基于位置社交网络的上下文感知的兴趣点推荐_任星怡
移动应用中基于位置的社交网络分析与推荐

移动应用中基于位置的社交网络分析与推荐随着智能手机和移动应用的普及,人们对于社交网络的需求越来越强烈。
在移动应用中,基于位置的社交网络成为了一种非常受欢迎的功能,用户可以通过该功能找到身边的朋友、参加附近的活动、发现感兴趣的地点等。
而对于移动应用开发者来说,基于位置的社交网络分析和推荐功能则是提高用户体验和吸引用户的重要手段。
基于位置的社交网络首先需要对用户的位置信息进行采集和处理。
通过手机定位技术,可以准确获取用户的地理坐标。
然后,基于位置的社交网络应用可以利用这些位置信息来建立用户之间的连接和关系。
通过手机的传感器数据,我们能够获得用户的行为数据,例如用户在某个地点停留的时间、用户与其他用户之间的距离以及用户在不同地点的访问频率等。
这些信息可以被用于分析用户的兴趣、行为模式和社交关系。
在基于位置的社交网络中,分析用户的社交关系可以帮助用户找到身边的朋友和共同兴趣的人。
通过分析用户的位置数据和社交网络数据,我们可以推断用户与其他人之间的联系强度。
例如,如果用户经常在某个地方停留,而这个地方也是其他用户常去的地方,那么可以认为这些用户之间有共同的兴趣爱好,或者是具有某种社交关系。
基于这些分析结果,我们可以向用户推荐其他用户,或者是向用户推送消息,提醒他们附近有可能感兴趣的人或者活动。
另一方面,基于位置的社交网络还可以通过分析用户的行为模式来推荐适合用户的地点或者活动。
根据用户的位置数据和行为数据,我们可以得到用户常去的地方、用户喜欢的活动类型以及用户的兴趣偏好等信息。
基于这些信息,我们可以将用户的行为模式与其他用户的行为模式进行比较,找到与用户兴趣匹配的地点或者推荐其他用户的行为。
这样,我们可以向用户推荐适合他们的地点、活动或者其他用户,提高用户的满意度和参与度。
在实现基于位置的社交网络分析和推荐功能时,数据隐私和信息安全是一个重要的考虑因素。
用户的位置和行为数据是非常敏感的个人信息,需要保证在传输和存储过程中的安全性。
基于上下文感知和个性化度量嵌入的下一个兴趣点推荐

基于上下文感知和个性化度量嵌入的下一个兴趣点推荐鲜学丰;陈晓杰;赵朋朋;杨元峰;Victor S.Sheng【期刊名称】《计算机工程与科学》【年(卷),期】2018(040)004【摘要】随着基于位置的社交网络推荐系统的逐步发展,兴趣点推荐成为了研究热门.兴趣点推荐的研究旨在为用户推荐兴趣点,并且为商家提供广告投放和潜在客户发掘等服务.由于用户签到行为的数据具有高稀疏性,为兴趣点推荐带来很大的挑战.许多研究工作结合地理影响、时间效应、社会相关性等方面的因素来提高兴趣点推荐的性能.然而,在大多数兴趣点推荐的工作中,用户访问的周期性习惯和伴随用户偏好的上下文情境信息没有被深度地挖掘.而且,下一个兴趣点推荐中一直存在着数据的高稀疏度.基于以上考虑,针对用户签到的数据稀疏性问题,将用户周期性行为模式归结为上下文情境信息,提出了一种基于上下文感知的个性化度量嵌入推荐算法,同时将用户签到的上下文情境信息考虑进来,从而丰富有效数据,缓解数据稀疏性问题,提高推荐的准确率,并且进一步优化算法,降低时间复杂度.在两个真实数据集上的实验分析表明,本文提出的算法具有更好的推荐效果.%With the rapid development of Location-Based Social Networks (LBSN) recommender system,Point-of-Interest (POI) recommendation has become a hot topic.The research of POI recommendation aims to recommend POIs for users and to provide services such as advertising and potential customer discovery.Due to the high data sparseness of users' check-ins,POI recommendation faces a great challenge.Many researches combine geographical influence,time awareness,social relevance and other factors to improve the performanceof POI recommendation.However,in most POI recommendation researches,the periodicity of mobility and the user preference varying with the change of contextual scenario have not been excavated indepth.Moreover,there exists high data sparseness in Next POI recommendation.Based on the above considerations,this paper proposes a Context-aware Personalized Metric Embedding (CPME) algorithm,which is based on the user's periodic behavior pattern.It takes into account the contextual information of users' check-ins,which can enrich the valid data,alleviate the data sparseness,improve the recommendation accuracy,and further optimize the algorithm to reduce the time complexity.The experimental analysis on two real LBSN datasets show that the proposed algorithm has better recommendation performance.【总页数】10页(P616-625)【作者】鲜学丰;陈晓杰;赵朋朋;杨元峰;Victor S.Sheng【作者单位】江苏省现代企业信息化应用支撑软件工程技术研发中心,江苏苏州215104;苏州大学智能信息处理及应用研究所,江苏苏州215006;苏州大学智能信息处理及应用研究所,江苏苏州215006;江苏省现代企业信息化应用支撑软件工程技术研发中心,江苏苏州215104;阿肯色中央大学计算机科学系,康威72035【正文语种】中文【中图分类】TP391.7【相关文献】1.基于位置社交网络的上下文感知的兴趣点推荐 [J], 任星怡;宋美娜;宋俊德2.基于位置社交网络的个性化兴趣点推荐 [J], 韩笑峰;牛保宁;杨茸3.基于位置的社交网络的个性化兴趣点推荐算法研究 [J], 李丹霞4.基于多维上下文感知图嵌入模型的兴趣点推荐 [J], 陈劲松;孟祥武;纪威宇;张玉洁5.基于时空循环神经网络的下一个兴趣点推荐方法 [J], 柴瑞敏;殷臣;孟祥福;张霄雁;关昕;齐雪月因版权原因,仅展示原文概要,查看原文内容请购买。
融合隐性和显性社交信息的连续兴趣点推荐方法研究

Copyright © 2020 by author(s) and Hans Publishers Inc. This work is licensed under the Creative Commons Attribution International License (CC BY 4.0). /licenses/by/4.0/
School of Management, Donghua University, Shanghai
Received: Nov. 27th, 2020; accepted: Dec. 14th, 2020; published: Dec. 21st, 2020
*通讯作者。
文章引用: 谷敏, 郑建国. 融合隐性和显性社交信息的连续兴趣点推荐方法研究[J]. 计算机科学与应用, 2020, 10(12): 2212-2226. DOI: 10.12677/csa.2020.1012233
Computer Science and Application 计算机科学与应用, 2020, 10(12), 2212-2226 Published Online December 2020 in Hans. /journal/csa https:///10.12677/csa.2020.1012233
现实中人类运动呈现序列模式的特点:在时间上,用户访问的兴趣点存在先后顺序;在空间上,用 户访问的兴趣点存在远近关系。而当前兴趣点推荐研究大多忽视了用户签到数据的序列性和连续性。因 此出现了通用兴趣点推荐的一个延伸问题——连续兴趣点推荐(successive POI recommendation) [2],基于 时间连续性特征的时空序列模式用于兴趣点推荐,其主要目的是在给定时间下,通过挖掘用户的历史签 到记录以及其他类型的相关信息,预测用户下一个可能访问的地点。
基于位置社交网络的上下文感知的兴趣点推荐
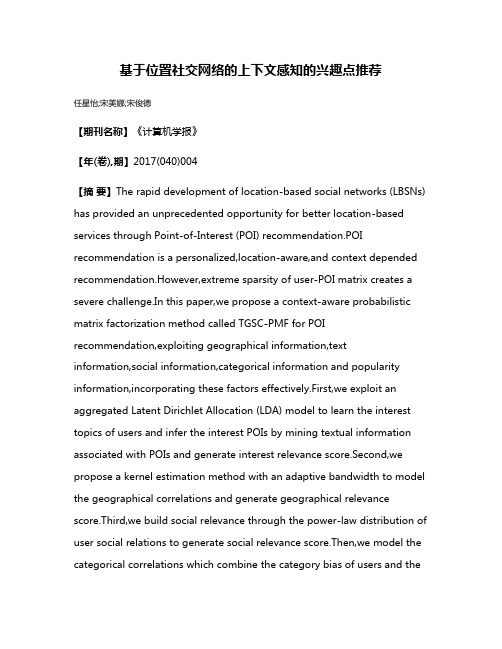
基于位置社交网络的上下文感知的兴趣点推荐任星怡;宋美娜;宋俊德【期刊名称】《计算机学报》【年(卷),期】2017(040)004【摘要】The rapid development of location-based social networks (LBSNs) has provided an unprecedented opportunity for better location-based services through Point-of-Interest (POI) recommendation.POI recommendation is a personalized,location-aware,and context depended recommendation.However,extreme sparsity of user-POI matrix creates a severe challenge.In this paper,we propose a context-aware probabilistic matrix factorization method called TGSC-PMF for POI recommendation,exploiting geographical information,text information,social information,categorical information and popularity information,incorporating these factors effectively.First,we exploit an aggregated Latent Dirichlet Allocation (LDA) model to learn the interest topics of users and infer the interest POIs by mining textual information associated with POIs and generate interest relevance score.Second,we propose a kernel estimation method with an adaptive bandwidth to model the geographical correlations and generate geographical relevance score.Third,we build social relevance through the power-law distribution of user social relations to generate social relevance score.Then,we model the categorical correlations which combine the category bias of users and thepopularity of POIs into categorical relevance score.Further,we exploit probabilistic matrix factorization model (PMF) to integrate the interest,geographical,social and categorical relevance scores for POI recommendation.Finally,we implement experiments on a real LBSN check-in dataset.Experimental results show that TGSC-PMF achieves significantly superior recommendation quality compare to other state-of-the-art POI recommendation techniques.%随着基于位置社交网络(Location-Based Social Networks,LBSN)的快速发展,兴趣点(Point-of-Interest,POI)推荐为基于位置的服务提供了前所未有的机会.兴趣点推荐是一种基于上下文信息的位置感知的个性化推荐.然而用户-兴趣点矩阵的极端稀疏给兴趣点推荐的研究带来严峻挑战.为处理数据稀疏问题,文中利用兴趣点的地理、文本、社会、分类与流行度信息,并将这些因素进行有效地融合,提出一种上下文感知的概率矩阵分解兴趣点推荐算法,称为TGSC-PMF.首先利用潜在狄利克雷分配(Latent Dirichlet Allocation,LDA)模型挖掘兴趣点相关的文本信息学习用户的兴趣话题生成兴趣相关分数;其次提出一种自适应带宽核评估方法构建地理相关性生成地理相关分数;然后通过用户社会关系的幂律分布构建社会相关性生成社会相关分数;另外结合用户的分类偏好与兴趣点的流行度构建分类相关性生成分类相关分数,最后利用概率矩阵分解模型(Probabilistic Matrix Factorization,PMF),将兴趣、地理、社会、分类的相关分数进行有效地融合,从而生成推荐列表推荐给用户感兴趣的兴趣点.该文在一个真实LBSN签到数据集上进行实验,结果表明该算法相比其他先进的兴趣点推荐算法具有更好的推荐效果.【总页数】18页(P824-841)【作者】任星怡;宋美娜;宋俊德【作者单位】北京邮电大学计算机学院信息网络工程研究中心教育部重点实验室北京 100876;北京邮电大学计算机学院信息网络工程研究中心教育部重点实验室北京 100876;北京邮电大学计算机学院信息网络工程研究中心教育部重点实验室北京 100876【正文语种】中文【中图分类】TP311【相关文献】1.位置社交网络中基于评论文本的兴趣点推荐 [J], 王啸岩;袁景凌;秦凤2.一种基于位置社交网络融合多种情景信息的兴趣点推荐模型 [J], 陈志雄;曾诚;高榕3.基于位置社交网络的个性化兴趣点推荐 [J], 韩笑峰;牛保宁;杨茸4.位置社交网络中谱嵌入增强的兴趣点推荐算法 [J], 刘真; 王娜娜; 王晓东; 孙永奇5.基于位置的社交网络的个性化兴趣点推荐算法研究 [J], 李丹霞因版权原因,仅展示原文概要,查看原文内容请购买。
基于用户兴趣和兴趣点流行度的个性化旅游路线推荐
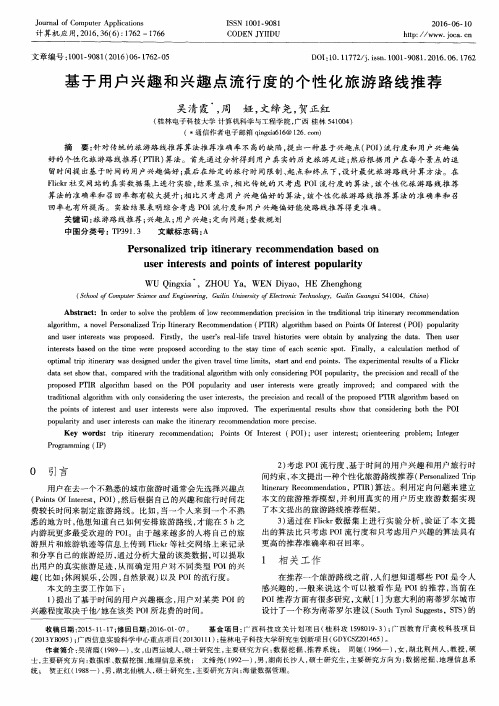
and user interests was proposed. Firstly, the user's real—life travel histories were obtain by analyzing the data. Then user interests based On the time were proposed according to the stay time of each scenic spot. Finally, a calculation method of optimal trip itinerary was designed under the given travel time limits, start and end points. The exper imental results of a Flickr data set show that, compared with the traditional algorithm with only consider ing POI popularity,the precision and reca ll of the proposed PTIR algorithm based on the POI popularity and user interests were greatly improved; and compared with the traditional algor ithm with only considering the user interests. the precision and recall of the proposed PTIR algorithm based on the points of interest and user interests were also improved. The experimental results show that considering both the POI popularity and user interests can make the itinerary recommendation more precise.
位置社交网络中基于评论文本的兴趣点推荐
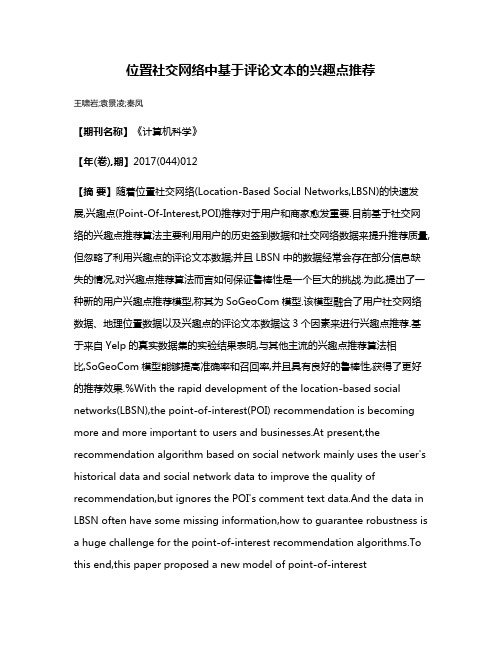
位置社交网络中基于评论文本的兴趣点推荐王啸岩;袁景凌;秦凤【期刊名称】《计算机科学》【年(卷),期】2017(044)012【摘要】随着位置社交网络(Location-Based Social Networks,LBSN)的快速发展,兴趣点(Point-Of-Interest,POI)推荐对于用户和商家愈发重要.目前基于社交网络的兴趣点推荐算法主要利用用户的历史签到数据和社交网络数据来提升推荐质量,但忽略了利用兴趣点的评论文本数据;并且LBSN中的数据经常会存在部分信息缺失的情况,对兴趣点推荐算法而言如何保证鲁棒性是一个巨大的挑战.为此,提出了一种新的用户兴趣点推荐模型,称其为SoGeoCom模型.该模型融合了用户社交网络数据、地理位置数据以及兴趣点的评论文本数据这3个因素来进行兴趣点推荐.基于来自Yelp的真实数据集的实验结果表明,与其他主流的兴趣点推荐算法相比,SoGeoCom模型能够提高准确率和召回率,并且具有良好的鲁棒性,获得了更好的推荐效果.%With the rapid development of the location-based social networks(LBSN),the point-of-interest(POI) recommendation is becoming more and more important to users and businesses.At present,the recommendation algorithm based on social network mainly uses the user's historical data and social network data to improve the quality of recommendation,but ignores the POI's comment text data.And the data in LBSN often have some missing information,how to guarantee robustness is a huge challenge for the point-of-interest recommendation algorithms.To this end,this paper proposed a new model of point-of-interestrecommendation,called SoGeoCom model.The model combines the user's social network data,geographic location data and the POI's comment text data to carry on the POI recommendation.Experimental results based on real data set from Yelp show that,compared with other mainstream POI recommendation models,the SoGeoCom model can improve the precision and recall rate,have good robustness,and get a better recommendation effect.【总页数】5页(P245-248,278)【作者】王啸岩;袁景凌;秦凤【作者单位】武汉理工大学计算机科学与技术学院武汉430070;武汉理工大学计算机科学与技术学院武汉430070;武汉理工大学计算机科学与技术学院武汉430070【正文语种】中文【中图分类】TP311【相关文献】1.基于位置社交网络的上下文感知的兴趣点推荐 [J], 任星怡;宋美娜;宋俊德2.一种融合情景和评论信息的位置社交网络兴趣点推荐模型 [J], 高榕;李晶;杜博;余永红;宋成芳;丁永刚3.一种基于位置社交网络融合多种情景信息的兴趣点推荐模型 [J], 陈志雄;曾诚;高榕4.基于位置社交网络的个性化兴趣点推荐 [J], 韩笑峰;牛保宁;杨茸5.基于位置的社交网络的个性化兴趣点推荐算法研究 [J], 李丹霞因版权原因,仅展示原文概要,查看原文内容请购买。
在线社交网络中用户地理位置预测综述

在线社交网络中用户地理位置预测综述
刘乐源;代雨柔;曹亚男;周帆
【期刊名称】《计算机研究与发展》
【年(卷),期】2024(61)2
【摘要】随着智能移动终端的日益普及,人们越来越多地利用社交网络平台(如Twitter、新浪微博等)获取信息、评论和交流.虽然全球卫星定位系统(GPS)设备能够精确获取位置信息,但是大量用户出于隐私和安全的考虑不会直接共享自己的位置信息.因此,如何获取在线用户的地理位置成为了一个前沿的研究领域以及学术界和工业界共同关注的重要课题,并且成为众多下游应用的基础,例如基于位置的定向广告投放、事件/地点的推荐、自然灾害或疾病预警和网络犯罪的追踪等.详细总结了预测社交网络用户地理位置的方法、数据、评价体系和基础算法.首先,归纳了不同的定位任务以及相应的评价指标;其次,针对不同的任务梳理所用的数据类型和数据融合方式,并且,详尽分析了已有的信息抽取和特征选择方式及其优缺点;再次,对现有定位模型和算法进行分类,从地名词典、传统机器学习和深度学习3个方面对用户定位方法进行阐述和分析;最后,总结了社交网络用户地理位置预测的难点和面临的挑战,并展望该领域的发展趋势和未来研究所需要关注的方向.
【总页数】28页(P385-412)
【作者】刘乐源;代雨柔;曹亚男;周帆
【作者单位】电子科技大学信息与软件工程学院;中国科学院信息工程研究所【正文语种】中文
【中图分类】TP391
【相关文献】
1.基于社交网络和地理位置的用户关系预测
2.社交网络用户认知域特征预测研究综述
3.社交网络用户在在线媒体中点播行为预测
4.在线社交网络用户分析研究综述
5.在线社交网络信息流行度预测综述
因版权原因,仅展示原文概要,查看原文内容请购买。
融合地理位置与社交关系的兴趣点推荐算法
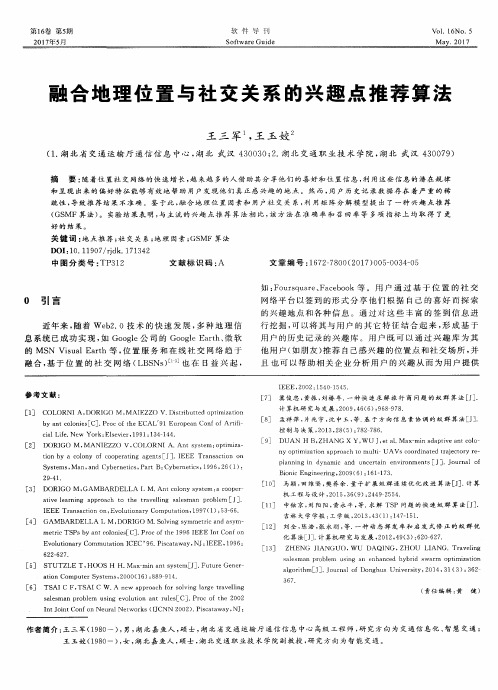
[ 7 ] 冀俊忠 , 黄振 , 刘椿年. 一种快速 求解旅 行商 问题的 蚁群 算法[ 刀.
计 算 机 研 究与 发 展 , 2 0 0 9 , 4 6 ( 6 ) : 9 6 8 9 7 8 .
[ 8 ] 孟祥萍 , 片兆宇, 沈 中玉 , 等. 基 于方 向 信 息 素 协 调 的 蚁 群 算 法 [ J ] .
融合 , 基于 位 置 的社 交 网络 ( L B S Ns ) 。 也 在 日益 兴企 业 分 析 用 户 的兴 趣 从 而 为 用 户 提 供
I EEE , 2 0 0 2: 1 5 4 0 1 5 4 5 .
参 考 文献
[ 1 ] C OL OR NI A, D ORI GO M, MAI E Z Z O V. Di s t r i b u t t e d o p t i mi z a t i o n
b y a n t c o l o n i e s [ C] . P r o c o f t h e E C AL 9 1 E u r o p e a n C o n f o f Ar t i f i —
c i a l Li f e . Ne w Yo r k: El s e vi e r , 1 9 9 1: 1 3 4 — 1 44 . DORI G0 M , M ANI EZ ZO V, COI ORNI A. Ant s y s t e m : o p t i mi z a —
控制与 决策, 2 O l 3, 2 8 ( 5 ): 7 8 2 7 86 .
E 2 ]
[ 9 ] DUAN H B, Z HANG x Y, WU J , e t a 1 . Ma x - ai r n a d a p t i v e a n t c o l o —
- 1、下载文档前请自行甄别文档内容的完整性,平台不提供额外的编辑、内容补充、找答案等附加服务。
- 2、"仅部分预览"的文档,不可在线预览部分如存在完整性等问题,可反馈申请退款(可完整预览的文档不适用该条件!)。
- 3、如文档侵犯您的权益,请联系客服反馈,我们会尽快为您处理(人工客服工作时间:9:00-18:30)。
计 算 机 学 报 CH I N E S E J OUR NA L O F C OMP UT E R S
V o l . 4 0 N o . 4 A r . 2 0 1 7 p
基于位置社交网络的上下文感知的兴趣点推荐
任星怡 宋美娜 宋俊德
R E N X i n i ONG M e i a ONG J u n e -Y S -N S -D g
( E n i n e e r i n R e s e a r c h C e n t e r o I n o r m a t i o n N e t w o r k s,M i n i s t r o E d u c a t i o n, S c h o o l o C o m u t e r S c i e n c e, g g f f y f f p ) B e i i n U n i v e r s i t o P o s t s a n d T e l e c o mm u n i c a t i o n s, B e i i n 0 0 8 7 6 w a r e P o i n t o f I n t e r e s t R e c o mm e n d a t i o n - - - L o c a t i o n B a s e d S o c i a l N e t w o r k s i n -
)h A b s t r a c t h e r a i d d e v e l o m e n t o f l o c a t i o n b a s e d s o c i a l n e t w o r k s( L B S N s a s a n r o v i d e d T - p p p u n r e c e d e n t e d o o r t u n i t f o r b e t t e r l o c a t i o n b a s e d s e r v i c e s t h r o u h P o i n t o f I n t e r e s t( P O I) - - - p p p y g , , r e c o mm e n d a t i o n. P O I r e c o mm e n d a t i o n i s a e r s o n a l i z e d l o c a t i o n a w a r e a n d c o n t e x t d e e n d e d - p p , r e c o mm e n d a t i o n.H o w e v e re x t r e m e s a r s i t o f u s e r P O I m a t r i x c r e a t e s a s e v e r e c h a l l e n e . I n - p y g ,w t h i s a e r e r o o s e a c o n t e x t a w a r e r o b a b i l i s t i c m a t r i x f a c t o r i z a t i o n m e t h o d c a l l e d T G S C - - p p p p p , , , e o r a h i c a l PMF f o r P O I r e c o mm e n d a t i o n e x l o i t i n i n f o r m a t i o n t e x t i n f o r m a t i o n s o c i a l g g p p g ,c ,i a t e o r i c a l i n f o r m a t i o n a n d o u l a r i t i n f o r m a t i o n n c o r o r a t i n t h e s e f a c t o r s i n f o r m a t i o n g p p y p g , e f f e c t i v e l . F i r s t w e e x l o i t a n a r e a t e d L a t e n t D i r i c h l e t A l l o c a t i o n( L D A)m o d e l t o l e a r n t h e y p g g g i n t e r e s t t o i c s o f u s e r s a n d i n f e r t h e i n t e r e s t P O I s b m i n i n t e x t u a l i n f o r m a t i o n a s s o c i a t e d w i t h p y g , a n d i n t e r e s t r e l e v a n c e s c o r e . S e c o n d w e a k e r n e l e s t i m a t i o n m e t h o d w i t h P O I s e n e r a t e r o o s e g p p a n a d a t i v e b a n d w i d t h t o m o d e l t h e e o r a h i c a l c o r r e l a t i o n s a n d e n e r a t e e o r a h i c a l r e l e v a n c e p g g p g g g p , s c o r e . T h i r d w e b u i l d s o c i a l r e l e v a n c e t h r o u h t h e l a w d i s t r i b u t i o n o f u s e r s o c i a l r e l a t i o n s o w e r - g p , t o e n e r a t e s o c i a l r e l e v a n c e s c o r e . T h e n w e m o d e l t h e c a t e o r i c a l c o r r e l a t i o n s w h i c h c o m b i n e t h e g g ,w c a t e o r b i a s o f u s e r s a n d t h e o u l a r i t o f P O I s i n t o c a t e o r i c a l r e l e v a n c e s c o r e .F u r t h e r e g y p p y g
4期
任星怡等 : 基于位置社交网络的上下文感知的兴趣点推荐
8 2 5
, , e x l o i t r o b a b i l i s t i c m a t r i x f a c t o r i z a t i o n m o d e l( PMF) t o i n t e r a t e t h e i n t e r e s t e o r a h i c a l p p g g g p ,w s o c i a l a n d c a t e o r i c a l r e l e v a n c e s c o r e s f o r P O I r e c o mm e n d a t i o n . F i n a l l e i m l e m e n t e x e r i m e n t s g y p p o n a r e a l L B S N c h e c k i n d a t a s e t .E x e r i m e n t a l r e s u l t s s h o w t h a t T G S C P M F a c h i e v e s s i n i f i c a n t l - - p g y u a l i t s u e r i o r r e c o mm e n d a t i o n c o m a r e t o o t h e r s t a t e o f t h e a r t P O I r e c o mm e n d a t i o n t e c h n i u e s . - - - q y p p q ;p ;t ; K e w o r d s l o c a t i o n b a s e d s o c i a l n e t w o r k o i n t o f i n t e r e s t r e c o mm e n d a t i o n o i c m o d e l - - - p y ; ; ; c o r r e l a t i o n s s o c i a l c o r r e l a t i o n s c a t e o r i c a l c o r r e l a t i o n s s o c i a l m e d i a e o r a h i c a l g g g p 相比 传 统 推 荐 系 统 的 发 展 , 兴趣点推荐系统的
; 收稿日期 : 在线出版日期 : 本课题得到国家 科 技 重 点 支 撑 项 目 ( 资 助. 任 星 怡, 女, 博 2 0 1 6 0 5 1 8 2 0 1 6 0 9 2 8. 2 0 1 4 B AK 1 5 B 0 1) 1 9 8 3年 生, - - - - : 士研究生 , 主要研究方向为推荐系统 、 数据挖掘 、 大数据 . 宋美娜, 女, 博士, 教授, 主要研究领域 E-m a i l x r e n@b u t . e d u. c n. 1 9 7 4年生, y p 为服务计算 、 云计算 、 超大规模信息服务系统 . 宋俊德 , 男, 博士 , 教授 , 主要研究领域为服务科学与工 程 、 云计算、 大数据、 物联 1 9 3 8 年生 , 网、 I C T 关键技术 .
( ) 北京邮电大学计算机学院信息网络工程研究中心教育部重点实验室 北京 1 0 0 8 7 6