Data Cache Energy Minimizations Through Programmable Tag Size Matching to the Applications
一种有效的异构盘高能效缓存机制

c mb n S a d HDD y u i g S D sa c c e f rHDD, ih ce ts mo e i l me frHDD h td wn f r o ie S D n b sn S a a h o wh c r ae r d et i o t su- o o o
在过 去 的 5 O多年里 ,由于硬盘 技术 的逐 渐成熟 ,
机 械结构 ,所 以突发响应 时间非常短 。这种多 片的体 系 结构还可 以发挥并行存 取的优势 , 获得极高 的数据
传 输速率 。
硬 盘 因其 容量大 、 性价 比高等众 多优 势成 为从个人计 算 机 到 企业 级存 储系 统 等计 算 机系 统 的主要 存储 设
p we svn . t h a' t , ep t ot ad cyefre e t e l e n lo tm ( E a oi m rso ) o r a ig A esne i w u fr ea-nocm n r a met g rh D l rh f hr - t l me h p c a i g t o t
21 0 1年 第 2 卷 第 1 O 1期
o n
计 算 机 系 统 应 用
一
种有效的异构盘高能效缓存机制①
窦少彬 ,杨 良怀 ,龚卫华
( 浙江 工业大学 计算机科 学与技术 学院 ,杭州 3 0 2 ) 10 3
摘 要 :固态盘具 有低功耗 、高 性能、耐冲击等优势 ,硬盘 具有高容量 、低 价格 等优势。通过改进文件系统 的
能和 降低 能耗。 关键词 :磁盘节 能;固态盘;缓存策略;存储系统;能效
Efe tv e g ・ f c e fe i c e ef rHe e o- i e f c i eEn r y- i i ntBuf r ng S h m o t r ・ v Ef Dr
提高缓存性能的方法及缓存系统[发明专利]
![提高缓存性能的方法及缓存系统[发明专利]](https://img.taocdn.com/s3/m/a23d63840b1c59eef8c7b4fd.png)
专利名称:提高缓存性能的方法及缓存系统专利类型:发明专利
发明人:李至哲
申请号:CN200810056990.5
申请日:20080128
公开号:CN101221539A
公开日:
20080716
专利内容由知识产权出版社提供
摘要:本发明提供了一种提高缓存性能的方法,该方法包括:以扇区为基本单位处理访问缓存Cache的输入输出IO请求,其中,所述扇区大小要小于缓存时隙Cache slot大小。
相应地,本发明还提供了一种缓存系统。
利用本发明提供的技术方案,能够减少IO请求响应时间,加快响应速度,提高缓存性能。
申请人:杭州华三通信技术有限公司
地址:310053 浙江省杭州市高新技术产业开发区之江科技工业园六和路310号华为杭州生产基地国籍:CN
代理机构:北京德琦知识产权代理有限公司
更多信息请下载全文后查看。
基于超窄数据的低功耗数据Cache方案
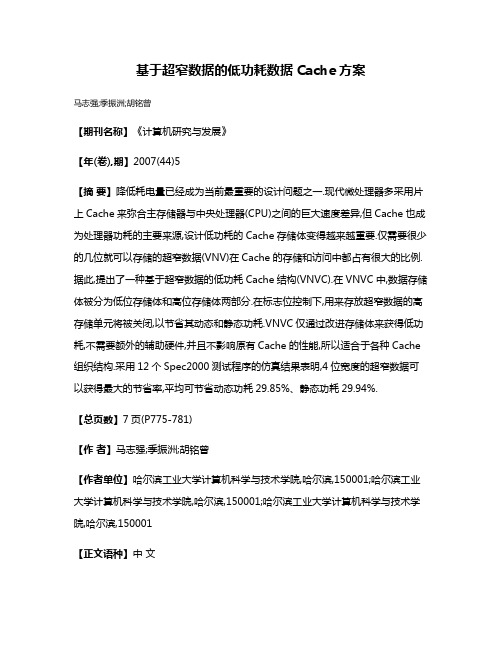
基于超窄数据的低功耗数据Cache方案马志强;季振洲;胡铭曾【期刊名称】《计算机研究与发展》【年(卷),期】2007(44)5【摘要】降低耗电量已经成为当前最重要的设计问题之一.现代微处理器多采用片上Cache来弥合主存储器与中央处理器(CPU)之间的巨大速度差异,但Cache也成为处理器功耗的主要来源,设计低功耗的Cache存储体变得越来越重要.仅需要很少的几位就可以存储的超窄数据(VNV)在Cache的存储和访问中都占有很大的比例.据此,提出了一种基于超窄数据的低功耗Cache结构(VNVC).在VNVC中,数据存储体被分为低位存储体和高位存储体两部分.在标志位控制下,用来存放超窄数据的高存储单元将被关闭,以节省其动态和静态功耗.VNVC仅通过改进存储体来获得低功耗,不需要额外的辅助硬件,并且不影响原有Cache的性能,所以适合于各种Cache 组织结构.采用12个Spec2000测试程序的仿真结果表明,4位宽度的超窄数据可以获得最大的节省率,平均可节省动态功耗29.85%、静态功耗29.94%.【总页数】7页(P775-781)【作者】马志强;季振洲;胡铭曾【作者单位】哈尔滨工业大学计算机科学与技术学院,哈尔滨,150001;哈尔滨工业大学计算机科学与技术学院,哈尔滨,150001;哈尔滨工业大学计算机科学与技术学院,哈尔滨,150001【正文语种】中文【中图分类】TP302【相关文献】1.利用基地址相关的低功耗数据cache设计 [J], 张宇弘;王界兵;严晓浪;汪乐宇2.低功耗的可重构数据Cache设计 [J], 肖斌;方亮;柴亦飞;陈章龙;涂时亮3.基于对指令数据区分访问的混合cache低功耗策略 [J], 王亮;张盛兵;谭永亮;潘永峰4.基于亚阈值漏电流的数据Cache低功耗控制策略研究 [J], 赵世凡;樊晓桠;李玉发5.基于Load重用的低功耗数据Cache设计 [J], 李泉泉;薛志远;张铁军;王东辉;侯朝焕因版权原因,仅展示原文概要,查看原文内容请购买。
算力冗余设计-概述说明以及解释
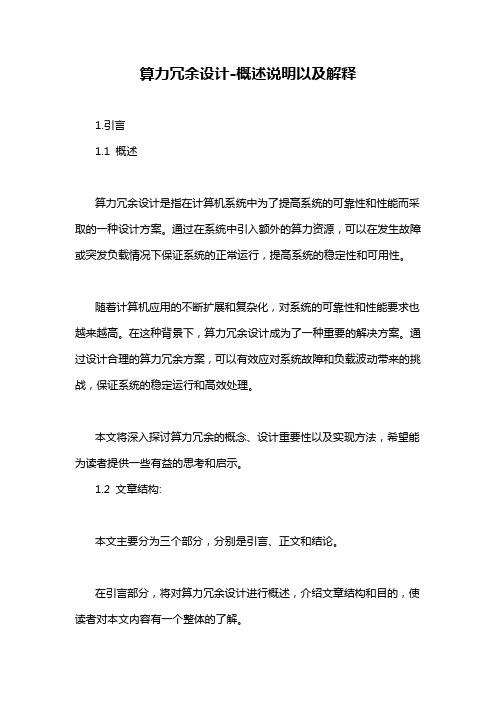
算力冗余设计-概述说明以及解释1.引言1.1 概述算力冗余设计是指在计算机系统中为了提高系统的可靠性和性能而采取的一种设计方案。
通过在系统中引入额外的算力资源,可以在发生故障或突发负载情况下保证系统的正常运行,提高系统的稳定性和可用性。
随着计算机应用的不断扩展和复杂化,对系统的可靠性和性能要求也越来越高。
在这种背景下,算力冗余设计成为了一种重要的解决方案。
通过设计合理的算力冗余方案,可以有效应对系统故障和负载波动带来的挑战,保证系统的稳定运行和高效处理。
本文将深入探讨算力冗余的概念、设计重要性以及实现方法,希望能为读者提供一些有益的思考和启示。
1.2 文章结构:本文主要分为三个部分,分别是引言、正文和结论。
在引言部分,将对算力冗余设计进行概述,介绍文章结构和目的,使读者对本文内容有一个整体的了解。
在正文部分,将深入探讨算力冗余的概念,分析设计算力冗余的重要性,以及介绍算力冗余的实现方法。
通过具体的案例和技术细节,阐述算力冗余设计的必要性和实施方式。
在结论部分,对整篇文章进行总结,概括算力冗余设计的应用前景,展望未来的发展趋势,为读者提供对于算力冗余设计的深入思考和展望。
1.3 目的算力冗余设计的目的在于提高系统的可靠性和稳定性。
通过引入冗余的算力资源,系统可以在某些组件出现故障或性能下降时,仍能保持正常运行。
这样可以有效避免单点故障,提高系统的稳定性和可用性。
另外,算力冗余设计也可以提高系统的性能和处理能力。
通过合理配置冗余算力资源,可以在高负载时自动触发冗余资源,从而提升系统的整体性能和响应速度。
总的来说,算力冗余设计的目的是为了提高系统的可靠性、稳定性和性能,确保系统能够持续正常运行并应对各种临时性故障或挑战。
2.正文2.1 算力冗余的概念算力冗余是指在计算机系统中为了提高系统的可靠性和稳定性而设计的一种策略。
在网络中,算力冗余通常指的是在数据中心或者分布式系统中部署额外的计算资源,以应对计算资源的突发故障或者负载过大的情况。
密集存储技术

密集存储技术密集存储技术(High-Density Storage Technology)是指在较小的物理空间内存储更多的数据的技术。
该技术在数字化时代中起着重要的作用,因为数据量的快速增长需要更高效的数据存储和管理方式。
同时,密集存储技术也有助于节省数据中心和企业的空间和成本。
密集存储技术的主要目标是在较小的物理空间内获得更高的数据存储密度。
为达到这个目标,有多种技术可供选择,其中包括:2.磁带库(Tape Library):磁带库是一种高容量的备份和归档存储设备。
磁带库可以通过多种方式进行备份和归档操作,实现快速数据检索和恢复。
3.固态硬盘(SSD):SSD是一种数据存储设备,与传统的机械硬盘相比,它使用闪存来存储数据,速度更快,可靠性更高,结构更简单,能耗更低。
目前,SSD已经成为一种流行的内部数据存储设备,广泛应用于笔记本电脑、平板电脑、服务器等领域。
4.云存储(Cloud Storage):云存储是一种可以通过互联网访问的数据存储方式。
它可以提供高可用性、高性能和高安全性,成本也相对较低。
使用云存储,企业可以在不增加设备投资的情况下,获取更高的数据存储密度。
除了这些技术之外,还有一些其他的密集存储技术,如混合存储(Hybrid Storage)、闪存阵列(All-flash Array)等。
这些技术的共同特点是具有高性能、高可靠性和高可用性,能够极大提高数字数据存储和管理的效率。
然而,密集存储技术也存在一些挑战和限制。
其中一个最明显的挑战就是随着数据量的不断增长,数据中心需要更多的存储容量,这将给企业带来更高的成本压力。
此外,数据安全性也是一个重要的考虑因素。
密集存储设备需要支持多种不同的备份和恢复策略,从而确保数据安全。
在未来,随着数字化时代的不断深入和数据量的不断爆发,密集存储技术将继续发挥重要的作用。
银行、保险、电商等行业的数据中心也将不断运用这些技术,以应对不断增长的数字数据。
电信5G协优考试题库(含答案)
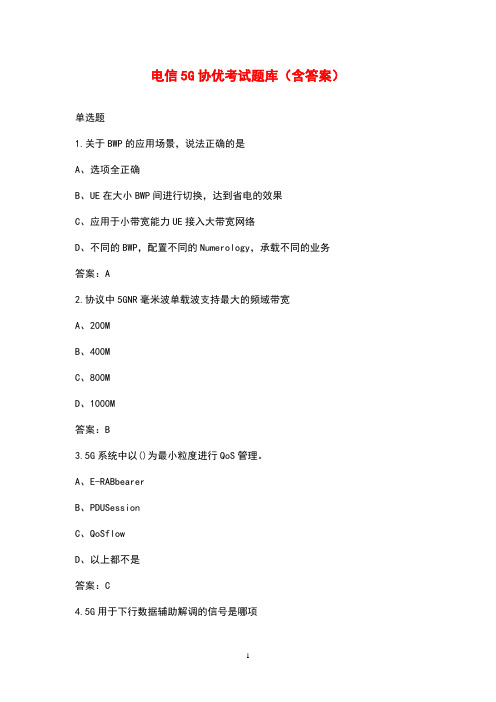
电信5G协优考试题库(含答案)单选题1.关于BWP的应用场景,说法正确的是A、选项全正确B、UE在大小BWP间进行切换,达到省电的效果C、应用于小带宽能力UE接入大带宽网络D、不同的BWP,配置不同的Numerology,承载不同的业务答案:A2.协议中5GNR毫米波单载波支持最大的频域带宽A、200MB、400MC、800MD、1000M答案:B3.5G系统中以()为最小粒度进行QoS管理。
A、E-RABbearerB、PDUSessionC、QoSflowD、以上都不是答案:C4.5G用于下行数据辅助解调的信号是哪项A、DMRSB、PT-RSC、ssD、CSI-RS答案:A5.56单站验证时,传输带宽的要求是?A、500MB、800MC、900MD、2G答案:B6.以下5GNRslotformat的说法对的有A、SCS=60KHz时,支持配置Periodic=0.625msB、Cell-specific的单周期配置中,单个配置周期内只支持一个转换点C、对DL/UL分配的修改以slot为单位答案:B7.在5G中,PUSCH支持的波形有A、DFT-S-OFDMB、DFT-a-OFDMC、DFT-OFDMD、S-OFDM答案:A8.电信选择的帧结构为()A、2ms单周期B、2.5ms单周期C、2.5ms双周期D、5ms单周期答案:C9.以下哪个参数用于指示对于SpCell,是否上报PHRtype2A、phr-Type2SpCellB、phr-Type2OtherCellC、phr-ModeOtherCGD、dualConnectivityPHR答案:A10.5G支持的新业务类型不包括A、eMBBB、URLLCC、eMTCD、mMTC答案:C11.你预计中国的5G将会在什么时候规模商用A、2018到2019.B、2020到2022C、2023到2025D、2025到2030答案:B12.一般情况下,NR基站的RSRP信号低于多少时,用户观看1080P视频开始出现缓冲和卡顿?A、-112dBmB、-107dBmC、-102dBmD、-117dBm答案:D13.IT服务台是一种:A、流程B、设备C、职能D、职称答案:C14.SN添加的事件为A、A2B、A3C、B1D、B2答案:C15.以下SSB的测量中,那些测量标识中只可以在连接态得到:A、SS-RSRPB、SS-RSRQC、SS-SINRD、SINR答案:C16.哪个docker镜像用于配置数据生效及查询?A、oambsB、nfoamC、brsD、ccm答案:B17.5G中,sub-6GHz频段能支持的最大带宽是A、60MHzB、80MHzC、100MHzD、200MHz答案:C18.eLTEeNB和gNB之间的接口称为()接口A、X1B、X2C、XnD、Xx答案:C19.ShortTTI子载波间隔为A、110KHzB、120KHzC、130KHzD、140KHz答案:B20.NR核心网中用于会话管理的模块是A、AMFB、SMFC、UDMD、PCF答案:B21.中移选择的帧结构为()A、2ms单周期B、2.5ms单周期C、2.5ms双周期D、5ms单周期答案:D22.ZXRAN室外宏站楼顶安装天线抱杆直径要求需满足?A、60mm~120mmB、40mm~60mmC、20mm~40mmD、10mm~20mm答案:A23.关于自包含帧说法错误的:A、同一子帧内包含DL、UL和GPB、同一子帧内包含对DL数据和相应的HARQ反馈C、采用自包含帧可以降低对发射机和接收机的硬件要求D、同一子帧内传输UL的调度信息和对应的数据信息答案:C24.属于LPWAN技术的是A、LTEB、EVDOC、CDMAD、NB-IOT答案:D25.5G天线下倾角调整的优先级是以下哪项?A、调整机械下倾>调整可调电下倾一>预置电下傾B、预置电下倾つ调整机械下傾->调整可调电下倾C、调整可调电下倾一>预置电下倾→调整机械下傾D、预置电下倾一>调整可调电下倾调整机械下倾答案:D26.5G的无线接入技术特性将(5GRATfeatures)会分阶段进行,即phase1和p hase2.请问5G的phase2是哪个版本?A、R13B、R14C、R15D、R16答案:D27.5GNR网管服务器时钟同步失败的可能原因有?A、网络连接不正常B、主备板数据库不一致C、EMS小区数目超限D、SBCX备板不在位答案:A28.以下对5GNR切换优化问题分析不正确的是?A、是否漏配邻区B、测试点覆盖是否合理C、小区上行是否存在干扰D、后台查询是否有用户答案:D29.5G系统中,1个CCE包含了多少个REG?A、2B、6C、4D、8答案:B30.NR网络中,PRACH信道不同的序列格式对应不同的小区半径,小区半径最大支持多少KMA、110B、89C、78D、100答案:D31.协议已经定义5G基站可支持CU和DU分离部署架构,在()之间分离A、RRC和PDCPB、PDCP和RLCC、RLC和MACD、MAC和PHY答案:B32.AAU倾角调整优先级正确描述为以下哪项?A、调整可调电下倾->调整机械下倾->数字下倾->设计合理的预置电下倾B、设计合理的预置电下倾->调整可调电下倾->调整机械下倾->数字下倾C、调整可调电下倾->调整机械下倾->设计合理的预置电下倾->数字下倾D、调整可调电下倾->设计合理的预置电下倾->调整机械下倾->数字下倾答案:B33.5G的基站和4G的基站的主要差异在A、RRUB、BBUC、CPRID、接口答案:B34.UE最多监听多少个不同的DCIFormatSizePerSlotA、2B、3C、4D、5答案:C35.仅支持FR1的UE在连接态下完成配置了TRS,TRS与SSB可能存在下面哪个QCL关系A、QCL-TypeAB、QCL-TypeBC、QCL-TypeCD、QCL-TypeD答案:C36.5G网络中,回传承载的是和之间的流量A、DU、CUB、AAU、DUC、CU、核心网D、AAU、CU答案:C37.不属于5G网络的信道或信号是()A、PDSCHB、PUSCHC、PDCCHD、PCFICH答案:D38.5G的愿景是A、一切皆有可能B、高速率,高可靠C、万物互联D、信息随心至,万物触手及答案:D39.关于MeasurementGap描述错误的是A、EN-DC下,网络可以配置Per-UEmeasurementgap,也可以配置Per-FRmeasur ementgap;B、EN-DC下,LTE服务小区和NR服务小区(FR1)的同属于perFR1measurementg ap;C、EN-DC下,gap4~gap11可以用于支持Per-FR1measurementgap的UE;D、EN-DC下,支持per-UEmeasurementgap的UE,若同时用于NR和非NR邻区测量,可以用gap0~11。
memc方法论-概述说明以及解释
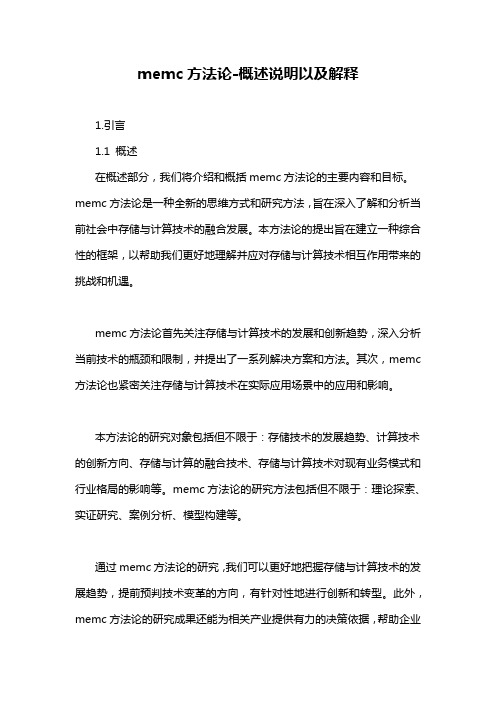
memc方法论-概述说明以及解释1.引言1.1 概述在概述部分,我们将介绍和概括memc方法论的主要内容和目标。
memc方法论是一种全新的思维方式和研究方法,旨在深入了解和分析当前社会中存储与计算技术的融合发展。
本方法论的提出旨在建立一种综合性的框架,以帮助我们更好地理解并应对存储与计算技术相互作用带来的挑战和机遇。
memc方法论首先关注存储与计算技术的发展和创新趋势,深入分析当前技术的瓶颈和限制,并提出了一系列解决方案和方法。
其次,memc 方法论也紧密关注存储与计算技术在实际应用场景中的应用和影响。
本方法论的研究对象包括但不限于:存储技术的发展趋势、计算技术的创新方向、存储与计算的融合技术、存储与计算技术对现有业务模式和行业格局的影响等。
memc方法论的研究方法包括但不限于:理论探索、实证研究、案例分析、模型构建等。
通过memc方法论的研究,我们可以更好地把握存储与计算技术的发展趋势,提前预判技术变革的方向,有针对性地进行创新和转型。
此外,memc方法论的研究成果还能为相关产业提供有力的决策依据,帮助企业和组织在竞争激烈的市场中找到与时俱进的发展策略。
综上所述,memc方法论是一个全面系统地研究存储与计算技术发展和融合的方法体系,它将带来深刻的理论和实践意义。
在接下来的文章中,我们将详细介绍memc方法论的各个要点,并展望其在未来的研究和应用中的潜力和前景。
1.2文章结构文章结构是指文章的整体组织框架和内容划分方式。
一个良好的文章结构能够使读者更容易理解文章的逻辑顺序和思路发展,同时也能提高文章的可读性和表达效果。
在本文中,我们将按照以下结构来组织文章内容:2. 正文2.1 第一个要点在这个部分,我们将详细介绍memc方法论的第一个要点。
我们将分析其原理、核心思想以及相关的应用实例。
通过这些具体的实例和案例,读者可以更加深入地理解memc方法论的实际运用和优势所在。
2.2 第二个要点在这个部分,我们将探讨memc方法论的第二个要点。
内存技术的能耗与功耗优化方法(二)
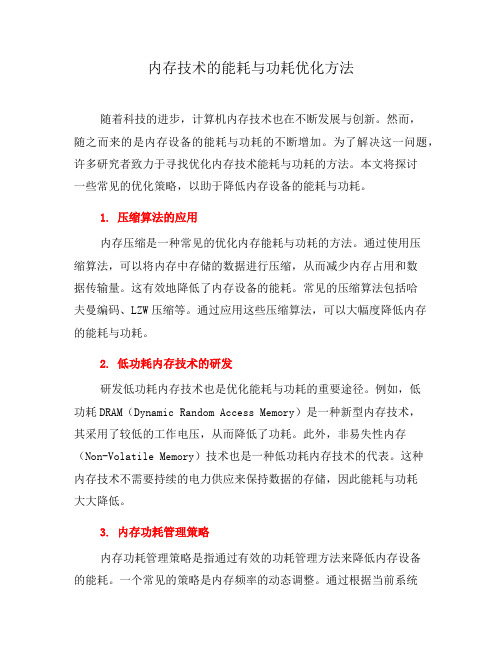
内存技术的能耗与功耗优化方法随着科技的进步,计算机内存技术也在不断发展与创新。
然而,随之而来的是内存设备的能耗与功耗的不断增加。
为了解决这一问题,许多研究者致力于寻找优化内存技术能耗与功耗的方法。
本文将探讨一些常见的优化策略,以助于降低内存设备的能耗与功耗。
1. 压缩算法的应用内存压缩是一种常见的优化内存能耗与功耗的方法。
通过使用压缩算法,可以将内存中存储的数据进行压缩,从而减少内存占用和数据传输量。
这有效地降低了内存设备的能耗。
常见的压缩算法包括哈夫曼编码、LZW压缩等。
通过应用这些压缩算法,可以大幅度降低内存的能耗与功耗。
2. 低功耗内存技术的研发研发低功耗内存技术也是优化能耗与功耗的重要途径。
例如,低功耗DRAM(Dynamic Random Access Memory)是一种新型内存技术,其采用了较低的工作电压,从而降低了功耗。
此外,非易失性内存(Non-Volatile Memory)技术也是一种低功耗内存技术的代表。
这种内存技术不需要持续的电力供应来保持数据的存储,因此能耗与功耗大大降低。
3. 内存功耗管理策略内存功耗管理策略是指通过有效的功耗管理方法来降低内存设备的能耗。
一个常见的策略是内存频率的动态调整。
通过根据当前系统的工作负载动态调整内存频率,可以在不降低性能的情况下降低能耗与功耗。
此外,内存的休眠与唤醒策略也是一种有效的内存功耗管理方法。
将未使用的内存单元置于休眠状态,可以减少功耗。
4. 具备能效优化的硬件设计通过在硬件设计阶段着重考虑能效问题,可以在源头上降低内存设备的能耗与功耗。
例如,采用先进的电源管理系统设计,以降低内存设备的待机功耗。
此外,采用低功耗的集成电路设计、优化内存控制器等也是有效的硬件设计策略。
总结起来,优化内存技术的能耗与功耗是一个复杂的问题,需要从多个角度综合考虑。
压缩算法、低功耗内存技术、内存功耗管理策略和能效优化的硬件设计等都是有效的方法。
通过结合各种策略,可以实现内存设备能耗与功耗的降低,进而提高计算机系统的效能和节能水平。
- 1、下载文档前请自行甄别文档内容的完整性,平台不提供额外的编辑、内容补充、找答案等附加服务。
- 2、"仅部分预览"的文档,不可在线预览部分如存在完整性等问题,可反馈申请退款(可完整预览的文档不适用该条件!)。
- 3、如文档侵犯您的权益,请联系客服反馈,我们会尽快为您处理(人工客服工作时间:9:00-18:30)。
Data Cache Energy Minimizations Through Programmable Tag Size Matching to the ApplicationsPeter Petrov and Alex OrailogluComputer Science&Engineering DepartmentUniversity of California,San Diego(ppetrov,alex)@ABSTRACTAn application-specific customization methodology for minimizing the energy dissipation in the data cache of embedded processors is pre-sented in this paper.The data cache subsystem is one of the most power consuming microarchitectural parts of embedded processors. We target in this work particularly the data cache tag operations and show how an exceedingly small number of tag bits,if any,are needed to compute the miss/hit behavior for the vast majority of load/store instructions executed within application loops.The energy needed to perform the tag reads and comparisons can be thus dramatically reduced.We follow up this conceptual enhancement with a presen-tation of an efficient,reprogrammable implementation that utilizes application-specific information to apply the suggested energy min-imization approach.The conducted experimental results confirm the expected significant decrease of energy dissipation for a set of impor-tant numerical kernels.1.INTRODUCTIONAn ever increasing and significant portion of the consumer elec-tronic market nowadays is dominated by embedded systems.A large part of the functionality of such systems is typically implemented on a set of embedded processors.Major benefits of using embedded pro-cessors include improved time-to-market,flexible system implemen-tation,and low-cost system design.The embedded processor cores impose though in turn significant penalties in terms of performance and power,mainly due to their generality.With the advent of the mobile electronic system,such as cellphones, PDAs,and laptop computers,power consumption minimization is be-coming one of the major quality requirements,since less power con-sumption of the product translates to longer battery life.Consequently, overall product quality is highly dependent on techniques for minimiz-ing system power consumption.These techniques can be applied on various design abstraction levels,from circuit level to system archi-tecture.This work is supported by an IBM Graduate Fellowship and NSF Grant0082325.Permission to make digital or hard copies of all or part of this work for personal or classroom use is granted without fee provided that copies are not made or distributed for profit or commercial advantage and that copies bear this notice and the full citation on thefirst page.To copy otherwise,to republish,to post on servers or to redistribute to lists,requires prior specific permission and/or a fee.ISSS’01,October1-3,2001,Montr´e al,Qu´e bec,Canada.Copyright2001ACM1-58113-418-5/01/0010...$5.00.Circuit-level power minimization techniques have been the dom-inant approach in designing energy efficient designs so far[1,2]. However,in recent years,architecture-level approaches have attained popularity due to their ability to eliminate redundancies on a higher, microarchitectural level,thus resulting in even larger power optimiza-tions[3,4].In[5],a small and energy efficient L0data cache has been introduced in order to reduce the power consumption of the memory hierarchy.The price paid is an increased miss rate and longer ac-cess time.A power optimization technique applied during behavioral synthesis for memory intensive applications has been presented in[6]. The behavior of the memory access patterns is utilized to minimize the number of transitions on the address bus and decoder,thus reducing power consumption.In[4]an L0instruction cache has been proposed with run-time techniques for accommodating only the frequently exe-cuted basic blocks.The small size of this cache translates directly to power consumption reductions.The speculative execution in modern high-end processors results in high instruction execution overhead.In [3]a technique for speculation control and pipeline gating has been presented for energy reduction in speculative processors.A new en-ergy estimation framework for microprocessors has been proposed re-cently in[7].The simulation environment employs a transition based power model and quickly achieves very precise power estimations. In this paper,we propose a technique for application-specific cus-tomization of the data cache(D-cache)subsystem of embedded pro-cessor cores,one of the most power devouring components of the pro-cessor architecture.The proposed technique is particularly suitable for applications that contain data-intensive,numerical loops,a trait shared by a variety of DSP applications.We describe an architecture,capable of utilizing application-specific information in a microarchitecturally reprogrammable way.The technique enables re-customization in a post-manufacturing fashion,thus effectively covering a large class of real-life applications with no need for spinning new silicon. Application-specific customization of embedded processor archi-tectures is a technique that transfers application information to the pro-cessor architecture[8].In this case,the microarchitecture performs in-formed decisions as to how to handle various architecture-specific ac-tions.Fundamentally,this approach extends the communication link between compiler and processor architecture by transferring applica-tion information directly to the microarchitecture,while keeping the traditional compiler techniques unaffected.The static analysis infor-mation transfer is accomplished by utilizing a reprogrammable hard-ware implementation.The architecture we propose allows application changes to be applied infield by loading the new application informa-tion,in a manner similar to program reloading.The proposed methodology utilizes information about the place-ment of the data being accessed within the application loops and more specifically the possible D-Cache conflicts and the minimal numberFigure1:DM cache organizationof tag bits needed to identify these conflicts.The tag operations as-sociated to the D-cache are designed for a worst case scenario and they utilize the entire effective address.These operations are very ex-pensive in terms of power and usually carry a large amount of redun-dancy since conflicting references are frequently close to each other in the address space and a large part of their tags is identical.We show how the minimal necessary number of tag bits for identifying cache conflicts can be inferred from the data layout.In the extreme case of an application loop with a dataset thatfits squarely in the D-cache, there would be no cache conflicts;hence no tag operations would be required at all.Only the cold misses when entering the loop require special attention.To handle the cold misses,the valid bits associ-ated to the cache lines could be used.An efficient way of invalidat-ing the cache lines corresponding to the loop prior to its execution is proposed,leading to a power efficient solution for handling the cold misses and enabling the application of the tag minimization frame-work that we propose.We complement our methodology with a discussion of an efficient hardware implementation for the proposed D-cache customization. Not only is the hardware solution efficient,but is also reprogrammable, thus providing theflexibility to re-customize the embedded processor core in a post-manufacturing fashion.Consequently,the described hardware implementation constitutes a unified microarchitectural so-lution,capable of handling a large set of important applications through in-field re-customization,thus maintaining the market benefits of high-volume productions.2.MOTIV ATIONThe D-cache is used to move the data closer to the processor core, so that the time needed to load data from memory is minimized and the performance gap between processor core and memory alleviated.A typical direct-mapped cache organization is shown in Figure1.In this paper we consider only direct-mapped caches,but the approach can be easily extended to set-associative organizations.The referenced effective address is separated into block index,cache index,and tag. The block index is used to address a word within a cache line,while the cache index is used to address the cache line;the tagfield checks whether there is a conflict with a memory location with the same cache index.The tagfield of each cache line is stored in a separate tag memory array.Each time an access is performed to the D-cache,the tag associated to the cache line is read and compared to the tag of the effective address being referred.However,if the referred locations are close in the address space,a large part of these tagfields is identical. Consequently,a large amount of power is spent in reading,comparing and writing unnecessarily large tags.In the domain of computationally intensive applications,such as DSP processing,application loops work on a set of data arrays.Per-formance considerations force manual optimization of these loops fre-quently.The loops rarely have any additional memory references, such as spill andfill code.The only data being addressed in the mem-ory space is the actual data on which the loops operate.Figure2shows for(i1=0;i1<64;i1++)for(i2=0;i2<64;i2++)for(i3=0;i3<64;i3++)C[i1][i3]+=A[i1][i2]*B[i2][i3];Figure2:Matrix multiplication codea matrix multiplication loop.The loop operates on three matrices and the only accesses to data memory consist of the array references. Figures3a and3b depict the memory layout of the three matrices ,,and from the example.The data memory space is dividedinto regions that correspond to one D-cache size and for each of these memory regions the tag part of the address is a constant.We denote these memory regions as0-tag regions.The left part of Figure3shows a configuration in which the data set of the example resides within a single-tag region.It is evident that in such a case there will be no conflicts within the arrays of data in the D-cache and there is no need to perform any tag operations.This is a straightforward consequence of the fact that a tag region has size exactly equal to the D-cache size and the tag part of the address is a constant.Figure3a depicts a con-figuration in which the dataset spans two tag regions.In this case there will be conflicts in the D-cache;nonetheless,the tagfields of two con-flicting addresses will differ on average by an exceedingly small num-ber of bits.If the least significant bit of the tag for a given tag region is0,then the address tag associated with the subsequent tag region in the memory will differ only in the least significant bit,which will be1. In this way the tag regions in neighboring pairs for which the address tag differs only by one bit,the least significant bit,can be grouped. Such pairs of0-tag regions are denoted as1-tag regions.The entire memory space is covered by a set of disjoint1-tag regions.A straightforward inference that can be drawn from the above ob-servations is that there typically exists a large amount of redundancy in reading the entire tag from the tag array and comparing it to the effective address tag,given the proximity of the dataset references;a large number of identical bits in the tag components is to be expected, consequently.Given that application-specific information about the application loop dataset layout in the data memory is present during program execution,a large part of the tag redundancy can be elimi-nated,resulting in significant energy savings.The application infor-mation can be obtained during compile time and provided to the D-Cache microarchitecture in a reprogrammable way.Furthermore,cer-tain compile-time optimization techniques can be utilized,to ensure that the actual loop dataset layout minimizes the number of required tag bits for identifying D-cache conflicts.For example,if possible,the compiler could try to place the data within a-tag region.If the data does notfit,the compiler can try incrementaly larger tag regions until the required minimal number of tag bits is identified.Subsequent sections in the paper present our methodology for elim-inating the aforementioned tag redundancy in anapplication-specificFigure3:Memory layoutmanner,thus achieving significant energy reductions.Since the flex-ibility of straightforward incorporation of application code changes is one of the paramount advantages of utilizing embedded processor cores,an efficient reprogrammable implementation that defines a uni-fied architecture capable of capturing application information in-field is presented.This architecture preserves the generality of the em-bedded processor core in terms of functional programmability,while eliminating the inherent tag redundancy in the cache subsystem.3.TAG UTILIZATION ANALYSISAs demonstrated in the previous section,if the dataset of the fre-quently executed application fragments resides within a -tag region in the memory space,then no D-cache conflicts are possible;hence no tag operations are needed.When the loop dataset spans more than one tag region,a few tag bits are needed depending on the number and size of the tag regions the data spans.The structure and the position of the tag regions are determinative for extracting the redundancy in the tag fields.We explain subsequently the formation and structure of the tag regions within the main memory space.3.1Tag region formationTo understand the structure of the tag regions,let’s consider a D-cache organization and memory address space that requires three bits of tag.This memory space can be divided into eight 0-tag regions with the tag field of the effective address a constant.A generalization of the 0-tag regions can be effected by noticing that all 0-tag regions can be grouped in pairs for which the tag field differs only in the least significant bit;for the first 0-tag region it contains the value of 0,while for the second one,the value is 1.We denote the region formed by the pair of such 0-tag regions as a 1-tag region.If a dataset resides within a 1-tag region,then only the least significant bit from the tag field needs to be used for conflict identification in the D-cache.In a similar vein,the 1-tag regions can be grouped into 2-tag regions.Generally,a-tag region is formed by a pair of -tag regions that differ in no more than the least significant bits of the tag field.All the tagregions are nested within each other;for example a-tag region contains two -tag regions and each of the -tag regions contains two-tag regions in turn.The set of all -tag regions,for any valueof ,covers the entire memory space.A -tag region corresponds to a portion of the memory space with size equal to the size of D-caches and tags differing only in the least significant bits.The -tag region that covers the complete memory corresponds to a region that requires all the tag bits for detecting conflicts.The general-purpose caches operates under the extreme and general case assumption that all the application datasets reside in the -tag memory region,which corresponds to the entire memory space.Their inability to incorpo-rate application knowledge regarding the refinement of the tag regions within which the application data resides is the fundamental reason for the significant amount of tag operation redundancy.Based on the above observations,it is evident that if the D-cache architecture incorporates application knowledge of the loop dataset layout,the energy expensive tag reads can be optimized to read only the minimum required number of tag bitlines from the tag SRAM ar-ray.At the same time,the compiler can try to ensure that the loop data are placed in such a way that they span the minimal tag region that corresponds to their size.3.2Dataset with no D-cache conflictsIf the dataset for the particular application loop has size smaller than the D-cache,then it can be placed within a -tag region.This size analysis is performed at compile time and the loop data is placed within a -tag region in the memory.In this case,no conflicts in the D-cache for the loop data are possible and hence no tag operationsneeded.Figure 4:Loop dataset placementDetection solely of the loop data cold cache misses is required for ensuring the correct D-cache operation.Detecting the cold cache misses can be achieved by invalidating the cache content in which the data resides;if the data spans the whole cache,then the cache needs to be invalidated completely.As the cache reuse across loops is negligi-ble,if any,no performance penalty is incurred,or at worst in the case of data reuse between different loops,the insigni ficant penalty of the cold misses of the second loop data,constitutes the only performance penalty.Once the cache part that will accommodate the loop data is invali-dated,the cold misses are detected naturally through the usage of the valid bits of the cache lines.Noteworthy is that cold misses can occur not only in the first loop iteration but also in subsequent iterations,due to variantly traversed control paths in loop iterations.Consequently,the valid bit usage is a natural solution for detecting cold misses within the loop dataset.3.3Minimal tag usage for conflicting datasetsIf the loop dataset cannot fit within a -tag region,then there will be D-cache con flicts and some tag bits will be needed for con flict identi-fication.Since we want to minimize the number of tag bits needed for comparison,a compile time data placement algorithm is suggested in order to place the dataset within the boundaries of a -tag region with minimal .An even further re finement of placing the dataset within the tag regions can be de fined by observing that when placing a dataset within the boundaries of a -tag region,for some of the data arrays only tag bits might be enough to check for con flicts within the D-cache.Figure 4depicts an example in which the dataset of the example from the previous section is placed into a 1-tag region and its size does not allow the dataset to fit within a 0-tag region.Since the 1-tag region comprises two 0-tag regions,part of the loop dataset (matrices and )is placed in the first 0-tag region,and another part (the matrix )in the second 0-tag region.As the size of these matrices is identical,one can observe that matrixoverlaps in the D-cache with matrix ,while matrix from the first 0-tag region does not overlap with any data in the second 0-tag region.Consequently,when accessing matrix no tag bits would be needed for con flict checking,while in accessing and ,one tag bit is required for detecting con flicts.This principle can be generalized to larger tag regions.If part of the loop dataset is placed into a -tag region and the rest of it is placed into the next -tag region,then for the components in the first -tag region that do not con flict with the components in the second -tag region,only tag bits would be suf ficient for D-cache con flict identi fication.We can see that there are two different scenarios for the number of tag bits needed for the D-cache operations within a loop.If the entire dataset resides in a single -tag region,with overlap possibilities for all data components,then only tag bits are needed for this loop.If part of the data is placed in the next -tag region as described inthe previous paragraph,then for parts of the data components tag bits would be enough,while for the remainder,tag bits will be required.The proposed methodology for energy reduction in the D-cache subsystem consists of two basic steps.Thefirst step is the compile-time support that was presented in this section.This support includes placing the loop dataset within a tag region,or spanning more than one tag region by overlapping only some of the data arrays.During compile time,the minimal number of tag bits needed for each loop is determined and provided to the D-cache microarchitecture through the means outlined in the next section,wherein we describe the required hardware support.4.IMPLEMENTATIONThe proposed methodology requires a hardware support that would be able to dynamically enable only the minimum required number of bits from the tag array for the program loops.The hardware requires exact information as to how many tag bits are needed for a particular loop or functions within this loop.First we present an efficient hardware for manipulating the tag mem-ory array so that only the required minimal number of tag bits are used per application loop.The tag array in the cache subsystem is typically implemented as an SRAM array,possibly divided into multiple banks. The SRAM data array contains horizontal wordlines for each tag word and a vertical bitline for each bit within the tag word.A read operation from the SRAM array is performed in the follow-ing way.The address decoder selects the wordline to be read from the array.All the bitlines are precharged and if the selected memory cell by the wordline contains logic zero,then the bitlines start to get dis-charged.Since the discharge is a quite slow process,a sense amplifier is utilized at the end of each bitline.If a small drop of the voltage level is detected,a logic zero is registered.The precharge and discharge of bitlines are the most energy consuming operations with SRAM data arrays[9].By eliminating most of the bitline precharge and discharge opera-tions,our approach greatly reduces the energy dissipation in the tag SRAM array.This is achieved by gating the bitlines according to the minimal number of tag bits required to check for D-cache conflicts. Only the needed bitlines,if any,are precharged and discharged,thus effectively eliminating the redundant reads.The sense amplifiers for the disabled bitlines are gated as well.Furthermore,the tag compara-tor cells are gated in order to perform the comparison only on the required tag bits.The number of tag bits for each loop needs to be determined be-fore entering the loop,so that the appropriate number of bitlines is enabled.Since this number isfixed for the loop,it can be stored in a special control register before entering the loop.Each bit in this spe-cial control register directly corresponds to an enable signal of bitline and sense amplifier.The default value of this register specifies that all tag bitlines are enabled.The current value of this register is used to determine the number of bitlines to enable.The only delay imposed by this implementation is the insignificant delay of the gating logic, which roughly corresponds to the delay of a simple and gate.If there are data arrays placed in the next-tag region with partial overlap with data arrays from the previous-tag region,then the ac-cesses to these arrays require only tag bits.An identification mecha-nism is needed to distinguish the load instructions to these arrays from the others.One possible approach is the incorporation of an additional bit to the load instructions that will indicate whether an additional tag bit is needed.While highly cost effective in terms of hardware sup-port,nonetheless,because of the optimized opcode size in most em-bedded processors,this approach might be infeasible.An alternative implementation consists of a small table,namely,a Load Identifica-tion Table(LIT).When a load instruction is encountered,the LIT is indexed by the PC of that load instruction and a bit in it specifies whether an additional tag bit is needed.Note that the size of these ta-bles must be limited to a very small number of entries in order to keep the benefits of optimizing the additional tag bit.A reasonable solution would contain at most8entries,which compared to the bitline saved from the much larger tag array is negligible.The proposed implementation is highly cost effective,while inher-ently reprogrammable.It does not impose a timing constraint to the D-cache organization and can be reprogrammed in a post-manufacturing fashion.The application information is provided by the LIT and by writing values to the special control register forfixing the minimal number of tag bits.This part is performed during compile time,by inserting an instruction for writing the correct value into the regis-ter before entering the loop,and an instruction for writing the default value after exiting the loop.The content of the LIT is loaded at the same time as loading the application code into the program memory. Since there might be multiple program loops,multiple LITs might be needed.This can be achieved in a practical way by implementing the LIT as a bigger table and parts of it treated as separate smaller tables. When switching program loops,a switch between these smaller tables needs to be effected.This can be done easily in software by writing a control information to a register that selects the LIT.Another issue that needs to be addressed is the invalidation of the cache lines before entering a program loop so as to use the valid bits for detecting the cold cache misses for the loop dataset.Prior to en-tering the loop that is targeted for tag optimization,the data cache content needs to be invalidated.This can be effectively achieved by software in terms of setting a control bit just before entering the loop. Setting the value for enabling the tag bitlines,switching the LITs, and invalidating the corresponding cache part are the operations that are performed in software.Noteworthy is that all of them are per-formed outside the loop,thus contributing no additional instructions (and no consequent delay)inside the loop.The lookup in the LIT is performed by hardware only for the load instructions;therefore,again no delay is added in executing the loop or accessing the D-cache. 5.EXPERIMENTAL RESULTSIn our experimental study we evaluate the ability of our method-ology to reduce energy consumption by minimizing the number of tag bits used in the D-cache.The results demonstrate the proposed approach on16K and32K direct-mapped D-caches.Both D-cache configurations contain4instructions per cache line.We utilize four numerical computation applications:Matrix multiplication(mmul)of matrices with size64x64;LU decomposition(lu)[10]on a matrix with size64x64;Extrapolated Jacobi-iterative method(ej)[10]on a64x64 grid;and successive over-relaxation(sor)[11]on a matrix with size 64x64.Figures5and6show execution and power data for the benchmarks on general-purpose16K and32K direct-mapped instruction caches. Thefirst row gives the number of D-cache hits.The second row of the tables corresponds to the number of D-cache misses,while the third row presents the energy dissipated in the D-cache in mJ.In order to generate the statistics for the D-cache behavior,we utilize the Sim-pleScalar[12]simulator.We utilize for the cache configuration power models obtained by using the Cacti tool[13]assuming0.35um pro-cess technology.The total energy dissipation is computed by using the execution statistics from SimpleScalar and the static power model produced by Cacti.The energy for the main memory is based on the data presented in[14]and assumes4.95nJ per access.To evaluate the proposed power optimization technique we have identified the application loops and computed the size of the loop dataset and consequently,the-tag region with minimal,in whichmmul sor ej lu #hits1,003,00646,556563,849126,884 #misses45,5701072615,1517,937 energy 2.310.102 4.210.302mmul sor ej lu #hits1,011,90446,588808,529132,930 #misses36,6721040370,4711,891 energy 3.170.143 4.220.352Figure5:Access and energy(mJ)statistics for16K DM D-cache Figure6:Access and energy(mJ)statistics for32K DM D-cachemmul sor ej lu tag bits2032 energy 2.060.143 4.080.271 reduction0.250.0210.130.031 reduction(%)10.82%20.59% 3.08%10.26%mmul sor ej lu tag bits1021 energy 2.790.117 3.940.352 reduction0.380.0260.280.05 reduction(%)11.99%18.18% 6.64%12.44%Figure7:Energy(mJ)statistics for tag optimized16K D-cache Figure8:Energy(mJ)statistics for tag optimized32K D-cachethe dataset can be placed.The subsequent computation of the D-cache access statistics for all the references within the application loops was performed by applying manual assembly level instrumentation of the applications and effecting the corresponding modifications to the sim-ulator.Finally,using power models for the optimized tag array we compute the energy consumed in the D-cache subsystem after opti-mizing the number of tag bits required for D-cache conflict identifi-cation.By utilizing Cacti,we compute the energy dissipated per tag bitline,sense amp,and comparator cell,which allows computation of the energy dissipation for a tag array with only tag bits enabled. Figures7and8show the results achieved for the benchmarks.Thefirst row in these tables shows the minimal number of tag bits required for D-cache conflict identification.The sor benchmark re-quires no tag bits,since it works on a single array thatfits within both 16K and32K D-caches.The second row of these tables shows the absolute amount of energy dissipation(in mJ)for the tag optimized D-caches.The third row corresponds to the absolute energy reduc-tion compared to general-purpose D-cache configurations from Fig-ures5and6,while the fourth row shows the percentage improvement. One can observe that the improvements vary from3%to above20%. Of course,the relative energy reduction is a function not only of the number of tag bits utilized,but also of the miss rate.When the cache misses are considerable,the relative improvement is diminished,since the energy dissipated in the main memory due to misses overwhelms the energy saved from removing some of the tag bits.In case no tag bits are needed whatsoever,which is the case for sor,then the tag subsystem is completely turned off,including the tag decoder and wordline selection,thus further decreasing the energy dissipation.Together with the insignificant miss rate,this complete absence of tag bits underlies the significant energy improvement for the sor benchmark.6.CONCLUSIONWe have presented an optimization methodology for reducing the energy for the data cache subsystem of embedded processors.The proposed framework consists of compile time support for placing the application loop’s dataset into the memory in such a way that the re-quired number of tag bits for accessing the D-cache are minimized. The approach transfers certain application information to the D-cache microarchitecture and dynamically utilizes it to further eliminate the redundancy in the tag operations.An efficient reprogrammable imple-mentation has been proposed for the presented application-specific, power optimization technique.It preserves the fundamental advan-tage of processor-based implementations offlexibility,design reuse, and high-volume productions.Power consumption is a crucial quality factor in numerous mod-ern applications.Our experimental results demonstrate the strength of the proposed approach on a set of real-life applications and suggest the viability of the power minimization technique for a large range of important applications.7.REFERENCES[1]M.B.Kamble and K.Ghose,“Analytical energy dissipationmodels for low-power caches”,in ISLPED,pp.143–148,Au-gust1997.[2]K.Ghose and M.B.Kamble,“Reducing power in superscalarprocessor caches using subbanking,multiple line buffers and bit-line segmentation”,in ISLPED,pp.70–75,August1999. [3]S.Manne,A.Klauser and D.Grunwald,“Pipeline gating:specu-lation control for energy reduction”,in25th ISCA,pp.132–141, June1998.[4]N.Bellas,I.Hajj and C.Polychronopoulos,“Using dynamiccache management techniques to reduce energy in a high-performance processor”,in ISLPED,pp.64–69,August1999.[5]J.Kin,M.Gupta and W.H.Mangione-Smith,“Thefilter cache:an energy efficient memory structure”,in30th MICRO,pp.184–193,April2001.[6]P.R.Panda and N.D.Dutt,“Low-power memory mappingthrough reducing address bus activity”,IEEE Transactions on VLSI Systems,vol.7,pp.309–320,1999.[7]N.Vijaykrishnan,M.Kandemir,M.J.Irwin,H.S.Kim andW.Ye,“Energy-driven integrated hardware-software optimiza-tions using SimplePower”,in27th ISCA,pp.95–106,June2000.[8]P.Petrov and A.Orailoglu,“Towards effective embedded pro-cessors in codesigns:customizable partitioned caches”,in9th CODES,pp.79–84,April2001.[9]N.Bellas,I.Hajj and C.Polychronopoulos,“A detailed,transistor-level energy model for SRAM-based caches”,in ISCAS,pp.198–201,June1999.[10]S.Nakamura,Applied Numerical Methods with Software,Prentice-Hall,Englewood Cliffs,N.J.,1991.[11]M.E.Wolf and m,“A Data Locality Optimizing Algo-rithm”,in PLDI,pp.30–44,June1991.[12]D.Burger and T.M.Austin,“The SimpleScalar Tool Set,Ver-sion2.0”,Technical Report1342,University of Wisconsin-Madison,CS Department,June1997.[13]G.Reinman and N.Jouppi,“An Integrated Cache Timing andPower Model”,Technical report,Western Research Lab,1999.[14]W-T.Shiue and C.Chakrabarti,“Memory exploration for lowpower,embedded systems”,in36th DAC,pp.140–145,June 1999.。