P2P流量识别
P2P流量识别技术的研究
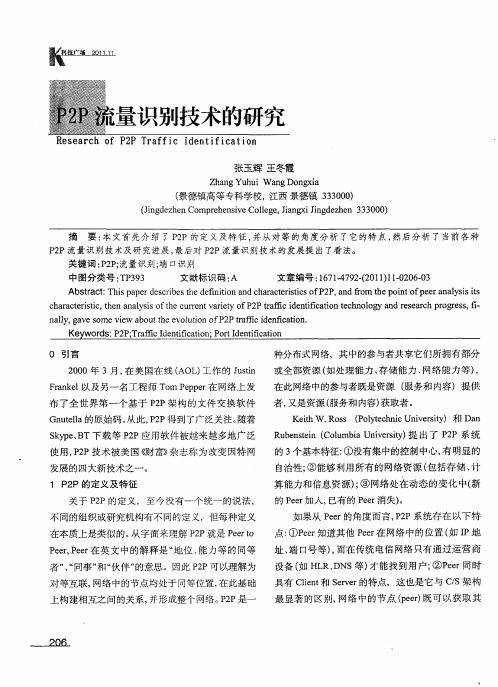
如果从 Pe 的角度而言,2 er P P系统存在 以下特 点: er @Pe 知道其他 P e 在网络 中的位置 ( I 地 er 如 I ) 址 、 口号等) 而在传统 电信网络只有通过运营商 端 , 设备 ( H R、 N 如 L D S等) 才能找到用户; er  ̄P e 同时
具有 Ci t Sre 的特 点 ,这 也是 它 与 CS架 构 ln 和 evr e / 最 显著 的 区别 , 络 中的 节 点 (er既 可 以 获取 其 网 pe)
P P流 量识别 技 术及 研 究进 展 , 后对 P P流 量识别 技术 的发 展提 出了看 法 。 2 最 2
关 键词 : 2 ; 量识 别: 口识别 P P流 端
中图分 类 号 :P 9 T 33
文献标 识 .7 2( l)1 260 2
K y r s P PTr伍 CIe t c to ; o t d n i c t n e wo d : 2 ; a ni ain P r I e t ai d i f i f o
0 引 言
种分布式网络,其中的参与者共享它们所拥有部分
或全 部 资源 ( 处 理 能力 、 储 能力 、 络 能力 等 ) 如 存 网 ,
之 j( 二 如互 联 网) ④ 多个 P e 可 以组成 为一 个 Pe ; er er 组 , er Pe 的这 种 属 性一 方 面 可 以符 合 人类 社 会 的群 组特性, 同时也降低了技术实现难度 。
Ab ta t T i p p r ec ie ed f io n h r ceit s f 2 , n o tep it f e ra ay i i s r c: h s a e sr s h e nt n a dc aa tr i P P a df m on p e n lss t d b t i i sc o r h o s
带背景流的P2P流量识别技术研究

带背景流的P2P流量识别技术研究欧阳玲;宋克【摘要】The Diversity and complexity of Peer hosts in P2P application systems and P2P application traffic make P2P traffic identification approach based on only typical feature inaccurate. We propose a novel multi-phase identification method to reveal P2P traffic from traffic aggregation. Our method is based on a set of heuristics derived from the robust properties of P2P traffic. Experiments indicate the classification accuracy of our proposed method can reach 99. 7%, while the false positive is lower than 0. 3%.%针对P2P (peer- to peer,对等体网络)应用系统中对等体主机的行为特征与P2P业务流量特征多样化、复杂化,使得单纯利用一种典型特征的P2P流量分类技术的识别精度不高的问题,提出了一种新的P2P流量多阶段识别方法;该方法根据P2P应用流量的一系列固有特征,可以从聚合网络流中识别P2P流量;通过实验表明,该方法P2P流识别精度可达99.7%,同时错误分类精度0.3%.【期刊名称】《计算机测量与控制》【年(卷),期】2011(019)010【总页数】3页(P2562-2563,2570)【关键词】P2P;聚合流量;典型特征;流量分类【作者】欧阳玲;宋克【作者单位】中原工学院,河南郑州 450052;国家数字交换系统工程技术研究中心,河南郑州 450005【正文语种】中文【中图分类】TP393.00 引言近几年来,P2P作为一项全新的Internet技术得到飞速发展,不断涌现出新型的P2P协议及应用软件,如国外的BitT-orrent、eDonkey、Skype,国内的迅雷、PPLive、QQ等,P2P给网民带来方便快捷的同时,已成为当前网络带宽的“杀手级”应用,其上传/下载比趋近于1,造成传统xDSL网络的上行链路极易拥塞,并且存在许多安全隐患。
P2P网络流量识别技术的研究

1引 言
Itme 的 迅速 发 展带 动 了 网 络 带 宽 的 快 速 增长 , 大 的 ne t 广
网 络 使 用 者 喜 欢 使 用 各 类 P P和 点 对 服 务 器 技 术 2 ( ert P e o
对 于 以 上情 况 , 制 P S 控 2 P这 类 应 用 流 量 , 决 P S 解 2 P带 来 的 网络 拥 塞 。最 简 单 的方 法就 是 禁 止 P P和 P S 2 2 P的应 用 . 但 目前 P P和 P S 2 2 P的 应 用 已经 被 非 常 多 的 用 户所 喜 爱 。 一旦 禁
tef w. is ae ec b sapia o d terltdtc n lge nP e ev r e r P P , a s fsv r pc rfc h o Th p rd sr e p l t na h eae eh oo is e rt S re&P e ( 2 ) a l i o ea t ia ta l p i ci n o o n ys e l y l i
【 关键 词】流 量 识 别 P P 深度 包检 测 2 连 接 模 式
中 图分 类 号 : P 9 文 献 标 识码 : 文章 编 号 :0 8 1 3 ( 0 0 0 — 0 3 T 33 A 10 — 7 9 2 1 )6 6 —
The S v y o P a c I ntfc to e ha im ur e fP2 Tr f de i ai e eo me to ne ta d bo d a d n t rs P e o P e (2 )a d P S p L a o saei- sr c : t te rpd d v lp n fItme n ra b n ewok , e rt er P P n 2 P a p ct n r h i n
基于BP神经网络聚类算法的P2P流量识别
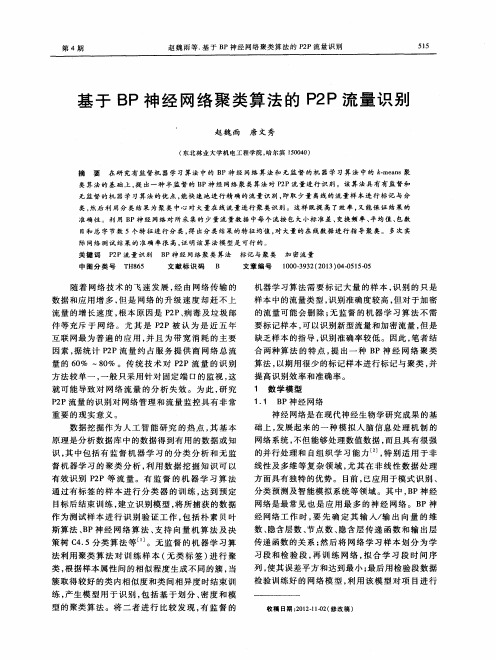
习段 和检 验 段 , 再 训 练 网络 , 拟 合 学 习段 时 间 序
列, 使其 误 差平 方和 达到最 小 ; 最 后用 检验 段数 据
数据 挖 掘作 为 人 工 智 能 研究 的 热 点 , 其 基 本 原 理 是分 析数 据 库 中的数 据得 到有 用 的数据 或知
识, 其 中包 括 有 监督 机 器 学 习 的 分类 分 析 和 无 监 督 机 器学 习 的 聚类 分 析 , 利用 数 据 挖 掘 知 识 可 以 有 效识 别 P 2 P等 流 量 。有 监 督 的 机 器 学 习 算 法
策树 c 4 . 5分类 算 法 等 … 。无 监督 的机 器 学 习算
法 利 用聚类 算 法 对 训 练 样 本 ( 无类标 签 ) 进 行 聚 类, 根 据样 本属 性 间 的相 似程度 生成 不 同 的簇 , 当 簇 取得 较好 的类 内相似 度 和类 间相 异度 时结 束 训 练, 产 生模 型 用 于 识 别 , 包括基于划分、 密 度 和 模
无监 督 的 机 嚣 学 习 算 法 的优 点 , 能 快速 地进 行 精 确 的 流 量 识 别 , 即取 少 量 离线 的 流 量样 本 进 行 标 记 与 分
类. 然 后 利 用 分 类 结 果 为 聚 类 中心 对 大 量在 线 流 量 进 行 聚 类 识 别 。 这 样 既 提 高 了效 率 , 又 能 保 证 结 果 的 准确性。利用 B P神 经 网 络 对 所 采 集 的 少 量 流 量 数 据 中每 个 流按 包 大 小标 准差 、 变换 频 率 、 平 均值 、 包数 目和 总 字 节 数 5个 特 征 进 行 分 类 , 得 出 分 类 结 果 的 特 征 均值 , 对 大量 的在 线数 据 进 行 指 导 聚 类 。 多次 实 际 网络 测 试 结 果 的 准 确 率 很 高 , 证 明 该 算 法 模 型 是 可行 的 关键词 P 2 P流 量 识 别 B P神 经 网 络 聚 类 算 法 标 记 与 聚 类 加 密流 量
P2P流量识别和管控技术分析

流 量 识 别 和 管控
技术分析
_ 马 少 武 唐 雄 燕 姜智 峰 张 辉
_ 中国 网 通 集 团研 究 院
摘要 : 基于
P 2P
北 京 市 西 城 区 金 融 大街 2
l
号
10 0 0 3 2
模 式 的业 务 和 应 用 给 互 联 网 的发 展 带 来 了 巨 大 影 响
,
,
本 文 在 简 要分 析 了 现 网
-
特 征 字 如 果在数 据 包 的 相 应 位 置 能够找 到 这 些 特
征 字就 可 以 判 断 数据 包属 于 哪 种 类 型 的 网 络应 用
由此 可 以 大 致 判 别 出
。
M G C P
等
。
数 据 流 所 对应 的 应 用 业 务 类 型 能够 发 现 未 知
P 2P
此 方法 的 优 点 是
以 上 P 2 P 业 务还 可 以 分 为 基 于 T C P 和 U D P 的
P 2 P 业 务两 大 类
。 『 I
。
应用
,
具 有对 新 P 2 P 应 用 的 感
。
a
tu r e
特 征 值进行应
,
k
t
In
p e c tio n
)
用 层 流量 识 别
网 络应 用 的 数 据 包 中
一
各种 应 用 在
a
常用 端
用
一
口 检 测法 即利 用 P 2 P
应 用 发 展 的 初期使
不 同的 数据 包 位 置 都有
,
些 特有 的 固 定 的 S i g n
tu
r e
些 固 定 端 口 进行控制 和数 据 的 通 信 原 理 进 行检 如早 期 e D o n k
基于流量与行为特征的P2P流量识别模型
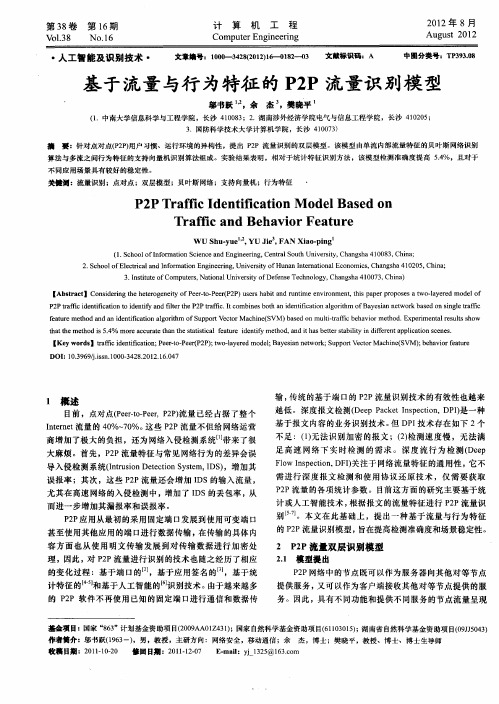
V0 . 1 38
・
计
算
机
工
程
21 0 2年 8月
A u us 01 g t2 2
N O.6 1
Co p t rEng n e i m ue i e rng
人工 智能 及识 别技 术 ・
文章缡号;1 0_ 2( 1 l _ 8—0 0 —3 8 02 6 o 2_ 文献标识码: 0 4 2 )— 1 3 A
[ ywo d l t fcie t ct n P e- -er 2 )t - y rdmo e; a ei ewok S p o co c ieS Ke rs ri ni ai ; er oP e( P;wol ee d lB ysa n t r; u p rVetr hn(VM)bh vo aue a d f o i t・ P - a n t Ma ; e air etr f
2 S h o f e ti a nd I f r a in E i e rn , i e st fHu n I t r a i n l o o c , a g h 0 5 Ch n ; . c o l Elc rc l o a n o m to ng n e i g Un v r i o y na n e n t a o Ec n mi ห้องสมุดไป่ตู้ Ch n s a41 20 , i a
W U Shu・ yue’, U e , Y Ji FA N a pi Xi o- ng ( . c o fIf r ainS inc n gn e ig Ce r l uhUnv ri Ch n s a41 0 3 Chn ; 1 S ho l n om to ce ea dEn ie rn , nta t iest o So y, a g h 0 8 , ia
基于聚类分析的P2P流量识别

P2 r f i d ntfc to a e n c u t r a a y i P t a fc i e i ia i n b s d o l s e n l s s
ZH AO i Ka ,SH ICh ng q o g,ZH ANG — r c :Cur e l ta t r nty P2P t a fc i ntfc to o l m sbe n a f usa ti e e ui r fi de iia in pr b e ha e oc nd i s a pr r q — st o fe tv a ge e to P ta fc i r r t n g h e wo k be t r Tr di ief r e f c i e m na m n fP2 r fi n o de o ma a e t e n t r t e . a — ton li e tfc to t o r o ge f e tv n hi a e e l t r a a y i p— i a d n iia i n me h ds a e no l n r e f c i e a d t s p p r us sc us e n l ss a p o c o i e iy P2 ta fc r a h t d ntf P r fi.Gi e he c r c e itc fP2 ta fc,t l t rng f a ur v n t ha a t rs iso P r fi hec us e i e t e te s d fn d,by c lul tng t e d t r e i e i e a c a i h a a whih i c nn d fom he ne wo k,we o an t c s s a e r t t r bt i he
基于神经网络集成的P2P流量识别研究

P Pf w dtc o d l sdv l e yuigcr l i —ae etr e c o ( F ) a o tm t 2 o e t nmo e i ee p db s or a o b sdfa esl t n C S l rh o l ei o n e tn u ei gi
第3 0卷
第 3期
南 京
邮 电 大 学 学 报
( 自 然 科
学 版
)
Vo . No. 130 3
21 0 0年 6月
Jun l f a igU i r t o ot adT l o muia os N t a Sine ora o m n nv sy f s n e cm nct n( a rl cec ) N e i P s e i u
Re e r h o 2P Tr f c I e tfc to s d o s a c fP a i d n i a i n Ba e n i Ne r lNe wo k En e b e u a t r s m l
X U e , ANG uo p ng W ANG H W S . i , Ru. h n , c ua ZHAo n Da
e ta tP x r c 2P o c a a t rsis.a tlzn i ns mb e n u a ewo k y d n mi i h e n e r — l f w h r ce tc i nd u iii g sx e e l e r ln t r sb y a c weg td i tg a to t d. T r ug e pe me tl c mp rs n bewe n h s r p s d mo e a ta iin l i n meho ho h x r i n a o a o t e t i p o o e d l nd r d t a meho , i o t ds
- 1、下载文档前请自行甄别文档内容的完整性,平台不提供额外的编辑、内容补充、找答案等附加服务。
- 2、"仅部分预览"的文档,不可在线预览部分如存在完整性等问题,可反馈申请退款(可完整预览的文档不适用该条件!)。
- 3、如文档侵犯您的权益,请联系客服反馈,我们会尽快为您处理(人工客服工作时间:9:00-18:30)。
Research of P2P Traffic Identification Based on BP Neural NetworkShen FukeComputer ScienceDepartment, East China NormalUniversity Shanghai 200062, China fkshen@Change PanComputer ScienceDepartment,East China NormalUniversityShanghai 200062, Chinapchang @Ren XiaoliComputer ScienceDepartment,East China NormalUniversityShanghai 200062, Chinaxlren@AbstractToday’s P2P application is a big challenge to network traffic workload. In contrast to first generation P2P network s which used well-defined port numbers, current P2P applications have ability to disguise their existence through the use of arbitrary ports. Our goal is to give out a new approach for P2P traffic identification based BP Neural Network, and without relying on k eyword matching. This article introduces BP algorithm, analyzes the characters of P2P traffic, gives out the BP network based on connection patterns of P2P networks. The trained BPNN was applied as a P2P traffic identifier, which can be used to distinguish any k ind of P2P applications from non-P2P applications. This feasible solution has many advantages in P2P traffic identification. We believe our approach is the first method for characterizing P2P traffic using network dynamics based on BP network rather than any user payload.1. IntrodoctionToday’s Peer-to-Peer (P2P) application is a big challenge to Internet traffic workload and P2P represents a formidable component of campus network traffic. Network traffic identification on campus network exit link or backbone node can provide support to network resource planning and traffic control [5]. In contrast to first generation P2P networks which used well-defined port numbers, current P2P applications have ability to disguise their existence through the use of arbitrary ports [3]. Most existing traffic identification technology is based on the ports number or payload keyword matching named Deep Packet Inspection (DPI) [8]. Not only do most P2P applications now operate on top of nonstandard, custom-designed proprietary protocols, but also current P2P clients can easily operate on any port number, even HTTP, FTP, or other popular app’s port [9]. These circumstances portend a frustrating conclusion: robust identification of P2P traffic is only possible by inspecting user payload [10]. Yet packet payload capture and analysis poses a set of often insurmountable methodological landmines: legal, privacy, technical, efficient, etc. Further obfuscating workload characterization attempt is the increasing tendency of P2P protocols to support payload encryption. Indeed, the frequency with which P2P protocols are introduced and/or upgraded renders packet payload analysis not only impractical but also glaringly inefficient.P2P flow inspection based traffic flow characteristic can be viewed as a Pattern Recognition problem which has two output, one is Yes and another is No [11]. So, Neural Network technology can be usedin P2P traffic inspection based traffic characteristic. In this paper we develop a BP neural network method to identify P2P flows at the campus exit link, based on flow connection patterns of P2P traffic, and without depending on packet payload. The significance of our algorithm lies in its ability to identify P2P protocols without relying on their underlying format, and we can identify previously unknown P2P protocols.2. BP Neural Network (BPNN)The attraction of neural networks is that they are best suited to solving the problems that are the most difficult to solve by traditional computational methods. BP Neural Network is a multi-tier network which weight value is trained by non-linear differentiable function. BPNN’s structure is simple and fictile, It is widely used in pattern recognition, voice recognition, image processing, Compression, Data mining, etc.Suppose we have P training samples, that means we have P input/output pairs(I p,T p), p=1,2,…P, and:I p =(i p1,Ăi pm )T ,(input vector), T p =(t p1,Ăt pn )T ,(output vector),The m is the dimension number of input vector, and n is the dimension number of output vector.O p =(o p1,…,o pn )T is the actual output vector corresponding to the input vector I p . W ij is the weight value of i th (i=1,…,n) output component corresponding to the j th (j=1,…,m) input component. ǻW ij denotes modification value of increase by degrees one time, so one time increasing by degrees formula is:W ij +ǻW ij =>W ij (1)We can explain formula (1) with: put the W ij ’s modification value ǻW ij to W ij and get a new W ij . And ǻW ij =¦Ș(t pi -o pi )i pj =Șįpi i pj , (2)Pp 1¦Pp 1įpi =t pi -o piȘ is learning efficiency. Formula (1) and (2) are named Delta learning rule. A BP network learns by example, that is, we must provide a learning set that consists of some input examples and the known-correct output for each case. So, we use these input-output examples to show the network what type of behavior is expected, and the BP algorithm allows the network to adapt. When the BPNN is trained, compare the network Resulting output with desired output gradually, and change the connection weight value according to the Delta learning rule. The cycle is repeated until the overall error value drops below some pre-determined threshold.For BP network, a continuous function in any closed interval can be asymptotic by single hidden layer BPNN. One of the easiest forms of BPNN topology is made of three layers: one input layer (the inputs of our network), one hidden layer, one output layer (the outputs of our network). All neurons from one layer are connected to all neurons in the next layer. The number of input neurons is determined by the number of input class traits, the number of output layer neurons is the number of target class. So, before set up a BP Neural Network, we need determine the traits value of input dataset (the number is m), and the number of output layer nodes. If the BP Neural Network is used as a classifier, and there is n class mode, then the neurons number of output layer is n. The number of hidden layer neurons is related to requirement of problem, the number of input/output layer neurons, etc. Too big hidden layer neurons number can result to long training time, and the error is not always best of all, can result to bad fault-tolerant and can not identify sample unseen before. So, there must be a best hidden layer neurons number, neither very big nor very small. Here is a reference formula of best hidden layer neurons number [13].1n m n +a (3)Thereinto, m is input layer neurons number, n is output layer neurons number, a is a constant between 1 and 10.Another important parameter is the training times, which is the number of input training data set traversing the BP Neural Network. Increasing the training times can improve the veracity of the BPNN model, but also can cost long training time. Decreasing the training times can reduce the veracity of the BPNN model, but also can cost short training time. So, we can choice one between 100 and 300 by experience.Learning efficiency Ș can be chose between 0.1 and 0.9. Lower learning efficiency needs more training iterative loop. Higher learning efficiency can make the BPNN overlay speedily and get a more non-linear result. If the hidden layer neurons number is too small, we can get a higher training error rate, and we must tolerant higher generalization error rate. If the hidden layer neurons number is too big, although training error rate falls, we can also get a higher generalization error rate because of exceeding appropriate value.3. BPNN in P2P Traffic IdentificationBefore traffic identification, we need understand the traffic behavior of different applications, analyze and select the character of different traffic. Table 1 illustrates some character of popular Internet services. From Table 1, we can see, P2P flow is easy to be distinguished from other services except FTP service [7]. One way to distinguish P2P from FTP is to see if user interaction exists. P2P application has 2 states, message associate state and data transfer state, which alternates in a very high frequency. Message associating is a way of user interaction. We can distinguish P2P from FTP by this feature.Character Selection is defined as choosing a best subset from input character set. Character extraction is defined as conversion or integration of original characters. There are 2 characters which can represent user interaction of data flow [6][12]:ƔMean Squared Deviation (MSD) of every flow’s packet sizeWhen a date flow’s packet size is variational and the variation is very big, the mean squared deviation of this flow’s packet size is very big. Although both WWW and P2P service have big MSD, We can distinguish P2P from WWW by flow duration, P2P flow has longer duration than WWW.ƔSwitching Frequency of every flow’s packet size Switching Frequency of P2P flow’s packet size is higher than that of other services.Table 1. Some characters of popular Internet servicesSo, we choose MSD(Mean Squared Deviation of the flow’s packet size), Switching Freq(Switching Frequency of the flow’s packet size), Rates(Average of the flow’s packet size), Packets(packet quantity of the flow ) and Bytes(Total bytes of the flow) as input characters. That means m=5.We inspect the traffic flow, only to identify the flow is P2P or not. So, the output has 2 options, that means n=2.So, we get: Input neurons number m=5, output neurons number n=2, hidden layer neurons number=4, training times epcoh ˙250, learningefficiency Ș=0.7.1n Using MATLAB’s Neural Network toolskit, we analyzed the data flow captured from East China Normal University campus network, we get 250 groups’ data flow samples (Table 2 gives out 8 of them). There are P2P flows and non-P2P flows. Sample 1 to 4 is P2P flow, and sample 5 to 8 is non-P2P. We denote P2P flow with (1,0), denote non-P2P with (0,1), So, Sample 1 to 4’s output is (1,0), and sample 5 to 8’s output is (0,1).Now we have a BPNN. Before we use the BPNN, we must train it. Only after having been trained enough, It can be used as a data flow classifier. In our experiment, we set the satisfactory threshold to be over 98%, that means it can not exceed 5 mis-identifications among 250 training samples. For testing our BPNN, we use 5 computers running FTP and WWW together with 2 of them running BitTorrent and eMule, other 3 of them running eDonkey and Tuotu(supporting encrypted transmission). We get 48875 flows, 2340268packets, 643.7M bytes by sniffer. Through our identification system, we get the number of P2P flow is 2787 while the actual number is 2895, the discovery rate is 96.3%. After 6 experiments in different time slot, we get discovery rate between 93% and 99%. Because this resolution is based on traffic characters, it can identify encrypted and unknown P2P traffic, it has a very good adaptability. We compare our solution with[1],[2],[3], we get comparison table 3. [1] is solution 1 in table 3, based on Deep Packet Inspection. In [1], the claimed discovery rate 99.4% is aimed at known P2P, this solution can not identify unknown and encrypted P2P. [2] is based on network layer and transport layer connection patterns of P2P networks. [3] gives out a solution based on Bayesian Analysis Techniques. From table 3, we can see our solution has advantages in flexibility, adaptability and discovery rate.4. ConclusionThe application layer signature is not operational because it must enumerate all possible signatures and it is useful to unknown P2P applications. The trained BPNN is based on P2P traffic characters rather than any user payload, which can be used to distinguish any kind of P2P applications from non-P2P applications. This solution is independent of the type of P2P application, port number, and transmission encrypted or not. So it has good adaptability. Because our experiment is offline, what we will do next step is to test this solution online, which needsService Duration Average Rate Count of Bytes HTTPshorthighFrom lower to higher VPN long lowhigh G ames long lowhigh Streaming longmidhighTelnet long low mid FTP/P2PlongMid to highhighhigher performance. We also need more data to verify whether the five characters are enough toidentify unknown P2P.Table 2. Samples of flow captured from ECNUTable 3. Comparison with other solutionSolution Solution Description Encrypted or notForged portDiscovery Rate Solution 1 Based on packet payload NoYes99.4%Solution 2 Based on traffic behavior of network layer and transport layerYes Yes 95%Solution 3 Using Bayesian Analysis Techniques and FCBFYes Yes 55.18% Our SolutionBased on traffic charactersYesYes96%5. References[1]S. Sen, O.Spatscheck, D.Wang. Accurate, Scalable In-Network Identification of P2P traffic Using Application Signatures. May 2004[2]A. W. Moore, K. Papgiannaki. Toward the Accurate Identification of Network Applications. March 2005[3]T. Karagiannis, A.Broido, M.Faloutsos. Transport Layer Identification of P2P Traffic. October 2004[4]A. W. Moore, D.Zuev. Internet Traffic Classification Using Bayesian Analysis Techniques. June 2005[5]/adsl2006/adsl2006/p2p.html[6] Li JiangTao, Jiang YongLing <P2P Traffic Identification and management technology> Telecom Science,2005 49(3).[7] /infocus/1843/1~3 [8]/doc.aspx?nid=58&language=sc&mid=14&smid=58 [9] [10]Sample IDMSDSwitching FreqRatesPacketsBytesType1 464.1424 1.4312 1363.875 152 207309 P2P 2 452.6442 1.0364 1397.611 163 227811 P2P 3534.8935 4.4694 893.5 149 133132 P2P 4 558.6362 3.8437 1074.848 154165527 P2P5701.5207 0.2592 747.25 9772483 Non-P2P 6103.6535 0.0069 14512417980 Non-P2P 7102.6203 0.0086 149.0357 136 20269 Non-P2P 8103.1136 0.0122151.0654 13119790Non-P2P[11]/PUBLICATIONS/AB STRACTS/EAGE98/eage98_hcd.pdf [12][13]FeiSi Science and Technology R&D Center <Neural Network Theory and MATLAB7 Implementation>,Beijng. Electronic Industry Public House. Mar. 2005.。