数据挖掘技术英语论文
大数据领域英文作文
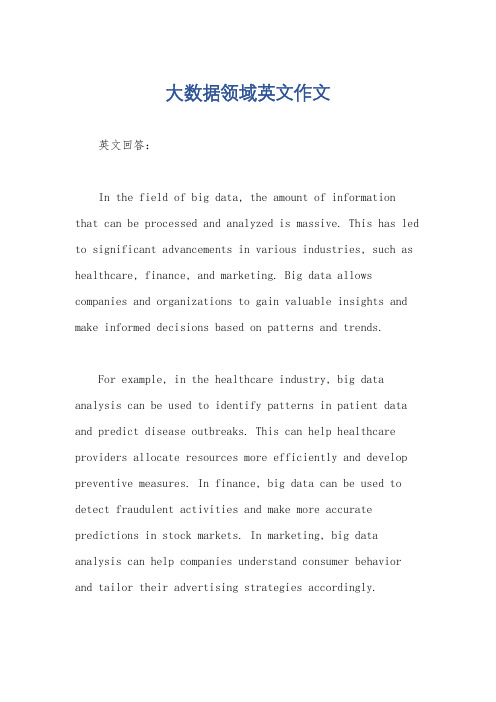
大数据领域英文作文英文回答:In the field of big data, the amount of informationthat can be processed and analyzed is massive. This has led to significant advancements in various industries, such as healthcare, finance, and marketing. Big data allows companies and organizations to gain valuable insights and make informed decisions based on patterns and trends.For example, in the healthcare industry, big data analysis can be used to identify patterns in patient data and predict disease outbreaks. This can help healthcare providers allocate resources more efficiently and develop preventive measures. In finance, big data can be used to detect fraudulent activities and make more accurate predictions in stock markets. In marketing, big data analysis can help companies understand consumer behavior and tailor their advertising strategies accordingly.Furthermore, big data has also revolutionized the waywe conduct research and make scientific discoveries. With the help of big data analytics, researchers can analyzelarge datasets and uncover hidden patterns and correlations. This has led to breakthroughs in various scientific fields, such as genomics, astronomy, and climate science.Moreover, big data has also contributed to the development of artificial intelligence (AI) and machine learning. By analyzing large datasets, AI algorithms can learn and improve their performance over time. This has led to the development of AI-powered technologies, such asvirtual assistants, autonomous vehicles, and personalized recommendations.In conclusion, big data has had a profound impact on various industries and has opened up new possibilities for innovation and growth. The ability to process and analyze massive amounts of information has allowed companies and organizations to make more informed decisions and gain valuable insights. Additionally, big data has also contributed to advancements in research, AI, and machinelearning. Overall, big data is shaping the future and will continue to play a crucial role in our society.中文回答:在大数据领域,可以处理和分析的信息量是巨大的。
大数据技术的作文英语
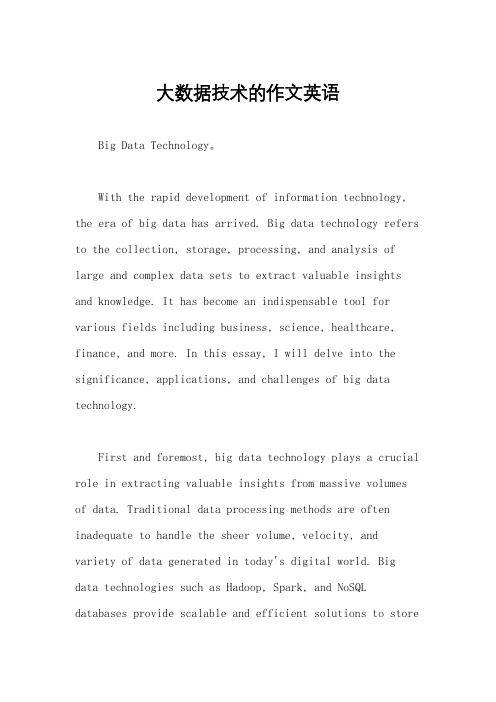
大数据技术的作文英语Big Data Technology。
With the rapid development of information technology, the era of big data has arrived. Big data technology refers to the collection, storage, processing, and analysis of large and complex data sets to extract valuable insights and knowledge. It has become an indispensable tool for various fields including business, science, healthcare, finance, and more. In this essay, I will delve into the significance, applications, and challenges of big data technology.First and foremost, big data technology plays a crucial role in extracting valuable insights from massive volumes of data. Traditional data processing methods are often inadequate to handle the sheer volume, velocity, andvariety of data generated in today's digital world. Big data technologies such as Hadoop, Spark, and NoSQL databases provide scalable and efficient solutions to storeand process vast amounts of data. These technologies enable organizations to gain deeper insights into customer behavior, market trends, and operational efficiency.One of the significant applications of big data technology is in business and marketing. Companies can analyze customer data to understand their preferences, purchasing behavior, and sentiment towards products or services. This enables personalized marketing campaigns, targeted advertising, and product recommendations, leading to better customer engagement and increased sales. For example, e-commerce giant Amazon utilizes big dataanalytics to recommend products based on users' browsing and purchasing history, resulting in a significant increase in sales revenue.Moreover, big data technology has revolutionized healthcare by facilitating data-driven decision-making and personalized medicine. Healthcare providers can analyze electronic health records, medical imaging, and genomic data to identify patterns, diagnose diseases, and recommend personalized treatment plans. This leads to better patientoutcomes, reduced healthcare costs, and improved population health management. For instance, IBM's Watson Health platform leverages big data analytics to assist healthcare professionals in diagnosing and treating cancer patients more effectively.Furthermore, big data technology has immense potential in scientific research and discovery. Scientists can analyze large datasets generated from experiments, simulations, and observations to uncover new insights and knowledge across various disciplines. This includes areas such as climate modeling, genomics, particle physics, and astronomy. For example, the Large Hadron Collider (LHC) generates petabytes of data from particle collisions, which are analyzed using big data techniques to discover new particles and understand the fundamental laws of physics.Despite its numerous benefits, big data technology also poses several challenges. One of the primary challenges is data privacy and security. As large volumes of sensitive data are collected and stored, there is a risk of data breaches, unauthorized access, and misuse of personalinformation. Ensuring data privacy and compliance with regulations such as GDPR (General Data Protection Regulation) and HIPAA (Health Insurance Portability and Accountability Act) is crucial to maintain trust and integrity in the use of big data.Another challenge is the need for skilled professionals proficient in big data technologies and data analytics. There is a growing demand for data scientists, data engineers, and data analysts who can manage and analyze large datasets effectively. However, there is a shortage of talent with the necessary skills and expertise, leading to a competitive job market and recruitment challenges for organizations.In conclusion, big data technology has revolutionized the way we collect, store, process, and analyze data, enabling unprecedented insights and opportunities across various industries. From business and marketing to healthcare and scientific research, big data technology has transformed the way we make decisions, innovate, and solve complex problems. However, it also poses challenges such asdata privacy, security, and skills shortage. Addressing these challenges will be crucial in harnessing the full potential of big data technology for the benefit of society.。
数据挖掘论文医学数据论文:医学数据挖掘综述
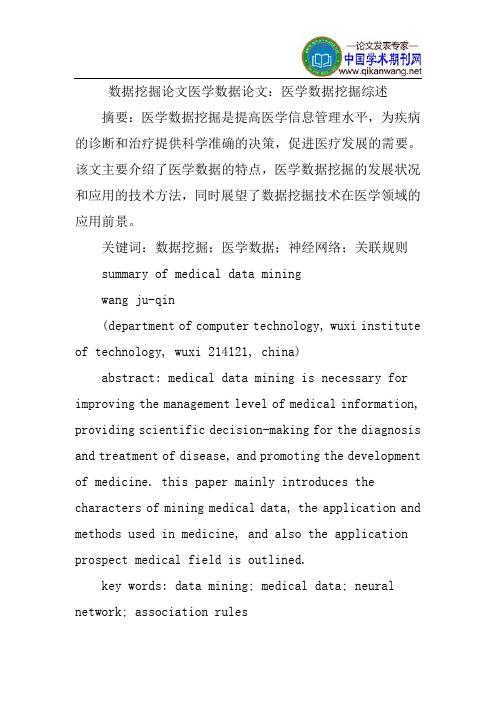
数据挖掘论文医学数据论文:医学数据挖掘综述摘要:医学数据挖掘是提高医学信息管理水平,为疾病的诊断和治疗提供科学准确的决策,促进医疗发展的需要。
该文主要介绍了医学数据的特点,医学数据挖掘的发展状况和应用的技术方法,同时展望了数据挖掘技术在医学领域的应用前景。
关键词:数据挖掘;医学数据;神经网络;关联规则summary of medical data miningwang ju-qin(department of computer technology, wuxi institute of technology, wuxi 214121, china)abstract: medical data mining is necessary for improving the management level of medical information, providing scientific decision-making for the diagnosis and treatment of disease, and promoting the development of medicine. this paper mainly introduces the characters of mining medical data, the application and methods used in medicine, and also the application prospect medical field is outlined.key words: data mining; medical data; neural network; association rules1 数据挖掘的产生1.1 产生背景在当今信息化和网络化的社会条件下,随着计算机、数据库技术的迅速发展以及数据库管理系统的广泛应用,各行各业都开始采用计算机以及相应的信息技术进行管理和运营,由此积累了大量的数据资料;另外,互联网的发展更是为我们带来了海量的数据和信息。
人工智能技术论文英文
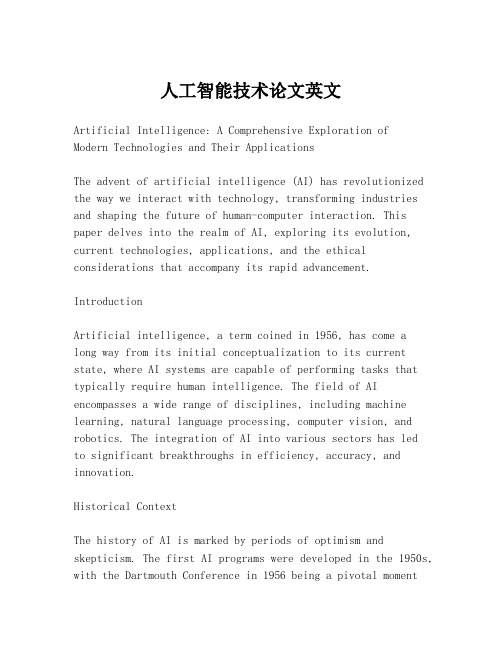
人工智能技术论文英文Artificial Intelligence: A Comprehensive Exploration of Modern Technologies and Their ApplicationsThe advent of artificial intelligence (AI) has revolutionized the way we interact with technology, transforming industries and shaping the future of human-computer interaction. This paper delves into the realm of AI, exploring its evolution, current technologies, applications, and the ethical considerations that accompany its rapid advancement.IntroductionArtificial intelligence, a term coined in 1956, has come along way from its initial conceptualization to its current state, where AI systems are capable of performing tasks that typically require human intelligence. The field of AI encompasses a wide range of disciplines, including machine learning, natural language processing, computer vision, and robotics. The integration of AI into various sectors has ledto significant breakthroughs in efficiency, accuracy, and innovation.Historical ContextThe history of AI is marked by periods of optimism and skepticism. The first AI programs were developed in the 1950s, with the Dartmouth Conference in 1956 being a pivotal momentthat set the stage for AI research. The 1960s and 1970s saw the development of the first AI programs, including ELIZA and SHRDLU. However, the field faced a period of stagnation known as the "AI winter" due to unfulfilled promises and lack of funding. It wasn't until the late 1990s and early 2000s that AI research gained momentum again, with the advent of machine learning and the availability of big data.Fundamental ConceptsAt the core of AI are algorithms and computational modelsthat enable machines to learn from data, make decisions, and perform tasks autonomously. Machine learning, a subset of AI, involves the development of algorithms that can learn from and make predictions or decisions based on data. Deep learning, a subset of machine learning, uses neural networks with many layers to model complex patterns in data.Current TechnologiesThe current landscape of AI technologies is diverse and includes:1. Machine Learning Platforms: These platforms provide the tools and frameworks for developers to build and train AI models.2. Natural Language Processing (NLP): NLP enables machines to understand, interpret, and generate human language.3. Computer Vision: This technology allows machines to interpret and analyze visual information from the world.4. Robotics: AI-powered robots can perform tasks that requirephysical manipulation and movement.5. Expert Systems: These systems use AI to simulate the decision-making ability of a human expert.ApplicationsAI has found its way into numerous applications across various industries:1. Healthcare: AI is used for diagnosis, treatment planning, and personalized medicine.2. Finance: AI technologies are employed for fraud detection, algorithmic trading, and risk management.3. Transportation: Autonomous vehicles and smart traffic systems are powered by AI.4. Retail: AI enhances customer experience through personalized recommendations and inventory management.5. Education: Adaptive learning systems powered by AI cater to individual learning needs.Challenges and Ethical ConsiderationsAs AI continues to advance, it brings with it a set of challenges and ethical considerations:1. Bias and Fairness: AI systems can inherit and amplify the biases present in their training data, leading to unfair outcomes.2. Privacy: The use of AI in data analysis raises concerns about individual privacy and data protection.3. Job Displacement: The automation of tasks by AI has thepotential to displace jobs, leading to economic and social implications.4. Transparency and Explainability: The complexity of AI models can make it difficult to understand how they arrive at certain decisions.The Future of AILooking ahead, AI is poised to become more integrated intoour daily lives, with advancements in areas such as general AI, which aims to create machines that can perform any intellectual task that a human being can. The development ofAI also calls for a collaborative approach between technologists, policymakers, and society to ensure its responsible and beneficial use.ConclusionArtificial intelligence stands as a testament to human ingenuity and our relentless pursuit of innovation. While it presents numerous opportunities for societal advancement, it also poses significant challenges that must be addressed. As we move forward, it is crucial to foster a balanced approach that harnesses the potential of AI while mitigating its risks. The journey of AI is not just about creating intelligent machines; it is about shaping a future that is inclusive, ethical, and beneficial for all.In conclusion, the field of AI is dynamic and ever-evolving.It holds the promise of transforming our world in ways we are only beginning to understand. As we continue to explore anddevelop AI technologies, it is imperative that we do so with a keen eye on their societal impact, ensuring that they serve to enhance and enrich our lives in a manner that is responsible and sustainable.。
写一篇关于大数据专业的英语作文
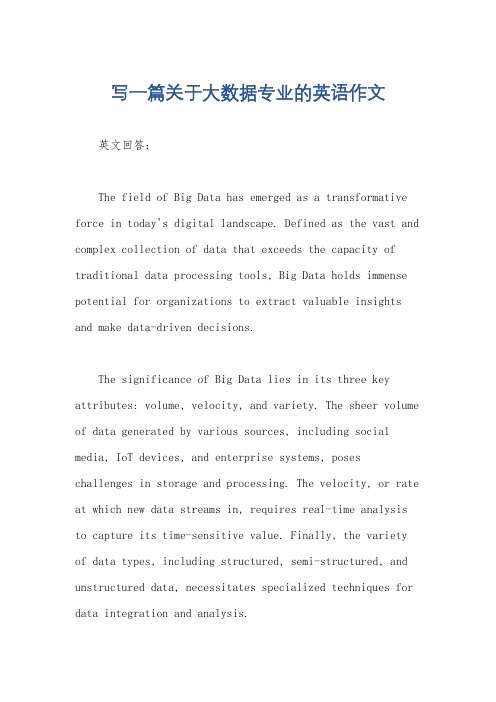
写一篇关于大数据专业的英语作文英文回答:The field of Big Data has emerged as a transformative force in today's digital landscape. Defined as the vast and complex collection of data that exceeds the capacity of traditional data processing tools, Big Data holds immense potential for organizations to extract valuable insights and make data-driven decisions.The significance of Big Data lies in its three key attributes: volume, velocity, and variety. The sheer volume of data generated by various sources, including social media, IoT devices, and enterprise systems, poses challenges in storage and processing. The velocity, or rate at which new data streams in, requires real-time analysis to capture its time-sensitive value. Finally, the variety of data types, including structured, semi-structured, and unstructured data, necessitates specialized techniques for data integration and analysis.The benefits of leveraging Big Data are multifaceted.It enables organizations to:Enhance decision-making: Data-driven insights empower businesses to make informed decisions, optimize processes, and predict market trends.Improve customer experience: By analyzing customer data, organizations can personalize experiences, anticipate preferences, and address pain points.Detect fraud and security breaches: Big Data analytics can detect anomalies and identify suspicious patterns in financial transactions and security logs.Drive innovation: Data-powered research and development foster innovation by identifying new opportunities and developing cutting-edge products and services.Increase operational efficiency: Data analysis canuncover inefficiencies, streamline operations, and optimize resource allocation.To harness the full potential of Big Data, organizations must invest in robust data management and analytics infrastructure. This includes scalable storage solutions, powerful processing engines, and specialized analytics tools. Additionally, skilled data scientists and engineers who possess expertise in data mining, statistical modeling, and machine learning are essential for extracting meaningful insights from vast data volumes.The impact of Big Data is not confined to the business world. It has profound implications for society as a whole:Healthcare: Big Data analytics can improve patient outcomes, optimize drug discovery, and personalize medical treatments.Environmental monitoring: Data from sensors and satellites helps track environmental changes, predict natural disasters, and protect ecosystems.Transportation: Data analysis can optimize traffic flow, improve vehicle efficiency, and enhancetransportation safety.Government: Big Data supports data-driven policymaking, fraud detection, and anti-corruption measures.As technology continues to advance, the volume, velocity, and variety of Big Data will only increase. This presents both opportunities and challenges fororganizations and society alike. By embracing the potential of Big Data and investing in data management and analytics capabilities, we can unlock unprecedented insights anddrive innovation for the benefit of future generations.中文回答:大数据专业。
大数据挖掘外文翻译文献
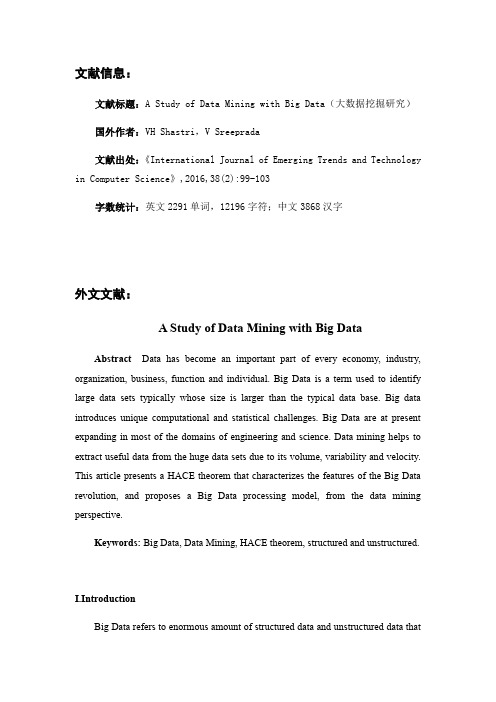
文献信息:文献标题:A Study of Data Mining with Big Data(大数据挖掘研究)国外作者:VH Shastri,V Sreeprada文献出处:《International Journal of Emerging Trends and Technology in Computer Science》,2016,38(2):99-103字数统计:英文2291单词,12196字符;中文3868汉字外文文献:A Study of Data Mining with Big DataAbstract Data has become an important part of every economy, industry, organization, business, function and individual. Big Data is a term used to identify large data sets typically whose size is larger than the typical data base. Big data introduces unique computational and statistical challenges. Big Data are at present expanding in most of the domains of engineering and science. Data mining helps to extract useful data from the huge data sets due to its volume, variability and velocity. This article presents a HACE theorem that characterizes the features of the Big Data revolution, and proposes a Big Data processing model, from the data mining perspective.Keywords: Big Data, Data Mining, HACE theorem, structured and unstructured.I.IntroductionBig Data refers to enormous amount of structured data and unstructured data thatoverflow the organization. If this data is properly used, it can lead to meaningful information. Big data includes a large number of data which requires a lot of processing in real time. It provides a room to discover new values, to understand in-depth knowledge from hidden values and provide a space to manage the data effectively. A database is an organized collection of logically related data which can be easily managed, updated and accessed. Data mining is a process discovering interesting knowledge such as associations, patterns, changes, anomalies and significant structures from large amount of data stored in the databases or other repositories.Big Data includes 3 V’s as its characteristics. They are volume, velocity and variety. V olume means the amount of data generated every second. The data is in state of rest. It is also known for its scale characteristics. Velocity is the speed with which the data is generated. It should have high speed data. The data generated from social media is an example. Variety means different types of data can be taken such as audio, video or documents. It can be numerals, images, time series, arrays etc.Data Mining analyses the data from different perspectives and summarizing it into useful information that can be used for business solutions and predicting the future trends. Data mining (DM), also called Knowledge Discovery in Databases (KDD) or Knowledge Discovery and Data Mining, is the process of searching large volumes of data automatically for patterns such as association rules. It applies many computational techniques from statistics, information retrieval, machine learning and pattern recognition. Data mining extract only required patterns from the database in a short time span. Based on the type of patterns to be mined, data mining tasks can be classified into summarization, classification, clustering, association and trends analysis.Big Data is expanding in all domains including science and engineering fields including physical, biological and biomedical sciences.II.BIG DATA with DATA MININGGenerally big data refers to a collection of large volumes of data and these data are generated from various sources like internet, social-media, business organization, sensors etc. We can extract some useful information with the help of Data Mining. It is a technique for discovering patterns as well as descriptive, understandable, models from a large scale of data.V olume is the size of the data which is larger than petabytes and terabytes. The scale and rise of size makes it difficult to store and analyse using traditional tools. Big Data should be used to mine large amounts of data within the predefined period of time. Traditional database systems were designed to address small amounts of data which were structured and consistent, whereas Big Data includes wide variety of data such as geospatial data, audio, video, unstructured text and so on.Big Data mining refers to the activity of going through big data sets to look for relevant information. To process large volumes of data from different sources quickly, Hadoop is used. Hadoop is a free, Java-based programming framework that supports the processing of large data sets in a distributed computing environment. Its distributed supports fast data transfer rates among nodes and allows the system to continue operating uninterrupted at times of node failure. It runs Map Reduce for distributed data processing and is works with structured and unstructured data.III.BIG DATA characteristics- HACE THEOREM.We have large volume of heterogeneous data. There exists a complex relationship among the data. We need to discover useful information from this voluminous data.Let us imagine a scenario in which the blind people are asked to draw elephant. The information collected by each blind people may think the trunk as wall, leg as tree, body as wall and tail as rope. The blind men can exchange information with each other.Figure1: Blind men and the giant elephantSome of the characteristics that include are:i.Vast data with heterogeneous and diverse sources: One of the fundamental characteristics of big data is the large volume of data represented by heterogeneous and diverse dimensions. For example in the biomedical world, a single human being is represented as name, age, gender, family history etc., For X-ray and CT scan images and videos are used. Heterogeneity refers to the different types of representations of same individual and diverse refers to the variety of features to represent single information.ii.Autonomous with distributed and de-centralized control: the sources are autonomous, i.e., automatically generated; it generates information without any centralized control. We can compare it with World Wide Web (WWW) where each server provides a certain amount of information without depending on other servers.plex and evolving relationships: As the size of the data becomes infinitely large, the relationship that exists is also large. In early stages, when data is small, there is no complexity in relationships among the data. Data generated from social media and other sources have complex relationships.IV.TOOLS:OPEN SOURCE REVOLUTIONLarge companies such as Facebook, Yahoo, Twitter, LinkedIn benefit and contribute work on open source projects. In Big Data Mining, there are many open source initiatives. The most popular of them are:Apache Mahout:Scalable machine learning and data mining open source software based mainly in Hadoop. It has implementations of a wide range of machine learning and data mining algorithms: clustering, classification, collaborative filtering and frequent patternmining.R: open source programming language and software environment designed for statistical computing and visualization. R was designed by Ross Ihaka and Robert Gentleman at the University of Auckland, New Zealand beginning in 1993 and is used for statistical analysis of very large data sets.MOA: Stream data mining open source software to perform data mining in real time. It has implementations of classification, regression; clustering and frequent item set mining and frequent graph mining. It started as a project of the Machine Learning group of University of Waikato, New Zealand, famous for the WEKA software. The streams framework provides an environment for defining and running stream processes using simple XML based definitions and is able to use MOA, Android and Storm.SAMOA: It is a new upcoming software project for distributed stream mining that will combine S4 and Storm with MOA.Vow pal Wabbit: open source project started at Yahoo! Research and continuing at Microsoft Research to design a fast, scalable, useful learning algorithm. VW is able to learn from terafeature datasets. It can exceed the throughput of any single machine networkinterface when doing linear learning, via parallel learning.V.DATA MINING for BIG DATAData mining is the process by which data is analysed coming from different sources discovers useful information. Data Mining contains several algorithms which fall into 4 categories. They are:1.Association Rule2.Clustering3.Classification4.RegressionAssociation is used to search relationship between variables. It is applied in searching for frequently visited items. In short it establishes relationship among objects. Clustering discovers groups and structures in the data.Classification deals with associating an unknown structure to a known structure. Regression finds a function to model the data.The different data mining algorithms are:Table 1. Classification of AlgorithmsData Mining algorithms can be converted into big map reduce algorithm based on parallel computing basis.Table 2. Differences between Data Mining and Big DataVI.Challenges in BIG DATAMeeting the challenges with BIG Data is difficult. The volume is increasing every day. The velocity is increasing by the internet connected devices. The variety is also expanding and the organizations’ capability to capture and process the data is limited.The following are the challenges in area of Big Data when it is handled:1.Data capture and storage2.Data transmission3.Data curation4.Data analysis5.Data visualizationAccording to, challenges of big data mining are divided into 3 tiers.The first tier is the setup of data mining algorithms. The second tier includesrmation sharing and Data Privacy.2.Domain and Application Knowledge.The third one includes local learning and model fusion for multiple information sources.3.Mining from sparse, uncertain and incomplete data.4.Mining complex and dynamic data.Figure 2: Phases of Big Data ChallengesGenerally mining of data from different data sources is tedious as size of data is larger. Big data is stored at different places and collecting those data will be a tedious task and applying basic data mining algorithms will be an obstacle for it. Next we need to consider the privacy of data. The third case is mining algorithms. When we are applying data mining algorithms to these subsets of data the result may not be that much accurate.VII.Forecast of the futureThere are some challenges that researchers and practitioners will have to deal during the next years:Analytics Architecture:It is not clear yet how an optimal architecture of analytics systems should be to deal with historic data and with real-time data at the same time. An interesting proposal is the Lambda architecture of Nathan Marz. The Lambda Architecture solves the problem of computing arbitrary functions on arbitrary data in real time by decomposing the problem into three layers: the batch layer, theserving layer, and the speed layer. It combines in the same system Hadoop for the batch layer, and Storm for the speed layer. The properties of the system are: robust and fault tolerant, scalable, general, and extensible, allows ad hoc queries, minimal maintenance, and debuggable.Statistical significance: It is important to achieve significant statistical results, and not be fooled by randomness. As Efron explains in his book about Large Scale Inference, it is easy to go wrong with huge data sets and thousands of questions to answer at once.Distributed mining: Many data mining techniques are not trivial to paralyze. To have distributed versions of some methods, a lot of research is needed with practical and theoretical analysis to provide new methods.Time evolving data: Data may be evolving over time, so it is important that the Big Data mining techniques should be able to adapt and in some cases to detect change first. For example, the data stream mining field has very powerful techniques for this task.Compression: Dealing with Big Data, the quantity of space needed to store it is very relevant. There are two main approaches: compression where we don’t loose anything, or sampling where we choose what is thedata that is more representative. Using compression, we may take more time and less space, so we can consider it as a transformation from time to space. Using sampling, we are loosing information, but the gains inspace may be in orders of magnitude. For example Feldman et al use core sets to reduce the complexity of Big Data problems. Core sets are small sets that provably approximate the original data for a given problem. Using merge- reduce the small sets can then be used for solving hard machine learning problems in parallel.Visualization: A main task of Big Data analysis is how to visualize the results. As the data is so big, it is very difficult to find user-friendly visualizations. New techniques, and frameworks to tell and show stories will be needed, as for examplethe photographs, infographics and essays in the beautiful book ”The Human Face of Big Data”.Hidden Big Data: Large quantities of useful data are getting lost since new data is largely untagged and unstructured data. The 2012 IDC studyon Big Data explains that in 2012, 23% (643 exabytes) of the digital universe would be useful for Big Data if tagged and analyzed. However, currently only 3% of the potentially useful data is tagged, and even less is analyzed.VIII.CONCLUSIONThe amounts of data is growing exponentially due to social networking sites, search and retrieval engines, media sharing sites, stock trading sites, news sources and so on. Big Data is becoming the new area for scientific data research and for business applications.Data mining techniques can be applied on big data to acquire some useful information from large datasets. They can be used together to acquire some useful picture from the data.Big Data analysis tools like Map Reduce over Hadoop and HDFS helps organization.中文译文:大数据挖掘研究摘要数据已经成为各个经济、行业、组织、企业、职能和个人的重要组成部分。
数据挖掘论文精选5篇论文

数据挖掘论⽂精选5篇论⽂数据挖掘论⽂精选5篇论⽂ 数据挖掘⼀: 题⽬:数据挖掘技术在神经根型颈椎病⽅剂研究中的优势及应⽤进展 关键词:数据挖掘技术; 神经根型颈椎病; ⽅剂; 综述; 1 数据挖掘技术简介 数据挖掘技术[1] (Knowledge Discovery in Datebase, KKD) , 是⼀种新兴的信息处理技术, 它融汇了⼈⼯智能、模式别、模糊数学、数据库、数理统计等多种技术⽅法, 专门⽤于海量数据的处理, 从⼤量的、不完全的、有噪声的、模糊的、随机的数据集中, 提取隐含在其中的、⼈们事先不知道的、但⼜是潜在的有⽤的信息和知识, 其⽬的是发现规律⽽不是验证假设。
数据挖掘技术主要适⽤于庞⼤的数据库的研究, 其特点在于:基于数据分析⽅法⾓度的分类, 其本质属于观察性研究, 数据来源于⽇常诊疗⼯作,应⽤的技术较传统研究更先进, 分析⼯具、理论模型与传统研究区别较⼤。
其操作步骤包括[2]:选择数据, 数据处理, 挖掘分析, 结果解释, 其中结果解释是数据挖掘技术研究的关键。
其⽅法包括分类、聚类、关联、序列、决策树、贝斯⽹络、因⼦、辨别等分析[3], 其结果通常表⽰为概念、规则、规律、模式、约束、可视化等形式图[4]。
当今数据挖掘技术的⽅向主要在于:特定数据挖掘, ⾼效挖掘算法, 提⾼结果的有效性、确定性和表达性, 结果的可视化, 多抽象层上的交互式数据挖掘, 多元数据挖掘及数据的安全性和保密性。
因其优势和独特性被运⽤于多个领域中, 且结果运⽤后取得显着成效, 因此越来越多的中医⽅剂研究者将其运⽤于⽅剂中药物的研究。
2 数据挖掘术在神经根型颈椎病治⽅研究中的优势 中医对于神经根型颈椎病的治疗准则为辨证论治, 从古⾄今神经根型颈椎病的中医证型有很多, 其治⽅是集中医之理、法、⽅、药为⼀体的数据集合, 具有以“⽅-药-证”为核⼼的多维结构。
⽅剂配伍本质上表现为⽅与⽅、⽅与药、药与药、药与剂量, 以及⽅药与证、病、症交叉错综的关联与对应[5], ⽽中医⽅剂讲究君⾂佐使的配伍, 药物有升降沉浮, 四⽓五味及归经之别, 对于神经根型颈椎病的治疗, 治⽅中药物的种类、炮制⽅法、⽤量、⽤法等都是千变万化的, ⽽这些海量、模糊、看似随机的药物背后隐藏着对临床有⽤的信息和规律, 但这些⼤数据是⽆法在可承受的时间范围内可⽤常规软件⼯具进⾏捕捉、管理和处理的, 是需要⼀个新处理模式才能具有更强的决策⼒、洞察⼒和流程优化能⼒, ⽽数据挖掘技术有可能从这些海量的的数据中发现新知识, 揭⽰背后隐藏的关系和规则, 并且对未知的情况进⾏预测[6]。
数据挖掘技术应用论文
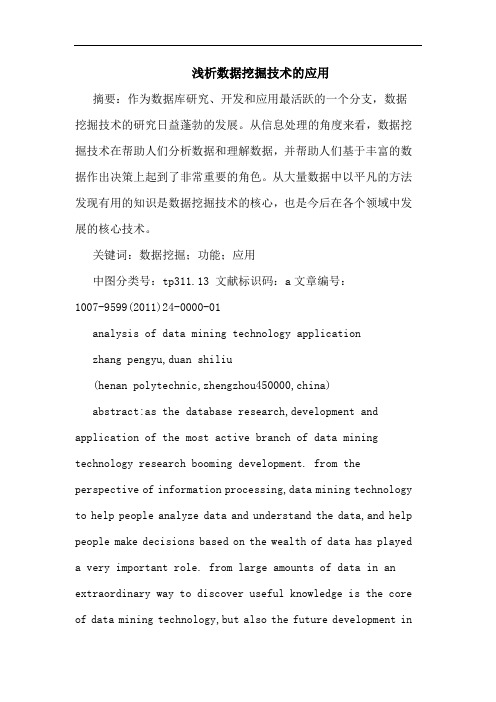
浅析数据挖掘技术的应用摘要:作为数据库研究、开发和应用最活跃的一个分支,数据挖掘技术的研究日益蓬勃的发展。
从信息处理的角度来看,数据挖掘技术在帮助人们分析数据和理解数据,并帮助人们基于丰富的数据作出决策上起到了非常重要的角色。
从大量数据中以平凡的方法发现有用的知识是数据挖掘技术的核心,也是今后在各个领域中发展的核心技术。
关键词:数据挖掘;功能;应用中图分类号:tp311.13 文献标识码:a文章编号:1007-9599(2011)24-0000-01analysis of data mining technology applicationzhang pengyu,duan shiliu(henan polytechnic,zhengzhou450000,china)abstract:as the database research,development and application of the most active branch of data mining technology research booming development. from the perspective of information processing,data mining technology to help people analyze data and understand the data,and help people make decisions based on the wealth of data has played a very important role. from large amounts of data in an extraordinary way to discover useful knowledge is the core of data mining technology,but also the future development invarious fields in the core technology.keywords:data mining;function;application一、数据挖掘概述近年来,数据挖掘引起了信息产业界和整个社会的极大关注,其主要原因是存在可以广泛使用大量数据,并且迫切需要将这些数据转换成有用的信息和知识。
- 1、下载文档前请自行甄别文档内容的完整性,平台不提供额外的编辑、内容补充、找答案等附加服务。
- 2、"仅部分预览"的文档,不可在线预览部分如存在完整性等问题,可反馈申请退款(可完整预览的文档不适用该条件!)。
- 3、如文档侵犯您的权益,请联系客服反馈,我们会尽快为您处理(人工客服工作时间:9:00-18:30)。
Good evening, ladies and gentlemen. I’m very glad to stand here and give you a short speech. Today I would introduce data mining technology to you. What is the data mining technology and what’s advantage and disadvantage. Now let's talk about this.
Data mining refers to "Extracting implicit unknown valuable information from the data in the past” or “a scientific extracting information from a large amount of data or databases”, In general,it needs strict steps to be taken.including understanding, aquistion, intergration, data cleaning, assumptions and interpretation.
By using these steps, we could get implicit and valuable information from the data. However, in spite of these complete steps, there are still many shortcomings.
First of all, the operator has many problems in its development, such as the target market segmentation is not clear,the demand of data mining and evaluation of information is not enough; product planning and management are difficult to meet the customer information needs; the attraction to partners is a little less, and it has not yet formed a win-win value chain; in the level of operation management and business process, the ability of sales team and group informatization service are not adapted to the development of business.In a word, there’re still have a lot of things to be solved. It needs excellent statistics and technology. It
also needs greater power of refining and summary.
Secondly,it’s easy to listen only by the data.”let the data speak”is not wrong, but we should keep it in mind that :next,parties! If the data and tools can solve the problem,what should people do? The data itself can only help analysts to find what are significant results,but it can’t tell you whether the result is right or wrong.So it also requires us to check up the relevant information seriously in case of being cheated by the data. Thirdly, Related to data mining,it also involves privacy issues, for example: an employer can access medical records to screen out those who have diabetes or serious heart disease, which is aimed to reduce the insurance expenditure. However, this approach will lead to ethical and legal issues.
Data mining of government and commerce may involve in the national security or commercial confidentiality issues . It is also a big challenge to confidentiality.. In this aspect,it need the user obey social morals and government strengthen regulation.
All in all,every technology has its own advantages and disadvantages. We need to learn to recognize it and how to use it effectively. In order to create greater benefits for mankind.we still have many things to be discovered about data mining. That’s
all,thanks for your listening.
,。