全知识普及,提高公众的消防安全意识和应急能力。
•完善消防设施:对于存在故障或隐患的消防设施,要及时维修和更换,确保其正常运行。
消防安全风险评估与分析
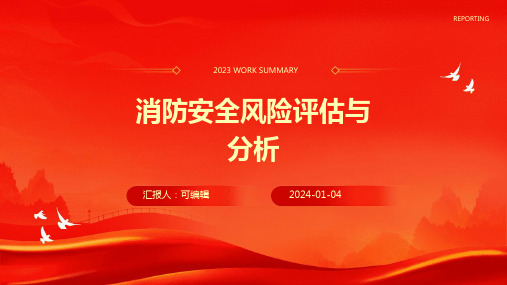
故的能力。
提高安全意识
风险评估有助于提高企业和个人的消 防安全意识,促进对消防安全问题的 重视。
优化资源配置
了解火灾风险分布和严重程度,有助 于合理配置消防安全资源,提高资源 利用效率。
对企业和个人的建议
企业应定期进行消防安全风险评估,及时发现 和整改火灾隐患,确保生产场所和设施符合消
事故原因
操作失误引发化学反应失控,产生大量热能 和有毒气体。
风险评估
化工厂存在高风险因素,如易燃易爆物质、 高温高压设备等,且事故后果严重。
应对措施
严格遵守操作规程,加强设备维护和巡检, 建立完善的应急预案和救援体系。
高层建筑火灾应对策略
高层建筑基本情况
楼层高、垂直通道多、人员密集、疏散困难 。
火灾特点
常见消防安全风险源
电气火灾
总结词
电气火灾是常见的消防安全风险之一,由于电线老化、过载或接触不良等原因 ,可能引发火灾。
详细描述
电气火灾通常发生在建筑物内部,尤其是老旧建筑中,由于电线老化、过载或 接触不良等原因,可能引发火灾。此外,不规范的电气安装和使用也是电气火 灾的重要原因之一。
易燃易爆物品
THANKS
感谢观看
REPORTING
商场消防设施不完善,缺乏定期 维护和检查,员工消防安全意识
薄弱。
02
事故原因
电气线路老化短路引发火灾,火 势迅速蔓延。
04
应对措施
加强消防安全管理,定期进行消 防设施检查和维护,提高员工消 防安全意识和应急处理能力。
某化工厂火灾风险评估与控制
化工厂基本情况
涉及多种危险化学品,生产工艺复杂,高温 高压等特殊条件。
消防安全与保险产品的风险评估模型比较
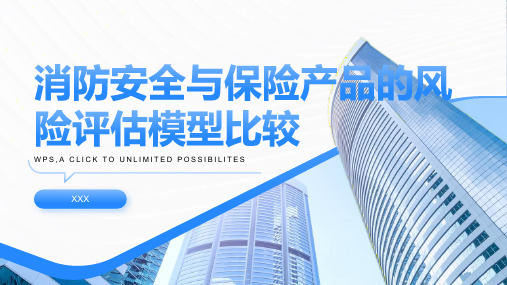
XXX
目录
CONTENTS
评估火灾发生的可能性 识别潜在的火灾危险源
评估火灾发生后可能造成的 损失
确定风险等级并进行优先级 排序
火灾发生的可能性
火灾发生后可能造成的损失
建筑物的结构特点和材料
消防设施的配备和运行状况
定性评估:基于专家经验和判断的方法,对火灾发生的可能性和后果进行评估。
评估方法:说明在案例中采 用的风险评估方法,如定性
评估、定量评估等。
评估结果:介绍案例中风险 评估的结果,如风险等级、
应对措施等。
案例名称:某保险公司火灾保险产品风险 评估
案例简介:该案例主要针对火灾保险产品 的风险评估,通过分析历史数据和风险因 素,评估保险产品的风险水平。
风险评估方法:采用定量和定性相结合的 方法,包括统计分析和专家评估等。
消防安全与保险产品的风险评估模型在评估风险和提供保障方面具有重要 意义。 不同模型之间存在差异,需要根据具体情况选择合适的模型。
未来研究可以进一步探讨模型优化和数据来源的可靠性等方面。
实际应用中需要综合考虑各种因素,提高风险评估的准确性和可靠性。
建立多层次评估 指标体系,全面 考虑消防安全各 个方面的影响因 素。
级、损失程度等
风险应对措施:针对不同风 险制定相应的防范和应对措
施
风险发生概率 风险损失程度 风险可接受程度 风险控制成本
风险识别:识别潜在的风险因素, 为评估提供基础数据。
风险评估标准:根据风险发生的 可能性和影响程度,确定风险等 级。
添加标题
添加标题
添加标题
添加标题
风险衡量:对风险发生的可能性、 影响程度进行量化评估。
基于AHP法的消防风险评估模型研究

t mietei p c ses e t f at n c r, e r iigf t ( e )o ahl e e e n at ssm n c radf t s i dt m nn c r st f c a rb— r h m a of o ao n e ao s s e y
力 一 。
新月异 , 城市人 口密度、 建筑密度不断增大 , 建筑 高度不断增加 , 不论是大到消防队 ( 、 站) 消防安 全重点单位 , 还是小到消火栓等 , 共同的特点都具 有 空 间分布 的特 性 , 类 信 息 置 于空 间分 布 中进 各 行管理和综合分析 的能力 , 十分符合消 防数据多 样化 、 空 间的 管 理特 点 , 于 A P法 消 防 风 险 大 基 H 评估模型 , 更有助 于信息化高新技术引入到消防
第2 卷 第1 9 期
21 0 1年 2月
江
西
科
学
Vo . 9 No 1 12 . F b. O1 e 2 1
JANG S I I XI C ENC E
文章编号 :0 1— 69 2 1 ) 1 0 0 0 10 3 7 (0 1 0 - 15— 4
基 于 A P法 的消 防 风 险评估 模 型 研 究 H
收稿 1期 :0 0一l —1 ; 3 21 1 1 修订 日期 :0 1 O 2 2 1 一 1— 7 作者简介 : 邓
l 算法理论基 础
区域 火 灾 风 险 评 估 模 型 采 用 层 级 分 析 法 (H A P法 ) 确定 影 响评 估 的 因子 和 因 素r引; 在 7 并 ' 确定 每层 因子 ( 素 ) 因 的权 重 时 采 用 的 9标 度 的 比较法 和专 家经验 比较 因子 间 的相对 重要 性和 每 个 因 子包含 的 因素 间 的相 对 重 要性 , 后 根据 归 最
火灾风险评估模型研究及应用实践案例分享
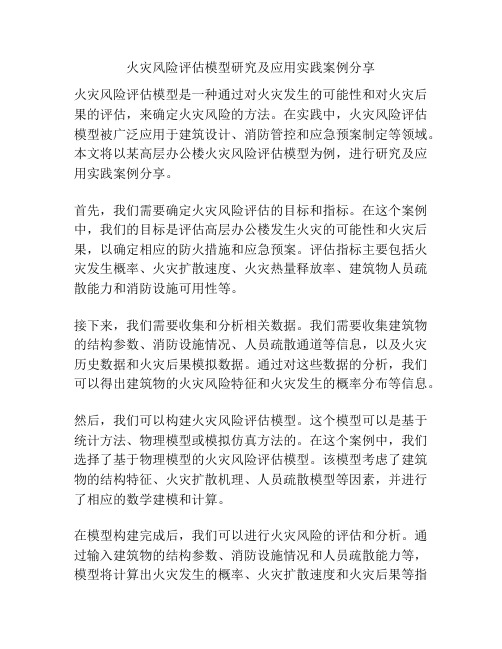
火灾风险评估模型研究及应用实践案例分享火灾风险评估模型是一种通过对火灾发生的可能性和对火灾后果的评估,来确定火灾风险的方法。
在实践中,火灾风险评估模型被广泛应用于建筑设计、消防管控和应急预案制定等领域。
本文将以某高层办公楼火灾风险评估模型为例,进行研究及应用实践案例分享。
首先,我们需要确定火灾风险评估的目标和指标。
在这个案例中,我们的目标是评估高层办公楼发生火灾的可能性和火灾后果,以确定相应的防火措施和应急预案。
评估指标主要包括火灾发生概率、火灾扩散速度、火灾热量释放率、建筑物人员疏散能力和消防设施可用性等。
接下来,我们需要收集和分析相关数据。
我们需要收集建筑物的结构参数、消防设施情况、人员疏散通道等信息,以及火灾历史数据和火灾后果模拟数据。
通过对这些数据的分析,我们可以得出建筑物的火灾风险特征和火灾发生的概率分布等信息。
然后,我们可以构建火灾风险评估模型。
这个模型可以是基于统计方法、物理模型或模拟仿真方法的。
在这个案例中,我们选择了基于物理模型的火灾风险评估模型。
该模型考虑了建筑物的结构特征、火灾扩散机理、人员疏散模型等因素,并进行了相应的数学建模和计算。
在模型构建完成后,我们可以进行火灾风险的评估和分析。
通过输入建筑物的结构参数、消防设施情况和人员疏散能力等,模型将计算出火灾发生的概率、火灾扩散速度和火灾后果等指标。
通过对这些指标的评估,我们可以了解到火灾风险的大小,以及火灾对人员和建筑物的影响程度。
最后,基于评估结果,我们可以制定相应的防火措施和应急预案。
防火措施可以包括增加消防设施、加强建筑物的防火隔离等;应急预案可以包括定期演练、培训员工的疏散技能等。
通过这些措施和预案的实施,我们可以最大限度地减小火灾的风险,保护人民的生命财产安全。
在实践中,我们将该火灾风险评估模型应用于某高层办公楼。
通过对建筑结构和消防设施的评估,我们发现建筑物的火灾风险较高。
于是,我们向业主提出了增加消防设施和培训员工的疏散技能等建议,并针对火灾风险较高的区域制定了相应的应急预案。
消防安全评估方法的分析
消防安全评估方法的分析1. 引言消防安全是指预防和控制火灾及其相关危险的措施和程序。
对于建筑物、设施以及人员而言,消防安全评估是确保消防安全的重要工作之一。
消防安全评估方法是评估建筑物及其环境中的消防风险,并提供相应的安全措施的过程。
本文将对常见的消防安全评估方法进行分析。
2. 传统风险评估方法2.1. 公式计算法公式计算法是最常见的风险评估方法之一。
它通过计算各个消防风险因素的权重和得分,然后将得到的结果进行加权计算,得出综合风险评估值。
公式计算法通常包括定性与定量两种评估方法。
定性方法通过主观判断给出各个风险因素的等级,然后进行加权得分计算。
定量方法则通过统计数据和算法给出绝对的风险评估值。
2.2. 专家评估法专家评估法是利用专家的经验和知识,根据建筑物或设施的特点和消防安全要求,对其进行评估。
专家评估法常用于一些特殊场所,如化工厂、油库等,因为这些场所的消防风险较高,需要更加准确和专业的评估。
专家评估法的优点是能够结合实际情况进行评估,但缺点是过于依赖专家的主观意见,容易出现偏差。
2.3. 统计法统计法通过对大量的历史火灾数据进行分析和统计,得出各种火灾原因和风险因素的发生概率,然后根据建筑物或设施的特点进行相应的风险评估。
统计法的优点是能够基于客观的数据进行评估,但缺点是无法完全覆盖所有的情况,适用范围有限。
3. 现代风险评估方法3.1. 定量风险评估法定量风险评估法是一种利用数学模型对建筑物或设施的消防风险进行准确评估的方法。
该方法通过对火灾的发生概率、火势的蔓延速度、建筑物或设施的结构特点等进行建模和计算,最终得出消防风险的综合评估值。
定量风险评估法的优点是结果准确可靠,但缺点是需要较多的数据和专业知识支持。
3.2. 实地调研法实地调研法是指通过对建筑物或设施的实际情况进行调查和观察,确定其存在的消防隐患和安全问题。
该方法要求评估人员具备较高的消防知识和经验,能够通过观察和检测来发现隐患和问题。
火灾风险评估模型研究
火灾风险评估模型研究第一章绪论随着城市化进程的加速,建筑物数量和规模不断增大,火灾可能随时发生。
火灾是一种突发事件,常常会造成严重的人员伤亡和财产损失。
因此,在城市建设中,火灾风险评估和预防是至关重要的。
本文基于现有研究,探讨火灾风险评估模型的研究现状和发展趋势。
第二章火灾风险评估模型的类别火灾风险评估模型可以基于不同的评估指标进行分类,本文将其分为以下几类:2.1 基于建筑物参数的模型建筑物的结构、材料以及功能等是决定其火灾风险的重要因素。
因此,基于建筑物参数的模型是火灾风险评估的一种常见方法。
基于这种模型,研究者需要对建筑物结构参数、使用参数等进行量化,再开发出适合的评估指标,从而得到一个火灾风险评估模型,这种方法具有较高的精度和可靠性。
2.2 基于火灾历史数据的模型基于火灾历史数据的模型可以通过对过往火灾事件的分析,建立相应的评估模型。
这种方法通常会使用统计学方法和计算机模拟等技术进行模型的开发,通过对历史数据的分析来确定影响建筑物火灾风险的主要因素,同时可以对建筑物进行风险等级的分类,使得建筑物管理者能够及时采取相应的措施。
2.3 基于随机模型的模型基于随机模型的模型可以对火灾风险进行网格化评估,通过对网格内的参数进行随机抽样,得到整体的建筑物火灾风险模型。
这种方法需要建立相应的随机模型,同时需要对数据进行预处理,包括数据清洗、数据格式化和有效数据的提取等。
第三章火灾风险评估模型的应用火灾风险评估模型可应用于建筑物管理、火灾预防以及应急处理等方面。
3.1 建筑物管理基于火灾风险评估模型,可以对建筑物进行风险等级的评估和分类。
这种方法可以为建筑物的管理者提供建议和指导,如在高风险地区建立专业的消防队伍,增强灭火器材和人员配备等,从而提高建筑物的防火安全性。
3.2 火灾预防火灾风险评估模型可以为火灾预防提供科学的依据。
通过评估建筑物的火灾风险,可以预测潜在的火灾发生可能性,根据评估结果采取相应的预防措施,如提高建筑物的安全防护等级、加强火源管理、安装自动报警系统等措施,从而减少火灾事故的发生。
消防安全风险评估与管理
消防安全风险评估与管理消防安全是指在防火、灭火和应急救援等方面保障人民生命财产安全的工作。
针对建筑物、企事业单位和公共场所等不同区域的消防安全风险,进行评估与管理是非常重要的。
本文将探讨消防安全风险的评估与管理方法,并介绍相关的实践经验,以期提高消防安全的管理水平。
一、消防安全风险评估方法1.风险辨识风险辨识是指通过对建筑物和场所的巡查、检测等手段,识别可能存在的消防安全风险。
可以运用消防安全检查表、安全评估模型等工具,对不同区域进行系统的评估。
同时,还需考虑消防设施的更新、设备的维修保养情况,以及人员的防火宣传教育培训等因素。
2.风险评估风险评估是指对辨识出来的消防安全风险进行综合评估,确定其对人员生命财产安全的影响程度。
评估可以从火灾的发生概率、火势蔓延速度、人员疏散时间等角度进行考量,并根据评估结果进行等级划分。
3.风险分析风险分析是指对已评估的风险进行剖析和解释,找出导致风险产生的主要原因和隐患。
通过分析,可以确定风险的优先级,并制定相应的消防管理措施。
在分析过程中需要充分考虑建筑物的结构、火灾扩散途径、人员疏散通道和应急出口等问题。
4.风险控制风险控制是指通过采取合理可行的措施,降低或消除风险的可能性和影响。
针对不同类型的风险,可以采取技术手段、管理制度、应急预案等方式进行控制。
例如,对于高层建筑,可以加强防火墙和疏散通道的建设;对于涉及易燃易爆物品的场所,可以加强存储管理和安全防护等措施。
二、消防安全风险管理实践1.建立完善的消防安全管理制度建立完善的消防安全管理制度是保障消防安全的基础。
制度应包括消防设施、消防器材的维护与检修要求,人员的消防知识培训与演练要求,火灾事故的报告与调查要求等内容,以确保各项工作符合法律法规和标准要求。
2.加强消防设施的维护与管理消防设施是保障消防安全的重要设备,需要定期检查、保养和更新。
管理人员应建立健全的消防设施台账,并定期组织消防设施的维护保养工作,确保其正常运行。
火灾风险评估模型研究与应用
火灾风险评估模型研究与应用一、引言火灾一旦发生,常常会带来极大的人身财产损失。
为了减少火灾的发生和危害,进行火灾风险评估显得尤为重要。
对于火灾风险评估模型的研究和应用,是保障公共安全、维护社会稳定的重要举措。
二、火灾风险评估模型的基本原理火灾风险评估模型是一种用于评估建筑物火灾风险的数学模型。
其基本原理是将建筑物的特定参数进行量化,并结合历史数据和专家经验,对建筑物的火灾风险进行预测和评估。
1、建筑物参数的量化建筑物参数的量化是火灾风险评估模型的核心。
典型的参数包括建筑物结构、建筑物用途、建筑物内部装修材料等。
通过对建筑物参数进行量化,可以更客观地对火灾风险进行评估。
2、历史数据和专家经验的应用历史数据和专家经验是火灾风险评估模型的参考依据。
对于历史发生的火灾事件,可以通过对案例的分析和总结,提炼出规律和经验。
专家的经验和专业知识可以更深入地对建筑物的火灾风险进行评估。
三、火灾风险评估模型的应用火灾风险评估模型的应用范围非常广泛。
可以用于评估不同类型的建筑物,如商业建筑、住宅建筑、工业建筑等。
同时,火灾风险评估模型也逐渐被应用到城市和地区的规划和管理中。
1、建筑物火灾风险评估针对不同类型的建筑物,可以使用不同的火灾风险评估模型。
通过对建筑物的参数进行量化,并结合历史数据和专家经验,可以更好地评估建筑物的火灾风险,提出相应的改进建议。
2、城市火灾风险评估城市火灾风险评估主要是对城市内部的火灾风险进行评估。
包括城市的规划和建设、城市建筑物的火灾风险评估、城市消防队伍的建设等。
通过对城市火灾风险进行评估,可以提高城市的消防安全水平。
四、火灾风险评估模型的案例分析1、商业建筑火灾风险评估案例某商业建筑平均客流量为2000人/天,建筑面积为10000平方米,采用混凝土结构,内部装修材料为耐热性好的材料。
通过火灾风险评估模型,评估该商业建筑的火灾风险为中等。
2、住宅建筑火灾风险评估案例某住宅小区由6栋楼房组成,每栋楼房高度为7层,每层5户住户,住户总数为180户。
- 1、下载文档前请自行甄别文档内容的完整性,平台不提供额外的编辑、内容补充、找答案等附加服务。
- 2、"仅部分预览"的文档,不可在线预览部分如存在完整性等问题,可反馈申请退款(可完整预览的文档不适用该条件!)。
- 3、如文档侵犯您的权益,请联系客服反馈,我们会尽快为您处理(人工客服工作时间:9:00-18:30)。
City Fire Risk Assessment Model Based on the Adaptive Genetic Algorithm and BPNetworkJIAO AIHONGDepartment of Fire Commanding Chinese People’s Armed Police Forces Academy Lang fang China, 065000e-mail:ylzmyradio@YUAN LIZHENo.3 Department Nanjing Artillery Academy Langfang China, 065000 e-mail:ylzmyradio@Abstract—Based on the risk evaluation index system of city fire, a comprehensi ve evaluati on model wi th the adapti ve geneti c algorithm and BP neural network (AGA-BP) is established in the arti cle.In former process of the hybri d algori thm, the adapti ve geneti c algori thm i s appli ed to adjust wei ghts and thresholds of the three-layer BP neural network and train the BP neural network for locati ng the global opti mum, and the error back propagat i on algor i thm i s used to search i n ne ghborhoods of the approx mate opt mal solut on n the later process. The program wri tten i n VB6.0 i s used to learn some samples of c i ty f i re r i sk accord i ng to the AGA-BP algorithm and the general BP algorithm. The results show that the learning precision of AGA-BP algorithm is more correctly than that of the general BP algorithm. The training speed and convergence rate of the former i s s i gn i f i cantly i mproved because of the combi nati on of AGA and BP algori thm. It i s helpful to realize automated evaluation for city fire risk.Keywords-fire risk assessment; adaptive genetic algorithm; back propagation algorithmI.I NTRODUCTIONCity fire risk assessment is given a comprehensive evaluation conclusion on the probability of fire accidents and the vulnerability assessment of city facilities and the resistance ability of fire in the city,which is based on statistical analysis of city history fire data and hazard identification of the heavy danger sources. At present, the research on city fire risk assessment work is still very weak. Some foreign scholars are mainly concerationed on how to assess the city fire risk and reduce city fire losses and giving some assessment methods. It is helpful to plan city fire force and give a fire safety grade to the district by the fire risk evaluation conclusion. The home researchers is mostly focused on giving a synthetic evaluation conslusion for a certain producing enterprise or a particular building, while for fire risk assessment of the whole city is at a early stage presently.With the development of economy, there are more and more large and high buildings in big cities,and the spatial morphology is changing, and the population is increasing, and the wealth concentrated increasingly, oil, gas, electricity and decoration materials are widespread used in our living life, so the structure of city is complex, and the number of city fire hazards is growing.The safety evaluation methods in common use is including safety check list method, accident type and analysis method, fuzzy synthetic evaluation method, accident tree method, analytic hierarchy process and so on. These methods are short of further studies about the effect factors of fire, because the city security against fire as a whole, density of population, quantity of electricity and other factors are fireare interrelated, interaction and mutual checks each other. So, we need to notice that the evaluation process is dynamic and nonlinear. If we use artificial neural networks (ANN) and expert system to simulate the judgement reasoning and the decision-making process of city fire risk evaluation process, the limitations of traditional methods and the subjectiveness of experts can be avoided because of its good evaluation model structure and working platform.II.E RROR B ACK PROPAGATION ALGORITHMFigure 1three-layer BP network structure. TheThe three-layer BP neural network structure is shown in Fig.1. Error back propagation algorithm is one of the most popular neural network learning algorithms,which has been used widely in many fields, such as pattern recognition, fault diagnosis and automatic controls[1]. The BP algorithm trains a given feed-forward multilayer neural network for a given set of input patterns with known samples. When each entry of the sample set is presented to the network, the network examines its output response to the sample input pattern. The output response is then compared to the known and desired output and the error value is calculated. Based2012 International Conference on Industrial Control and Electronics Engineeringon the error, the connection weights and thresholds are adjusted.The backpropagation algorithm is based on Widrow-Hoff delta learning rule in which the weight adjustment is done through mean square error of the output response to the sample input. The set of these sample patterns are repeatedly presented to the network until the error value is minimized.III.A DAPTIVE G ENETIC A LOGRITHMSimple Genetic Algorithms (SGA) was firstly proposed by John Holland and his students[2].Now,genetic algorithms have been extensively used in different domains as a type of robust optimization method. However, genetic algorithm todemonstrate the more serious question is “premature convergence” problem, less capable local optimization, the later slow convergence and can not guarantee convergence to the global optimal solution and so on. In recent years,many scholars try to improve genetic algorithms, such asimproving the encoding scheme, fitness function, geneticoperator design. For this reason, the adaptive genetic algorithms is proposed in this paper, which the crossoveroperation c and mutation operation at randomrespectively as fellow: p m p 1211'''()()c c cc max c p p f f p f p f f p f !°° ®°d °¯f f (1) 1211()()m m m m max m p p f f p f p f fp f !°° ®°d °¯f f(2)Here, max fis the best individual’s fitness, 'f is the better individual’s fitness in every group, f is the average fitness, and f is every individual’s fitness in the currentgeneration. IV.F IRE RISK E VALUATION MODEL BASED ON AGA ̢BP AL GORITHMIn actually, the city fire is a complex system, which is not only having a great number of risk evaluation indexes but also having a large calculation about the indexes and a wide area involved. So, a number of clear concepts and clear borderlines and readily accepted indexes are summarized in accordance with its intrinsic link and affiliation to create the evaluation indexes system. We can use the system to evaluate the fire condition of a city by the quantitative analysis and qualitative analysis method and get a comprehensive assessment score. To ensure some contexts of the system, it is need for extensive research and further detailed analysis and synthesis.Based on absorbing the anterior research fruit of other people and city fire fundamental characteristics, some evaluation indicatorsincluding the historical circumstances of city fire 1x , the development of city economic 2x , the characteristics of city industry 3x , the characteristics of city buildings 4x ,the layout of city structure 5x , the construction of municipal facilities 6x , the construction of fire control forces 7x , the masses fire qualities 8x , the fire safety culture 9x and the city meteorological conditions 10x is created in the paper. To choose the best connection weights and thresholds for a three-layer BP neural network, a combination algorithm with adaptive genetic algorithm and error back propagation algorithm is put forward for the city fire evaluation. Here are some evaluation steps about how to get the city fire comprehensive assessment score based on the AGA-BP algorithm [3].Step 1. Set a fire risk assessment objects set as learning samples. Step 2. Create a risk evaluation indexes system.It can portrait the risk condition from different aspects.Step 3. Initialize the connection weights and thresholds in the three-layer BP network for the risk evaluation indexesby generating some random numbers Some chromosomes are constructed with real-code schema in [] like X .k k a b ˈ11111111111111(,)i n j ij nj j p ip np p j p t jt pt t q jq pq q X w w w w w w w v v v v v v v v v T T T J J J """"""""""""""" ˈˈ w w (3)Some GA parameters including the population size popsize , the crossover probability ,, the mutation probability ,, the max evolutional generation MaxGen and the 1c P 2c P 1m P 2m P variable evolutional generation Gen are initialized, and BP parameters including iteration times epoch , learning rate D ,E are also initialized. If the error function can be represented as2111(2q m kk t t k t E y ¦¦)c (4)Then the fitness function f is defined as1/f E (5) Step 4.Set the variable number Gen asGen Gen (6) Step 5.Choose some samples from the fire risk assessment objects set by turn to the BP neural network, and calculate the mean-square error according to the actualoutput and the expected output, then get the fitness of chromosome X from (5).Step 6. Make some genetic operations in the evolution process. Selection is adopted by roulette wheel and keeping the best individual of each generation, crossover and mutation with randomly as (1), (2) by the fitness of every individual to get new connection weights and thresholds.Step 7. If the evolution process is completed, output the optimal individual, then go to step 8, or else, go back to step 4.Step 8.Do some times iteration calculation using BP algorithm for the optimal individual in the evolution process, then get the near global optimal solution of the problem.Step 9. Check the correctness and validity of the BP neural network, if the learning precision is satisfied, we can use it to solve some similar problems.V.C ON CLUSIONChoose the data in table ĉ as learning samples to the three–layer 10×25×1 BP neural network, table Ċ is the result of comparing with the AGA-BP algorithm and the traditional BP algorithm, which are written by VB6.0 with the start of same connection weights ,{}ij w {}jt v and thresholds {}j T ,{}t J . Here are The AGA-BP algorithm parameters:Popsize=50, MaxGen=2000, epoch =2000, =0.9, =0.6, 1c P 2c P 1m P =0.01 and 2m P =0.001.In the AGA-BP algorithm, The BP neural network parameters epoch =25000. By monitoring the convergence of error values during the learning process, some conclusions can be drawn as follow:(1) To get the result in table Ċ, it spends the AGA-BP Algorithm 76.203 seconds and the BP algorithm 90.578 seconds in iterative computing in iterative computing in the PC with Intel ® Core™2 Duo Processor T5500 and 2GB memory and Windows XP operation system. It indicatesthat genetic algorithm can shorten training time of the BP neural network, and it can get a smaller error because of genetic algorithm in the AGA-BP algorithm. After the learning process is completed, the former error is nearby 0.00006 and the later is 0.00078.(2)To get the global error (˘0.001, the former algorithm reachs the convergence value in the 987th iterative calculation, while the later algorithm gets it in the 22033 th iterative calculation. It’s also shown that the AGA-BP algorithm is powerful,which combines the advantages of genetic algorithm with parallel computing and strong global searching capacity and the advantages of BP algorithm with powerful local-optimization ability.It is helpful to realize automated evaluation for city fire risk.TABLE II. T HE L EARNING R ESULT OF T HE S AMPLES AGA-BP algorithmBP algorithmNoExcepted OutputOutputRelative Error (%)OutputRelative Error (%)10.9330.93082-0.2335570.941370.89699920.856 0.86954 1.5816890.83849-2.04601230.792 0.793940.2449360.77832-1.72742140.863 0.86087-0.2463680.86049-0.29123050.688 0.68395-0.5882410.67957-1.22567860.852 0.852050.0006250.83610-1.86614370.739 0.739250.0334160.75828 2.60829180.801 0.802820.2266540.81095 1.24194990.682 0.682640.094440.684700.395739100.6350.639280.6746870.63184-0.497592R EFERENCES[1]Zhongzhi Shi, Neural Computation, Beijing:Press of electronic Industry, 1993.[2]J.H. Holland, Outline for a logical theory of adaptive systems, J. Assoc. Computer, Mach. 3 (1962) 297–314.[3]Aihong, Jiao and Lizhe, Yuan. Fault diagnosis based on adaptive genetic algorithm and BP neural network:ICCET 201 ü2010 International Conference on Computer Engineering and Technology, Proceedings, v6, p 427-430, 16-18 Apr 2010 [C], chengdu,China.TABLE I. T HE S AMPLESEvaluation Elements No 1x 2x 3x 4x 5x 6x 7x 8x 9x 10x Result11x 10.880.920.880.970.950.890.910.930.970.920.93320.810.850.790.830.800.860.840.810.850.840.85630.780.810.740.750.770.790.730.760.720.780.79240.840.830.880.820.760.880.850.870.830.810.86350.660.740.710.700.660.680.670.730.750.720.68860.840.770.910.840.810.860.830.790.870.840.85270.720.710.730.750.760.790.720.750.760.740.73980.870.820.850.870.820.830.760.770.790.780.80190.740.700.630.680.640.720.710.640.690.670.682100.610.600.620.660.610.600.640.670.650.620.635。