基因调控网络(精)
基因转录调控网络研究

基因转录调控网络研究是现代分子生物学领域的重要研究方向之一。
在基因表达的过程中,细胞需要通过调控多种信号通路上的信号转导,从而控制基因的转录和翻译,最终实现细胞内、细胞间以及生物体内的生理过程。
因此,探索基因转录调控网络对于理解细胞生命活动本质、阐明疾病的发生机制以及开发治疗手段具有重要的意义。
一、基因转录调控网络的研究方法基因转录调控网络是指遗传信息的传递、调控和控制网络在细胞内的交互作用。
研究基因转录调控网络需要从分子水平、细胞水平、生物系统水平等多个层面进行综合研究。
现有的研究方法主要有:(1)芯片技术。
芯片技术是一种将大量的DNA序列装配在芯片上进行高通量的DNA检测方法,可以用来刻画基因表达模式和转录因子的结合特异性。
(2)基因敲除/过表达技术。
通过对基因进行敲除或过表达,可以在生物体内研究目标基因在转录调控网络中的功能和作用机制。
(3)蛋白质-蛋白质相互作用技术。
蛋白质-蛋白质相互作用技术是一种用于研究蛋白质之间相互作用的方法,可以用来揭示转录因子、辅因子以及共激活子等蛋白质的作用机制。
(4)转录组学和蛋白质组学等综合方法。
通过转录组学和蛋白质组学等综合方法可以研究基因表达的变化和转录因子调控的多个层面。
二、基因转录调控网络的应用基因转录调控网络的研究在许多领域都具有重要的应用价值。
以下介绍几个典型领域:(1)癌症的发生和治疗。
癌症是一种多基因参与的疾病,通过研究基因转录调控网络可以揭示癌症发生的关键因素和治疗靶点,有望为癌症治疗提供新的方向和手段。
(2)药物靶点的鉴定与设计。
基因转录调控网络的研究可以帮助分析药物与细胞之间的相互作用,以及药物与目标基因之间的关系,从而有助于新药的设计和开发。
(3)功能基因组学。
通过对基因转录调控网络的深入研究可以为各种生物学问题提供基因功能注释、代谢网络重构和生命科学研究辅助等方面的帮助。
三、基因转录调控网络的挑战与前景基因转录调控网络的研究面临着一些挑战。
基因之间的调控关系及其网络结构

基因之间的调控关系及其网络结构基因是生命的基础单位,而基因的表达则涉及到细胞内许多调控因素的作用和相互影响。
近年来,随着基因测序等技术的不断发展,科学家们对基因调控网络的研究也越来越深入。
本文将从基因调控的角度,探讨基因之间的调控关系及其网络结构。
一、基因调控的发现历程最初对基因调控的研究是由俄国遗传学家梅切尼科夫在20世纪初期提出的,他认为基因是通过化学物质的作用来控制的。
后来,迈克尔斯等人在20世纪50年代证实了基因调控是通过某些蛋白质分子相互作用而实现的。
1959年,雅各布和芬哈姆提出了「具有二元状态的遗传信息储存在这一物质体系中;这些物质体系之间的相互作用决定了遗传系统的行为」的结论。
这被认为是现代分子生物学和遗传学基础理论的奠基之作。
此后,科学家们逐渐发现,基因的表达分为转录、剪切、运输、翻译、修饰和降解等多个步骤,每一步都受到许多调控因子的影响。
二、基因之间的调控关系在基因调控网络中,每个基因都有一些调控元件或启动子,它们可能对基因的转录产生正向或负向的调控作用。
这些调控元件包括转录因子(Transcription factor)、转录共激活因子(Co-activator)、转录协同因子(Co-factor)和DNA甲基化等。
转录因子是最为常见的一种调控元件,它们能够结合到基因的启动子区域,从而激活或抑制基因的表达。
在转录因子的结构上,它们具有一个DNA结合结构域和一个激活或抑制信号域。
激活或抑制信号域通过与一些共激活因子或共抑制因子相互作用来影响基因表达。
另一类调控元件是miRNA(microRNA),它们在小RNA中占有重要的地位。
miRNA一般通过降解mRNA或抑制翻译来调控基因。
miRNA一般与mRNA3’非翻译区域上的相互匹配序列结合,并将mRNA降解或转录抑制,从而影响基因表达。
三、基因调控网络结构基因调控是一个复杂的过程,其调控因子也非常复杂。
这些调控因子之间相互作用,构成了一个复杂的调控网络,由于相互作用的复杂性,绘制这种网络就成为了科学家们的研究方向之一。
基因调控网络模型研究综述
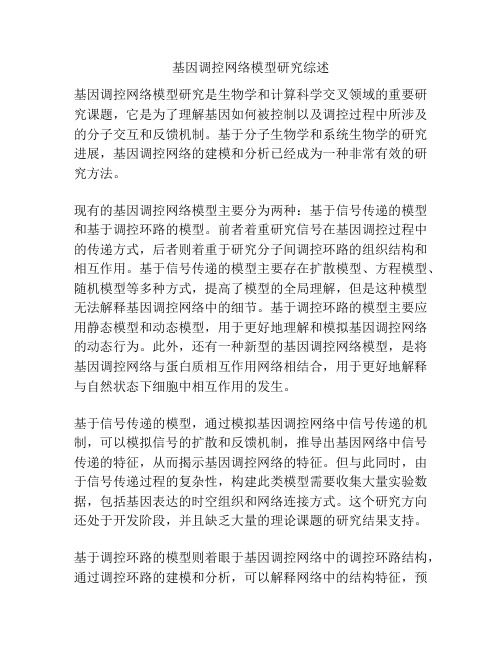
基因调控网络模型研究综述基因调控网络模型研究是生物学和计算科学交叉领域的重要研究课题,它是为了理解基因如何被控制以及调控过程中所涉及的分子交互和反馈机制。
基于分子生物学和系统生物学的研究进展,基因调控网络的建模和分析已经成为一种非常有效的研究方法。
现有的基因调控网络模型主要分为两种:基于信号传递的模型和基于调控环路的模型。
前者着重研究信号在基因调控过程中的传递方式,后者则着重于研究分子间调控环路的组织结构和相互作用。
基于信号传递的模型主要存在扩散模型、方程模型、随机模型等多种方式,提高了模型的全局理解,但是这种模型无法解释基因调控网络中的细节。
基于调控环路的模型主要应用静态模型和动态模型,用于更好地理解和模拟基因调控网络的动态行为。
此外,还有一种新型的基因调控网络模型,是将基因调控网络与蛋白质相互作用网络相结合,用于更好地解释与自然状态下细胞中相互作用的发生。
基于信号传递的模型,通过模拟基因调控网络中信号传递的机制,可以模拟信号的扩散和反馈机制,推导出基因网络中信号传递的特征,从而揭示基因调控网络的特征。
但与此同时,由于信号传递过程的复杂性,构建此类模型需要收集大量实验数据,包括基因表达的时空组织和网络连接方式。
这个研究方向还处于开发阶段,并且缺乏大量的理论课题的研究结果支持。
基于调控环路的模型则着眼于基因调控网络中的调控环路结构,通过调控环路的建模和分析,可以解释网络中的结构特征,预测生物体内的基因表达水平以及基因调控网络的稳定性。
但这类模型仍然存在的挑战是建立一个完整的基因调控网络,这需要大量的实验数据,这是一个不简单的事情。
近年来,随着人工智能、深度学习和强化学习等技术的迅猛发展,很多学者开始探索基于深度学习的基因调控网络模型。
基于深度学习的方法可以将大量的数据集成到模型中,从而实现准确的预测,让基因调控网络模型走向新的高峰。
此类模型目前仍处于起步阶段,还需要更多的深度研究,以求将其推向更为广泛的应用领域。
基因调控网络分析的方法及其应用

基因调控网络分析的方法及其应用基因调控是涉及到细胞和器官发育、生长、分化和去向的一个核心概念。
鉴定基因调控因子、研究调控机制以及构建调控网络也成为了当前生命科学研究中的热点问题之一。
基因调控网络是由不同生物分子之间互相作用而得的复杂网络系统,包括基因、蛋白质、DNA和RNA等,可以帮助我们全面了解与疾病有关的基因调控机制。
目前,随着高通量技术的发展,人们研究基因调控网络的方法也不断提高和创新,分析基因调控网络的方法已经成为了研究生物信息学、计算生物学和系统生物学的重要突破口之一。
一、基因调控网络分析的基本方法基于传统的生物学实验往往只能研究单个基因的表达,然而生命体的基因表达轮廓是由一系列功能关联的基因组成的复杂调控系统控制的,因此需要建立基因调控网络以揭示各个基因之间的相互作用与调控。
为了解决这一问题,人们开发出了一系列分析和挖掘基因调控网络的方法。
1. 转录因子和miRNA的调控基因预测:通过挖掘转录因子和miRNA的结构、功能和区域特征,可以预测它们可能调控的基因。
研究转录因子和miRNA对调控的基因进行预测,是基因调控网络中预处理实验数据的一个重要前提。
2. 基因共表达网络构建:基于微阵列技术和RNA测序技术,可以将不同生境下同类样本的基因表达数据进行聚类分析和共表达网络构建。
利用基因表达相似性以及数据挖掘和网络分析技术等方法,可以施行对基因调控网络中一个具有影响力的调控子集的分析。
3. ChIP-Seq和ATAC-Seq:结合精简的大规模测序技术,可以洞察转录因子和结合DNA上其他物质的区域。
这些技术可以得出哪些蛋白质与DNA紧密结合,并在一些特殊情况下发挥调控作用。
基于此技术可以进行在某一特定区域内研究转录因子活动的采集数据,是建立基因调控网络的重要手段之一。
4. 利用线型模型(linear modeling)识别基因调控网络:线性模型建立线性关系,识别基因间关系并构建网络。
线性建模是非常通用和简单的,因此在基因调控网络分析中经常被使用。
基因调控网络:数学模型与仿真-V1
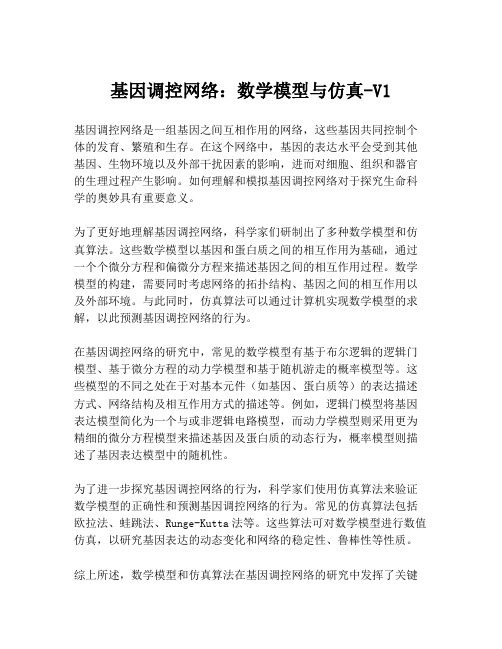
基因调控网络:数学模型与仿真-V1基因调控网络是一组基因之间互相作用的网络,这些基因共同控制个体的发育、繁殖和生存。
在这个网络中,基因的表达水平会受到其他基因、生物环境以及外部干扰因素的影响,进而对细胞、组织和器官的生理过程产生影响。
如何理解和模拟基因调控网络对于探究生命科学的奥妙具有重要意义。
为了更好地理解基因调控网络,科学家们研制出了多种数学模型和仿真算法。
这些数学模型以基因和蛋白质之间的相互作用为基础,通过一个个微分方程和偏微分方程来描述基因之间的相互作用过程。
数学模型的构建,需要同时考虑网络的拓扑结构、基因之间的相互作用以及外部环境。
与此同时,仿真算法可以通过计算机实现数学模型的求解,以此预测基因调控网络的行为。
在基因调控网络的研究中,常见的数学模型有基于布尔逻辑的逻辑门模型、基于微分方程的动力学模型和基于随机游走的概率模型等。
这些模型的不同之处在于对基本元件(如基因、蛋白质等)的表达描述方式、网络结构及相互作用方式的描述等。
例如,逻辑门模型将基因表达模型简化为一个与或非逻辑电路模型,而动力学模型则采用更为精细的微分方程模型来描述基因及蛋白质的动态行为,概率模型则描述了基因表达模型中的随机性。
为了进一步探究基因调控网络的行为,科学家们使用仿真算法来验证数学模型的正确性和预测基因调控网络的行为。
常见的仿真算法包括欧拉法、蛙跳法、Runge-Kutta法等。
这些算法可对数学模型进行数值仿真,以研究基因表达的动态变化和网络的稳定性、鲁棒性等性质。
综上所述,数学模型和仿真算法在基因调控网络的研究中发挥了关键作用。
这些工具和方法不仅有助于我们理解和预测基因调控网络的行为,也为未来研究提供了可靠的工具。
未来,随着人工智能、机器学习等技术的普及,基于数学模型和仿真算法的基因调控网络研究也将进入新的阶段。
Genetic regulatory network——基因调控网络知识点

Gene regulatory networkFrom Wikipedia, the free encyclopedia(Redirected from Genetic regulatory network) A gene regulatory network or genetic regulatory network (GRN) is a collection of DNA segments in a cell which interact with each other (indirectly through their RNA and protein expression products) and with other substances in the cell, thereby governing the rates at which genes in the network are transcribed into mRNA. In general, each mRNA molecule goes on to make a specific protein (or set of proteins). In some cases this protein will be structural, and will accumulate at the cell-wall or within the cell to give it particular structural properties. In other cases the protein will be an enzyme; a micromachine that catalyses a certain reaction, such as the breakdown of a food source or toxin. Some proteins though serve only to activate other genes, and these are the transcription factors that are the main players in regulatory networks or cascades. By binding to the promoter region at the start of other genes they turn them on, initiating the production of another protein, and so on. Some transcription factors are inhibitory. In single-celled organisms regulatory networks respond to the external environment, optimising the cell at a given time for survival in this environment. Thus a yeast cell, finding itself in a sugar solution, will turn on genes to make enzymes that process the sugar to alcohol.[1] This process, which we associate with wine-making, is how the yeast cell makes its living, gaining energy to multiply, which under normal circumstances would enhance its survival prospects.Structure of a Gene Regulatory Network.Control process of a Gene Regulatory Network.In multicellular animals the same principle has been put in the service of gene cascades that control body-shape. [2] Each time a cell divides, two cells result which, although they contain the same genome in full, can differ in which genes are turned on and making proteins. Sometimes a 'self-sustaining feedback loop' ensures that a cell maintains its identity and passes it on. Less understood is the mechanism of epigenetics by which chromatin modification may provide cellular memory by blocking or allowing transcription. A major feature of multicellular animals is the use of morphogen gradients, which in effect provide a positioning system that tells a cell where in the body it is, and hence what sort of cell to become. A gene that is turned on in one cell may make a product that leaves the cell and diffuses through adjacent cells, entering them and turning on genes only when it is present above a certain threshold level. These cells are thus induced into a new fate, and may even generate other morphogens that signal back to the original cell. Over longer distances morphogens may use the active process of signal transduction. Such signalling controls embryogenesis, the building of a body plan from scratch through a series of sequential steps. They also control maintain adult bodies through feedback processes, and the loss of such feedback because of a mutation can be responsible for the cell proliferation that is seen in cancer. In parallel with this process of building structure, the gene cascade turns on genes that make structural proteins that give each cell the physical properties it needs. It has been suggested that, because biological molecular interactions are intrinsically stochastic, gene networks are the result of cellular processes and not their cause. (i.e. Cellular Darwinism) However, recent experimental evidence has favored the attractor view of cell fates.Contents■ 1 Overview ■ 2 Modelling ■ 2.1 Coupled ODEs ■ 2.2 Boolean network ■ 2.3 Continuous networks ■ 2.4 Stochastic gene networks ■ ■ ■ ■ ■ ■ 3 Prediction 4 Network connectivity 5 See also 6 Notes 7 References 8 External linksOverviewAt one level, biological cells can be thought of as "partially-mixed bags" of biological chemicals – in the discussion of gene regulatory networks, these chemicals are mostly the mRNAs and proteins that arise from gene expression. These mRNA and proteins interact with each other with various degrees of specificity. Some diffuse around the cell. Others are bound to cell membranes, interacting with molecules in the environment. Still others pass through cell membranes and mediate long range signals to other cells in a multi-cellular organism. These molecules and their interactions comprise a gene regulatory network. A typical gene regulatory network looks something like this: The nodes of this network are proteins, their corresponding mRNAs, and protein/protein complexes. Nodes that are depicted as lying along vertical lines are associated with the cell/environment interfaces, while the others are free-floating and diffusible. Implied are genes, the DNA sequences which are transcribed into the mRNAs that translate into proteins. Edges between nodes represent individual molecular reactions, the protein/protein and protein/mRNA interactions through which the products of one gene affect those of another, though the lack of experimentally obtained information often implies that some reactions are not modeled at such a fine level of detail. These interactions can be inductive (the arrowheads), with an increase in the concentration of one leading to an increase in the other, or inhibitory (the filled circles), with an increase in one leading to a decrease in the other. A series of edges indicates a chain of such dependences, with cycles corresponding to feedback loops. The network structure is an abstraction of the system's chemical dynamics, describing the manifold ways in which one substance affects all the others to which it is connected. In practice, such GRNs are inferred from the biological literature on a given system and represent a distillation of the collective knowledge about a set of related biochemical reactions. Genes can be viewed as nodes in the network, with input being proteins such as transcription factors, and outputs being the level of gene expression. The node itself can also be viewed as a function which can be obtained by combining basic functions upon the inputs (in the Boolean network described below these are Boolean functions, typically AND, OR, and NOT). These functions have been interpreted as performing a kind of information processing within the cell, which determines cellular behavior. The basic drivers within cells are concentrations of some proteins, which determine both spatial (location within the cell or tissue) and temporal (cell cycle or developmental stage) coordinates of the cell, as a kind of "cellular memory". The gene networks are only beginning to be understood, and it is a next step for biology to attempt to deduce the functions for each gene "node", to help understand the behavior of the system in increasing levels of complexity, from gene to signaling pathway, cell or tissue level (see systems biology). Mathematical models of GRNs have been developed to capture the behavior of the system being modeled, and in some cases generate predictions corresponding with experimental observations. In some other cases, models have proven to make accurate novel predictions, which can be tested experimentally, thus suggesting new approaches to explore in an experiment that sometimes wouldn't be considered in the design of the protocol ofan experimental laboratory. The most common modeling technique involves the use of coupled ordinary differential equations (ODEs). Several other promising modeling techniques have been used, including Boolean networks, Petri nets, Bayesian networks, graphical Gaussian models, Stochastic, and Process Calculi. Conversely, techniques have been proposed for generating models of GRNs that best explain a set of time series observations.ModellingCoupled ODEsIt is common to model such a network with a set of coupled ordinary differential equations (ODEs) or stochastic ODEs, describing the reaction kinetics of the constituent parts. Suppose that our regulatory network has N nodes, and let represent the concentrations of the N corresponding substances at time t. Then the temporal evolution of the system can be described approximately bywhere the functions fj express the dependence of Sj on the concentrations of other substances present in the cell. The functions fj are ultimately derived from basic principles of chemical kinetics or simple expressions derived from these e.g. Michaelis-Menten enzymatic kinetics. Hence, the functional forms of the fj are usually chosen as low-order polynomials or Hill functions that serve as an ansatz for the real molecular dynamics. Such models are then studied using the mathematics of nonlinear dynamics. System-specific information, like reaction rate constants and sensitivities, are encoded as constant parameters.[3] By solving for the fixed point of the system:for all j, one obtains (possibly several) concentration profiles of proteins and mRNAs that are theoretically sustainable (though not necessarily stable). Steady states of kinetic equations thus correspond to potential cell types, and oscillatory solutions to the above equation to naturally cyclic cell types. Mathematical stability of these attractors can usually be characterized by the sign of higher derivatives at critical points, and then correspond to biochemical stability of the concentration profile. Critical points and bifurcations in the equations correspond to critical cell states in which small state or parameter perturbations could switch the system between one of several stable differentiation fates. Trajectories correspond to the unfolding of biological pathways and transients of the equations to short-term biological events. For a more mathematical discussion, see the articles on nonlinearity, dynamical systems, bifurcation theory, and chaos theory.Boolean networkThe following example illustrates how a Boolean network can model a GRN together with its gene products (the outputs) and the substances from the environment that affect it (the inputs). Stuart Kauffman was amongst the first biologists to use the metaphor of Boolean networks to model genetic regulatory networks.[4][5] 1. Each gene, each input, and each output is represented by a node in a directed graph in which there is an arrow from one node to another if and only if there is a causal link between the two nodes. 2. Each node in the graph can be in one of two states: on or off. 3. For a gene, "on" corresponds to the gene being expressed; for inputs and outputs, "on" corresponds to the substance being present. 4. Time is viewed as proceeding in discrete steps. At each step, the new state of a node is a Boolean function of the prior states of the nodes with arrows pointing towards it. The validity of the model can be tested by comparing simulation results with time series observations.Continuous networksContinuous network models of GRNs are an extension of the boolean networks described above. Nodes still represent genes and connections between them regulatory influences on gene expression. Genes in biological systems display a continuous range of activity levels and it has been argued that using a continuous representation captures several properties of gene regulatory networks not present in the Boolean model.[6] Formally most of these approaches are similar to an artificial neural network, as inputs to a node are summed up and the result serves as input to a sigmoid function, e.g.,[7] but proteins do often control gene expression in a synergistic, i.e. non-linear, way.[8] However there is now a continuous network model[9] that allows grouping of inputs to a node thus realizing another level of regulation. This model is formally closer to a higher order recurrent neural network. The same model has also been used to mimic the evolution of cellular differentiation [10] and even multicellular morphogenesis.[11]Stochastic gene networksRecent experimental results[12] [13] have demonstrated that gene expression is a stochastic process. Thus, many authors are now using the stochastic formalism, after the work by.[14] Works on single gene expression[15] and small synthetic genetic networks,[16][17] such as the genetic toggle switch of Tim Gardner and Jim Collins, provided additional experimental data on the phenotypic variability and the stochastic nature of gene expression. The first versions of stochastic models of gene expression involved only instantaneous reactions and were driven by the Gillespie algorithm.[18] Since some processes, such as gene transcription, involve many reactions and could not be correctly modeled as an instantaneous reaction in a single step, it was proposed to model these reactions as single step multiple delayed reactions in order to account for the time it takes for the entire process to be complete.[19] From here, a set of reactions were proposed[20] that allow generating GRNs. These are then simulated using a modified version of the Gillespie algorithm, that can simulate multiple time delayed reactions (chemical reactions where each of the products is provided a time delay that determines when will it be released in the system as a "finished product"). For example, basic transcription of a gene can be represented by the following single-step reaction (RNAP is the RNA polymerase, RBS is the RNA ribosome binding site, and Pro i is the promoter region of gene i):A recent work proposed a simulator (SGNSim, Stochastic Gene Networks Simulator),[21] that can model GRNs where transcription and translation are modeled as multiple time delayed events and its dynamics is driven by a stochastic simulation algorithm (SSA) able to deal with multiple time delayed events. The time delays can be drawn from several distributions and the reaction rates from complex functions or from physical parameters. SGNSim can generate ensembles of GRNs within a set of user-defined parameters, such as topology. It can also be used to model specific GRNs and systems of chemical reactions. Genetic perturbations such as gene deletions, gene over-expression, insertions, frame shift mutations can also be modeled as well. The GRN is created from a graph with the desired topology, imposing in-degree and out-degree distributions. Gene promoter activities are affected by other genes expression products that act as inputs, in the form of monomers or combined into multimers and set as direct or indirect. Next, each direct input is assigned to an operator site and different transcription factors can be allowed, or not, to compete for the same operator site, while indirect inputs are given a target. Finally, a function is assigned to each gene, defining the gene's response to a combination of transcription factors (promoter state). The transfer functions (that is, how genes respond to a combination of inputs) can be assigned to each combination of promoter states as desired. In other recent work, multiscale models of gene regulatory networks have been developed that focus on synthetic biology applications. Simulations have been used that model all biomolecular interactions in transcription, translation, regulation, and induction of gene regulatory networks, guiding the design of synthetic systems.[22]PredictionOther work has focused on predicting the gene expression levels in a gene regulatory network. The approaches used to model gene regulatory networks have been constrained to be interpretable and, as a result, are generally simplified versions of the network. For example, Boolean networks have been used due to their simplicity and ability to handle noisy data but lose data information by having a binary representation of the genes. Also, artificial neural networks omit using a hidden layer so that they can be interpreted, losing the ability to model higher order correlations in the data. Using a model that is not constrained to be interpretable, a more accurate model can be produced. Being able to predict gene expressions more accurately provides a way to explore how drugs affect a system of genes as well as for finding which genes are interrelated in a process. This has been encouraged by the DREAM competition[23] which promotes a competition for the best prediction algorithms.[24] Some other recent work has used artificial neural networks with a hidden layer.[25]Network connectivityEmpirical data indicate that biological gene networks are sparsely connected, and that the average number of upstream-regulators per gene is less than two.[26] Theoretical results show that selection for robust gene networks will favor minimally complex, more sparsely connected, networks.[26] These results suggest that a sparse, minimally connected, genetic architecture may be a fundamental design constraint shaping the evolution of gene network complexity.See also■ ■ ■ ■ ■ ■ ■ Body plan Cis-regulatory module Genenetwork (database) Morphogen Operon Synexpression Systems biologyNotes1. ^ "Transcriptional Regulatory Networks in Saccharomyces cerevisiae" (/young/regulator_network/) . Young Lab. /young/regulator_network/. 2. ^ Davidson E, Levin M (April 2005). "Gene regulatory networks" (/cgi/content/full/102/14/4935) . Proc. Natl. Acad. Sci. U.S.A. 102 (14): 4935. doi:10.1073/pnas.0502024102 (/10.1073%2Fpnas.0502024102) . PMC 556010 (/articlerender.fcgi?tool=pmcentrez&artid=556010) . PMID 15809445 (/pubmed/15809445) . /cgi/content/full/102/14/4935. 3. ^ Chu D, Zabet NR, Mitavskiy B (April 2009). "Models of transcription factor binding: sensitivity of activation functions to model assumptions" (/retrieve/pii/S0022-5193(08)00631-0) . J. Theor. Biol. 257 (3): 419–29. doi:10.1016/j.jtbi.2008.11.026 (/10.1016%2Fj.jtbi.2008.11.026) . PMID 19121637 (/pubmed/19121637) . /retrieve/pii/S0022-5193(08)00631-0. 4. ^ Kauffman, Stuart (1993). The Origins of Order. ISBN 0195058119. 5. ^ Kauffman SA (1969). "Metabolic stability and epigenesis in randomly constructed genetic nets" (http://lis.epfl.ch/~markus/References/Kauffman69.pdf) . Journal of Theoretical Biology (22): 437–467. http://lis.epfl.ch/~markus/References/Kauffman69.pdf. 6. ^ Vohradsky J (September 2001). "Neural model of the genetic network" (/cgi/pmidlookup? view=long&pmid=11395518) . J. Biol. Chem. 276 (39): 36168–73. doi:10.1074/jbc.M104391200 (/10.1074%2Fjbc.M104391200) . PMID 11395518 (/pubmed/11395518) . /cgi/pmidlookup? view=long&pmid=11395518. 7. ^ Geard N, Wiles J (2005). "A gene network model for developing cell lineages" (/doi/abs/10.1162/1064546054407202?url_ver=Z39.882003&rfr_id=ori:rid:&rfr_dat=cr_pub%) . Artif. Life 11 (3): 249–67.8. 9.10.11.12.13.14.15.16. 17. 18. 19.20.21.doi:10.1162/1064546054407202 (/10.1162%2F1064546054407202) . PMID 16053570 (/pubmed/16053570) . /doi/abs/10.1162/1064546054407202?url_ver=Z39.882003&rfr_id=ori:rid:&rfr_dat=cr_pub%. ^ Schilstra MJ, Bolouri H (2 January 2002). "Modelling the Regulation of Gene Expression in Genetic Regulatory Networks" (/bio/maria/NetBuilder/Theory/NetBuilderModelling.htm) . Biocomputation group, University of Hertfordshire. /bio/maria/NetBuilder/Theory/NetBuilderModelling.htm. ^ Knabe JF, Nehaniv CL, Schilstra MJ, Quick T (2006). "Evolving Biological Clocks using Genetic Regulatory Networks" (/viewdoc/download?doi=10.1.1.72.5016&rep=rep1&type=pdf) . Proceedings of the Artificial Life X Conference (Alife 10). MIT Press. pp. 15–21. /viewdoc/download? doi=10.1.1.72.5016&rep=rep1&type=pdf. ^ Knabe JF, Nehaniv CL, Schilstra MJ (2006). "Evolutionary Robustness of Differentiation in Genetic Regulatory Networks" (/viewdoc/download?doi=10.1.1.71.8768&rep=rep1&type=pdf) . Proceedings of the 7th German Workshop on Artificial Life 2006 (GWAL-7). Berlin: Akademische Verlagsgesellschaft Aka. pp. 75–84. /viewdoc/download?doi=10.1.1.71.8768&rep=rep1&type=pdf. ^ Knabe JF, Schilstra MJ, Nehaniv CL (2008). "Evolution and Morphogenesis of Differentiated Multicellular Organisms: Autonomously Generated Diffusion Gradients for Positional Information" (http://panmental.de/papers/FlagPottsGRNALife11.pdf) . Artificial Life XI: Proceedings of the Eleventh International Conference on the Simulation and Synthesis of Living Systems. MIT Press. http://panmental.de/papers/FlagPottsGRNALife11.pdf. ^ Elowitz MB, Levine AJ, Siggia ED, Swain PS (August 2002). "Stochastic gene expression in a single cell" (/cgi/pmidlookup?view=long&pmid=12183631) . Science 297 (5584): 1183–6. doi:10.1126/science.1070919 (/10.1126%2Fscience.1070919) . PMID 12183631 (/pubmed/12183631) . /cgi/pmidlookup? view=long&pmid=12183631. ^ Blake WJ, KAErn M, Cantor CR, Collins JJ (April 2003). "Noise in eukaryotic gene expression" (/abl/pdf/blake2003.pdf? file=/nature/journal/v422/n6932/full/nature01546_fs.html&content_filetype=PDF) . Nature 422 (6932): 633–7. doi:10.1038/nature01546 (/10.1038%2Fnature01546) . PMID 12687005 (/pubmed/12687005) . /abl/pdf/blake2003.pdf? file=/nature/journal/v422/n6932/full/nature01546_fs.html&content_filetype=PDF. ^ Arkin A, Ross J, McAdams HH (August 1998). "Stochastic kinetic analysis of developmental pathway bifurcation in phage lambda-infected Escherichia coli cells" (/articlerender.fcgi? tool=pmcentrez&artid=1460268) . Genetics 149 (4): 1633–48. PMC 1460268 (/articlerender.fcgi?tool=pmcentrez&artid=1460268) . PMID 9691025 (/pubmed/9691025) . /articlerender.fcgi? tool=pmcentrez&artid=1460268. ^ Raser JM, O'Shea EK (September 2005). "Noise in gene expression: origins, consequences, and control" (/cgi/pmidlookup?view=long&pmid=16179466) . Science 309 (5743): 2010–3. doi:10.1126/science.1105891 (/10.1126%2Fscience.1105891) . PMC 1360161 (/articlerender.fcgi?tool=pmcentrez&artid=1360161) . PMID 16179466 (/pubmed/16179466) . /cgi/pmidlookup? view=long&pmid=16179466. ^ Elowitz MB, Leibler S (January 2000). "A synthetic oscillatory network of transcriptional regulators". Nature 403 (6767): 335–8. doi:10.1038/35002125 (/10.1038%2F35002125) . PMID 10659856 (/pubmed/10659856) . ^ Gardner TS, Cantor CR, Collins JJ (January 2000). "Construction of a genetic toggle switch in Escherichia coli". Nature 403 (6767): 339–42. doi:10.1038/35002131 (/10.1038%2F35002131) . PMID 10659857 (/pubmed/10659857) . ^ Gillespie DT (1976). "A general method for numerically simulating the stochastic time evolution of coupled chemical reactions". J. Comput. Phys. 22: 403–34. doi:10.1016/0021-9991(76)90041-3 (/10.1016% 2F0021-9991%2876%2990041-3) . ^ Roussel MR, Zhu R (November 2006). "Validation of an algorithm for delay stochastic simulation of transcription and translation in prokaryotic gene expression" (/1478-3967/3/274) . Phys Biol 3 (4): 274–84. doi:10.1088/1478-3975/3/4/005 (/10.1088%2F1478-3975%2F3%2F4%2F005) . PMID 17200603 (/pubmed/17200603) . /1478-3967/3/274. ^ Ribeiro A, Zhu R, Kauffman SA (November 2006). "A general modeling strategy for gene regulatory networks with stochastic dynamics". J. Comput. Biol. 13 (9): 1630–9. doi:10.1089/cmb.2006.13.1630 (/10.1089%2Fcmb.2006.13.1630) . PMID 17147485 (/pubmed/17147485) . ^ Ribeiro AS, Lloyd-Price J (March 2007). "SGN Sim, a stochastic genetic networks simulator" (/cgi/pmidlookup?view=long&pmid=17267430) . Bioinformatics 23 (6): 777–9. doi:10.1093/bioinformatics/btm004 (/10.1093%2Fbioinformatics%2Fbtm004) . PMID 17267430 (/pubmed/17267430) . /cgi/pmidlookup?view=long&pmid=17267430.22. ^ Kaznessis YN (2007). "Models for synthetic biology" (/1752-0509/1/47) . BMC Syst Biol 1: 47. doi:10.1186/1752-0509-1-47 (/10.1186%2F1752-0509-1-47) . PMC 2194732 (/articlerender.fcgi?tool=pmcentrez&artid=2194732) . PMID 17986347 (/pubmed/17986347) . /1752-0509/1/47. 23. ^ "The DREAM Project" (/dream/index.php/The_DREAM_Project) . Columbia University Center for Multiscale Analysis Genomic and Cellular Networks (MAGNet). /dream/index.php/The_DREAM_Project. 24. ^ Gustafsson M, Hörnquist M (2010). "Gene Expression Prediction by Soft Integration and the Elastic Net—Best Performance of the DREAM3 Gene Expression Challenge" (/article/info%3Adoi% 2F10.1371%2Fjournal.pone.0009134) . PLoS ONE 5 (2). /article/info%3Adoi%2F10.1371% 2Fjournal.pone.0009134. 25. ^ Smith MR, Clement M, Martinez T, Snell Q (2010). "Time Series Gene Expression Prediction using Neural Networks with Hidden Layers" (/viewdoc/download? doi=10.1.1.173.4686&rep=rep1&type=pdf) . Proceedings of the 7th Biotechnology and Bioinformatics Symposium (BIOT 2010). pp. 67-69. /viewdoc/download?doi=10.1.1.173.4686&rep=rep1&type=pdf. 26. ^ a b Leclerc, RD (August 2008). "[/msb/journal/v4/n1/full/msb200852.html Survival of the sparsest: robust gene networks are parsimonious (/articlerender.fcgi? tool=pmcentrez&artid=2538912) "]. Mol Syst Biol. 4 (213): 213. doi:10.1038/msb.2008.52 (/10.1038%2Fmsb.2008.52) . PMC 2538912 (/articlerender.fcgi? tool=pmcentrez&artid=2538912) . PMID 18682703 (/pubmed/18682703) . /articlerender.fcgi?tool=pmcentrez&artid=2538912.References■ Bolouri, Hamid; Bower, James M. (2001). Computational modeling of genetic and biochemical networks. Cambridge, Mass: MIT Press. ISBN 0-262-02481-0. ■ Kauffman SA (1969). "Metabolic stability and epigenesis in randomly constructed genetic nets". J. Theoret. Biol. 22: 434–67.External links■ Gene Regulatory Networks (/science/generegulatorynetwork.shtml) — Short introduction ■ Open source web service for GRN analysis (/) ■ BIB: Yeast Biological Interaction Browser (/bio) ■ Graphical Gaussian models for genome data (/notes/ggm.html) — Inference of gene association networks with GGMs ■ A bibliography on learning causal networks of gene interactions (http://www.molgen.mpg.de/~markowet/docs/network-bib.pdf) - regularly updated, contains hundreds of links to papers from bioinformatics, statistics, machine learning. ■ http://mips.gsf.de/proj/biorel/ BIOREL is a web-based resource for quantitative estimation of the gene network bias in relation to available database information about gene activity/function/properties/associations/interactio. ■ Evolving Biological Clocks using Genetic Regulatory Networks (http://panmental.de/GRNclocks) Information page with model source code and Java applet. ■ Engineered Gene Networks (/abl) ■ Tutorial: Genetic Algorithms and their Application to the Artificial Evolution of Genetic Regulatory Networks (http://panmental.de/ICSBtut/) ■ BEN: a web-based resource for exploring the connections between genes, diseases, and other biomedical entities (/) Retrieved from "/wiki/Gene_regulatory_network" Categories: Gene expression | Networks | Systems biology ■ This page was last modified on 19 March 2011 at 16:15. ■ Text is available under the Creative Commons Attribution-ShareAlike License; additional terms may apply. See Terms of Use for details. Wikipedia® is a registered trademark of the Wikimedia Foundation, Inc., a non-profit organization.。
生物分子网络 第4章 基因调控网络及其模型
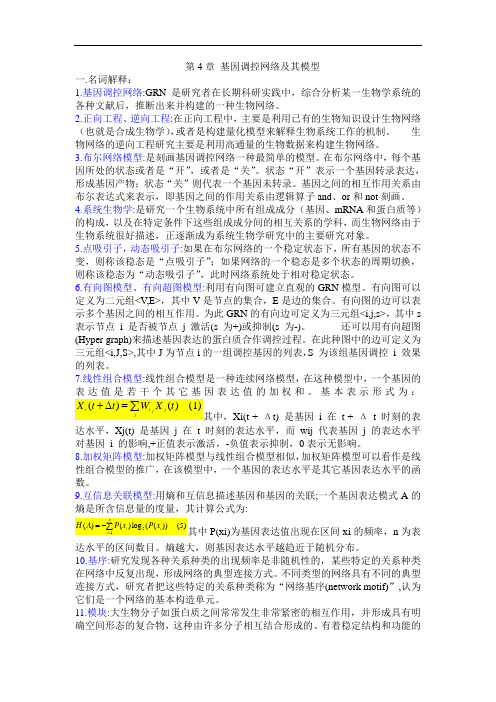
第4章 基因调控网络及其模型一.名词解释:1.基因调控网络:GRN 是研究者在长期科研实践中,综合分析某一生物学系统的各种文献后,推断出来并构建的一种生物网络。
2.正向工程、逆向工程:在正向工程中,主要是利用已有的生物知识设计生物网络(也就是合成生物学),或者是构建量化模型来解释生物系统工作的机制。
生物网络的逆向工程研究主要是利用高通量的生物数据来构建生物网络。
3.布尔网络模型:是刻画基因调控网络一种最简单的模型。
在布尔网络中,每个基因所处的状态或者是“开”,或者是“关”。
状态“开”表示一个基因转录表达,形成基因产物;状态“关”则代表一个基因未转录。
基因之间的相互作用关系由布尔表达式来表示,即基因之间的作用关系由逻辑算子and 、or 和not 刻画。
4.系统生物学:是研究一个生物系统中所有组成成分(基因、mRNA 和蛋白质等)的构成,以及在特定条件下这些组成成分间的相互关系的学科,而生物网络由于生物系统很好描述,正逐渐成为系统生物学研究中的主要研究对象。
5.点吸引子,动态吸引子:如果在布尔网络的一个稳定状态下,所有基因的状态不变,则称该稳态是“点吸引子”;如果网络的一个稳态是多个状态的周期切换,则称该稳态为“动态吸引子”,此时网络系统处于相对稳定状态。
6.有向图模型、有向超图模型:利用有向图可建立直观的GRN 模型。
有向图可以定义为二元组<V ,E>,其中V 是节点的集合,E 是边的集合。
有向图的边可以表示多个基因之间的相互作用。
为此GRN 的有向边可定义为三元组<i,j,s>,其中s 表示节点i 是否被节点j 激活(s 为+)或抑制(s 为-)。
还可以用有向超图(Hyper graph)来描述基因表达的蛋白质合作调控过程。
在此种图中的边可定义为三元组<i,J,S>,其中J 为节点i 的一组调控基因的列表,S 为该组基因调控 i 效果的列表。
7.线性组合模型:线性组合模型是一种连续网络模型,在这种模型中,一个基因的表达值是若干个其它基因表达值的加权和。
基因转录调控的机制和调控网络
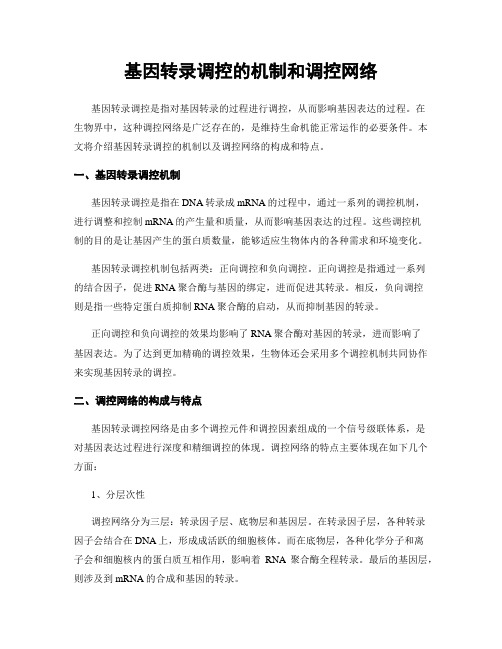
基因转录调控的机制和调控网络基因转录调控是指对基因转录的过程进行调控,从而影响基因表达的过程。
在生物界中,这种调控网络是广泛存在的,是维持生命机能正常运作的必要条件。
本文将介绍基因转录调控的机制以及调控网络的构成和特点。
一、基因转录调控机制基因转录调控是指在DNA转录成mRNA的过程中,通过一系列的调控机制,进行调整和控制mRNA的产生量和质量,从而影响基因表达的过程。
这些调控机制的目的是让基因产生的蛋白质数量,能够适应生物体内的各种需求和环境变化。
基因转录调控机制包括两类:正向调控和负向调控。
正向调控是指通过一系列的结合因子,促进RNA聚合酶与基因的绑定,进而促进其转录。
相反,负向调控则是指一些特定蛋白质抑制RNA聚合酶的启动,从而抑制基因的转录。
正向调控和负向调控的效果均影响了RNA聚合酶对基因的转录,进而影响了基因表达。
为了达到更加精确的调控效果,生物体还会采用多个调控机制共同协作来实现基因转录的调控。
二、调控网络的构成与特点基因转录调控网络是由多个调控元件和调控因素组成的一个信号级联体系,是对基因表达过程进行深度和精细调控的体现。
调控网络的特点主要体现在如下几个方面:1、分层次性调控网络分为三层:转录因子层、底物层和基因层。
在转录因子层,各种转录因子会结合在DNA上,形成成活跃的细胞核体。
而在底物层,各种化学分子和离子会和细胞核内的蛋白质互相作用,影响着RNA聚合酶全程转录。
最后的基因层,则涉及到mRNA的合成和基因的转录。
2、灵敏性和准确性调控网络能够根据外界环境和内部信息,调控基因的表达水平,使得生物体的形态结构、生理功能等方面得到了准确和灵敏的调控。
例如,当生物体感受到外部机械或化学刺激时,会立刻响应并启动相关的调控网络。
3、互动性和可塑性调控网络通过各种调控元件和调控因素之间的复杂相互作用,形成了复杂的基因转录调控网。
这些元件和因素之间相互作用的程度和方式不同,使得调控网络具有较高的可塑性和互动性。
- 1、下载文档前请自行甄别文档内容的完整性,平台不提供额外的编辑、内容补充、找答案等附加服务。
- 2、"仅部分预览"的文档,不可在线预览部分如存在完整性等问题,可反馈申请退款(可完整预览的文档不适用该条件!)。
- 3、如文档侵犯您的权益,请联系客服反馈,我们会尽快为您处理(人工客服工作时间:9:00-18:30)。
解微分方程
₪ 一般采用四阶定步长Runge-Kutta算法计算解常 微分方程。该算法结构简单。求解方法如下: ₪ 首先定义四个附加变量: k1 = f(x(t),t) k2 = f(x(t)+hk1/2,t+h/2) k3 = f(x(t)+hk2/2,t+h/2) k4 = f(x(t)+hk3,t+h/2) 其中:h为计算步长,在实际应用中该步长是一 个常数,这样由四阶Runge-Kutta算法可以由当 前状态变量x(t)的值求解出下个状态变量x(t +1) 的值 x(t +1) = x(t) + h(k1 /6 + k2 /3 + k3 /3 + k4 /6)
4
₪ 例如, dt f ( x , x ,..., x )(i 1, 2,..., n) ,其中xi表示第i个基因的表达水 平,n表示基因调控网络中的基因数。所以主要的工作就 是对于右端任意的微分方程系统的演化。
i 1 2 n
dxi (t )
₪ 关于这方面的研究主要集中在两个方面: (1). 微分方程结构的优化; (2). 微分方程系数和常数的优化。 研究表明:微分方程演化的越准确,得到的生物网络模 型越精确,越接近目标网络模型 。
3
研究现状
₪ 当前对于基因调控网络研究的方法一般包括以下两个步 骤: 1. 模型选择 线性模型、布尔模型、贝叶斯网络模型、微分方程模 型、随机方程模型等 2. 模型构建或优化的算法 梯度下降法、支持向量机、贪心算法、遗传算法、遗 传编程等 近年来,微分方程模型逐渐成为系统生物学领域中的热 点。微分方程模型是以其它基因表达水平和外部环境的 因素组成的函数来描述基因表达的变化,可以充分模拟 基因调控网络的动态行为。相比较其他模型,微分方程 模型非常强大灵活,利于研究基因网络中的复杂关系。
是
是
否
选择一定数量的 个体参数优化
结束
8
分裂
₪ 采用分裂思想,可以大量的减少方程组得搜索空 间。假设一个微分方程组的方程最大表达式长度 为c,则假如有一个变量,则搜索空间为1c,若 有两个变量,则搜索空间为2c× 2c=22c。对于n个 变量的微分方程组,则搜索空间为nnc。若采用 分裂法,即每个微分方程分别优化,则搜索空间 变为nc+1,明显变小。 ₪ 单独优化每一个微分方程,在结构和系数编码、 优化上都与前面一样。只是在求每个染色体适应 值时,解微分方程需要做一下改动。
2
₪ 基因调控网络本质上是一个连续而复杂的动态系 统,即复杂的动力系统网络。建模时为了简化求 解的需要,往往对其进行简化。基因调控网络有 许多特性,如复杂性(即基因网络包含着不同层 次的错综复杂的物质、关系和功能结构,基因的 复杂性还体现在基因的组合性质方面)、稳定性 (基因网络系统能够通过自动调节达到稳定)、可 进化性和有限连通性等。
₪ 统计每个方程对应系数的个数和其对应的位置, 把方程组的系数组合成一个粒子,通过粒子群优 化算法(PSO),得到好的系数。
1: x1 2: x3 3: + 1,1 4: * 1,3 5: 1 6: - 2,5 P1 P3 P2
7
P4
P5
流程图
初始化种群 评价个体适应值 否
代数是否达到要求
是否达到要求 保留好的, k1 k2 k3 k4 x(t) 为n 维向量,函数组f = {f1, f2, …, fn}。 ₪ 由于采用分裂思想,每个方程单独优化。假设优 化第一个微分方程(下图为优化方程的训练数 据),
11
₪ 生成的种群只代表第一个微分方程,即函数组中只有f1 已知,只能计算出k1 k2 k3 k4 中的第一个元素的值,其他 元素的值未知。利用训练数据,在根据当前状态变量x(t) 的值求解出下个状态变量x(t +1)的值时, k1 k4 中的其他 元素可以分别取x(t), x(t +1)相应的值,但k2 k3的确定出 现了问题。 ₪ 对于这种情况,我们采用一种近似的处理方法。即在根 据当前状态变量x(t)的值求解出下个状态变量x(t +1)的值 时, k2 k3 向量中的其他元素一律采用xi = [x(t +1)+ x(t)]/2。由于采用这中近似四阶定步长Runge-Kutta算法, 不可避免有些误差,通过实验,用这种方法,在大幅度 提高减少搜索空间的同时,又对效果影响不大。 ₪ 但在研究过程中,发现如果采用线性插值的方法确定 k2 k3 向量中的其他元素,误差会小一些。即在确定k2中的
5
研究过程
₪ 基于多表达式程序设计(MEP)的一些优点,如线 性的染色体结构、实现简单、一个染色体包含多 个基因等,我们采用MEP优化微分方程的结构, 粒子群优化算法(PSO)优化方程中的系数和常数。 ₪ 例如,我们选取F = {+, -, *},T = {X1,...,Xn ,1}(1 表示常数)。对于含三个未知数的微分方程组
6
₪ 可表示一组染色体形式(E1,E6,E6):
dx1/dt 1: x1 2: x2 3: + 1,1 4: * 1,3 5: +1,2 6: - 2,5 dx2/dt 1: x1 2: x2 3: + 1,2 4: - 1,3 5: x3 6: + 3,5 dx3/dt 1: x1 2: x2 3: + 1,2 4: - 1,2 5: x3 6: + 3,5
基因调控网络
杨斌
2007 济南
1
背景
₪ 基因表达(gene expression)是指细胞在生命过程中, 把储存在DNA顺序中遗传信息经过转录和翻译,转 变成具有生物活性的蛋白质分子。生物体内的各种 功能蛋白质和酶都是同相应的结构基因编码的。 ₪ 一个基因的表达受其他基因的影响,而这个基因又 影响其他基因的表达,这种相互影响相互制约的关 系构成了复杂的基因表达调控网络。更一般些,几 乎所有的细胞活动都被基因网络所控制。对系统科 学的研究促使生物学家以系统的观点认识高度复杂 的生命现象。生命是存储并加工信息的复杂系统, 从而,孤立地研究单个基因及其表达往往不能确切 地反映生命现象本身的内在规律。因此,科学家们 开始从复杂系统的角度研究基因网络。