弱目标检测
复杂海况下海面弱目标的精准与智能探测1
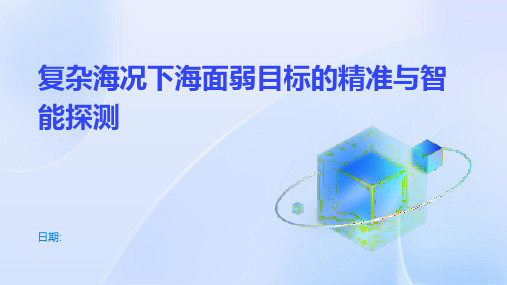
海面弱目标信号通常具有低信噪比、非线性和时变等特点,容易被海洋背景噪声 淹没。
信号提取方法
针对弱目标信号的特点,可采用匹配滤波、时频分析、波形识别等方法,提高信 号提取的准确性和效率。
噪声抑制与目标增强技术
噪声来源与特性:海面弱目标探测中的噪声主要 来源于海洋环境噪声、电子设备噪声等,具有宽 频带、非平稳等特性。
该技术可用于海上军事目标的侦察与 监视,提高海上作战能力。
海上搜救
在海上事故或灾难发生时,该技术能 够快速定位并救援海上遇险人员。
海洋科学研究
海面弱目标探测技术为海洋生物学、 海洋地理学等研究提供有力支持,推 动海洋科学的发展。
THANKS
感谢观看
பைடு நூலகம்
03
复杂海况下的精准探测技术
高分辨率雷达探测技术
高频率波段
利用高频波段的雷达探测技术 ,可以有效穿透海浪、海雾等 干扰因素,实现对海面弱目标
的高分辨率探测。
先进信号处理技术
采用先进的信号处理技术,如恒虚 警率处理、多普勒处理等,提高雷 达探测的精度和稳定性。
多目标跟踪算法
应用多目标跟踪算法,对复杂海况 下的多个弱目标进行准确跟踪和定 位,确保探测结果的可靠性。
海上实际应用
将经过验证的智能探测算法应用于实际海上场景,辅助船 员或自主航行系统实现对海面弱目标的精准探测和识别, 提高航行安全性和效率。
05
系统集成与实验验证
精准与智能探测系统总体设计
系统架构设计
针对复杂海况下的海面弱目标探测,设计一种高效、稳定的系统架 构,确保在各种海况条件下都能实现精准探测。
02
海面弱目标探测技术基础
电磁波与海洋环境的交互作用
双基地雷达Radon-Fourier变换弱目标积累检测
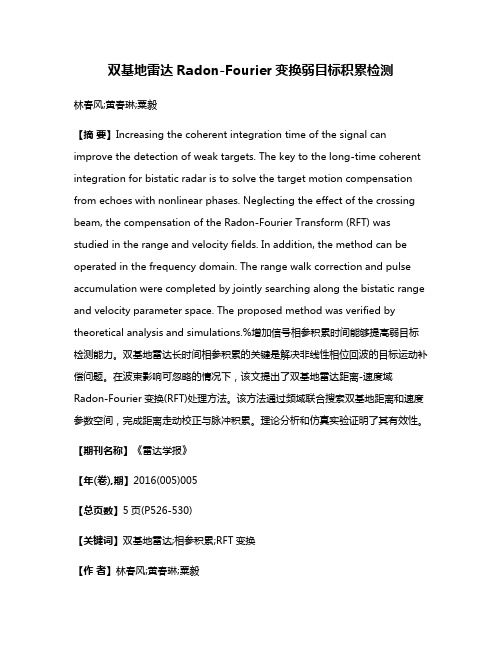
双基地雷达Radon-Fourier变换弱目标积累检测林春风;黄春琳;粟毅【摘要】Increasing the coherent integration time of the signal can improve the detection of weak targets. The key to the long-time coherent integration for bistatic radar is to solve the target motion compensation from echoes with nonlinear phases. Neglecting the effect of the crossing beam, the compensation of the Radon-Fourier Transform (RFT) was studied in the range and velocity fields. In addition, the method can be operated in the frequency domain. The range walk correction and pulse accumulation were completed by jointly searching along the bistatic range and velocity parameter space. The proposed method was verified by theoretical analysis and simulations.%增加信号相参积累时间能够提高弱目标检测能力。
双基地雷达长时间相参积累的关键是解决非线性相位回波的目标运动补偿问题。
在波束影响可忽略的情况下,该文提出了双基地雷达距离-速度域Radon-Fourier变换(RFT)处理方法。
红外图像中弱小目标检测前跟踪算法研究综述概要
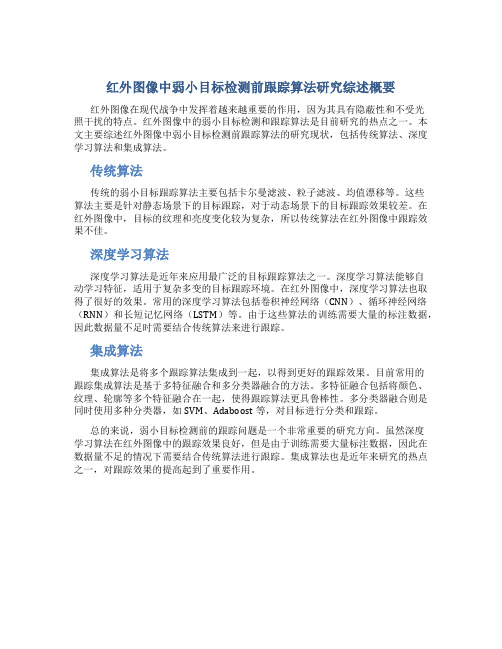
红外图像中弱小目标检测前跟踪算法研究综述概要红外图像在现代战争中发挥着越来越重要的作用,因为其具有隐蔽性和不受光照干扰的特点。
红外图像中的弱小目标检测和跟踪算法是目前研究的热点之一。
本文主要综述红外图像中弱小目标检测前跟踪算法的研究现状,包括传统算法、深度学习算法和集成算法。
传统算法传统的弱小目标跟踪算法主要包括卡尔曼滤波、粒子滤波、均值漂移等。
这些算法主要是针对静态场景下的目标跟踪,对于动态场景下的目标跟踪效果较差。
在红外图像中,目标的纹理和亮度变化较为复杂,所以传统算法在红外图像中跟踪效果不佳。
深度学习算法深度学习算法是近年来应用最广泛的目标跟踪算法之一。
深度学习算法能够自动学习特征,适用于复杂多变的目标跟踪环境。
在红外图像中,深度学习算法也取得了很好的效果。
常用的深度学习算法包括卷积神经网络(CNN)、循环神经网络(RNN)和长短记忆网络(LSTM)等。
由于这些算法的训练需要大量的标注数据,因此数据量不足时需要结合传统算法来进行跟踪。
集成算法集成算法是将多个跟踪算法集成到一起,以得到更好的跟踪效果。
目前常用的跟踪集成算法是基于多特征融合和多分类器融合的方法。
多特征融合包括将颜色、纹理、轮廓等多个特征融合在一起,使得跟踪算法更具鲁棒性。
多分类器融合则是同时使用多种分类器,如SVM、Adaboost等,对目标进行分类和跟踪。
总的来说,弱小目标检测前的跟踪问题是一个非常重要的研究方向。
虽然深度学习算法在红外图像中的跟踪效果良好,但是由于训练需要大量标注数据,因此在数据量不足的情况下需要结合传统算法进行跟踪。
集成算法也是近年来研究的热点之一,对跟踪效果的提高起到了重要作用。
一种弱小目标智能检测识别方法与流程

一种弱小目标智能检测识别方法与流程一、引言随着人工智能技术的不断发展,弱小目标的智能检测识别在安防、无人驾驶、环境监测等领域具有重要意义。
由于弱小目标的特殊性,其在图像视频中的检测识别面临着诸多困难,如低对比度、模糊不清、光照不均等问题。
本文将介绍一种针对弱小目标的智能检测识别方法与流程,旨在提高其检测识别的精确度和效率。
二、弱小目标智能检测识别方法1. 数据预处理对输入的图像视频数据进行预处理,包括去噪、增强、光照校正等操作,以提高图像质量和对比度。
针对弱小目标的特点,需要采用特定的预处理方法,如低对比度增强、细节增强等。
2. 特征提取利用计算机视觉技术提取图像视频中的特征,包括颜色特征、纹理特征、形状特征等。
针对弱小目标的特殊性,需要选择对噪声和模糊具有鲁棒性的特征提取方法,如局部二值模式、HOG特征等。
3. 目标检测采用目标检测算法对输入的图像视频数据进行检测,如基于深度学习的卷积神经网络(CNN)、级联分类器(Cascaded Classifier)等。
针对弱小目标,需要调整算法参数和模型结构,以提高检测的灵敏度和准确度。
4. 目标识别对检测到的目标进行识别和分类,可以采用基于机器学习的分类器,如支持向量机(SVM)、k最近邻(K-Nearest Neighbor)等。
针对弱小目标,需要结合图像视频的上下文信息和特定的领域知识,提高识别的准确性。
三、弱小目标智能检测识别流程1. 数据采集获取包含弱小目标的图像视频数据,可以通过摄像头、传感器等设备进行采集,也可以利用已有的数据集进行实验和测试。
2. 数据预处理对采集到的图像视频数据进行预处理,包括去噪、增强、尺度归一化等操作,以提高后续处理的效果。
3. 特征提取利用预处理后的数据提取图像视频中的特征,例如颜色、纹理、形状等,为后续的目标检测和识别做准备。
4. 目标检测利用目标检测算法对提取到的特征进行检测,识别出图像视频中的弱小目标,并给出其位置和边界框。
红外图像中弱小目标检测技术研究

红外图像中弱小目标检测技术研究红外图像中弱小目标检测技术研究摘要:随着红外图像技术日益发展和应用的广泛,红外图像中弱小目标的检测问题日益引起研究者的关注。
传统的目标检测方法在红外图像中表现出较差的性能,特别是在检测弱小目标时更为困难。
因此,本文对红外图像中弱小目标检测技术进行了深入研究,提出了一种基于深度学习的弱小目标检测方法,并进行了实验验证,证明了该方法的有效性和优越性。
第一章引言1.1 研究背景红外图像具有遥感、夜间监测等领域的广泛应用,然而在红外图像中,弱小目标的检测一直是一个具有挑战性的问题。
传统的目标检测方法在红外图像中无法准确地识别出目标,在弱小目标的检测问题上表现尤为明显。
1.2 研究目的本文旨在探索一种能够有效检测红外图像中弱小目标的技术方法,提高目标检测的准确性和鲁棒性。
第二章相关概念和理论2.1 红外图像红外图像是一种由红外辐射产生的图像,它记录了被物体辐射出的红外能量,常用于军事、医学、环境监测等领域。
2.2 弱小目标弱小目标是指在红外图像中大小较小、明暗度较低、形状不规则等特征明显弱于背景的目标,例如小型无人机、远程火炮等。
第三章弱小目标检测方法研究3.1 传统的目标检测方法传统的目标检测方法主要包括基于特征提取与分类器的方法,如Haar特征和SVM(支持向量机)方法等。
然而,这些方法对于红外图像中的弱小目标检测效果较差。
3.2 基于深度学习的弱小目标检测方法近年来,深度学习技术在图像处理领域取得了巨大的突破。
本文提出了一种基于深度学习的弱小目标检测方法。
该方法采用卷积神经网络(CNN)进行特征提取,并利用目标检测器进行目标的定位和分类。
实验结果表明,该方法在红外图像中检测弱小目标的准确率和鲁棒性较传统方法有明显提高。
第四章实验与结果分析本文在红外图像数据集上进行了实验,比较了传统的目标检测方法和基于深度学习的弱小目标检测方法的性能。
实验结果表明,本文提出的方法在检测弱小目标方面具有明显的优势,能够准确地定位和识别红外图像中的弱小目标。
一种基于动态规划的弱目标检测方法
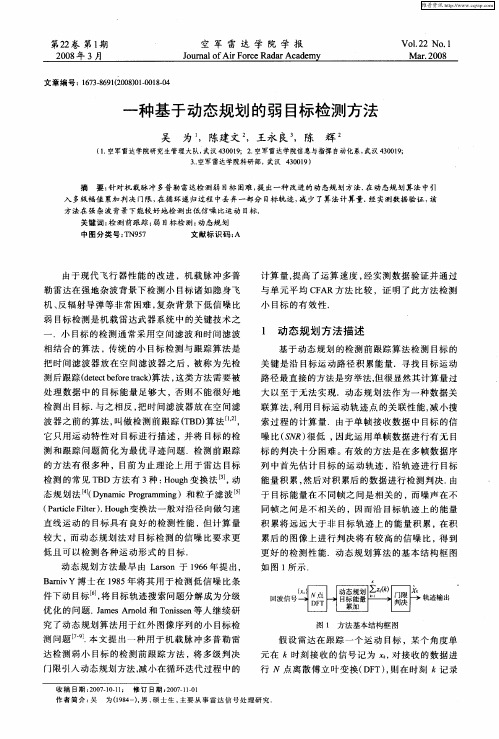
B riY博 士在 18 年将 其 用于 检测 低信 噪 比条 a v n 95
件下 动 目标 , 目标 轨迹搜索 问题分解 成为 分级 J 将
优 化 的问题 . a sA od T ns n等人 继续 研 Jme r l 和 o i e n s 究 了动态 规划 算法 用于红 外 图像序列 的小 目标 检
相 结合 的算 法 , 统 的小 目标检 测与 跟踪 算法 是 传
把 时 间滤 波器放 在 空 间滤波器 之后 , 被称 为先 检 测 后跟踪 (e cb f erc) 法 , dt teo t k算 e r a 这类方法 需要 被 处 理 数据 中 的 目标 能量 足够 大 ,否则 不 能很好 地 检 测 出 目标 . 与之 相反 , 时间滤 波器放 在空 间滤 把 波 器 之前 的算 法, 叫做检 测前 跟踪 (B ) 法 u J TD算 ., 2 它只用 运 动特性 对 目标进 行描 述 , 将 目标 的检 并
VO12 . 2 No. 1 Ma. 0 r20 8
一
种基于动态规划的弱 目标检测方法
吴 为 ,陈建文 ,王永 良 ,陈 辉
(. 1 空军雷达学院研究生管理大 队, 武汉 4 0 1;2 空军雷达学院信息与指挥 自动化系, 309 . 武汉 4 0 1 ; 309 3 空军雷达学院科研部, . . 武汉 4 0 1 ) 3 0 9
维普资讯
第2 卷 第 1 2 期 20 年 3月 08
文 章编 号 : 6389 (080 . 1.4 17.6 120)1 0 80 0
空 军 雷 达 学 院 学 报
J un l f r oc d r a e o r a r eRa a o Ai F Ac d my
基于改进子空间投影的无源雷达弱目标检测方法
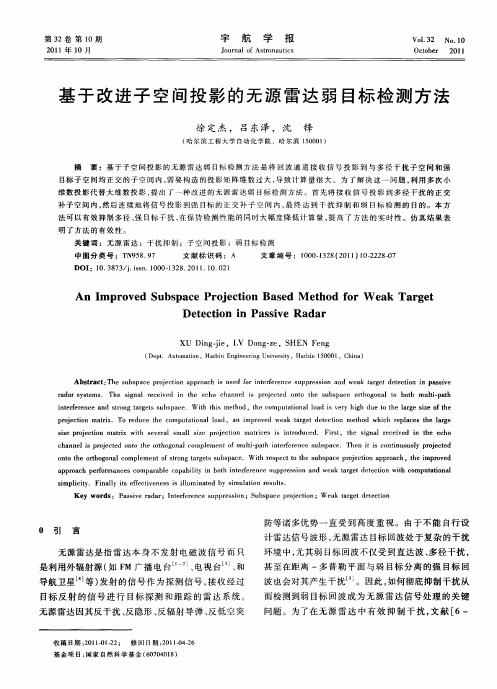
An I r v d S b p c r j cin B sd M eh d frW e k T r e mp o e u s a eP o e t a e t o a a g t o o
De e to n Pa sv d r t c i n i s i e Ra a
a pr c e o ma c sc m p r b e c p blt n boh i tree e s p e so n a a g td tci n wih c m p tto a p oa h p r r n e o f aa l a a iiy i t n e r nc u prs in a d we k tre ee to t o f ua in l smp iiy Fi al t f ci e e s i lu n td b i l t n rs ls i lct . n ly is ef tv n s s i mi ae y smu ai e ut . e l o
poet n mar .T e u etec mp tt n lla rjci t x ord c h o uai a o d,a mpo e e k tre ee t n meh d whc e lcstelre o i o n i rv d w a agtd tci to ih rpae h ag o sz rjcin mar t eea mal ie poet n mar e s it d cd Frt h s n lrc ie n te eh i p oet ti wi s vrls l z rjci tc s i nr u e . is,te i a e ev d i h c o e o x h s o i o g c a n lspoc td o t h rhg n lc mpe n fmu ip t nefrn esb p c .T e tsc niu ul mjoe h n e i rjoe no teotoo a o lme t h —ah itr e c u sa e h n i i o t o syp c td o e n o t h rh gn l o lme to togtressb p c .Wi ep c h u s aepoe t na po c tei rv d noteoto o a mpe n fs n agt u s ae c r t rse totes bp c rjci p ra h, h mp oe h t o
基于形态成分稀疏表示的红外小弱目标检测

关键 词 : 小 弱 目标 检 测 ; 稀疏表示 ; 形 态成分分析 ; 自适 应 分 类 字典
中图分类号 : T P 3 9 1 . 4 文献标志码 : A
I nf r a r e d Di m Ta r g e t De t e c t i o n Ba s e d o n Mo r p h o l o g i c a l
A n e f f i c i e n t me t h o d b a s e d o n mo r p h o l o g i c a l c o mp o n e n t a n a l y s i s( MC A)wa s p r o p o s e d f o r i n f r a r e d d i m t a r g e t d e t e c t i o n i n t h i s p a p e r ,c o m—
基 于 形态 成 分 稀 疏 表 示 的 红 外 小 弱 目标检 测
李 正周 , 王会 改 , 刘 梅 , 丁 浩 , 金 钢
( 1重 庆 大 学 通 信 工 程 学 院 , 重庆 4 0 0 0 4 4 ; 2中 国 空 气 动 力 研 究 与 发 展 中心 , 四川 绵 阳 6 1 0 2 0 9 ) 6 2 1 0 0 0 ; 3中 国科 学 院 光 电技 术 研 究 所 , 成都
第3 3卷
第 4期
弹箭Biblioteka 与制导学
报
V0 1 . 3 3 No . 4 Au g 2 01 3
2 0 1 3年 8月
J o u r n a l o f P r o j e c t i l e s ,R o c k e t s ,Mi s s i l e s a n d Gu i d a n c e
- 1、下载文档前请自行甄别文档内容的完整性,平台不提供额外的编辑、内容补充、找答案等附加服务。
- 2、"仅部分预览"的文档,不可在线预览部分如存在完整性等问题,可反馈申请退款(可完整预览的文档不适用该条件!)。
- 3、如文档侵犯您的权益,请联系客服反馈,我们会尽快为您处理(人工客服工作时间:9:00-18:30)。
A High-resolution Weak Signal Detection
Method Based on Stochastic Resonance
Key works: weak signals, stochastic resonance, superhet technology
Ⅰ. INTRODUCTION
Compared with noise, the amplitude of a signal is weak; the signal is completely submerged in the noise. On signal detection, the detection of the particular frequency is the most important.
In the past, the weak signal detection in general focused on the methods of reducing noise to improve the signal to noise ratio (SNR), but often damaging the actual signal in the process of suppressing noise. Stochastic resonance (SR) in this bistable system can take advantage of the noise energy to improve the signal detection capability. But stochastic resonance can only be used for the low frequency signals, thus, its utility has limited application[1-2]. In this paper, the frequency range of
The concept of stochastic resonance was put forward by the Italian physicist Roberto Benzi, American physicist Alfonso Sutera and Italian physicist Angelo Vulpoiani in the study of ancient meteorological glaciers in 1981 [1].
2Harbin Institute of Technology 3University of Science and Technology of China
Abstract-A detection method based on the strong low-frequency weak-signal detection ability of stochastic resonance is proposed, aimed at the detection of the unknown weak signal. By mixing the unknown signal with a continuous linear changing local oscillator signal, a difference frequency signal will be generated, which will be sent to the stochastic resonance system. Thus, an obviously changed output can be obtained. Because the stochastic resonance system is extremely sensitive to low frequency, the system will have a maximum output when the local oscillator and frequency of the unknown signal are closest. The frequency of the unknown signal will be measured precisely from the local oscillator frequency and the difference frequency. It can be inferred from the theoretical analysis and numerical simulation that this method has a large detection range, high resolution, and good prospect.
detection of stochastic resonance system is discussed and recommendations are made for a high-frequency weak signal detection method.
Ⅱ. THE PRINCIPLE OF STOCHASTIC RESONANCE
and Superhet Technology
12Shuo Shi, 12Wanyi Yin, 12Mingchuan Yang, 3Mingjie He 1National Key Laboratory of Communication System and Information Control Technology
������ ������ = 1 ������������2 + 1 ������������4 − ������(������������������������ ������������ + ������(������))
2
4
(2)
329
978-1-4673-2699-5/12/$31.00 © 2012 IEEE
������������ ������������
=
������������
−
������������3
+
������������������������(������0������)
+
������(������)
(1)
In equation (1), a,b are a real numbers representing the shape parameters of the potential well (In figure1, ������ = 1, ������ = 1 ). ������ ������ = ������������������������ 2������������0������ , ������(������) is the Gaussian white noise. Among them. ������ is amplitude of signals, ������0 is modulation frequency. The potential function of a bistable system is shown as follows.
4������
states������ = ±
������ ������
,
and
the
output
state
oftheFra biblioteksystem
should
be determined by the initial state. The signal and noise that are gradually increased or adjusted; the shape parameters cause the potential well to form, and this makes the particles go back and forth between the two potential wells. Since the potential difference between the potential wells of the bistable systems is much larger than the amplitude of the signal input, it makes the amplitude of the output signal far larger than the input. So the input signal is amplified effectively. Meanwhile, effectively suppressing the noise in the system output, transferring less energy of noise into signal, and enhances the SNR[4].
Stochastic resonance describes the phenomenon of back and forth transition between noise and the periodic signal, in non-linear bistable system under overdamped Brownian particle motion[4]. The potential curve of the non-linear bistable system and the transitions curve of particlse between the two potential wells is shown in Fig.1. x(t) is the position of the output particles at any time, and this introduces the equation describing the particle motion-Langevin equation[5-6].