Greedy Algorithm贪心算法
贪婪算法是一种什么方法
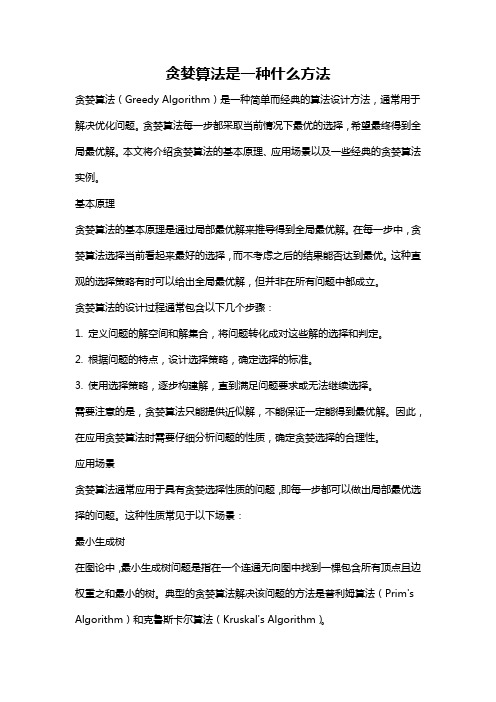
贪婪算法是一种什么方法贪婪算法(Greedy Algorithm)是一种简单而经典的算法设计方法,通常用于解决优化问题。
贪婪算法每一步都采取当前情况下最优的选择,希望最终得到全局最优解。
本文将介绍贪婪算法的基本原理、应用场景以及一些经典的贪婪算法实例。
基本原理贪婪算法的基本原理是通过局部最优解来推导得到全局最优解。
在每一步中,贪婪算法选择当前看起来最好的选择,而不考虑之后的结果能否达到最优。
这种直观的选择策略有时可以给出全局最优解,但并非在所有问题中都成立。
贪婪算法的设计过程通常包含以下几个步骤:1. 定义问题的解空间和解集合,将问题转化成对这些解的选择和判定。
2. 根据问题的特点,设计选择策略,确定选择的标准。
3. 使用选择策略,逐步构建解,直到满足问题要求或无法继续选择。
需要注意的是,贪婪算法只能提供近似解,不能保证一定能得到最优解。
因此,在应用贪婪算法时需要仔细分析问题的性质,确定贪婪选择的合理性。
应用场景贪婪算法通常应用于具有贪婪选择性质的问题,即每一步都可以做出局部最优选择的问题。
这种性质常见于以下场景:最小生成树在图论中,最小生成树问题是指在一个连通无向图中找到一棵包含所有顶点且边权重之和最小的树。
典型的贪婪算法解决该问题的方法是普利姆算法(Prim's Algorithm)和克鲁斯卡尔算法(Kruskal's Algorithm)。
普利姆算法从一个起始顶点出发,每次选择与当前生成树连接的最短边对应的顶点,直到生成树包含所有顶点。
而克鲁斯卡尔算法则是从边集合中每次选择最小的边,同时保证不形成环,直到生成树包含所有顶点。
背包问题背包问题是在给定背包容量和一系列物品的重量和价值的情况下,如何选择物品放入背包中,使得背包中物品的总价值最大。
贪婪算法在背包问题的解决中有时也能给出较好的近似解。
一种典型的贪婪算法解决背包问题的方法是基于每个物品的单位价值(即单位重量所能获得的价值)来进行选择。
启发式算法分类

启发式算法分类
启发式算法可分为以下几类:
1. 贪心算法(Greedy Algorithm):每步选择当前最优解,有时能得到全局最优解,但并非万能算法。
2. 分治算法(Divide and Conquer):将一个大问题分割成若干个小的子问题,然后递归地求解,最后把子问题的解合并成原问题的解。
3. 动态规划算法(Dynamic Programming):把大问题分解成若干个小问题,先求解子问题,把子问题的解缓存起来,最后把子问题的解合并成原问题的解。
与分治算法不同的是,分治算法不能利用子问题的重叠性质,这就是动态规划算法的关键点。
4. 回溯算法(Backtracking):用于在搜索空间中找到所有或一个满足约束条件的解。
适用于解空间树有很多分支的问题,其实就是一种深度优先搜索。
5. 遗传算法(Genetic Algorithm):模拟生物进化过程的算法,用于寻找问题的最优解。
通过选择、交叉和变异等基因操作,生成一群解,并利用适应度函数对解进行排序,优胜劣汰,产生新的一代解。
6. 模拟退火算法(Simulated Annealing):模拟物理上的退火过程,用于在凸
函数或多峰函数的曲面寻找全局最优解。
7. 禁忌搜索算法(Tabu Search):用于处理约束条件存在的优化问题。
算法探索解空间,并避免回到已经探索过的解。
为了能在较短时间内找到最优解,禁忌搜索具有启发性,即在探索解空间时,依据之前的经验和搜索方向进行探索。
贪心算法的基本原理

贪心算法的基本原理贪心算法(Greedy Algorithm)是一种常用的算法思想,它在求解最优化问题时通常能够得到较好的近似解。
贪心算法的基本原理是:每一步都选择当前状态下的最优解,从而希望最终能够得到全局最优解。
在实际应用中,贪心算法常常用于解决一些最优化问题,如最小生成树、最短路径、任务调度等。
一、贪心算法的特点贪心算法具有以下特点:1. 简单:贪心算法通常比较简单,易于实现和理解。
2. 高效:贪心算法的时间复杂度通常较低,能够在较短的时间内得到结果。
3. 局部最优:每一步都选择当前状态下的最优解,但不能保证最终能够得到全局最优解。
4. 适用范围:贪心算法适用于一些特定类型的问题,如无后效性、最优子结构等。
二、贪心算法的基本原理贪心算法的基本原理可以概括为以下几个步骤:1. 初始状态:确定问题的初始状态,定义问题的输入和输出。
2. 状态转移:根据当前状态,选择局部最优解,并更新状态。
3. 筛选解:判断当前状态下是否满足问题的约束条件,若满足则保留该解,否则舍弃。
4. 终止条件:重复以上步骤,直至满足终止条件,得到最终解。
三、贪心算法的应用举例1. 找零钱:假设有 25、10、5、1 四种面额的硬币,需要找零 41 元,如何使得找零的硬币数量最少?贪心算法可以先选择面额最大的硬币,然后逐步选择面额较小的硬币,直至找零完毕。
2. 区间调度:给定一组区间,如何选择最多的互不重叠的区间?贪心算法可以先按照区间的结束时间排序,然后依次选择结束时间最早的区间,直至所有区间都被覆盖。
3. 最小生成树:在一个连通的带权无向图中,如何选择边使得生成树的权值最小?贪心算法可以按照边的权值从小到大排序,然后依次选择权值最小且不构成环的边,直至所有顶点都被连接。
四、贪心算法的优缺点1. 优点:贪心算法简单高效,适用于一些特定类型的问题,能够在较短的时间内得到近似最优解。
2. 缺点:贪心算法不能保证一定能够得到全局最优解,可能会出现局部最优解不是全局最优解的情况。
贪心算法程序设计
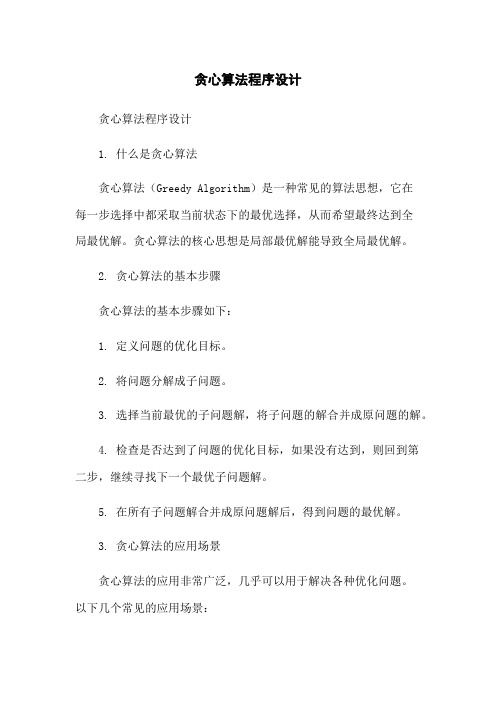
贪心算法程序设计贪心算法程序设计1. 什么是贪心算法贪心算法(Greedy Algorithm)是一种常见的算法思想,它在每一步选择中都采取当前状态下的最优选择,从而希望最终达到全局最优解。
贪心算法的核心思想是局部最优解能导致全局最优解。
2. 贪心算法的基本步骤贪心算法的基本步骤如下:1. 定义问题的优化目标。
2. 将问题分解成子问题。
3. 选择当前最优的子问题解,将子问题的解合并成原问题的解。
4. 检查是否达到了问题的优化目标,如果没有达到,则回到第二步,继续寻找下一个最优子问题解。
5. 在所有子问题解合并成原问题解后,得到问题的最优解。
3. 贪心算法的应用场景贪心算法的应用非常广泛,几乎可以用于解决各种优化问题。
以下几个常见的应用场景:1. 零钱找零问题:给定一定面额的纸币和硬币,如何找零使得所需纸币和硬币的数量最小?2. 区间调度问题:给定一些活动的开始时间和结束时间,如何安排活动使得可以办理的活动数量最大?3. 背包问题:给定一些具有重量和价值的物品,如何选择物品使得背包的总价值最大?4. 最小树问题:给定一个带权无向图,如何找到一棵树,使得它的边权之和最小?5. 哈夫曼编码问题:给定一组字符和相应的频率,如何构造一个满足最低编码长度限制的二进制编码?4. 贪心算法的优缺点贪心算法的优点是简单、高效,可以快速得到一个近似最优解。
而且对于一些问题,贪心算法能够得到全局最优解。
贪心算法的缺点在于它不一定能够得到全局最优解,因为在每一步只考虑局部最优解,无法回溯到之前的选择。
5. 贪心算法的程序设计在使用贪心算法进行程序设计时,通常需要以下几个步骤:1. 定义问题的优化目标。
2. 将问题分解成子问题,并设计子问题的解决方案。
3. 设计贪心选择策略,选择局部最优解。
4. 设计贪心算法的递推或迭代公式。
5. 判断贪心算法是否能够得到全局最优解。
6. 编写程序实现贪心算法。
6.贪心算法是一种常见的算法思想,它在每一步选择中都采取当前状态下的最优选择,从而希望最终达到全局最优解。
英语 算法 -回复
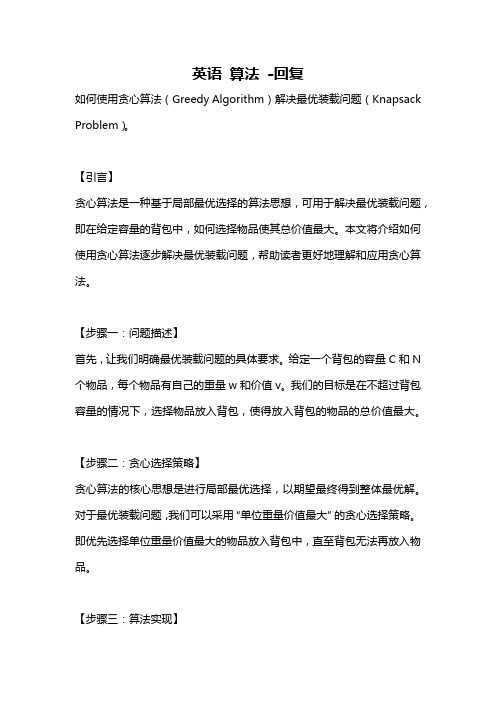
英语算法-回复如何使用贪心算法(Greedy Algorithm)解决最优装载问题(Knapsack Problem)。
【引言】贪心算法是一种基于局部最优选择的算法思想,可用于解决最优装载问题,即在给定容量的背包中,如何选择物品使其总价值最大。
本文将介绍如何使用贪心算法逐步解决最优装载问题,帮助读者更好地理解和应用贪心算法。
【步骤一:问题描述】首先,让我们明确最优装载问题的具体要求。
给定一个背包的容量C和N 个物品,每个物品有自己的重量w和价值v。
我们的目标是在不超过背包容量的情况下,选择物品放入背包,使得放入背包的物品的总价值最大。
【步骤二:贪心选择策略】贪心算法的核心思想是进行局部最优选择,以期望最终得到整体最优解。
对于最优装载问题,我们可以采用“单位重量价值最大”的贪心选择策略。
即优先选择单位重量价值最大的物品放入背包中,直至背包无法再放入物品。
【步骤三:算法实现】基于贪心选择策略,我们可以使用如下步骤实现算法:1. 根据物品的重量w和价值v,计算每个物品的单位重量价值vu = v / w。
2. 按照单位重量价值vu从大到小对物品进行排序。
3. 初始化当前背包的总价值val = 0和当前背包的剩余容量rc = C。
4. 逐个遍历排序后的物品列表:a. 如果当前物品的重量小于等于当前背包的剩余容量,则将该物品放入背包中,更新当前背包的总价值val和剩余容量rc。
b. 如果当前物品的重量大于当前背包的剩余容量,则放弃该物品,继续遍历下一个物品。
5. 返回最终的背包总价值val作为最优装载问题的解。
【步骤四:算法示例】接下来,我们通过一个简单的例子演示如何使用贪心算法解决最优装载问题。
假设背包容量C为10,有以下4个物品可供选择:物品1:重量w1 = 2,价值v1 = 5物品2:重量w2 = 3,价值v2 = 8物品3:重量w3 = 4,价值v3 = 9物品4:重量w4 = 5,价值v4 = 10按照贪心选择策略,首先计算每个物品的单位重量价值vu:物品1:vu1 = v1 / w1 = 5 / 2 = 2.5物品2:vu2 = v2 / w2 = 8 / 3 ≈2.67物品3:vu3 = v3 / w3 = 9 / 4 = 2.25物品4:vu4 = v4 / w4 = 10 / 5 = 2.0然后,按照单位重量价值vu从大到小对物品进行排序:物品2 > 物品1 > 物品3 > 物品4接下来,我们按照步骤三中的算法实现进行装载:初始化当前背包的总价值val = 0和剩余容量rc = 10。
贪心算法的概念和适用条件

贪心算法的概念和适用条件什么是贪心算法?贪心算法(Greedy Algorithm)是一种以局部最优解为导向的算法思想,通过每一步选择当前状态下的最佳操作来达到整体最优解的目标。
贪心算法的核心思想是每次都做出当前看来最优的选择,以期望能够达到整体的最优解。
贪心算法通常用于一些问题中,即每一步的选择只依赖于当前状态,而不考虑将来可能出现的情况。
贪心算法的适用条件:1. 贪心选择性质:贪心算法每一步都选择一个当前的最优解,此处的“最优”指的是局部最优。
这种最优选择可以确保问题能够被拆解,并且进行下一步求解。
2. 最优子结构性质:当问题的整体最优解能够通过局部最优解得到时,可以采用贪心算法求解。
这种情况下,问题的最优解可以由子问题的最优解推导出来。
3. 无后效性:贪心算法选择某一步操作时,只考虑当前状态,不会改变以前的操作,并且不关心未来的操作。
这种无后效性使得贪心算法在实际应用中操作简单、效率高。
贪心算法的基本步骤:1. 确定问题的局部最优解:贪心算法的核心是每一步都选择在当前情况下的最优解。
因此,需要确定问题如何拆解以及如何进行局部最优选择。
2. 定义问题的子问题:根据问题的最优子结构性质,将问题拆解为较小规模的子问题。
子问题应该是原问题的一个更小、更简单的实例。
3. 定义贪心选择策略:根据问题的特性,确定当前步骤下的最优选择策略。
这个选择应该是局部最优的,可以在不考虑子问题和整体未来状态的情况下得出。
4. 重复执行步骤2和3,直至求解出全局最优解。
贪心算法的优缺点:贪心算法具有简单易懂、快速高效的特点,适用于许多实际问题。
它可以避免穷举所有可能性,节省了计算时间。
此外,贪心算法常常能够找到近似最优解,尽管不一定能够保证全局最优解。
在实际问题中,近似最优解也往往可以满足实际需求。
然而,贪心算法并非适用于所有问题。
由于贪心算法只考虑当前状态的最优选择,而不考虑未来的影响,因此可能会导致局部最优解与全局最优解不一致。
算法的方法有哪些
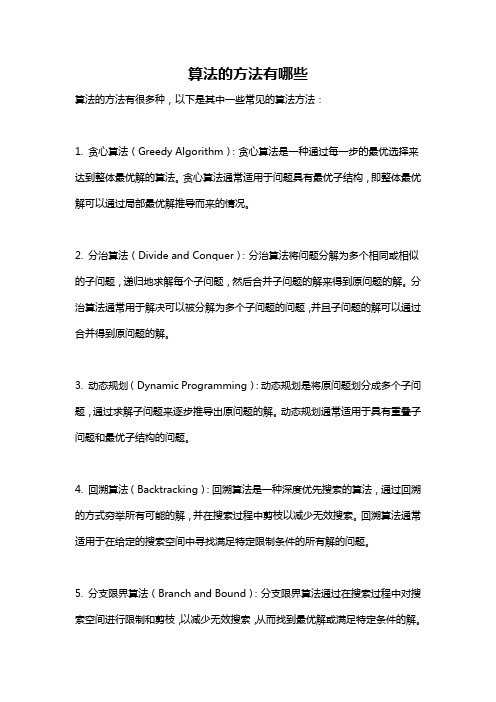
算法的方法有哪些算法的方法有很多种,以下是其中一些常见的算法方法:1. 贪心算法(Greedy Algorithm):贪心算法是一种通过每一步的最优选择来达到整体最优解的算法。
贪心算法通常适用于问题具有最优子结构,即整体最优解可以通过局部最优解推导而来的情况。
2. 分治算法(Divide and Conquer):分治算法将问题分解为多个相同或相似的子问题,递归地求解每个子问题,然后合并子问题的解来得到原问题的解。
分治算法通常用于解决可以被分解为多个子问题的问题,并且子问题的解可以通过合并得到原问题的解。
3. 动态规划(Dynamic Programming):动态规划是将原问题划分成多个子问题,通过求解子问题来逐步推导出原问题的解。
动态规划通常适用于具有重叠子问题和最优子结构的问题。
4. 回溯算法(Backtracking):回溯算法是一种深度优先搜索的算法,通过回溯的方式穷举所有可能的解,并在搜索过程中剪枝以减少无效搜索。
回溯算法通常适用于在给定的搜索空间中寻找满足特定限制条件的所有解的问题。
5. 分支限界算法(Branch and Bound):分支限界算法通过在搜索过程中对搜索空间进行限制和剪枝,以减少无效搜索,从而找到最优解或满足特定条件的解。
分支限界算法通常适用于在搜索空间很大且问题有明确界限的情况下。
6. 迭代法(Iterative Method):迭代法通过反复执行相同的计算步骤,逐渐逼近原问题的解。
迭代法通常适用于解决连续函数逼近、方程求根等问题。
7. 枚举法(Enumeration Method):枚举法是一种逐一检查所有可能解的方法,通过枚举所有可能的解空间来找到问题的解。
枚举法通常适用于问题的解空间较小且可以通过穷举法找到所有解的情况。
8. 随机化算法(Randomized Algorithm):随机化算法通过引入随机性来解决问题,可用于加速搜索过程、解决概率性问题或从多个解中选择一个。
数据库查询优化的启发式算法与优化策略

数据库查询优化的启发式算法与优化策略数据库查询优化是提高数据库系统性能和响应速度的关键任务之一。
在大型数据库系统中,查询优化能够有效地减少查询时间,提高查询效率,从而提高整个系统的性能。
本文将介绍数据库查询优化的启发式算法和优化策略,以帮助数据库管理员和开发人员更好地优化数据库查询。
1. 启发式算法在数据库查询优化中的应用启发式算法是一种基于经验和启迪规则的计算方法,能够在较短时间内找到搜索空间中接近最优的解。
在数据库查询优化中,启发式算法可以帮助我们在有限的时间内寻找到接近最优的查询执行计划。
以下是几种常见的启发式算法:(1) 贪心算法(Greedy Algorithm):该算法基于最佳资源利用准则,通过逐步选择最佳操作,直到找到一个近似最优解。
在数据库查询优化中,贪心算法可以优化选择最佳连接顺序的问题。
(2) 遗传算法(Genetic Algorithm):该算法模拟了自然界中的进化过程,通过选择、变异和交叉等操作,在个体之间产生新的解,并逐代演化,从而找到近似最优解。
在数据库查询优化中,遗传算法可以应用于选择最佳执行计划的问题。
(3) 禁忌搜索算法(Tabu Search Algorithm):该算法主要用于解决在搜索过程中出现的局部最优问题。
通过记录和禁忌一些解集合,在搜索过程中避免陷入局部最优解,从而更容易找到全局最优解。
在数据库查询优化中,禁忌搜索算法可以用于优化查询语句中的关联谓词问题。
2. 数据库查询优化的策略除了启发式算法,还有一些常见的优化策略可以应用于数据库查询优化。
下面是几种常见的优化策略:(1) 索引优化:合理创建索引可以大幅提高查询效率。
在数据库查询优化中,通过根据数据库中的查询频率和模式创建合适的索引,可以减少磁盘IO和CPU消耗,提高查询性能。
(2) 分区优化:将大型表分割成更小的物理存储单元可以提高查询性能。
通过将数据按照某种划分策略存储在不同的存储设备上,可以减少查询时的磁盘IO次数,提高查询效率。
- 1、下载文档前请自行甄别文档内容的完整性,平台不提供额外的编辑、内容补充、找答案等附加服务。
- 2、"仅部分预览"的文档,不可在线预览部分如存在完整性等问题,可反馈申请退款(可完整预览的文档不适用该条件!)。
- 3、如文档侵犯您的权益,请联系客服反馈,我们会尽快为您处理(人工客服工作时间:9:00-18:30)。
Greedy Algorithms Greedy AlgorithmsDr. Zhenyu HeAutumn 2008116Greedy Algorithms z16 Greedy AlgorithmsSimilar to dynamic programming. Used for optimization problems.2zzOptimization problems typically go through a sequence of steps, with a set of choices at each step.For many optimization problems, using dynamic programming to determine z y p p ,g y p g g the best choices is overkill (过度的杀伤威力).Greedy Algorithm: Simpler, more efficient16Greedy Algorithms z 16 Greedy AlgorithmsGreedy algorithms (GA) do not always yield 3y g ()y yoptimal solutions, but for many problems they do.161the activity selection problem 16.1, the activity-selection problem (活动安排)16.2, basic elements of the GA; knapsack prob. (贪婪算法的基本特征;背包问题)16.3, an important application: the design of data compression (Huffman) codes. (哈夫曼编码)16.5, unit-time tasks scheduling 6.5,u t t e tas s sc edu g (有限期作业调度)16Greedy Algorithms z 16 Greedy AlgorithmsThe greedy method is quite powerful and works 4g y q pwell for a wide range of problems:minimum-spanning-tree algorithms (Chap 23) minimum-spanning-tree algorithms (Chap 23)(最小生成图)h t t th f i l (Ch 24) shortest paths from a single source (Chap 24)(最短路径)set-covering heuristic (Chap 35).(集合覆盖)…First example:Activity Selection First example: Activity Selection Horseback Riding (骑马)5Canoeing (独木舟)Surfing (冲浪)Driving Swimming Rock Climbing (攀岩)z 91011121314151617How to make an arrangement to have the more activities?S1Sh i i fi 短优原S1. Shortest activity first (最短活动优先原则)Swimming , DrivingS2First starting activity first S2. First starting activity first (最早开始活动优先原则)Horseback Riding , DrivingS3. First finishing activity first (最早结束活动优先原则)Swimming , Rock Climbing , Surfing161An activity selection problem 16.1 An activity-selection problem 6i i i iz n activities require exclusive use of a common resource.Example, scheduling the use of a classroom.playground p e,sc edu g e use o c ss oo (n 个活动,1 项资源,任一活动进行时需唯一占用该资源)Set of activities S = {a 1, a 2, . . . , a n }. a i needs resource during period [s i , f i ), which is a half-open interval, where s i is start time and f i is finish time.Goal:Select the largest possible set of nonoverlapping (mutually Goal: Select the largest possible set of nonoverlapping (mutually compatible) activities. (安排一个活动计划,使得相容的活动数目最多)Other objectives: Maximize income rental fees , …j ,161An activity selection problemz16.1 An activity-selection problemn activities require exclusive use of a common resource.7Set of activities S = {a1, a2, . . . , a n}a i needs resource during period [s i , f i )l S fi i iz Example: S sorted by finish time:Maximum-size mutuallyMaximum size mutuallycompatible set:{a1 , a3 , a6 , a,a3,a6,a8}.Not unique: also{a2 , a5 , a7 , a9}.16.1.1 Optimal substructure of activity selection Space of subproblems8z S ij = {a k ∈S : f i ≤ s k < f k ≤ s j }= activities that start after a i finishes & finish before a j starts zActivities in S ij are compatible with all activities that finish by f i (完成时间早于f i 的活动), and ll ti iti th t t t li th z all activities that start no earlier than s j .To represent the entire problem, add fictitious activities:a 0=[0);1=[“ z0 = [﹣∞, 0); a n+1 = [∞, “∞+1”)We don’t care about ﹣∞ in a 0 or “∞+1” in a n+1.Then S = S 0,n+1. Range for S ij is 0 i, j n + 1.,g j ≤,j ≤16.1.1 Optimal substructure of activity selection Space of subproblems 9zz p pS ij = {a k ∈S : f i ≤ s k < f k ≤ s j }Assume that activities are sorted by monotonically increasing finish time Assume that activities are sorted by monotonically increasing finish time (以结束时间单调增的方式对活动进行排序)f 0 ≤ f 1 ≤ f 2 ≤ · · · ≤ f n < f n+1 (if i ≤ j, then f i ≤ f j )(16.1)11109876543210123456789101112131416.1.1 Optimal substructure of activity selectionif 012···n <f n+1(if i j then i )(161)10Then i ≥ j S ij =i k <f k j <f j i <f j z if f 0 ≤ f 1 ≤ f 2 ≤ ≤ f n < f n+1 (if i ≤ j, then f i ≤ f j ) (16.1)Proof If there exists a k ∈S ij , thenf i ≤ s k < f k ≤ s j < f j f i < f j .z But i ≥ j f i ≥ f j . Contradiction.So only need to worry about with 0 ≤i <j ≤ n + 1.S All other are ij S ij16.1.1 Optimal substructure of activity selection11S th t l ti t i l d H 2b b zSuppose that a solution to S ij includes a k . Have 2 sub-prob S ik (start after a i finishes, finish before a k starts)S kj (start after a k finishes, finish before a j starts)zSolution to S ij = (solution to S ik )∪{a k }∪(solution to S kj )Since a k is in neither of the subproblems, and the subproblems are disjoint |solution to S |=|solution to ik |+1+|solution to kj |zdisjoint, | solution to S | = | solution to S ik | + 1 + | solution to S kj | .Optimal substructure: If an optimal solution to S ij includes a k , then the solutions to S ik and S kj used within this solution must be optimal ll (l t d t t)zas well. (use usual cut-and-paste argument).Let A ij = optimal solution to S ij ,so A ij = A ik ∪{a k ∪A kj (16.2)so j {}j ,assuming: S ij is nonempty; and we know a k .()A il iz16.1.2 A recursive solution Let c[i, j ] = size of maximum-size subset of mutually compatible activities inij c[i j]ij 12i ≥ j S ij = c[i, j ] = 0.z S ij .(c[i,j] 表示S ij 相容的最大活动数)If S ij ≠ , suppose that a k is used in a maximum-size subsets of mutually S ij .Then c[i j]=c[i k]+1+c[k j ]zThen c[i, j] = c[i, k] + 1 + c[k, j ] .But of course we don’t know which k to use, and so0if Sij = (16.3)0 , if Sij ,c [i , j ] = max{c [i , k ] + c [k , j ] + 1} , if Sij ⎯ .i <k<j Why this range of k? Because S ij = {a k ∈S: f i ≤ s k < f k ≤ s j } a k can’t be a i or a j .k ija S ∈16.1.3 Converting a DP solution to a greedy solution 0 , if S ij = ,] 13 i < k < j c [i , j ]= max{c [i , k ] + c [k , j ] + 1} , if S ij ⎯ .(16.3)z a k S ijIt may be easy to design an algorithm to the problem based on recurrence(16.3).Di t i l ith (l it ?)zDirect recursion algorithm (complexity?)Dynamic programming algorithm (complexity?)Can we simplify our solution?16.1.3 Converting a DP solution to a greedy solutionTheorem 16.1L t d l t b th ti it i ith th li t fi i h ti 14Let S ij ≠ , and let a m be the activity in S ij with the earliest finish time: f m =min {f k : a k ∈S ij }. Then1. a m is used in some maximum-size subset of mutually compatible activities of S i j .(a m 包含在某个最大相容活动子集中)2. S im = , so that choosing a m leaves S mj as the only nonemptysubproblem.(仅剩下一个非空子问题S mj )p j 1110987654321123456789101112131416.1.3 Converting a DP solution to a greedy solutionTheorem 16.1L t d l t b th ti it i ith th li t fi i h ti 15Let S ij ≠ , and let a m be the activity in S ij with the earliest finish time: f m =min {f k : a k ∈S ij }. Then1. a m is used in some maximum-size subset of mutuallycompatible activities of S i j .(a m 包含在某个最大相容活动子集中)2. S im = , so that choosing a m leaves S mj as the onlynonempty subproblem. (仅剩下一个非空子问题S mj )Proof2. Suppose there is some .Then Then a S ∈i l l m m l m f s f s f f f <<≤<⇒<l ij a s ∈a m . Therefore, there is no .and it has an earlier finish time than , which contradicts our choice ofl imm f limima S S ∈⇒=∅16.1.3 Converting a DP solution to a greedy solutionTheorem 16.1 Let S ij ≠ , and let a m be the activity in S ij with the li t fi i h ti i {f }Th16earliest finish time: f m = min {f k : a k ∈S ij }. Then 1. a m is used in some maximum-size subset of mutuallycompatible activities of S i j .(a m 包含在某个最大相容活动子集中)p j Proof 1. Let A ij be a maximum-size subset of mutually Compatibleactivities in ij Order activities in ij in monotonically increasing activities in S ij . Order activities in A ij in monotonically increasing order of finish time. Let a k be the first activity in A ij .If a k = a m , done (a m is used in a maximum-size subset).a k a mOtherwise construct =A ij }(replace k by )first activity in A ij to finish. f m ≤f k a m doesn’t overlap anything Otherwise, construct B ij A ij -{a k }∪{a m } (replace a k by a m ).Activities in B ij are disjoint. (Activities in A ij are disjoint, a k is the else in ij ).Since |B ij |=ij |and ij is a maximum-size subset,else in B ij ). Since |B ij | |A ij | and A ij is a maximum size subset,so is B ij .16.1.3 Converting a DP solution to a greedy solution 0 , if S ij = ,,j ]= 17 i k k Sc [i , j ]max{c [i , k ] + c [k , j ] + 1} , if S ij ⎯ . a < < ij(16.3)Th 161L t d l t b th ti it iTheorem 16.1 Let S ij ≠ , and let a m be the activity in S ij with the earliest finish time: f m = min {f k : a k ∈S ij }. Then 1. a m is used in some maximum-size subset of mutually ycompatible activities of S i j .(a m 包含在某个最大相容活动子集中)2. S im = , so that choosing a m leaves S mj as the only nonempty b bl 仅剩下个非空子问题zsubproblem. (仅剩下一个非空子问题S mj )This theorem is great:before theoremafter theorem # of sub-prob in optimal solution #of choices to consider 2O (j i 1)11# of choices to considerO (j –i –1)16.1.3 Converting a DP solution to a greedy solutionTheorem 16.1 Let S ij ≠ , and let a m be the activity in S ij with the earliest finish time:=min {f k :k ij }Then18earliest finish time: f m = min {f k : a k ∈S ij }. Then 1. a m is used in some maximum-size subset of mutually compatible activitiesof S i j .(a m 包含在某个最大相容活动子集中)2i = so that choosing leaves j as the only nonempty subproblemz2. S im = , so that choosing a m leaves S mj as the only nonempty subproblem.(仅剩下一个非空子问题S mj )Now we can solve a problem ij in a top-down fashion Now we can solve a problem S ij in a top down fashion Choose a m ∈S ij with earliest finish time: the greedy choice. ( it leaves asmuch opportunity as possible for the remaining activities to be scheduled )(留下尽可能的时间来安排活动,贪心选择)Then solve S mj .z16.1.3 Converting a DP solution to a greedy solutionWhat are the subproblems?19Original problem is S 0,n+1 〔a 0 = [﹣∞, 0); a n+1 = [∞, “∞+1”) 〕Suppose our first choice is a m1 (in fact, it is a 1)Then ne t s bproblem is Then next subproblem isS m1,n+1Suppose next choice is a m2 (it must be a 2?)Next subproblem isS m2,n+1p ,And so on11107893546121234567891011121314z16.1.3 Converting a DP solution to a greedy solutionWhat are the subproblems?20Original problem is S 0,n+1Suppose our first choice is a m1Then next subproblem is m1n+1 Then next subproblem is S m1,n+1Suppose next choice is a m2Next subproblem is S m2,n+1zAnd so onEach subproblem is S mi ,n+1.And the subproblems chosen have finish times that increase zzAnd the subproblems chosen have finish times that increase. (所选的子问题,其完成时间是增序排列)Therefore, we can consider each activity just once, in monotonically increasing order of finish time increasing order of finish time.z 16.1.4 A recursive greedy algorithmOriginal problem is S 0,n+1E h b bl i 21z zEach subproblem is S mi ,n+1Assumes activites already sorted by monotonically increasing finish time. (If not, then sort in O(n lg n) time.) Return an optimal solution for S i,n+1:×a m√a ma ia iREC-ACTIVITY-SELECTOR(s,f,i,n)REC ACTIVITY SELECTOR(s, f, i, n)1 m ← i+12 while m ≤n and s m < f i // Find first activity in S i,n+1.3d 13 do m ← m+14 if m ≤ n5 then return {a m ∪REC-ACTIVITY-SELECTOR(s, f, m, n){}(,f,,)6 else returna 2a 116.1.4 A recursive greedy algorithmREC-ACTIVITY-SELECTOR(s, f, i, n)22(,f,,)1 m ← i+12 while m ≤n and s m < f i // Find first activity in S i,n+1.3do m3 do m ← m+14 if m ≤ n5 then return {a m }∪REC-ACTIVITY-SELECTOR(s, f, m, n)6else return6 else returnz √Initial call: REC-ACTIVITY-SELECTOR(s, f, 0, n).a mza iIdea: The while loop checks a i+1 , a i+2 , . . . , a n untilit finds an activity a m that is compatible with a i (need s m ≥ f i ).If the loop terminates because a m is found (m ≤n), thenrecursively solve S m,n+1, and return this solution, along with a m .If the loop never finds a compatible (m >n)then just returnIf the loop never finds a compatible a m (m > n), then just return empty set.a 2a 116.1.4 A recursive greedy algorithmREC-ACTIVITY-SELECTOR(s, f, i, n)23(,f,,)1 m ← i+12 while m ≤n and s m < f i // Find first activity in S i,n+1.3do m3 do m ← m+14 if m ≤ n5 then return {a m }∪REC-ACTIVITY-SELECTOR(s, f, m, n)6else return z6 else returnTime: Θ(n)—each activity examined exactly once.T (n ) =m 1 + T (n m 1 ) =m 1 + m 2 + T (n m 1 m 2 )123T n 123)= m 1 + m 2 + m 3 + T (n m 1 m 2 m 3 ) = =mk+ T (nm k )b 1 th 1∑k T (1)Θ(…because: nm k = 1, thenm k = n −1, mk + T (1) = n )z 16.1.4 A recursive greedy algorithmInitial call: REC-ACTIVITY-SELECTOR(s, f, 0, n).24zIdea: The while loop checksa i+1 , a i+2 , . . . , a n until it finds an activity that is finds an activity a m that is compatible with a i (need s m ≥ f i ). If the loop terminates because a m is found n)then rec rsi el (m ≤n), then recursively solve S m,n+1, and return thissolution, along witha m .,g If the loop never finds a compatible a m (m > n),h jthen just return empty set.z 16.1.5 An iterative greedy algorithmREC-ACTIVITY-SELECTOR is almost "tail recursive“.We easily can convert the recursive procedure to an iterative one (Some 25zWe easily can convert the recursive procedure to an iterative one. (Some compilers perform this task automatically)a 2a 1GREEDY-ACTIVITY-SELECTOR(s, f, n)1 A ← {a 1}2 i ← 1×a m √a m53 for m ← 2 to n 4 do if s m ≥ f i a i a ithen A ←A ∪{a m }6i ← m // a i is most recent addition to A 7 return AzReviewGreedy Algorithm Idea: When we have a choice to make, make the one that looks best right now Make a locally optimal choice in hope of getting a 26looks best right now. Make a locally optimal choice in hope of getting a globally optimal solution.(希望当前选择是最好的,每一个局部最优选择能产生全局最优选择)zGreedy Algorithm: Simpler, more efficient16Greedy Algorithms z 16 Greedy Algorithms16.1, the activity-selection problem (活动安排)27z ,y p 16.2, basic elements of the GA; knapsack probz(贪婪算法的基本特征;背包问题)16.3, an important application: the design of data compression (Huffman)codes compression (Huffman) codes(哈夫曼编码)162Elements of the greedy strategy z16.2 Elements of the greedy strategyThe choice that seems best at the moment is chosen(每次决策时当前所做的选择看起来是“最好”的)28z(每次决策时,当前所做的选择看起来是“最好”的)What did we do for activity selection?1Determine the optimal substructure 1. Determine the optimal substructure.2. Develop a recursive solution.3. Prove that at any stage of recursion, one of the optimal choices is y g ,pthe greedy choice.4. Show that all but one of the subproblems resulting from the greedy choice are empty. (通过贪婪选择,只有一个子问题非空)5. Develop a recursive greedy algorithm.6C t it t it ti l ith6. Convert it to an iterative algorithm.162Elements of the greedy strategy z 16.2 Elements of the greedy strategyThese steps looked like dynamic programming.29z zTypically, we streamline these steps (简化这些步骤)Develop the substructure with an eye towardmaking the greedy choice,leaving just one subproblem.F ti it l ti h d th t th d h i i li d zFor activity selection, we showed that the greedy choice implied that in S ij , only i varied, and j was fixed at n+1,So we could have started out with a greedy algorithm in mind:zSo, we could have started out with a greedy algorithm in mind:define S i = {a k ∈S : f i ≤ s k }, (所有在a i 结束之后开始的活动)show the greedy choice,first m to finish in show the greedy choice, first a m to finish in S icombined with optimal solution to S moptimal solution to S i .162Elements of the greedy strategy z16.2 Elements of the greedy strategyTypical streamlined steps (简化这些步骤)301. Cast the optimization problem as one in which we make a choice and areleft with one subproblem to solve.(最优问题为:做一个选择,留下一个待解的子问题)(最优问题为:做个选择,留下个待解的子问题)2. Prove that there’s always an optimal solution that makes the greedychoice, so that the greedy choice is always safe.3. Show that greedy choice and optimal solution to subproblem optimal solution to the problem (证明存在一个基于贪婪选择的最优解,因此贪婪选择是安全的)solution to the problem.(说明由贪婪选择和子问题的最优解 原问题的最优解)162Elements of the greedy strategy z 16.2 Elements of the greedy strategyNo general way to tell if a greedy algorithm is 31g y g y g optimal, but two key ingredients are(没有一般化的规则来说明贪婪算法是否最优,但有两个基本要点)1. greedy-choice property (贪婪选择属性)2. optimal substructurez 16.2.1 Greedy-choice propertyA globally optimal solution can be arrived at by making a locally optimal (greedy)choice 32(greedy) choice. (通过局部最优解可导出全局最优解)S i,r-1k i ..k r k r ..k j Dynamic programming k r i j i zDynamic programming S i,j .... Make a choice at each step.S r-1,j k k r-1k r+1k j Choice depends on knowing optimal solutions to subproblems.Solve subproblems first. (依赖于已知子问题的最优解再作出选择) Solve bottom-up.S 0,n+110911z Greedy a m1S m1,n+154678 Make a choice at each step.132Make the choice before solving the subproblems. (先作选择,再解子问题)Solve top-down.pz16.2.1 Greedy-choice property We must prove that a greedy choice at each step yields a globally optimal solution Difficulty!Cleverness may be required!33zsolution. Difficulty! Cleverness may be required!Typically, Theorem 16.1, shows that the solution (A ij ) can be modified to use the greedy choice (a m ), resulting in one similar but smaller subproblem (A mj ).z We can get efficiency gains from greedy-choice property. (For example, inactivity-selection, sorted the activities in monotonically increasing order of finish times needed to examine each activity just once )finish times, needed to examine each activity just once.)Preprocess input to put it into greedy orderz 16.2.2 Optimal substructureoptimal substructure: an optimal solution to the 34problem contains within it optimal solutions to subproblems.Just show that optimal solution to subproblem z p p (说明子问题的最优解和贪婪选择andgreedy choice optimal solution to problem.)原问题的最优解16.2.3 Greedy vs. dynamic programming bread cake 35pizza cellphone camera laptop 0-1knapsack problem 背包问题小偷问题)z0-1 knapsack problem (0-1背包问题,小偷问题)n items (n 个物品)Item i is worth $v i , weighs w i P (物品i 价值v i ,重w i ) ,g Find a most valuable subset of items with total weight ≤W.(背包的最大负载量为W ,如何选取物品,使得背包装的物品价值最大)Have to either take an item or not take it can’t take part of it z Have to either take an item or not take it—can t take part of it.(每个物品是一个整体,不能分割,因此,要么选取该物品,要么不选取)Fractional knapsack problem (分数背包问题,小偷问题)Lik h 01k k bl b k f i f iLike the 0-1 knapsack problem, but can take fraction of an item.z 16.2.3 Greedy vs. dynamic programming 0-1 knapsack problem (0-1背包问题,小偷问题)F ti l k k bl (分数背包问题小偷问题)36zzFractional knapsack problem (分数背包问题,小偷问题)Both have optimal substructure property.0-1 : choose the most valuable load j that weighs w j ≤ W,j g remove j , choose the most valuable load i that weighs w i ≤W-w j fractional:choose a weight w from item j (part of j )then fractional: choose a weight w from item j ( part of j ), then remove the part, the remaining load is the most valuable load weighing at most W-w that the thief can take from the n-1 original items plus w j -w pounds from item j.(若先选item j 的一部分,其重w ,则接下来的最优挑选方案为从余n-1j j -w z 下的n 1 个物品〔除j 外〕和j 的另外重w j w 的部分中挑选,其重量不超过W-w )But the fractional problem has the greedy-choice property, and the 0-1problem does not.p16.2.3 Greedy vs. dynamic programming37z Fractional knapsack problem has the greedy-choice property,FRACTIONAL-KNAPSACK(v,w,W) 1 load ← 0has the greedy choice property, and the 0-1 knapsack problem does not.2 i ← 13 while load < W and i ≤n4 do if w i ≤W -loadz To solve the fractional problem, rank decreasingly items by v i /w iL t//5 then take all of item i6 else take W-load of w i from item i7 add what was taken to load8i1z Let v i /w i ≥v i+1 /w i+18 i ←i + 1for all iz Time: O(nlg n) to sort, O(n) to greedy choice thereafter.z 16.2.3 Greedy vs. dynamic programming 0-1 knapsack problem 38has not the greedy-choice property zW = 50.G d l ti Optimal solution:z z Greedy solution:take items 1 and 2value =160weight =30Optimal solution:Take items 2 and 3value=220, weight=50 value 160, weight 3020 pounds of capacity leftover.No leftover capacity.(没有剩余空间)breadcakepizzacellphone camera laptop16Greedy Algorithms z16 Greedy Algorithms16.1, the activity-selection problem (活动安排)39z,y p 16.2, basic elements of the GA; knapsack prob z (贪婪算法的基本特征;背包问题)16.3, an important application: the design of data compression (Huffman)codes compression (Huffman) codes (哈夫曼编码)163Huffman codes z16.3 Huffman codesHuffman codes: widely used and very effective technique for compressing data 40 zdata.savings of 20% to 90%Consider the data to be a sequence of characters q Abaaaabbbdcffeaaeaeec z Huffman's greedy algorithm:uses a table of the frequencies of occurrence of the characters to build up an optimal way of representing each character as a binary string.p y p g y g(依据字符出现的频率表,使用二进串来建立一种表示字符的最佳方法)163Huffman codes z16.3 Huffman codesWish to store compactly 100,000-character data file 41only six different characters appear. frequency table a b c d e f Frequency (in thousands)4513121695Frequency (in thousands)Fixed-length codeword Variable-length codeword 45 13 12 16000 001 010 011 100 1010 101 100 111 1101 1100zz Many ways (encodes) to represent such a file of information binary character code (or code for short): each character is represented by a unique binary stringunique binary string.fixed-length code: if use 3-bit codeword, the file can be encoded in 300,000bits. Can we do better?163Huffman codes z16.3 Huffman codes100,000-character data file 4295a b c d e f Frequency (in thousands)Fixed-length codeword 45 13 12 16000 001 010 011 100 101z Variable-length codeword 0 101 100 111 1101 1100binary character code (or code for short)variable-length code: by giving frequent characters short codewords and infrequent characters long codewords, here the 1-bit string 0 represents a,and the 4-bit string 1100 represents f. (高频出现的字符以短字码表示;g p 低频→长字码)(45·1 + 13·3 + 12·3 + 16·3 + 9·4 + 5·4) · 1,000 = 224,000 bits163Huffman codes z16.3 Huffman codes100,000-character data file 4395a b c d e f Frequency (in thousands)45 13 12 16Fixed-length codeword Variable-length codeword 000 001 010 011 100 1010 101 100 111 1101 1100z binary character code (or code for short)fixed-length code: 300,000 bitsvariable length code:224000bits a savings of approximately 25%In variable-length code: 224,000 bits, a savings of approximately 25%. In fact, this is an optimal character code for this file.z 16.3.1 Prefix codes prefix codes (prefix-free codes): no codeword is a prefix of some other codeword (字首码前缀代码前置代码〔44codeword. (字首码,前缀代码,前置代码〔前缀无关码〕:没有字码是其他字码的前缀)95a b c d e f Frequency (in thousands)Fixed-length codeword 45 13 12 16000001010011100101zFixed length codeword Variable-length codeword 000 001 010 011 100 1010 101 100 111 1101 1100Encoding is always simple for any binary character code Encoding (编码)is always simple for any binary character code Concatenate (连接)the codewords representing each character. For example, “abc”, with the variable-length fi d 01011000101100h ‘’t z prefix code as 0·101·100 = 0101100, where we use ‘·’ to denote concatenation.Prefix codes simplify decoding (解码)z 16.3.1 Prefix codes prefix codes (prefix-free codes): no codeword is a prefix of some other codeword (字首码前缀代码前置代码〔前缀无关码〕:没有字码是45codeword. (字首码,前缀代码,前置代码〔前缀无关码〕:没有字码是其他字码的前缀)a b c d e f 0zVariable-length codeword 101 100 111 1101 1100Encoding is always simple for any binary character code P fi d i lif d diz Prefix codes simplify decoding Since no codeword is a prefix of any other, the codewordthat begins an encoded file is unambiguous that begins an encoded file is unambiguous (明确的).We can simply identify the initial codeword, translate itback to the original character, and repeat the decoding th i d f th d d filprocess on the remainder of the encoded file.Exam: 001011101 uniquely as 0·0·101·1101, which decodesto “aabe”.to aabe .16.3.1 Prefix codes a b c d e f460 101 100 111 1101 1100001011101uniquely as 0·0·101·1101,which decodes to “aabe”.D di z Decodingthe process needs a convenient representation for theprefix code so that the initial codeword can be easily p ypicked off.(为了使初始字码容易识别,解码过程需要一种前置无关码的方便表示) A binary tree whose leaves are the given charactersprovides one such representation.树是种方便的表示方法树叶为给定字符(二叉树是一种方便的表示方法,树叶为给定字符)16.3.1 Prefix codes a b c d e f470 101 100 111 1101 1100001011101uniquely as 0·0·101·1101,which decodes to “aabe”.z DecodingWe interpret the binary codeword for a character as thepath from the root to that character.(将字符的二叉码表示解释为一条路径:从树根到树叶)not binary search trees since the leaves need not appear innot binary search trees, since the leaves need not appear in sorted order and internal nodes do not contain character keys.(不是二叉搜索树)16.3.1 Prefix codes48An optimal code for a file is always represented by a full binary tree every zAn optimal code for a file is always represented by a full binary tree, every nonleaf node has two children (Ex16.3-1). The fixed-length code in our example is not optimal.We can restrict our attention to full binary treesz We can restrict our attention to full binary trees C is the alphabet,all character frequencies >0th t f ti l fi d h |C|l f h l tt f C the tree for an optimal prefix code has |C| leaves, one for each letter of C ,and exactly |C|-1 internal nodes.16.3.1 Prefix codes 49Compute # of bits required to encode a file T ) f )(165)c CB (T ) = f (c )d T (c ) (16.5)z Given a tree T corresponding to a prefix code, for each character c in thealphabet C,f (c): frequency of c in the file f ()q y d T (c): depth of c’s leaf in the tree (length of the codeword for character c ). Then, # of bits required to encode a filewhich we define as the cost of the tree T.16.3.2 Constructing a Huffman code HUFFMAN(C)1n |C|50Huffman code:a greedy algorithm 1 n ← |C|2 Q ← C3 for i ← 1 to n -14do )a new node that constructs an optimal prefix code4 do allocate (分配) a new node z5 left[z] ← x ← EXTRACT-MIN (Q)6 right[z] ← y ← EXTRACT-MIN (Q)7f [z]←f [x]+f 8z 7 f [z] f [x] f [y]INSERT(Q, z)9 return EXTRACT-MIN(Q) //return the root of the tree.C: set of n characters, c ∈C: an object with frequency f [c].j q y f Build the tree T corresponding to the optimal code.Begin with |C| leaves, perform |C|-1 “merging” operations.A min priority queue Q keyed on f is used to identify the twoA min-priority queue Q, keyed on f , is used to identify the two least-frequent objects to merge together. Result of the merger is a new object whose frequency is the sum of the frequencies of the bj h d two objects that were merged.。