遥感影像场景分类(IJEM-V8-N4-2)
使用遥感图像分类进行土地利用类型划分的方法

使用遥感图像分类进行土地利用类型划分的方法引言:近年来,随着遥感技术的快速发展和应用的广泛性,遥感图像分类在土地利用类型划分中起到了重要的作用。
通过遥感图像分类,可以快速、准确地获取大量土地利用信息,为城市规划、环境保护、农业生产等提供可靠的数据支持。
本文将介绍一种常用的遥感图像分类方法,并结合实际案例,探讨其应用效果和可行性。
一、遥感图像分类的基本原理遥感图像分类是指根据遥感图像中的光谱、几何、纹理等特征,将图像中的对象分为不同的类别。
一般而言,遥感图像分类可以分为有监督和无监督两种方法。
1.有监督分类方法有监督分类方法是指在分类过程中,需要事先提供样本数据集,并根据这些样本进行训练和分类。
常用的有监督分类方法有最大似然法、支持向量机、人工神经网络等。
2.无监督分类方法无监督分类方法是指在分类过程中,不需要提供样本数据集,系统通过数据的统计特性和聚类分析方法自动对图像进行分类。
常用的无监督分类方法有K-means 聚类、ISODATA聚类等。
二、实例分析:基于遥感图像分类的土地利用类型划分以某市区为例,使用高分辨率遥感图像进行土地利用类型的划分。
在进行分类前,首先对图像进行预处理,包括辐射校正、几何校正、大气校正等,以确保获取准确的数据。
然后,选择合适的特征提取方法,例如基于像素、对象、纹理等特征,将图像中的各类信息提取出来。
接下来,根据提取到的特征,使用有监督或无监督分类方法对图像进行分类。
在本例中,我们采用了最大似然法作为有监督分类方法。
首先,我们利用已知的样本数据对分类器进行训练,使其能够准确地区分不同的土地利用类型。
然后,使用分类器对整幅遥感图像进行分类,将其划分为不同的类别,如农田、居民区、工业区等。
在分类结果的基础上,我们可以进行后续的空间分析和统计分析。
例如,可以计算各类别的面积、周长和形状指数,进一步了解土地利用类型的分布和特征。
同时,还可以与地理信息系统(GIS)数据进行叠加分析,获得更全面和准确的土地利用信息。
遥感图像目视分类PPT课件
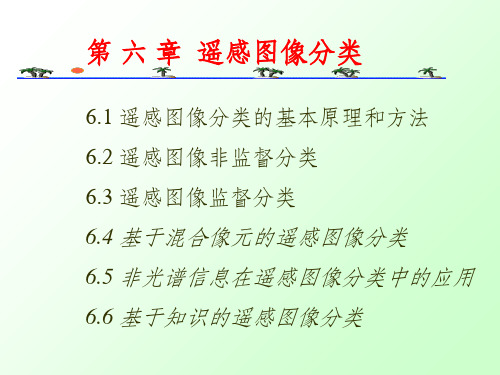
杭州TM影像
.
鄱阳湖区水田
.
◍ 图像分类的基本原理和方法
目视解译标志的建立
形状(shape)
用于判读的图像通常多是垂直拍摄的,因此遥感图 像上表现的目标地物形状是顶视平面图,它不同于
我们日常生活中经常看到的物体形状.
武汉城区卫星影像
.
◍ 图像分类的基本原理和方法
目视解译标志的建立 形状(shape)
用于判读的图像通常多是垂直拍摄的,因此遥感图 像由上于表成现像的方目式标的地不物同形、状飞是行顶姿视态平的面改图变,或它者不地同形于起 伏的变我化们都日会常造生成活同中一经目常标看物到在的图物像体上形呈状现. 出不同
形状,因此解译时须考虑遥感图像的成像方式.
.
◍ 图像分类的基本原理和方法
目视解译标志的建立
摄, 1米分辨率).
◍ 图像分类的基本原理和方法
目视解译标志的建立
色调(tone) 颜色(color)
遥感图像中目标地物的颜色是地物在不同波段中反射或发 射电磁辐射能量差异的综合反映,是判读遥感图像中目标 地物的基本识别标志.例如真彩色航空像片上,正常的针叶 林呈深绿色,枯萎的植被则呈现为黄绿色.
纹理
平滑
.
粗糙
◍ 图像分类的基本原理和方法
目视解译标志的建立 形状(shape)
大小(size)
纹理(texture)
图型(pattern)
——是指目标地物以一定规律排列而成的图形结构.
通例常如人住工宅地区物建往筑往群具在有图某像种上特呈殊现的的图图型型.,例农如田多与个周建边筑的物 有防序护排林列构构成成的的图街型区等以. 及地由物教的室图、型操揭场示和了跑不道同构地成物的之学 校间等的,它内们在都联具系有,也一为定解的译整者体识图别型这.些自地然物界提中供,一了些依地据物.也 具有特定的图型,如水系有树枝状、羽毛状和格网状等多 种图型,它们综合反映了流域内地质构造和水文特征.
遥感图像处理遥感图像分类

遥感图像的分类
遥感图像的分类
一.遥感图像分类概述 二.遥感图像分类原理 三.遥感图像分类基本过程 四.遥感图像分类方法 五.遥感图像分类后处理 六.遥感图像分类精度检查 七.遥感图像分类中存在的问题
一、遥感图像分类概述
1. 分类的定义 2. 分类的意义 3. 分类的难点
四、遥感图像分类方法
利用遥感图像进行分类(classification) 是以区别图像中所含的多个目标物为目的的, 对每个像元或比较匀质的像元组给出对应其特 征的名称。在分类中注重的是各像元的灰度及 纹理等特征。分类方法主要包括以下三大类:
1. 监督分类法 2. 非监督分类法 3. 新的探索:模糊分类法、面向对象分类法等;
分类原理—特征提取
➢ 统计特征变量可以构成特征空间,多波段遥感 图像特征变量可以构成高维特征空间。
➢ 一般说来,高维特征空间数据量大,但这些信 息中仅包含少量的样本分类信息。
➢ 为了抽取这些最有效的信息,可以通过变换把 高维特征空间所表达的信息内容集中在一到几 个变量图像上。
➢ 主成分变换可以把互相存在相关性的原始多波 段遥感图像转换为相互独立的多波段新图像, 而且使原始遥感图像的绝大部分信息集中在变 换后的前几个组分构成的图像上,实现特征空 间降维和压缩的目的。
(一)监督分类
➢监督分类法:选择具有代表已知地面覆盖类型 的训练样本区,用训练样本区中已知地面各类 地物样本的光谱特性来“训练”计算机,获得 识别各类地物的判别函数或模式(如均值、方 差、判别域等),并以此对未知地区的像元进 行分类处理,分别归入到已知具有最大相似度 的类别中。
➢监督分类主要包括:最小距离分类法、最近邻 分类法、多级切割分类法、最大似然比分类法 等;
《遥感图像分类》课件
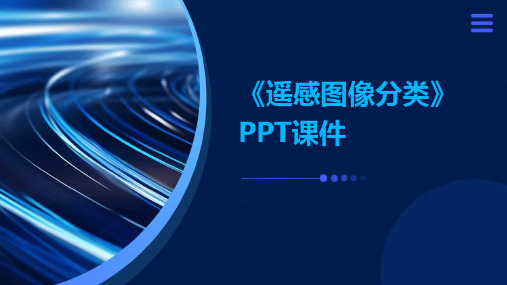
将原始特征进行变换,生成新的特征,以更好地 反映地物类别之间的差异。
分类器设计
监督分类
利用已知样本的训练集设计分类器,对未知样本进行分类。
非监督分类
对未知样本进行聚类分析,将相似的样本归为同一类。
混合分类
结合监督分类和非监督分类的优势,提高分类精度和稳定性。
分类结果评价
精度评价
通过比较分类结果与实际地物类别, 计算分类精度、混淆矩阵等指标。
THANKS
感谢观看
分类器。
多源遥感数据融合问题
多源遥感数据融合可以提高分类精度和可靠性,但同时也带 来了数据匹配、融合算法选择等问题。
解决多源遥感数据融合问题的策略包括使用先进的融合算法 ,如基于深度学习的融合方法,以及优化数据匹配方法。
遥感图像分类技术的发展趋势
01
遥感图像分类技术正朝着高精度、高效率和自动化的方向发展 。
可靠性评价
评估分类结果的稳定性、可靠性以及 抗干扰能力。
应用价值评价
根据分类结果在实际应用中的价值, 如土地利用、资源调查、环境监测等
,对分类方法进行综合评价。
04
CATALOGUE
遥感图像分类的挑战与展望
数据质量问题
遥感图像常常受到噪声、失真和 模糊等影响,导致数据质量下降
。
数据质量问题还表现在不同传感 器获取的图像之间的差异,以及 不同时间获取的图像之间的变化
遥感图像分类的应用
遥感图像分类在多个领域有广泛应用,如环境保护、城市规划、资源调查、军事 侦察等。
通过遥感图像分类,可以快速获取大范围的地物信息,为相关领域的决策提供科 学依据。
02
CATALOGUE
遥感图像分类的方法
遥感影像有关知识点总结
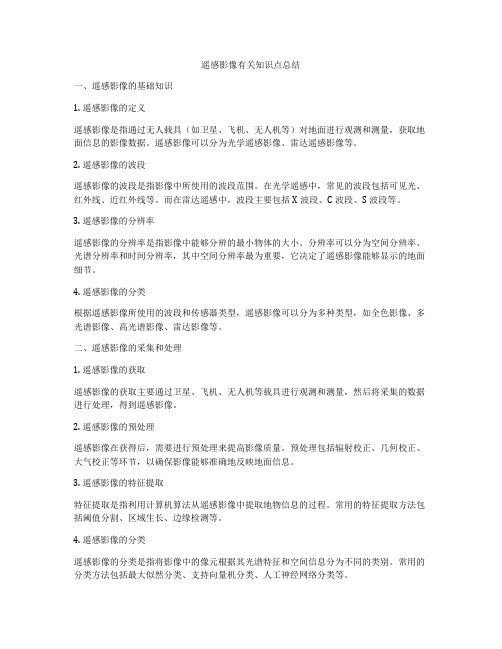
遥感影像有关知识点总结一、遥感影像的基础知识1. 遥感影像的定义遥感影像是指通过无人载具(如卫星、飞机、无人机等)对地面进行观测和测量,获取地面信息的影像数据。
遥感影像可以分为光学遥感影像、雷达遥感影像等。
2. 遥感影像的波段遥感影像的波段是指影像中所使用的波段范围。
在光学遥感中,常见的波段包括可见光、红外线、近红外线等。
而在雷达遥感中,波段主要包括X波段、C波段、S波段等。
3. 遥感影像的分辨率遥感影像的分辨率是指影像中能够分辨的最小物体的大小。
分辨率可以分为空间分辨率、光谱分辨率和时间分辨率,其中空间分辨率最为重要,它决定了遥感影像能够显示的地面细节。
4. 遥感影像的分类根据遥感影像所使用的波段和传感器类型,遥感影像可以分为多种类型,如全色影像、多光谱影像、高光谱影像、雷达影像等。
二、遥感影像的采集和处理1. 遥感影像的获取遥感影像的获取主要通过卫星、飞机、无人机等载具进行观测和测量,然后将采集的数据进行处理,得到遥感影像。
2. 遥感影像的预处理遥感影像在获得后,需要进行预处理来提高影像质量。
预处理包括辐射校正、几何校正、大气校正等环节,以确保影像能够准确地反映地面信息。
3. 遥感影像的特征提取特征提取是指利用计算机算法从遥感影像中提取地物信息的过程。
常用的特征提取方法包括阈值分割、区域生长、边缘检测等。
4. 遥感影像的分类遥感影像的分类是指将影像中的像元根据其光谱特征和空间信息分为不同的类别。
常用的分类方法包括最大似然分类、支持向量机分类、人工神经网络分类等。
5. 遥感影像的地物识别地物识别是指对遥感影像进行解译,识别影像中的地物类型。
常见的地物识别包括植被识别、水体识别、建筑物识别等。
6. 遥感影像的信息提取信息提取是指利用遥感影像获取地面信息,如地表覆盖类型、地面高程等。
信息提取可以借助数字高程模型、地物识别技术等手段。
三、遥感影像的应用1. 环境监测遥感影像可以用来监测大气污染、土壤侵蚀、植被覆盖等环境变化,为环境保护和治理提供数据支持。
遥感影像分类envi
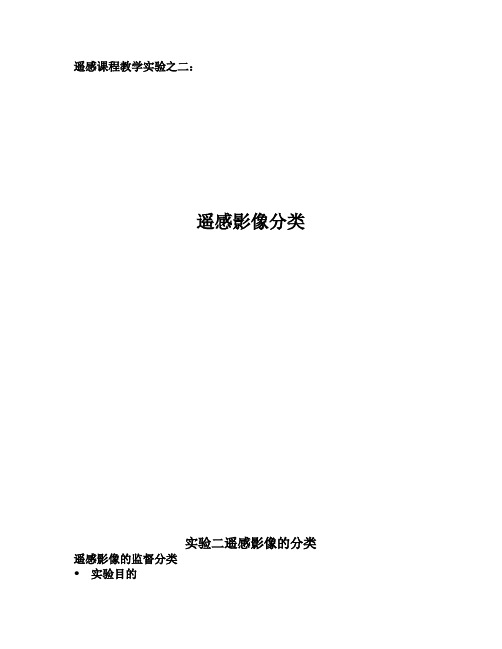
遥感课程教学实验之二:遥感影像分类实验二遥感影像的分类遥感影像的监督分类•实验目的理解计算机图像分类的基本原理以及监督分类的过程,学会利用遥感图像处理软ENVI件对遥感图像进行分类的方法。
•实验内容1、遥感图像分类原理。
2、遥感图像监督分类。
3、最大似然法分类•实验条件电脑、ENVI4.5软件。
厦门市TM遥感影像。
•实验步骤1、启动ENVI软件,从文件菜单打开多波段影像文件,从可用波段列表中装载彩色或假色影像,显示遥感影像。
2、从主图像窗口的工具Tools →Region of Interest →ROI Tools;3、在自动打开的ROI Tools窗口中,设定ROI_Type 为“Polygon”(多边形),选定样本采集的窗口类型,用Zoom(缩放窗口)进行采集。
4、在选定的窗口如Zoom用鼠标左键画出样本区域,在结束处击鼠标右键二次,样本区域被红色充填,同时ROI Tools窗口中显示采集样本的信息。
采集新的样本点击“New Region”,重新上述步骤进行多个地物样本采集。
5、从ENVI主菜单中,选 Classification > Supervised > Maximum Likelihood;或在端元像元采集对话框 Endmember Collection中选择 Algorithm >MaximumLikelihood 进行最大似然法分类。
6、在出现Classification Input File 对话框中,选择输入影像文件,出现 MaximumLikelihood Parameters 对话框。
7、输入常规的分类参数。
设定一个基于似然度的阈值(Set Prpbability Threshold):如不使用阈值,点击“None”按钮。
要对所有的类别使用同一个阈值,点击“Single Value”按钮,在“Probability Threshold”文本框中,输入一个0 到1 之间的值。
遥感图像的种类与特性
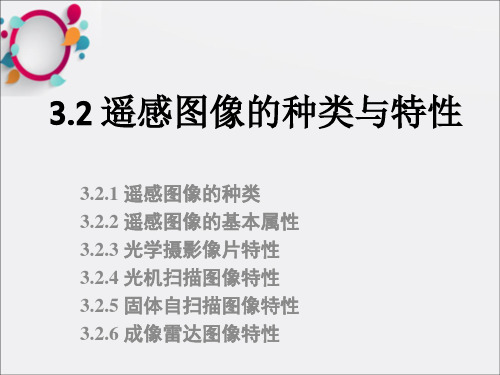
分辨率:5米,地面上每5米的物品在影像中占1个像素, 相当于视角高度约为4km
分辨率:2米,地面上每2米的物品在影像中占1个像素,相 当于视角高度约为1.8km
分辨率:1米,地面上每1米的物品在影像中占1个像素, 相当于视角高度约为500m
分辨率:0.5米,地面上每0.5米的物品在影像中占1个像素, 相当于视角高度约为300m
Google Earth的影像是卫星影像与航拍的数据整合. 卫星影像部分:QuickBird(快鸟)、LANDSAT-7等; 航拍部分:BlueSky公司(英国公司,以航拍、GIS/GPS相关业务为主)、Sanborn公
司、美国公DigitalGlobe公司的QuickBird(快鸟)、美国IKONOS及法国的SPOT5。
• (一)波谱特性(波谱分辨率辐射分辨率) • (二)空间特性 • (三)时间特性
㈠.波谱特性(波谱分辨率、辐射分辨率)
• 影像灰度或色彩差异——遥感图像上波谱特性差异。 实为其响应( 感测) 波段内电磁辐射能量大小的反映。
黑白全色像片 天然彩色像片 黑白红外像片 彩色红外像片
热红外图像
成像雷达图像
地形起伏越大,重叠率相应要加大。
旁向重叠
航向重叠
(三)帧幅式航片的空间特性
1.投影性质及比例尺 • 投影性质——地面的中心投影 • 比例尺:各处影像会出现不一致。
– 在地形起伏地区:各影像点相对航高不同--不同高程处地物影像比例尺不 同→地形起伏地区航片只能概略表示
中心投影与垂直投影的比较
◆两种投影方式比较,当投影面倾斜时,像片各部 分的比例尺变化不同,像片各部分的位移量(径向 距离)不等(倾斜误差)
二 者 等 比 例 与 不 等 比 例 之 缩 小
遥感影像图像分类算法的研究

遥感影像图像分类算法的研究遥感技术是指通过卫星、飞机等载体获取地面信息的一种技术手段。
遥感影像图像分类算法即是将遥感影像数据进行分类,为地物识别和应用提供数据支持。
遥感影像图像分类算法的研究已经成为遥感技术领域的一个热点问题,本文将对其进行探讨。
一、遥感影像分类算法的概述遥感影像图像分类算法是指对遥感影像数据进行分类识别的方法。
它是从遥感影像中提取出特征信息,经过预处理、特征选择和分类模型设计等步骤,最终将遥感影像数据划分为不同的类别,以实现对地表覆盖状况的识别。
遥感影像分类算法技术是遥感技术中的重要分支,能够处理大量的遥感影像数据信息,与地理信息系统(GIS)深度结合,为解决自然资源、环境保护等方面问题提供了强有力的支撑。
二、遥感影像分类算法的基本步骤遥感影像分类算法的基本流程通常包括:数据获取、数据预处理、特征选择、分类模型设计和分类结果评价等步骤。
1. 数据获取:指获取遥感影像数据源,如卫星数据、航空影像等。
2. 数据预处理:遥感影像数据中存在噪声、失真等问题,需要进行预处理,包括辐射校正、大气校正、几何校正、镶嵌处理等多种技术手段。
3. 特征选择:从遥感影像中提取有效信息,通常使用的特征包括颜色、形状、纹理、高度等。
4. 分类模型设计:根据特定的特征信息,设计相应的分类模型。
目前常用的分类模型主要包括最大似然分类、支持向量机分类、人工神经网络分类等。
5. 分类结果评价:对分类结果进行评价,主要包括分类精度、Kappa系数、混淆矩阵等指标。
三、遥感影像分类算法的应用遥感影像分类算法在土地利用、资源环境监测、城市规划、农业生产等领域具有广泛的应用。
1. 土地利用识别:遥感影像数据可以提供大量土地利用信息,通过遥感影像分类技术可以实现对地表覆盖区域的分类识别,如农田、林地、沙漠、城区等。
2. 资源环境监测:遥感影像分类技术可以用于监测水域面积、森林面积、土地利用面积等自然资源利用情况,也可以用于检测污染源、盐碱地等环境问题。
- 1、下载文档前请自行甄别文档内容的完整性,平台不提供额外的编辑、内容补充、找答案等附加服务。
- 2、"仅部分预览"的文档,不可在线预览部分如存在完整性等问题,可反馈申请退款(可完整预览的文档不适用该条件!)。
- 3、如文档侵犯您的权益,请联系客服反馈,我们会尽快为您处理(人工客服工作时间:9:00-18:30)。
I.J. Engineering and Manufacturing, 2018, 4, 13-20Published Online July 2018 in MECS ()DOI: 10.5815/ijem.2018.04.02Available online at /ijemRemote Sensing Image Scene ClassificationMd. Arafat Hussain, Emon Kumar Dey*Institute of Infromation Technology, University of Dhaka, Dhaka-1000, BangladeshReceived: 11 March 2018; Accepted: 06 June 2018; Published: 08 July 2018AbstractRemote sensing image scene classification has gained remarkable attention because of its versatile use in different applications like geospatial object detection, natural hazards detection, geographic image retrieval, environment monitoring and etc. We have used the strength of convolutional neural network in scene image classification and proposed a new CNN to classify the images. Pre-trained VGG16 and ResNet50 are used to reduce overfitting and the training time in this paper. We have experimented on a recently proposed NWPU-RESISC45 dataset which is the largest dataset of remote sensing scene images. This paper found a significant improvement of accuracy by applying the proposed CNN and also the approaches have applied.Index Terms: Convolutional Neural Network, Remote Sensing Image, Scene Classification, CNN.© 2018 Published by MECS Publisher. Selection and/or peer review under responsibility of the Research Association of Modern Education and Computer Science.1.IntroductionImage or picture is a visual representation anything. Human can see a complex picture, understand it and can interpret properly without thinking twice but it is a very difficult task for machine. A machine can learn from the provided labelled data. Due to machines learning capability, image recognition and classification has become an active research area.Image classification and recognition is important for various applications a like facial image classification, medical image classification, scene image classification, human pose classification, gender classification etc. Recently scene image classification has found remarkable attention for its growing importance. Researchers are trying to efficiently classify different types of scene images like natural scene, remote sensing scene, indoor scene etc.Satellite and airborne observing the earth and taking a huge amount of remote sensing scene images. With the development of modern satellite and airborne, high spatial resolution (HSR) remote sensing images can * Corresponding author.E-mail address: emonkd@iit.du.ac.bdprovide detailed information. Remote sensing image classification has great importance in many applications such as natural vegetation mapping, urban planning, geospatial object detection, hazards detection, geographic image retrieval and environment monitoring [1]. The effectiveness of these applications depends on the classification accuracy.The goal of the classification is to categorize a scene image in appropriate classes. In the past years, some datasets have proposed on remote sensing image. These datasets are very small and already found high accuracy using feature extraction based hand crafted methods. The benefits of deep learning approach cannot be found with these small datasets because of various reasons. Recently, few large datasets have been published and some models have already been applied also. But there is a huge scope to improve the performance of this dataset. For example, a recently published NWPU-RESISC45 dataset [2] with 311,000 images and 45 scene classes achieved 90.36% accuracy using fine tuning VGGNet-16 [3] which is the current state of the art solution.On the other hand, current state-of-the-art hand crafted feature extraction methods showing poor performance on large dataset for scene classification. For example, the NWPU-RESISC45 achieved only 24.84% accuracy using the colored histogram features of this dataset. So the major objective of this research is how we can improve the performances remote sensing dataset using hand engineered feature extraction method as well as deep learning architecture.Many experiments happened on focusing remote sensing scene image using different datasets. Local descriptors like local binary pattern, local ternary pattern, completed local binary pattern or histograms of oriented gradients have proven their worth in different scene classification. Hand engineered based feature extraction method was very popular for scene detection before the deep learning based approach was proposed. Still it is very attractive for its simplicities. Different researches have proposed different ways to solve this problem. Xiao et al. proposed the SUN for large scale scene detection in [4]. The dataset contains 899 categories of 130519 images. Which helped the scene detection research group of the world a lot. They also applied different feature extraction method to find the accuracy of the dataset and found a satisfactory state of the art result.Lienou et. al. [5] conducted experiment on satellite images using sematic concept. They applied latent dirichle allocation model is used to training for each class. The model basically obtains their feature by extracting image words. They obtained 96.5% accuracy which is comparatively support vector machine which gained 85% accuracy on QuickBird image dataset.Vatsavai et. al. [6] proposed an unsupervised way based on the Latent Dirichlet Allocation (LDA) method which is a semantic labelling framework. They collected 130 images of refineries, international nuclear plants, airports and coal power plants. Though there were reasonable overlapping between two classes they found 73% training accuracy and 67% testing accuracy.Deep learning has come with a revolutionary change in the field of machine learning. Accuracy of different datasets jumped after applying deep learning approach [21-23]. The typical Convolutional Neural Networks (ConvNet) including Alexnet [7], VGGNET [8], GoogleNet [9] has been applied for scene classification purpose. As, in most of the existing scene dataset number of images are small, the activations of different layers of ConvNet or their fusion considered as the visual representation sent to the classification layer of ConVNet. For example, in [10-12] the authors used deep learning model for scene classification based on 7 layer AlexNet or CaffeNet [13]. Castelluccio, Marco [14] achieved state of the art accuracy on UC Merced dataset and the Brazilian coffee scene dataset. They applied CaffeNet and GoogLeNet with various learning procedures on these datasets. They used these two network from scratch and also used fine-tuning on their experimental dataset and they used the procedure to reduce overfitting. They found 97.10% accuracy using fine-tuned GoogleNet on UC Merced dataset and 91.83% accuracy using GoogleNet on Brazilian coffee scene dataset.VGG architecture consisting 16 layers have also been applied in [15-16]. Wang, Limin, et al. used [17] VGG networks to find state of the art accuracy on Place205, MIT67 and SUN397. They used Caffe toolbox to design ConvNets. They designed three types of VGG nets named VGG11, VGG13, VGG16. Trained the VGG nets onPlace205 dataset. To reduce computation cost they loaded weight to VGG13 from VGG11 and from VGG13 to VGG16.Many deep learning based models have proposed to classify images of different dataset. But there is no specific model or approach which is best suited for all dataset. A deep knowledge in this field and many year research experience can give an appropriate path to design a good model for a dataset. In this paper we have focused on the improvement of the performances of large-scale image dataset. We have experimented on NWPU-RESISC45 using our proposed architecture of deep learning model. We have found a reasonable accuracy.2.MethodologyIn this paper we have applied two types of approaches to evaluate the dataset. We applied both transfer learning approach and training from the scratch. Transfer learning fine tunes the existing deep convolutional neural network models. We considered VGG16 and ResNet50 for the transfer learning. In 2.3 we described our proposed from the scratch. Following sub-sections describe the methodologies.2.1.Transfer Learning using Pre-trained VGG16 ModelWe fine-tuned the VGG16 model which was trained on ImageNet dataset [7]. As this dataset covers a huge variety of images, a model that is trained on this dataset with a good accuracy rate has a huge knowledge indeed. We just want to fit this knowledge for our dataset.We consider two factors, one is the size of the dataset another is the similarity with the ImageNet dataset. Our dataset is comparatively small and partially similar with ImageNet dataset. In this situation, partially training the VGG16 model will be best. We kept the convolutional part same as before but changed the fully connected layers. We replaced the first fully-connected layer with 256 neurons, second fully-connected layer with 256 neurons and third with 45 neurons. We replaced 4096 by 256 to reduce overfitting by reducing the parameters.2.2.Transfer Learning using Pre-trained ResNet50 ModelWe applied fine-tuning on pre-trained model not only changing the output layer and training the classifier but also training some layers near the last layer. Here last layer is replaced with six layers. After the last average pooling we added a global average pooling layer to reduce dimensionality of input. It also works an alternative of flatten layer by producing one dimensional vector from 3 dimension. Then a fully-connected layer is added with 256 neurons. After that a dropout layer is added and another fully-connected layer of 256 neurons follows the dropout layer. Another dropout layer follows the second fully connected layer. The last layer of the model is a fully-connected layer with 45 neurons with a softmax classifier.2.3.Proposed CNN model from ScratchThe model is a VGGNet based seven-layer convolutional neural network. First four layers are convolutional layer and other three are fully connected layers. There are four max-pooling layer followed by each convolutional layer. Two dropout layers are added which follows first two convolutional layers. Last fully-connected layer is basically classification layer which classify images into different classes. Figure 1 shows the basic architecture of our proposed model. Table 1 shows every layers, their output and parameters. Convolutional layers (marked in blue) and fully-connected layers (marked in yellow) are the skeleton of our model.Fig.1. Proposed ModelTable 1. Layers, Output Shape and Parameters of Proposed3.Experimental ResultsIn this paper we have used NWPU-RESISC45 dataset. We applied some hand engineered learning procedure and deep learning procedure in this experiment. In deep learning procedure we used two well-known deep learning model VGG16 and ResNet50. We, trained them from scratch as well as applied transfer learning for the dataset. We used Theano, Tensorflow as machine learning library, Keras as deep learning API. The experiment was implemented on Windows 10 operating system.3.1.DatasetNWPU-RESISC45 dataset is used in this experiment. It is one of the largest remote sensing image scene dataset. The dataset contains 31,500 images in total with 45 scene classes and each class contain 700 images. The dataset is developed from the inspiration of applying many data driven algorithms like deep learning. Scarcity of data severely limits the learning capability of deep learning models which was the main motivation behind this dataset. So, the dataset is produced to face the problem by combining a number of datasets. Firstly we have used twelve scene classes for our experiment due to our resource constraints. We split the dataset into two parts where 80% data is used for training and another 20% for validation. Images were randomly chosen for training and validation. Figure 2 shows some sample images from NWPU-RESISC45 dataset.Fig.2. Sample Images from NWPU-RESISC45 Dataset3.2.ResultsWe have applied the proposed models and compared the result with existing methods. Table 2 shows the experimental results.Table 2 shows that the handheld feature extraction method NABP shows 71.76% accuracy, again 72.28% accuracy have been found using CENTRIST and 73.80% using tCENTRIST. All of these three methods was performed using 5 fold cross validation with SVM classifier.Table 2. Experimental Results of Different Methods.In the next phase we applied VGG11, VGG16 and our proposed 7 layer CNN from the scratch. VGG11 and VGG16 shows comparatively less accuracy than our proposed CNN trained from scratch. VGG16 and VGG11 shows 70.52% and 76.9% of accuracy respectively and our proposed 7 layers CNN shows 87.57%. The result improved more than 10% than VGG11. Results of initial two models produce less accuracy. The main reason is the models are large enough and huge number of parameters but we could not feed enough data to these models due to resource constraints. So, the models over fits the data and yields a poor accuracy rate. We have reduced a number of parameters to reduce overfitting in our model.We found our best results using transfer learning. 91.9% accuracy is found when we used VGG16 as fixed feature extractor and trained only the softmax classifier, 90.48% accuracy found by fine-tuning the last three layers of VGG16.Fig.3. Experimental Results Comparison for Different Methods on NWPU-RESISC45 DatasetLastly we applied ResNet50 to classify the remote sensing image dataset and found best results. Usingoriginal ResNet50 we found 95.9% and after applying some fine tuning described previously we got 96.91% accuracy for our dataset. Figure 3 shows a comparison of results based on different findings using different procedures.4.ConclusionThe goal of this experiment was to increase accuracy of remote sensing scene images. We have chosen NWPU-RESISC45 dataset. The main dataset contains 31,500 images with 45 classes. Different machine learning procedures are applied on this dataset including deep learning. We applied various types of procedures on the dataset. We proposed a new model which yields the best result (87.57%) for the models learned from scratch. Best result has been found using transfer learning (fine-tuned ResNet50 96.91%). ResNet50 is a large model compared to the proposed 7 layer CNN model. It takes long time to find output from input using ResNet. Proposed CNN takes less than half time compared to ResNet50 to take decision. But, as accuracy is our main concern, transfer learning is the best suited for the dataset we have used. Recently a new large dataset on scene image has been published named by PatternNet [20]. It is a high scaled remote sensing image dataset containing 38 classes. In future we will try to do our research on a better environment on these largest datasets. These will help us to accurately classify remote sensing scene images.References[1]Guo, Zhenhua, Lei Zhang, and David Zhang. "A completed modeling of local binary pattern operatorfor texture classification." IEEE Transactions on Image Processing 19.6 (2010): 1657-1663.[2]Cheng, Gong, Junwei Han, and Xiaoqiang Lu. "Remote sensing image scene classification: benchmarkand state of the art." Proceedings of the IEEE 105.10 (2017): 1865-1883.[3]Simonyan, Karen, and Andrew Zisserman. "Very deep convolutional networks for large-scale imagerecognition." arXiv preprint arXiv:1409.1556 (2014).[4]Xiao, J., Hays, J., Ehinger, K. A., Oliva, A., & Torralba, A. (2010, June). Sun database: Large-scalescene recognition from abbey to zoo. In Computer vision and pattern recognition (CVPR), 2010 IEEE conference on (pp. 3485-3492). IEEE.[5]Lienou, Marie, Henri Maitre, and Mihai Datcu. "Semantic annotation of satellite images using latentDirichlet allocation." IEEE Geoscience and Remote Sensing Letters 7.1 (2010): 28-32.[6]Vatsavai, Ranga Raju, Anil Cheriyadat, and Shaun Gleason. "Unsupervised semantic labelingframework for identification of complex facilities in high-resolution remote sensing images." Data Mining Workshops (ICDMW), 2010 IEEE International Conference on. IEEE, 2010.[7] A. Krizhevsky, I. Sutskever, G. E. Hinton, Imagenet classification with deep convolutional neuralnetworks, in: Advances in Neural Information Processing Systems (NIPS), 2012, pp. 1097-1105.[8]K. He, X. Zhang, S. Ren, J. Sun, Spatial pyramid pooling in deep convolutional networks for visualrecognition, IEEE Transactions on Pattern Analysis and Machine Intelligence 37 (9) (2015) 1904-1916.[9] C. Szegedy, W. Liu, Y. Jia, P. Sermanet, S. Reed, D. Anguelov, D. Erhan, V. Vanhoucke, A.Rabinovich, Going deeper with convolutions, in: IEEE Conference on Computer Vision and Pattern Recognition (CVPR), 2015.[10] F. P. S. Luus, B. P. Salmon, F. van den Bergh, B. T. J. Maharaj, Multiview deep learning for land-useclassification, IEEE Geoscience and Remote Sensing Letters 12 (12) (2015) 2448-2452.[11] B. Zhao, B. Huang, Y. Zhong, Transfer learning with fully pretrained deep convolution networks forland-use classification, IEEE Geoscience and Remote Sensing Letters 14 (9) (2017) 1436-1440.[12] F. Hu, G.-S. Xia, J. Hu, L. Zhang, Transferring deep convolutional neural networks for the sceneclassification of high-resolution remote sensing imagery, Remote Sensing 7 (11) (2015) 14680-14707. [13]Y. Jia, E. Shelhamer, J. Donahue, S. Karayev, J. Long, R. Girshick, S. Guadarrama, T. Darrell, Ca_e:Convolutional architecture for fast feature embedding, in: ACM International Conference on Multimedia, 2014, pp. 675-678.[14]Castelluccio, Marco, et al. "Land use classification in remote sensing images by convolutional neuralnetworks." arXiv preprint arXiv:1508.00092 (2015)[15]K. Nogueira, O. A. Penatti, J. A. dos Santos, Towards better exploiting convolutional neural networksfor remote sensing scene classi_cation, Pattern Recognition 61 (2017) 539 -556.[16]G. Cheng, J. Han, X. Lu, Remote sensing image scene classi_cation: Benchmark and state of the art,Proceedings of the IEEE 105 (10) (2017) 1865-1883.[17]Wang, Limin, et al. "Places205-vggnet models for scene recognition." arXiv preprintarXiv:1508.01667 (2015).[18]Rahman, Md Mostafijur, et al. "Noise adaptive binary pattern for face image analysis." Computer andInformation Technology (ICCIT), 2015 18th International Conference On. IEEE, 2015.[19]Wu, J.; Rehg, J.M. CENTRIST: A visual descriptor for scene categorization. IEEE Trans. Pattern Anal.Mach. Intell. 2011, 33, 1489–1501[20]Zhou, W., Newsam, S., Li, C., & Shao, Z. (2017). Patternnet: a benchmark dataset for performanceevaluation of remote sensing image retrieval. arXiv preprint arXiv:1706.03424.[21]Dey, E. K., Tawhid, M. N. A., & Shoyaib, M. (2015). An automated system for garment texture designclass identification. Computers, 4(3), 265-282.[22]Islam, S. S., Rahman, S., Rahman, M. M., Dey, E. K., & Shoyaib, M. (2016, May). Application of deeplearning to computer vision: A comprehensive study. In Informatics, Electronics and Vision (ICIEV), 2016 5th International Conference on (pp. 592-597). IEEE.[23]Islam, S. S., Dey, E. K., Tawhid, M. N. A., & Hossain, B. M. (2017). A CNN Based Approach forGarments Texture Design Classification. Advances in Technology Innovation, 2(4), 119-125.[24]Rahman, M. M., Rahman, S., Dey, E. K., & Shoyaib, M. (2015). A gender recognition approach with anembedded preprocessing. International Journal of Information Technology and Computer Science (IJITCS), 7(7), 19.Authors’ ProfilesMd. Arafat Hussain Completed Bachelor of Science in Software Engineering from Institute ofInformation Technology (IIT), University of Dhaka, Bangladesh in 2017. His area of interest inresearch is machine learning, image processing and pattern recognition.Emon Kumar Dey is currently an assistant professor in Institute of Information Technology(IIT), University of Dhaka. He received his M.S degree from the Department of ComputerScience and Engineering, University of Dhaka, Bangladesh in 2011. His research area includepattern recognition, machine learning, image processing, LiDAR data processing, 3D buildingmodelling etc.How to cite this paper: Md. Arafat Hussain, Emon Kumar Dey,"Remote Sensing Image Scene Classification", International Journal of Engineering and Manufacturing(IJEM), Vol.8, No.4, pp.13-20, 2018.DOI: 10.5815/ijem.2018.04.02。