First Steps to an Audio Ontology-Based Classifier for
Esoteric N-03T网络音频传输系统说明书
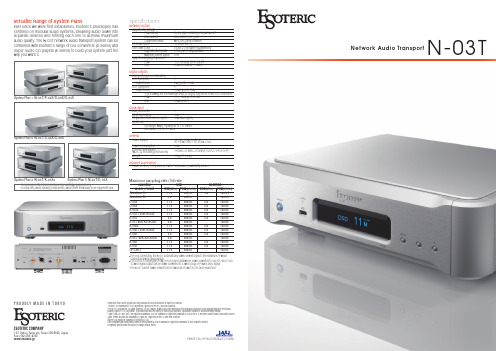
Network Audio Transpor tN-03TVersatile Range of System PlansEver since we were first established, Esoteric’s philosophy has centered on modular audio systems, breaking audio down into separate devices and refining each one to achieve maximum audio quality. The N-03T network audio transport system can be combined with Esoteric’s range of D/A converters (D series) and Super Audio CD players (K series) to build your system just the way you want it.USB XLR/RCAGrandioso K111.2 384/32 2.8 192/24Grandioso D1 5.6 384/32 - 192/24D-02X 11.2 384/32 2.8 192/24D-05X 11.2 384/32 2.8 192/24K-01Xs 11.2 384/32 2.8 192/24K-01X + VUK-K01Xs 11.2384/322.8192/24K-01X 5.6 384/32 2.8 192/24K-01+ V UK-K01XUSB 2.8 384/32 - 192/24K-03Xs 11.2 384/32 2.8 192/24K-03X + VUK-K03Xs 11.2 384/32 2.8 192/24K-03X 5.6 384/32 2.8 192/24K-03 + VUK-K01XUSB 2.8384/32-192/24K-05X 11.2 384/32 2.8 192/24K-07X 11.2 384/32 2.8 192/24OP-DAC111.2 384/32 - 192/24Maximum sampling rate / bit rateConnection Models / FormatDSD (MHz) PCM (kHz/bit) DSD (MHz) PCM (kHz/bit ) System Plan 1 N-03T/P-02X/D-02X/G-02XSystem Plan 2 N-03T/D-02X/G-02XSystem Plan 3 N-03T/K-03Xs System Plan 4 N-03T/D-05X* The arrangements in these photos are for illustrative purposes only.In actual use, avoid stacking components. Mount them individually in an equipment rack.PRINTED IN JAPAN 0218O05•TECD-0266ESOTERIC COMPANY1-47 Ochiai, Tama-shi, Tokyo 206-8530, Japan Fax: (042)356-9240www.esoteric.jpPlease note that Esoteric products are only available at select distributors in respective countries.“Esoteric” is a trademark of TEAC Corporation, registered in the U.S. and other countries.©2018 TEAC Corporation. All Rights Reserved. All text, images, graphics and other materials in this catalogue are subject to the copyright and other intellectual property rights of TEAC Corporation. These materials shall not directly or indirectly be published, reproduced, modified or distributed in any medium.“Super Audio CD” and “DSD” are registered trademarks. IOS is a trademark or registered trademark of Cisco in the U.S. and other countries and is used under license.Apple, iPhone and iPad are trademarks of Apple Inc., registered in the U.S. and other countries. Bulk Pet is a registered trademark of Interface Co.,Ltd.Other company names and product names in this document are the trademarks or registered trademarks of their respective owners.Design and specifications are subject to change without notice.P R O U D L Y M A D E I N T O K Y O•For USB connection, the N-03T automatically down-convert signal to the maximum Fs whichconnected device can playback.•For XLR/RCA connection, PCM 384/352.8kHz data will be down-converted to 192/176.4kHz. DSD 5.6MHz signal output can be down-converted to 2.8MHz (Dop) or PCM88.2kHz signal.*The N-03T cannot down-convert DSD11.2MHz and PCM768/705.6kHz music files.SpecificationsNetwork sectionSupported file formats PCM lossless FLAC , Apple Lossles s (ALAC), WAV, AIFFDSD lossless DSF , DSDIFF (DFF ), DoP Compressed audio MP3, AAC (m4a container ) ETHERNET port1(1000BASE-T)USB DRIVE ports 2 (USB 2.0 or higher recommended) Supported file formats FAT32, NTFS Single partition Maximum current supply 0.5ASupported sampling frequencies PCM 44.1–384kHz, 16/24/32 bitDSD 2.8MHz, 5.6MHz, 11.2MHzDigital outputsUSB port (USB 2.0 standard) 1 X LR connector 1Output level 3Vp-p (into 110Ω) RCA connector 1Output level0.5Vp-p (into 75Ω)*The following are the maximum limits for output from the XLR and RCA connectors. PCM 44.1–192kHz, 16/24 bitDSD2.8MHz (Do P )Clock inputBNC connector 1 Input impedance50ΩFrequencies that can be input 10MHz(±10ppm)Input level Rectangle wave: equivalent to TTL levels Sine wave: 0.5 to 1.0 VrmsGeneralPower supply AC 220-240V, 50/60HzAC 120V, 60Hz / AC 220V, 60Hz Power consumption31WExternal dimensions(W×H×D, including protrusions) 445m m ×131m m ×360mm(17 5/8"×5 1/4"×14 1/4") Weight17kg (37 1/2 lb)Included accessoriesPower cor d ×1, Felt pad s ×3, Owner’s manual ×1, Warranty car d ×1High-Sampling Digital OutputThe N-03T has a USB port enabling digital output up to DSD 11.2MHz and PCM 384kHz/32-bit. This allows the N-03T to be connected to a USB DAC or a disc player with a USB port.Two other digital output systems (XLRx1 and RCAx1) are also included, supporting PCM up to 192kHz/24-bit and DSD2.8MHz (DoP).Music Server FunctionThe N-03T can also be used as a simplified music server in which a player and a librar y can be integrated by connecting large-capacity storage devices to two USB ports on the front and rear of the unit.Compatible with a Wide Range of Streaming Services and Audio CodecsEsoteric has partnerships with a wide range of streaming service and audio codec providers, and preparations are underway for compatibility with new services.**Information on newly supported services will be released on the ESOTERIC website. However, regional restrictions may be implemented on some services.Compatible with a Wide Range of Audio SourcesCare has been taken with every detail to achieve audio quality suitable for high-end network playback. The N-03T is compatible with a vast range of formats (DSF, DSDIFF, FLAC, Apple Lossless, WAV, AIFF, MP3 and AAC) and supports playback up to DSD 11.2MHz and PCM 384kHz/32-bit by USB output. Gapless playback is also suppor ted for all lossless formats for uninterrupted playback of live or opera recordings.Esoteric Sound StreamEsoteric Sound Stream is an Apple iOS network playback app for tablets and smartphones designed with an emphasis on intuitive operability. Simply select music tracks by using your tablet or smartphone to create a customized playlist and then play the playlist. All screens are intuitively designed for easy operation and access to playlists and libraries, making it easy for anyone to use. It also has a wide range of advanced features that meet the demands of even the most experienced users.A key feature is the excellent search and retrieval function that fully utilizes tag information. Images are also stored in the app, enabling you to instantly scroll through album artwork and libraries according to categories such as artist, year of recording, composer or category.Equipped with Two Powerful Independent Power SuppliesThe N-03T is equipped with two large independent toroidal transformers, one for the internal network module and one for the other digital circuits, enabling the ideal supply of power to each circuit block. Unlike a standard switching power supply, these large linear power supplies are made with high qualit y components such as large filter capacitors and Schottky barrier diodes. The dedicated power supply for the network module also has an EDLC (Electric Double-layer Capacitor), a super-capacitor that has 1F (1,000,000μF) capacitance. These provide a dramatic improvement in audio quality.High-Rigidity Chassis ConstructionThe bottom chassis used to secure the circuit components has a dual layer structure with two steel plates (5mm and 2mm). Th e power supply transformer and oth er components are arranged th ree-dimensionally on th e two layers to prevent interference between components, and laser-cut slits are applied to each layer for effective controlling of vibration. And a th ick, h eavyweigh t aluminum panel enclosure and Esoteric’s unique pinpoint feet (patents no. JP4075477 and JP3778108) provide t oroug mechanical grounding against vibration.P R O U D L Y M A D E I N T O K Y ONetwork AudioThe N-03T fulfills every music lover’s dream: to access music freely from your living room chair and enjoy the very best audio quality. The DSD master audio source provides a crystal-clear audio experience, your CD collection is arranged in a library for easy access, and streaming services put new music at your fingertips. The N-03T is a network audio transport system specially designed to connect with an external DAC or a Super Audio CD player’s built-in DAC via USB connection. Other than your audio system, all you need is a home LAN (Wi-Fi router, etc.), a tablet or smartphone and a NAS (music server) to store your music library. That’s all you need for an easy, comfortable musicexperience where you don’t have to compromise on quality.N-03TNetwork Audio TransportMassive, super high quality modular systems are the key philosophy that Esoteric has followed since we were first established.We are now bringing this same philosophy to network playback systems with the N-03T network digital audio transport system.Your favorite D/A converter or Super Audio CD player can be digitally connected by USB, enabling you to build just the right system to enjoy playing files or streaming content your way.Esoteric takes digital transport to a new level with endless options and even more possibilities for audio playback.N-03TTablet / SmartphoneD/A converterSuper Audio CD player,etc.USB / XLR / RCA Digital connection Internet。
EZdrummer 2 使用手册说明书
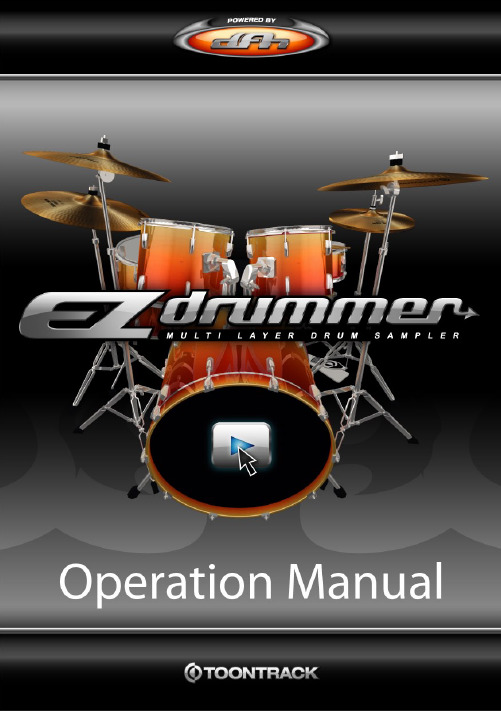
A BOUT THIS MANUALScreenshots included in this manual may differ from the actual product.Macintosh, Mac OS X and Audio Units are registered trademarks of Apple Computer, Inc. Windows is a trademark of Microsoft Corporation. VST is a trademark of Steinberg Media T echnology AG. RTAS is a trademark of Avid Corp. All other trademarks held by their respective owners.This manual is copyright T oontrack Music AB. No duplication, copying and distribution is permitted without written consent from the originator.EZdrummer2TABLE OF CONTENTS1 - INTRODUCTION 61.1 What is EZdrummer? 61.2 Recording Notes 72 - INSTALLATION 82.1 EZdrummer at a glance 82.2 System Requirements 82.3 Installing EZdrummer for Windows 82.4 Installing EZdrummer for Macintosh 92.5 Authorizing EZdrummer on your computer 93 - QUICK START GUIDE 103.1 Loading a Kit 103.2 Browsing the MIDI Library 113.3 Building your Drum T rack 113.4 Mixing the Kit 134 - ADDITIONAL FUNCTIONALITY 144.1 Advanced Routing 144.2 Help Menu 144.3 Adding MIDI Grooves to the Browser 154.4 Key Mapping 154.5 Expanding EZdrummer 16EZdrummer3EZdrummer 4CREDITSToontrack Development Team:Andreas Sundgren (name & concept)Erik Phersson (project management)Mattias Eklund (audio recordings and editing)Henrik Kjellberg (audio recordings)Olof Westman (programming)Rogue Marechal (support & testing)Fredrik Ärletun (graphic artist)Produced and engineered by:Neil Dorfsman, Pat Thrall, Mattias Eklund & Henrik Kjellberg. Played by Nir ZExternal consultants:Keith More (MIDI programming and velocity sweep concept) Philippe Decuyper (general expertise)Fredrik Hägglund - www.diod.nu (flash tutorial)Manual written by:Rogue Marechal & Andreas Sundgren.Proofreading by Chuck Butler.Betatesters (to whom our undying gratitude goes):Kevin Afflack, Marcello Azevedo, T ony Artimisi, Damian Blunt, Chuck Butler, Ray Campbell, John Christensen, Eric Colvin, Philippe Decuyper, Martin Fido, Lewis Gilbert, Chaim Goldman, Scott Griggs, Mark Heath, Svein Hyttebakk, Martin Keller, Joseph King, Mark King, Kenny Lee, Emmanuel Lorant, David Modisette, Motoyoshi Matsumoto, Murray McDowall, Jeffrey Naness, Kirk Pennak, John Rammelt, Robert Rainey, Marcel Ritsema, Chris Ryan, Daniel Shattuck, Fred Schendel, James Thompson.Additional Thanks:All our hard working distributors and supporting families.EZdrummer51 - INTRODUCTION1.1 What is EZdrummer?Somebody once suggested that we make a light version of Superior (Drummer). A great suggestion! Superior was and still is a monster of a box, designed with the mad scientist music producer in mind. When the time came around to actually realize the idea of a smaller drum sampler we decided to take the concept one step further. So, EZdrummer is a Superior LE and at the same time it isn’t. The experience gained from developing Superior Drummer is all there: sounds recorded and produced in partnership with the best in the business, microphone control, humanizing features, and TPC (T oontrack Percussive Compression) keeps RAM and disc space requirements to a minimum.We’ve also decided to take user friendliness above and beyond:In it’s most basic mode of operation, EZdrummer can yield a great drum track in just a few clicks. The microphone levels are all pre-set. Using the built in MIDI features you can create a drum sequence from a choice of thousands MIDI files by simply opening EZdrummer, selecting the file of your choice, and dragging it into your host.The internal mixer allows EZdrummer to work in both stereo and multitrack mode without the user having to step out of one version of the plug-in and into another. It also gives you control of levels between mics and ambience and overhead microphone leakage. Bringing all this to the user is an interface that we think speaks for itself.So who’s EZdrummer for? We think everyone. Combining quintessential features and advanced handling as well as low system requirements, EZdrummer is an entry level product but also ideal for the pros who need to be mobile. With EZdrummer we’ve taken the first step into the next generation of acoustic drum samplers. The journey starts here.Andreas Sundgren, T oontrack development teamEZdrummer6EZdrummer71.2 Recording NotesIn 2005 T oontrack Music was contacted by Pat Thrall with a request to record drums for the Superior Drummer software engine at the New Y ork studio where Pat had his professional home. We all knew Pats work (with Glenn Hughes, Black Crowes, etc) and jumped at the opportunity to work with one of our hero.We became even more excited when Pat enrolled Neil Dorfsman, another long-time hero of ours, to add his talents, passion, and experience to the recordings. Neil has been around since the 70s recording and producing artists like Kiss (oh the stories...), Bruce Springsteen, Dire Straits, Sting etc. Enough said?Pat also brought along renowned live and session drummer Nir Z, whose credits include such diverse acts as Genesis and Joss Stone, to play his GMS drums for the sessions. The team was rounded out by Mattias Eklund and Henrik Kjellberg from T oontrack Music, and together they performed a number of test recordings throughout 2005.The sounds for EZdrummer were finally recorded and produced at Avatar Studios New Y ork (formerly known as Power Station) on the 1st of October, 2005, by PatThrall, Neil Dorfsman, and Nir Z. Needless to say the recordings ended up every bit as great (and better) as expected.The timeless quality of the sound, the consistency of the playing and recording, and the legendary atmosphere from a studio that has seen many of the greats create their masterpieces within its walls, all make for a worthy start of the next generation of Toontrack Acoustic Drum samplers.EZdrummer82 - INSTALLATION 2.1 EZdrummer at a glancedfhEZdrummer is a state-of-the-art sample player powering a collection of stunning drum sounds played by a top notch session drummer and world class producers at Avatar Studios, New Y ork:• 7500 sound files at 16-bit / 44.1kHz equivalent to 5Gb of uncompressed wav files • Instant access to a large MIDI library with drag’n’drop functionality• Possibility for the user to add their own MIDI files to the library• Internal mixer with stereo and multitrack routing into the host• Preset mix modes for quick sound changes• Interface visualizes the drums and allows quick audition of the kit• Automatically combines drum hit randomizing and non-cycling• Controls for instant changes to MIDI data, extending groove context relevance • Operates in General MIDI and extend beyond the limits imposed by the standard • Direct manual, tutorial and internet help desk access from the program interface • First EZX expansion pack included with EZdrummer2.2 System Requirements• 1,5Gb free hard disc space, DVD drive• Windows XP , PIII/Athlon 1,8GHz with 512 Mb of Ram• Mac OS X 10.4, G4 1GHz with 512 Mb of Ram• Display capable of 800x600• A software sequencer or virtual instrument host• (recommended) sound card with ASIO or CoreAudio driver2.3 Installing EZdrummer for WindowsRun the EZdrummer Installer and optional EZX Cocktail Installer located in the \Install\ folder of the DVD and follow instructions.The plugin will be installed by default in the appropriate location for the currently installed host program. Y ou should however verify that this is the case and perform a custom install to change the destination target if this is not adequate for your host program (see your host manual for details).The sounds themselves, as well as various resources, are installed by default in:C:\Program Files\T oontrack\EZdrummer\Sounds\. Y ou may choose to install in a different location but samples cannot be relocated at a later stage.T o uninstall simply re-run the installer located on the media that came with thisproduct or use your operating system’s removal facility.EZdrummer92.4 Installing EZdrummer for MacintoshRun the EZdrummer Installer and optional EZX Cocktail Installerlocated in the /Install/ folder of the DVD and follow instructions. Please ensure you are logged in as an administrator before proceeding.The plugin will be installed in the default location for your operating system and should not be moved to remain available to all users and programs. The sounds themselves are installed in /Library/Application Support/EZdrummer/Sounds/ and cannot be relocated. Doing so will render the program unusable.T o uninstall simply run the EZdrummer Uninstaller provided and select thecomponents you wish to remove (it is possible to uninstall EZX Cocktail only using the appropriate Uninstaller).2.5 Authorizing EZdrummer on your computerOn first launching EZdrummer you will be presented with the authorization screen. Simply follow the instructions and, if this is your first T oontrack product, create a new user account at /register/1) Key in the Computer ID exactly as shown in the interface and serial number found on the DVD packaging. Add a short description (this can be anyting you want, for example ‘Studio B computer’).2) Generate the Authorization Code online. Y ou will receive a confirmation email. T ype in or paste the code if your application supports it.3) Y ou will be greeted with a congratulation message once EZdrummer has been authorized successfully.**********************************with your Computer ID and serial number if the authorization process fails for whatever reason.EZdrummer 103 - Quick Start GuideUsing EZdrummer is quite simple, and in this tutorial we’ll show you how to perform the most common operations. By the time you finish, you’ll know how to create a killer drum track in no time.Before you start you should ensure that your system is configured for basic audio and MIDI playback. Should you be unable to complete this tutorial, check first that your program is correctly set up and that you are able to audition other virtual instruments.3.1 Loading a KitWhen the plugin is first started the default drumcounter emphasized in the above screenshot will inform you of how much memory the kit uses.A visual representation will occupy the greatest part of theinterface of EZdrummer. If you want to hear what thedrums sound like simply click on them in the interface.If you would like to select a different drum at a certainposition (or the whole kit) simply click the constructionbar on each part of the kit and select from the menu thatcomes up:3.2 Browsing the MIDI Librarydries out? Stay in this window! All the levels between the drums in the kit are preset and the sounds are already mixed so you don’t have to worry about that... just concentrate on the music.Click the ‘Open Grooves’ button. The browser will open, allowing you to access the MIDI files that come with EZdrummer. Even without the optional expansion packs, EZdrummer ships with thousands of MIDI files to choose from.Finding the MIDI groove you want for your song could not be any easier: simply choose the overall style... let’s try the POP/ROCK library, and choose a “POP/ROCK Straight” feel in 4/4 time. Finally, select one of the Playing Variations.player section and listen to the loop. Note the beat indicator underneath the groove description.Change of tempos in your sequencer will automatically be reflected in EZdrummer’s. Instant access to a ‘double time’ or ‘half time’ variation of the groove is also available at the push of a button:example, if the playing is too aggressive for that laid back bridge youhad in mind), you can effortlessly refine the dynamics, from soft tohard at the twist of a knob using the velocity sweep control.3 through the grooves with the up/down navigationarrows. EZdrummer will seamlessly play the pat-terns as you browse through them.Once you have found the right groove to lift yoursong, simply drag and drop the MIDI file to yoursequencer right where it belongs. EZ! And there isno reason to stop there:Combine different patterns and join them together with amazing fills. We reckon you will have built your first track before dinner’s ready... how many times did that happen last year?Once you’ve dragged some MIDI files to a track, your sequencer will replay the grooves in the order they were placed. Of course, EZdrummer will synchronize to your sequencer’s master tempo, so you can change your mind and speed the song up, or slow it down, at any time.When EZdrummer is receiving MIDI information from the host, as aresult of playing back a sequence or playing an external MIDI controller,the activity LED will flash to confirm that the link is working properly.Still if you prefer your track machine-like we won’t stop you!EZdrummer3.4 Mixing the Kittrack, EZdrummer includes an internal mixer, similar tohardware you’ve probably used.Just like a real mixer you use the faders to set the levels of the different drums in the mix. Horizontal sliders at the top adjust the placement of the instruments. The global control to the left of the channel strips toggle between audience and drummer’s perspective, the latter being the default.Also like with a hardware mixer, you can mute one of the tracks to listen to a subset of the drums making up the kit. Or you can solo any drum, to hear it on its own.Y ou control how much of the room you want to be part of your drum track withthe fader farthest to the right. T urning the leakage in the snare bottom or overhead microphones OFF is also possible for that extra ‘dry’ sound.Y ou can also group the channels to slide, mute or ‘solo’ as a group.For example, to adjust the volume of all the toms at the sametime, multi-select their channels by clicking them one after theother. Click on any channel once more to deselect it.If you don’t want to mix the whole kit from scratch, there are preset mixers to change the overall character of your drum kit. Just choose one of the mixer configurations in the PRESETS pull-down menu in the upper left corner of the interface. Once you are happy with your mix, you can save a snapshot for use in your other projects. Simply select ‘Save As’ from the pull down menu and type a suitable description.4 - Additional Functionality4.1 Advanced RoutingEZdrummer routes into your host on one stereo track by default.Y ou can however route any instrument or microphone toany of the 8 available stereo tracks mapped to EZdrummer’soutputs. This will allow you to benefit from the maximumflexibility that your sequencer has to offer.T o perform the above click one of the mixer tracks andselect multichannel. This will select the most appropriaterouting for the kit. Of course your host has to be set upaccordingly to capture these outputs (see your application’smanual for details).Y ou are not limited to this configuration howeverand are free to assign drums to the track of yourchoosing by selecting the appropriate entry in thepull menu for each of the indivual channels.An alternative is to start in stereo mode andseparate a single instrument from the mix.As an exercise, go back to the stereo mode andthen try sending the kick to track 2:The kick will appear on track 2, the rest of the kitremaining on track 1, the default stereo pair.4.2 Help MenuThe help menu [?] gives you quick access to additional resources:- T ool Tips: turn the contextual tips ON or OFF- Visual Hits: turn the drum animation ON or OFF- PDF Manual: opens this manual in your PDF viewer application- Flash T utorial: a short walkthrough of EZdrummer- Online Support: opens the support website in the default browser- User MIDI folder: opens the MIDI folder reserved for your own MIDIIn addition specific resources, such as keyboard layout and recording notes will be available in product specific subfolders.4.3 Adding MIDI Grooves to the BrowserFuture expansion packs will of course ship with more MIDI grooves that are relevant to the genres they aim to address. Y ou may however extend and customize the library at any time with 3rd party MIDI packs or your own.The process is very straightforward: selecting the ‘User MIDI folder’ from the Help Menu will automatically open the relevant folder on your Desktop. Simply place your MIDI files in that location, and organize them in subfolders labelled as you see fit.On a related subject, note that grooves included in EZdrummer are not GM compliant and will not play back properly on GM devices. The hi-hat programming in particular makes use of the full extent of EZdrummer available articulations.4.4 Key MappingEZdrummer is a flexible tool that will not only allow Array you to build your drum track using the includedMIDI grooves library but also create patterns andfills from scratch in your sequencer.The layout on the left details the instruments andtechniques available for triggering from an externalMIDI controller or pencil in in your application‘piano roll’.Note that the map does extend below 20 and above65. These notes are reserved for use with futureEZX-s and should not be used with the defaultRock/Pop kit.If GM compatibility is important to you you shouldalways program the map between C1 and C3 only.This is to ensure playback on GM compatible devicesis accurate (cymbal chokes notwithstanding).Specificallly, all notes in this range are GM compliantswith the following exceptions: 39, 54, 58, 60.4.5 Expanding EZdrummerThey contain additional MIDI files toThe sounds are tweaked with specificThe first EZXT o access the expansion packs already installed on yourcomputer click the EZX display in the main window.When loading an EZX, an interactive picture of the drumkit contained in that expansion will appear in the main window of EZdrummer, giving instant access to all the prelistening and construction features specific to that particular expansion.Several EZX-s are planned or already in the works and will be announced shortly at . For now enjoy EZdrummer and your first EZX, and please let us know what expansion packs you would like to see released in the future. We will be delighted to hear from you!Why not check out Superior Drummer, EZdrummer’s bigger brother, the widely acclaimed professional line from T oontrack Music. Superior Drummer gives you even more control over your drum track with an endless variety of sounds that can be mixed to fit any style and any song. T urn to the back of this manual for a brief overview of what pros around the world use! More details at NEW! NEW!。
audiomentations用法
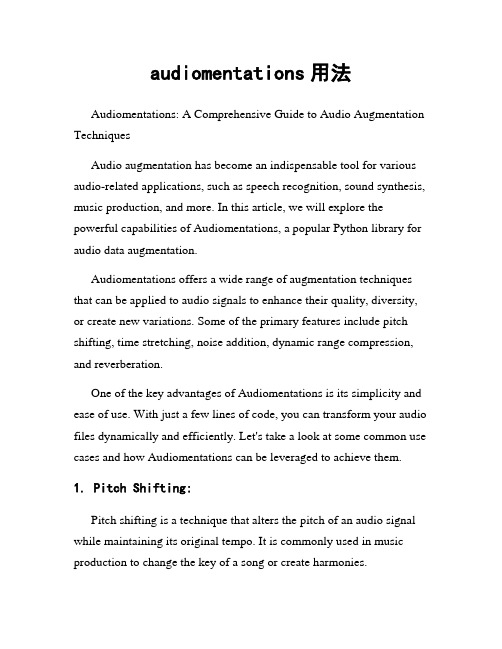
audiomentations用法Audiomentations: A Comprehensive Guide to Audio Augmentation TechniquesAudio augmentation has become an indispensable tool for various audio-related applications, such as speech recognition, sound synthesis, music production, and more. In this article, we will explore the powerful capabilities of Audiomentations, a popular Python library for audio data augmentation.Audiomentations offers a wide range of augmentation techniques that can be applied to audio signals to enhance their quality, diversity, or create new variations. Some of the primary features include pitch shifting, time stretching, noise addition, dynamic range compression, and reverberation.One of the key advantages of Audiomentations is its simplicity and ease of use. With just a few lines of code, you can transform your audio files dynamically and efficiently. Let's take a look at some common use cases and how Audiomentations can be leveraged to achieve them.1. Pitch Shifting:Pitch shifting is a technique that alters the pitch of an audio signal while maintaining its original tempo. It is commonly used in music production to change the key of a song or create harmonies.Audiomentations provides a flexible interface to pitch shift audio signals, allowing you to specify the desired shift in semitones.2. Time Stretching:Time stretching alters the duration of an audio signal without affecting its pitch. This can be useful for adjusting the tempo of a music track, creating sound effects, or synchronizing audio with visual content. Audiomentations incorporates time stretching algorithms that can be controlled by specifying the desired stretch factor.3. Noise Addition:Adding noise to an audio signal can simulate real-world environments or help improve the robustness of machine learning models. Audiomentations has a variety of noise generators, including white noise, pink noise, and brown noise, that can be easily applied to audio signals.4. Dynamic Range Compression:Dynamic range compression techniques reduce the difference between the loudest and softest parts of an audio signal. This can be helpful in improving the intelligibility of speech or making the audio more consistent. Audiomentations offers a range of compression algorithms and parameters, giving you control over the desired level of compression.5. Reverberation:Reverberation is a crucial component of audio processing, providing a sense of space or ambiance to sound recordings. Audiomentations includes reverberation algorithms that allow you to generate various types of reverbs, such as room, hall, or plate reverbs, and control parameters like decay time and pre-delay.In conclusion, Audiomentations is a versatile Python library that empowers audio engineers, researchers, and enthusiasts to augment audio signals effortlessly. Whether you are working on music production, sound design, or machine learning applications, Audiomentations provides an extensive set of tools to manipulate and transform audio data. Start exploring the possibilities of audio augmentation with Audiomentations today!。
fuurecord-recordingtools
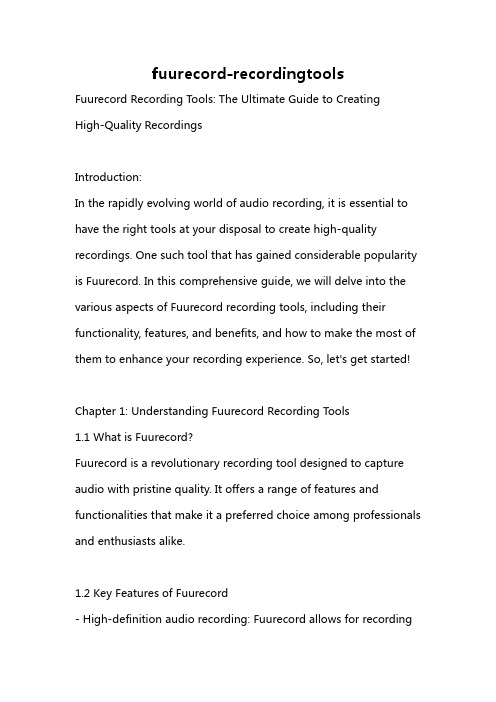
fuurecord-recordingtoolsFuurecord Recording Tools: The Ultimate Guide to CreatingHigh-Quality RecordingsIntroduction:In the rapidly evolving world of audio recording, it is essential to have the right tools at your disposal to create high-quality recordings. One such tool that has gained considerable popularity is Fuurecord. In this comprehensive guide, we will delve into the various aspects of Fuurecord recording tools, including their functionality, features, and benefits, and how to make the most of them to enhance your recording experience. So, let's get started!Chapter 1: Understanding Fuurecord Recording Tools1.1 What is Fuurecord?Fuurecord is a revolutionary recording tool designed to capture audio with pristine quality. It offers a range of features and functionalities that make it a preferred choice among professionals and enthusiasts alike.1.2 Key Features of Fuurecord- High-definition audio recording: Fuurecord allows for recordingaudio with exceptional clarity and precision, capturing everything from whispers to booming sounds with remarkable accuracy.- Wide frequency response: With an extended frequency range, Fuurecord can accurately reproduce sounds across the entire audible spectrum, ensuring no detail goes unnoticed.- Real-time monitoring: Fuurecord provides real-time monitoring of input sources, enabling users to make adjustments on the fly and ensuring optimal audio levels during recording.- Built-in audio enhancement tools: Fuurecord offers a comprehensive suite of audio processing tools, including noise reduction, EQ, compression, and reverb, allowing users to refine their recordings and achieve professional-grade sound.- Multi-track recording: With multi-track recording capabilities, Fuurecord enables users to record multiple sources simultaneously, making it the perfect tool for capturing performances or mixing sessions.Chapter 2: Setting Up Fuurecord Recording Tools2.1 System RequirementsTo utilize Fuurecord recording tools effectively, ensure that your system meets the necessary requirements in terms of operating system, processor, memory, and storage.2.2 Installing Fuurecord SoftwareFollow the step-by-step instructions provided on the Fuurecord website or the software package to install the necessary software for your recording tools. Ensure that you have the latest version to benefit from the latest features and bug fixes.Chapter 3: Optimizing Fuurecord Recording Settings3.1 Configuring Audio SettingsAccess the audio settings within the Fuurecord software to adjust sample rates, bit depth, buffer size, and input/output settings. Adapting these settings to your specific needs will help ensure optimal recording performance and quality.3.2 Setting Up Input SourcesConnect your microphones, instruments, or other audio sources to the appropriate inputs on your audio interface. Configure the input settings within Fuurecord to match the connected devices and ensure proper signal flow.Chapter 4: Recording with Fuurecord Recording Tools4.1 Selecting the Recording ModeFuurecord offers various recording modes, such as single track, multi-track, or loop recording. Choose the appropriate mode based on your recording requirements and preferences.4.2 Preparing for RecordingFine-tune the input levels using the provided meters and gain controls. Ensure that the recording environment is free from unwanted background noise or interference to achieve the best possible recording results.4.3 Recording Techniques and Best PracticesExperiment with microphone placement, gain staging, and other recording techniques to capture the desired sound accurately. Remember to maintain steady levels and avoid clipping to prevent distortion.Chapter 5: Post-Processing with Fuurecord Recording Tools5.1 Editing and Arranging TracksUse the Fuurecord software's editing capabilities to trim, cut, and arrange recorded tracks. This allows for more precise control over the final musical or audio composition.5.2 Applying Audio EnhancementsUtilize the built-in audio enhancement tools to clean up recordings, remove background noise, balance frequencies with EQ, and apply dynamic processing to achieve a polished sound.5.3 Mixing and MasteringUtilize Fuurecord's multi-track capabilities to mix recorded tracks together, adjusting levels, panning, and applying effects. Once the mix is finalized, use the mastering features to optimize the overall sound for a professional release.Conclusion:Fuurecord recording tools have revolutionized the way audio is captured in the digital age. With their advanced features, ease of use, and exceptional recording quality, they have become a go-to choice for professionals and enthusiasts alike. By following the steps outlined in this guide, you can make the most of your Fuurecord tools, ensuring the creation of high-quality recordings that truly shine. So, go ahead and unleash your creativity with Fuurecord!。
TinyX显示驱动在ARM开发板上的移植
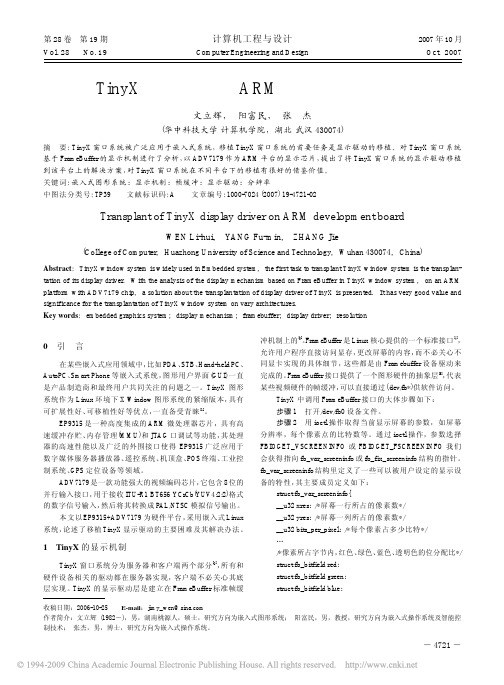
YUV4: 2: 2 采用的是打包格式,它为每个像素保留 Y 分 量,而 UV 分量在水平方向上每两个像素采样一次[8]。一个宏 像素为 4 个字节,实际表示 2 个像素。(4:2:2 的意思为一个宏 像素中有 4 个 Y 分量、2 个 U 分量和 2 个 V 分量。)图像数据中 YUV 分量排列顺序为:y0 u y1 v y2 u y3 v…,其中,y0 为左点 的亮度值;y1 为右点的亮度值。u 和 v 为两个点共享的色度值。
接 下 来 还 需 创 建 用 于 存 放 YUV 数 据 的 缓 冲 区 和 设 计 RGB 转 YUV 的转换程序,最后将 YUV 数据缓冲区中的数据 写入到显示芯片 ADV7179。 3.1 YUV 数据 缓 冲 区 的 设 计
YUV4:2:2 格式是打包格式,缓冲区的数据相应地也设计 成按 YUV4:2:2 格式存放。对于 704×576 分辨率的电视屏幕, 其对应的 YUV 数据缓冲区的大小为 704×576×2 个字节。
2 ADV7179 的输入信号格式
ADV7179 的输入格式是 YUV4: 2:2。YUV 是另外一种表 示 颜 色 信 息 的 标 准 ,广 泛 被 视 频 和 电 视 信 号 传 输 采 用 ,它 用 亮 度信号 Y 和色度信号 U、V 表示颜色。如果只有 Y 信号分量 而没有 U、V 分量,那么这样表示的图像就是黑白灰度图像, 因此可以在黑白电视中显示。
YUV4: 2: 2 格式表示的是扫描线上两个点共用 U、V 色度 值,当两个点的亮度值 Y 差别不是很大时,人眼有可能对这两 个 像 素 点 显 示 的 图 像 分 辨 不 清 。 因 此 ,电 视 屏 幕 适 合 显 示 过 渡比较明显的画面。而图形系统对画面的质量要求比较高, 例如绘制一条一个像素宽度的直线都要能清晰的显示出来。 为了解决清晰度的问题,我们让两个点的 Y 值也相等,即这两 个点都对应虚拟屏幕缓冲区中的一个 RGB 颜色格式的像素 点。采用这种机制,TinyX 的图像显示到电视屏幕上,宽度将 放 大 到 原 来 的 两 倍 ,但 这 时 字 体 图 像 显 示 出 来 将 会 变 成 很 难 看长条形状,为了让字体图像也保持正方形显示,就必须将高 度放大到原来的两倍,即电视屏幕垂直方向上两个点的 YUV 值也相等,也都对应一个 RGB 颜色格式的像素点 (如图 2 所 示)。这样,TinyX 虚拟屏幕缓冲区的水平分辨率、垂直分辨率 都降到电视屏幕的一半,为 352× 288。
老年听力损失的筛查工具
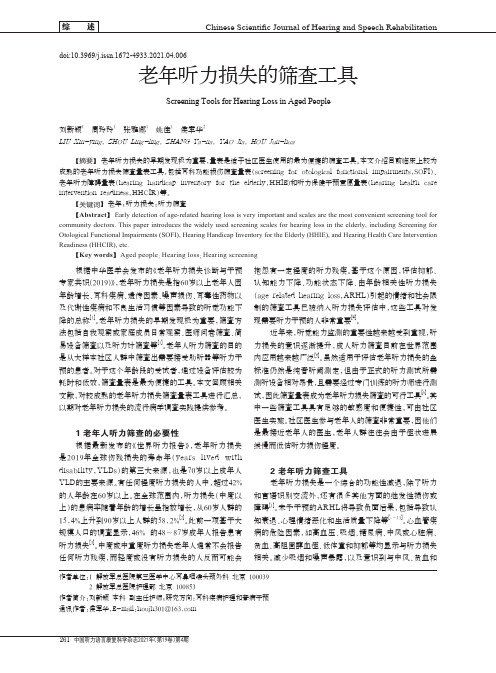
是从大样本社区人群中筛查出需要接受助听器等听力干 内应用越来越广泛[5]。虽然适用于评估老年听力损失的金
预的患者。对于这个年龄段的受试者,通过设备评估较为 标准仍然是纯音听阈测定,但由于正式的听力测试所需
耗时和低效,筛查量表是最为便捷的工具。本文回顾相关 测听设备相对昂贵,且需要经过专门训练的听力师进行测
作者单位:1 解放军总医院第三医学中心耳鼻咽喉头颈外科 北京 100039 2 解放军总医院护理部 北京 100853
作者简介:刘新颖 本科 副主任护师;研究方向:耳科疾病护理和聋病干预 通讯作者:侯军华,E-mail:houjh301@
261 中国听力语言康复科学杂志2021年(第19卷)第4期
E-12 听力问题会使您感到紧张吗?
S-13 E-14* S-15*
是否由于听力问题,您不愿像以往那样经常探亲访友了? 听力问题会引起您与家人的争吵吗? 在看电视或听广播时,听力问题是否会使您感到困难?
文献,对较成熟的老年听力损失筛查量表工具进行汇总, 试,因此筛查量表成为老年听力损失筛查的可行工具[6]。其
以期对老年听力损失的流行病学调查实践提供参考。
中一些筛查工具具有足够的敏感度和便捷性,可由社区
医生实施。社区医生参与老年人的筛查非常重要,因他们
1 老年人听力筛查的必要性
是最接近Hale Waihona Puke 年人的医生,老年人群往往会由于症状进展
【关键词】 老年;听力损失;听力筛查 【Abstract】 Early detection of age-related hearing loss is very important and scales are the most convenient screening tool for community doctors. This paper introduces the widely used screening scales for hearing loss in the elderly, including Screening for Otological Functional Impairments (SOFI), Hearing Handicap Inventory for the Elderly (HHIE), and Hearing Health Care Intervention Readiness (HHCIR), etc. 【Key words】Aged people; Hearing loss; Hearing screening
EVALUATION OF MUSICAL FEATURES FOR EMOTION CLASSIFICATION
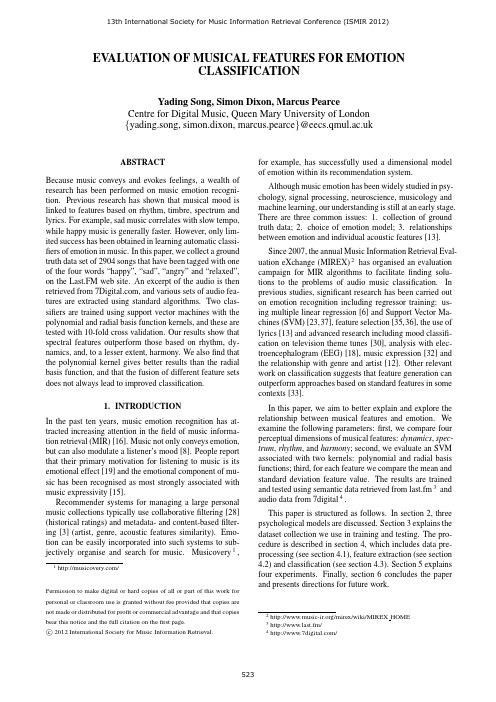
EV ALUATION OF MUSICAL FEATURES FOR EMOTIONCLASSIFICATIONYading Song,Simon Dixon,Marcus PearceCentre for Digital Music,Queen Mary University of London{yading.song,simon.dixon,marcus.pearce}@ABSTRACTBecause music conveys and evokes feelings,a wealth of research has been performed on music emotion recogni-tion.Previous research has shown that musical mood is linked to features based on rhythm,timbre,spectrum and lyrics.For example,sad music correlates with slow tempo, while happy music is generally faster.However,only lim-ited success has been obtained in learning automatic classi-fiers of emotion in music.In this paper,we collect a ground truth data set of2904songs that have been tagged with one of the four words“happy”,“sad”,“angry”and“relaxed”, on the Last.FM web site.An excerpt of the audio is then retrieved ,and various sets of audio fea-tures are extracted using standard algorithms.Two clas-sifiers are trained using support vector machines with the polynomial and radial basis function kernels,and these are tested with10-fold cross validation.Our results show that spectral features outperform those based on rhythm,dy-namics,and,to a lesser extent,harmony.We alsofind that the polynomial kernel gives better results than the radial basis function,and that the fusion of different feature sets does not always lead to improved classification.1.INTRODUCTIONIn the past ten years,music emotion recognition has at-tracted increasing attention in thefield of music informa-tion retrieval(MIR)[16].Music not only conveys emotion, but can also modulate a listener’s mood[8].People report that their primary motivation for listening to music is its emotional effect[19]and the emotional component of mu-sic has been recognised as most strongly associated with music expressivity[15].Recommender systems for managing a large personal music collections typically use collaborativefiltering[28] (historical ratings)and metadata-and content-basedfilter-ing[3](artist,genre,acoustic features similarity).Emo-tion can be easily incorporated into such systems to sub-jectively organise and search for music.Musicovery1, 1/Permission to make digital or hard copies of all or part of this work for personal or classroom use is granted without fee provided that copies are not made or distributed for profit or commercial advantage and that copies bear this notice and the full citation on thefirst page.c 2012International Society for Music Information Retrieval.for example,has successfully used a dimensional model of emotion within its recommendation system.Although music emotion has been widely studied in psy-chology,signal processing,neuroscience,musicology and machine learning,our understanding is still at an early stage. There are three common issues:1.collection of ground truth data;2.choice of emotion model;3.relationships between emotion and individual acoustic features[13].Since2007,the annual Music Information Retrieval Eval-uation eXchange(MIREX)2has organised an evaluation campaign for MIR algorithms to facilitatefinding solu-tions to the problems of audio music classification.In previous studies,significant research has been carried out on emotion recognition including regressor training:us-ing multiple linear regression[6]and Support Vector Ma-chines(SVM)[23,37],feature selection[35,36],the use of lyrics[13]and advanced research including mood classifi-cation on television theme tunes[30],analysis with elec-troencephalogram(EEG)[18],music expression[32]and the relationship with genre and artist[12].Other relevant work on classification suggests that feature generation can outperform approaches based on standard features in some contexts[33].In this paper,we aim to better explain and explore the relationship between musical features and emotion.We examine the following parameters:first,we compare four perceptual dimensions of musical features:dynamics,spec-trum,rhythm,and harmony;second,we evaluate an SVM associated with two kernels:polynomial and radial basis functions;third,for each feature we compare the mean and standard deviation feature value.The results are trained and tested using semantic data retrieved from last.fm3and audio data from7digital4.This paper is structured as follows.In section2,three psychological models are discussed.Section3explains the dataset collection we use in training and testing.The pro-cedure is described in section4,which includes data pre-processing(see section4.1),feature extraction(see section 4.2)and classification(see section4.3).Section5explains four experiments.Finally,section6concludes the paper and presents directions for future work.2/mirex/wiki/MIREX HOME3st.fm/4/2.PSYCHOLOGICAL EMOTION MODELS One of the difficulties in representing emotion is to distin-guish music-induced emotion from perceived emotion be-cause the two are not always aligned[5].Different psycho-logical models of emotion have been compared in a study of perceived emotion[7].Most music related studies are based on two popular approaches:categorical[10]and dimensional[34]mod-els of emotion.The categorical approach describes emo-tions with a limited number of innate and universal cate-gories such as happiness,sadness,anger and fear.The di-mensional model considers all affective terms arising from independent neurophysiological systems:valence(nega-tive to positive)and arousal(calm to exciting).Recently a more sophisticated model of music-induced emotion-the Geneva Emotion Music Scale(GEMS)model-consisting of9dimensions,has been proposed[42].Our results and analysis are based on the categorical model since we make our data collection through human-annotated social tags which are categorical in nature.3.GROUND-TRUTH DATA COLLECTIONAs discussed above,due to the lack of ground truth data, most researchers compile their own databases[41].Man-ual annotation is one of the most common ways to do this. However,it is expensive in terms offinancial cost and hu-man labour.Moreover,terms used may differ between in-dividuals.Different emotions may be described using the same term by different people which would result in poor prediction[38].However,with the emergence of music discovery and recommendation websites such as last.fm which support social tags for music,we can access rich human-annotated pared with the tradi-tional approach of web mining which gives noisy results, social tagging provides highly relevant information for mu-sic information retrieval(MIR)and has become an im-portant source of human-generated contextual knowledge [11].Levy[24]has also shown that social tags give a high quality source of ground truth data and can be effective in capturing music similarity[40].Thefive mood clusters proposed by MIREX[14](such as rollicking,literate,and poignant)are not popular in so-cial tags.Therefore,we use four basic emotion classes: happy,angry,sad and relaxed,considering these four emo-tions are widely accepted across different cultures and cover the four quadrants of the2-dimensional model of emo-tion[22].These four basic emotions are used as seeds to retrieve the top30tags from last.fm.We then obtain a list of songs labelled with the retrieved tags.Table1and table 2show an example of the retrieved results.Given the retrieved titles and the names of the singers, we use a public API to get previewfiles.The results cover different types of pop music,meaning that we avoid partic-ular artist and genre effects[17].Since the purpose of this step is tofind ground truth data,issues such as cold start, noise,hacking,and bias are not relevant[4,20].Most datasets on music emotion recognition are quiteHappy Angry Sad Relaxhappy angry sad relax happy hardcore angry music sad songs relax trance makes me happy angry metal happysad relax music happy music angry pop music sad song jazz relax happysad angry rock sad&beautiful only relax Table1.Top5tags returned by last.fmSinger TitleNoah And The Whale5Years TimeJason Mraz I’m YoursRusted Root Send Me On My WayRoyksopp Happy Up HereKaren O and the Kids All Is LoveTable2.Top songs returned with tags from the“happy”category.small(less than1000items),which indicates that2904 songs(see table3)for four emotions retrieved by social tags is a good size for the current experiments.The dataset will be made available5,to encourage other researchers to reproduce the results for research and evaluation.Emotion Number of SongsHappy753Angry639Sad763Relaxed749Overall2904Table3.Summary of ground truth data collection4.PROCEDURESThe experimental procedure consists of four stages:data collection,data preprocessing,feature extraction,and clas-sification,as shown infigure1.4.1Data PreprocessingAs shown in Table1,there is some noise in the data such as confusing tags and repeated songs.We manually remove data with the tag happysad which existed in both the happy and sad classes and delete the repeated songs,to make sure every song will only exist once in a single class.Moreover, we convert our dataset to standard wav format(22,050Hz sampling rate,16bit precision and mono channel).The song excerpts are either30seconds or60seconds,rep-resenting the most salient part of the song[27],therefore there is no need to truncate.At the end,we normalise the excerpts by dividing by the highest amplitude to mitigate the production effect of different recording levels.4.2Feature ExtractionAs suggested in the work of Saari and Eerola[35],two dif-ferent types of feature(mean and standard deviation)with 5The dataset can be found at https:///projects-/emotion-recognitionFigure1.Procedurea total of55features were extracted using the MIR tool-box6[21](shown in table4).The features are categorized into the following four perceptual dimensions of music lis-tening:dynamics,rhythm,spectral,and harmony.4.3ClassificationThe majority of music classification tasks[9](genre clas-sification[25,39],artist identification[29],and instrument recognition[31])have used k-nearest neighbour(K-NN) [26]and support vector machines(SVM)[2].In the case of audio input features,the SVM has been shown to per-form best[1].In this paper,therefore,we choose support vector ma-chines as our classifier,using the implementation of the se-quential minimal optimisation algorithm in the Weka data mining toolkit7.SVMs are trained using polynomial and radial basis function(RBF)kernels.We set the cost factor C=1.0,and leave other parameters unchanged.An in-ternal10-fold cross validation is applied.To better under-stand and compare features in four perceptual dimensions, our experiments are divided into four tasks.Experiment1:we compare the performance of the two kernels(polynomial and RBF)using various features.Experiment2:four classes(perceptual dimensions)of features are tested separately,and we compare the results tofind a dominant class.Experiment3:two types of feature descriptor,mean and standard deviation,are calculated.The purpose is to com-pare values for further feature selection and dimensionality reduction.6Version1.3.3:https://www.jyu.fi/music/coe/materials/mirtoolbox 7/ml/weka/Dimen.No.Features Acronyms Dynamics1-2RMS energy RMSm,RMSstd 3-4Slope Ss,Sstd5-6Attack As,Astd7Low energy LEm Rhythm1-2Tempo Ts,Tstd3-4Fluctuation peak(pos,mag)FPm,FMm5Fluctuation centroid FCm Spec.1-2Spectrum centroid SCm,SCstd3-4Brightness BRm,BRstd5-6Spread SPm,SPstd7-8Skewness SKm,SKstd9-10Kurtosis Km,Kstd11-12Rolloff95R95s,R95std13-14Rolloff85R85s,R85std15-16Spectral Entrophy SEm,SEstd17-18Flatness Fm,Fstd19-20Roughness Rm,Rstd21-22Irregularity IRm.IRstd23-24Zero crossing rate ZCRm,ZCRstd25-26Spectralflux SPm,SPstd27-28MFCC MFm,MFstd29-30DMFCC DMFm,DMFstd31-32DDMFCC DDm,DDstd Harmony1-2Chromagram peak CPm,CPstd3-4Chromagram centroid CCm,CCstd5-6Key clarity KCm,KCstd7-8Key mode KMm,KMstd9-10HCDF Hm,Hstd Table4.The feature set used in this work;m=mean,std =standard deviation.Experiment4:different combinations of feature classes (e.g.,spectral with dynamics)are evaluated in order to de-termine the best-performing model.5.RESULTS5.1Experiment1In experiment1,SVMs trained with two different kernels are compared.Previous studies[23]have found in the case of audio input that the SVM performs better than other classifiers(Logistic Regression,Random Forest,GMM, K-NN and Decision Trees).To our knowledge,no work has been reported explicitly comparing different kernels for SVMs.In emotion recognition,the radial basis func-tion kernel is a common choice because of its robustness and accuracy in other similar recognition tasks[1].Polynomial RBFFeature Class Accuracy Time Accuracy Time No.Dynamics37.20.4426.332.57Rhythm37.50.4434.523.25Harmony47.50.4136.627.410Spectral51.90.4048.114.332 Table5.Experiment1results:time=model building time, No.=number of features in each classThe results in table5show however that regardless of the features used,the polynomial kernel always achieved the higher accuracy.Moreover,the model construction times for each kernel are dramatically different.The av-erage construction time for the polynomial kernel is0.4 seconds,while the average time for the RBF kernel is24.2seconds,around60times more than the polynomial ker-nel.The following experiments also show similar results. This shows that polynomial kernel outperforms RBF in the task of emotion recognition at least for the parameter val-ues used here.5.2Experiment2In experiment2,we compare the emotion prediction re-sults for the following perceptual dimensions:dynamics, rhythm,harmony,and spectral.Results are shown infig-ure2).Dynamics and rhythm features yield similar re-sults,with harmony features providing better results,but the spectral class with32features achieves the highest ac-curacy of51.9%.This experiment provides a baseline model, and further exploration of multiple dimensions is performed in experiment4.parison of classification results for the four classes of features.5.3Experiment3In this experiment,we evaluate different types of feature descriptors,mean value and standard deviation for each feature across all feature classes,for predicting the emotion in music.The results in table6show that the use of both mean and standard deviation values gives the best results in each case.However,the processing time increased,so choosing the optimal descriptor for each feature is highly desirable.For example,choosing only the mean value in the harmony class,we lose2%of accuracy but increase the speed while the choice of standard deviation results in around10%accuracy loss.As the number of features in-creases,the difference between using mean and standard deviation will be reduced.However,more experiments are needed to explain why the mean in harmony and spectral features,and standard deviation values of dynamics and rhythm features have higher accuracy scores.5.4Experiment4In order to choose the best model,thefinal experiment fuses different perceptual features.As presented in table7, optimal accuracy is not produced by the combination of all features.Instead,the use of spectral,rhythm and harmony (but not dynamic)features produces the highest accuracy.Features Class Polynomial No.featuresDynamics all37.27Dynamics mean29.73Dynamics std33.83Rhythm all37.55Rhythm mean28.71Rhythm std34.21Harmony all47.510Harmony mean45.35Harmony std38.35Spectral all51.932Spectral mean49.616Spectral std47.516Spec+Dyn all52.339Spec+Dyn mean50.519Spec+Dyn std48.719Spec+Rhy all52.337Spec+Rhy mean49.817Spec+Rhy std47.817Spec+Har all53.342Spec+Har mean51.321Spec+Har std50.321Har+Rhy all49.115Har+Rhy mean45.66Har+Rhy std41.26Har+Dyn all48.817Har+Dyn mean46.98Har+Dyn std42.48Rhy+Dyn all41.712Rhy+Dyn mean32.04Rhy+Dyn std38.84parison of mean and standard deviation(std) features.Features Accuracy No.featuresSpec+Dyn52.339Spec+Rhy52.337Spec+Har53.342Har+Rhy49.115Har+Dyn48.817Rhy+Dyn41.712Spec+Dyn+Rhy52.444Spec+Dyn+Har53.849Spec+Rhy+Har54.047Dyn+Rhy+Har49.722All Features53.654Table7.Classification results for combinations of feature sets.6.CONCLUSION AND FUTURE WORKIn this paper,we collected ground truth data on the emo-tion associated with2904pop songs from last.fm tags.Au-dio features were extracted and grouped into four percep-tual dimensions for training and validation.Four experi-ments were conducted to predict emotion labels.The re-sults suggest that,instead of the conventional approach us-ing SVMs trained with a RBF kernel,a polynomial ker-nel yields higher accuracy.Since no single dominant fea-tures have been found in emotion recognition,we explored the performance of different perceptual classes of feature for predicting emotion in music.Experiment3found that dimensionality reduction can be achieved through remov-ing either mean or standard deviation values,halving the number of features used,with,in some cases,only2%ac-curacy loss.The last experiment found that inclusion of dynamics features with the other classes actually impairedthe performance of the classifier while the combination of spectral,rhythmic and harmonic features yielded optimal performance.In future work,we will expand this research both in depth and breadth,tofind features and classes of features which best represent emotion in music.We will examine higher-level dimensions such as temporal evolution fea-tures,as well as investigating the use of auditory ing the datasets retrieved from Last.fm,we will compare the practicability of social tags with other human-annotated datasets in emotion recognition.Through these studies of subjective emotion,we will develop methods for incorporating other empirical psychological data in a sub-jective music recommender system.7.ACKNOWLEDGEMENTSWe acknowledge the support of the Queen Mary University of London Postgraduate Research Fund(QMPGRF)and the China Scholarship Council.We would like to thank the reviewers and Emmanouil Benetos for their advice and comments.8.REFERENCES[1]K.Bischoff,C.S.Firan,R.Paiu,W.Nejdl,urier,and M.Sordo.Music Mood and Theme Classification -A Hybrid Approach.In10th International Society for Music Information Retrieval Conference,number Is-mir,pages657–662,2009.[2]E.Boser,N.Vapnik,and I.M.Guyon.Training Algo-rithm Margin for Optimal Classifiers.In ACM Confer-ence on Computational Learning Theory,pages144–152,1992.[3]P.Cano,M.Koppenberger,and N.Wack.Content-based Music Audio Recommendation.In Proceedings of the13th annual ACM international conference on Multimedia,number ACM,pages211–212,2005. [4]O.Celma.Foafing the Music:Bridging the SemanticGap in Music Recommendation.In The Semantic Web-ISWC,2006.[5]T.Eerola.Are the Emotions Expressed in Mu-sic Genre-specific?An Audio-based Evaluation of Datasets Spanning Classical,Film,Pop and Mixed Genres.Journal of New Music Research,40(March 2012):349–366,2011.[6]T.Eerola,rtillot,and P.Toiviainen.Predictionof Multdimensional Emotional Ratings in Music from Audio Using Multivariate Regression Models.In10th International Society for Music Information Retrieval Conference,number Ismir,pages621–626,2009. [7]T.Eerola and J.K.Vuoskoski.A Comparison of theDiscrete and Dimensional Models of Emotion in Mu-sic.Psychology of Music,39(1):18–49,August2010.[8]Y.Feng and Y.Zhuang.Popular Music Retrieval byDetecting Mood.In International Society for Music In-formation Retrieval Conference,volume2,pages375–376,2003.[9]Z.Fu,G.Lu,K.M.Ting,and D.Zhang.A Sur-vey of Audio-based Music Classification and Anno-tation.IEEE Transactions on Multimedia,13(2):303–319,2011.[10]K.Hevner.Experimental studies of the elements of ex-pression in music.The American Journal of Psychol-ogy,48:246–268,1936.[11]X.Hu,M.Bay,and J.S.Downie.Creating a SimplifiedMusic Mood Classification Grouth-truth Set.In Inter-national Conference on Music Information Retrieval, pages3–4,2007.[12]X.Hu and J.S.Downie.Exploring Mood Metadata:Relationships with Genre,Artist and Usage Metadata.In8th International Conference on Music Information Retrieval,2007.[13]X.Hu,J.S.Downie,and A.F.Ehmann.Lyric Text Min-ing in Music Mood Classification.In10th Interna-tional Society for Music Information Retrieval Confer-ence,number Ismir,pages411–416,2009.[14]X.Hu,J.S.Downie, urier,and M.Bay.The2007MIREX Audio Mood Classification Task:Les-son Learned.In International Society for Music Infor-mation Retrieval Conference,pages462–467,2008.[15]P.N.Juslin,J.Karlsson,E.Lindstr¨o m,A.Friberg,andE.Schoonderwaldt.Play it Again with Feeling:Com-puter Feedback in Musical Communication of Emo-tions.Journal of experimental psychology.Applied, 12(2):79–95,June2006.[16]Y.E.Kim,E.M.Schmidt,R.Migneco,B.G.Morton,P.Richardson,J.Scott,J.A.Speck,and D.Turnbull.Music Emotion Recognition:A State of the Art Re-view.In11th International Society for Music Informa-tion Retrieval Conference,number Ismir,pages255–266,2010.[17]Y.E.Kim, D.S.Williamson,and S.Pilli.TowardsQuantifying the Album Effect in Artist Identification.In International Society for Music Information Re-trieval Conference,2006.[18]S.Koelstra,C.Muhl,and M.Soleymani.Deap:ADatabase for Emotion Analysis Using Physiological Signals.IEEE Trans.on Affective Computing,pages1–15,2011.[19]C.L.Krumhansl.Music:A Link Between Cogni-tion and Emotion.American Psychological Society, 11(2):45–50,2002.[20]mere.Social Tagging and Music Information Re-trieval.Journal of New Music Research,37(2):101–114,June2008.[21]rtillot and P.Toiviainen.MIR in Matlab(II):AToolbox for Musical Feature Extraction from Audio.In International Conference on Music Information Re-trieval,number Ii,pages237–244,2007.[22]urier and J.Grivolla.Multimodal Music MoodClassification Using Audio and Lyrics.In Int.Conf.Machine Learning and Applications,pages1–6,2008.[23]urier,P.Herrera,M.Mandel,and D.Ellis.AudioMusic Mood Classification Using Support Vector Ma-chine.In MIREX task on Audio Mood Classification, pages2–4,2007.[24]M.Levy.A Semantic Space for Music Derived fromSocial Tags.In Austrian Compuer Society,volume1, page12.Citeseer,2007.[25]B.Lines,E.Tsunoo,G.Tzanetakis,and N.Ono.Be-yond Timbral Statistics:Improving Music Classifica-tion Using Percussive.IEEE Transactions on Audio, Speech and Language Processing,19(4):1003–1014, 2011.[26]T.M.Cover and P.E.Hart.Nearest Neighbor PatternClassification.IEEE Transactions on Information The-ory,13(1):21–27,1967.[27]K.F.MacDorman,S.Ough,and C.Ho.AutomaticEmotion Prediction of Song Excerpts:Index Construc-tion,Algorithm Design,and Empirical Comparison.Journal of New Music Research,36(4):281–299,De-cember2007.[28]T.Magno and C.Sable.A Comparison of Signal ofSignal-based Music Recommendation to Genre Labels, Collaborative Filtering,Musicological Analysis,Hu-man Recommendation and Random Baseline.In Pro-ceedings of the9th International Conference of Music Information Retrieval,pages161–166,2008.[29]M.Mandel.Song-level Features and Support Vec-tor Machines for Music Classification.In Proc.Inter-national Conference on Music Information Retrieval, 2005.[30]M.Mann,T.J.Cox,and F.F.Li.Music Mood Classi-fication of Television Theme Tunes.In12th Interna-tional Society for Music Information Retrieval Confer-ence,number Ismir,pages735–740,2011.[31]J.Marques and P.J.Moreno.A Study of Musical In-strument Classification Using Gaussian Mixture Mod-els and Support Vector Machines,1999.[32]L.Mion and G.D.Poli.Score-Independent AudioFeatures for Description of Music Expression.IEEE Transactions on Audio,Speech,and Language Pro-cessing,16(2):458–466,2008.[33]F.Pachet and P.Roy.Analytical Features:AKnowledge-Based Approach to Audio Feature Gener-ation.EURASIP Journal on Audio,Speech,and Music Processing,2009(2):1–23,2009.[34]J.A.Russell,A.Weiss,and G.A.Mendelsohn.Af-fect Grid:A Single-item Scale of Pleasure and Arousal.Journal of Personality and Social Psychology, 57(3):493–502,1989.[35]P.Saari,T.Eerola,and rtillot.Generalizabilityand Simplicity as Criteria in Feature Selection:Appli-cation to Mood Classification in Music.IEEE Trans-actions on Audio,Speech,and Language Processing, 19(6):1802–1812,2011.[36]E.M.Schmidt,D.Turnbull,and Y.E.Kim.FeatureSelection for Content-Based,Time-Varying Musical Emotion Regression Categories and Subject Descrip-tors.In Multimedia Information Retrieval,pages267–273,2010.[37]B.Schuller,J.Dorfner,and G.Rigoll.Determinationof Nonprototypical Valence and Arousal in Popular Music:Features and Performances.EURASIP Journal on Audio,Speech,and Music Processing,2010:1–19, 2010.[38]D.Turnbull,L.Barrington,and nckriet.Five Ap-proaches to Collecting Tags for Music.In Proceedings of the9th International Conference of Music Informa-tion Retrieval,pages225–230,2008.[39]G.Tzanetakis and P.Cook.Musical Genre Classifica-tion of Audio Signals.IEEE Transactions on Speech and Audio Processing,10(5):293–302,2002.[40]D.Wang,T.Li,and M.Ogihara.Tags Better ThanAudio Features?The Effect of Joint use of Tags and Audio Content Features for Artistic Style Clutering.In11th International Society on Music Information Retrieval Conference,number ISMIR,pages57–62, 2010.[41]D.Yang and W.S.Lee.Disambiguating Music Emo-tion Using Software Agents.In Proceedings of the5th International Conference on Music Information Re-trieval,pages52–58,2004.[42]M.Zentner,D.Grandjean,and K.R.Scherer.Emo-tions evoked by the sound of music:characterization, classification,and measurement.Emotion(Washing-ton,D.C.),8(4):494–521,August2008.。
七年级英语音乐作品创作灵感来源单选题80题
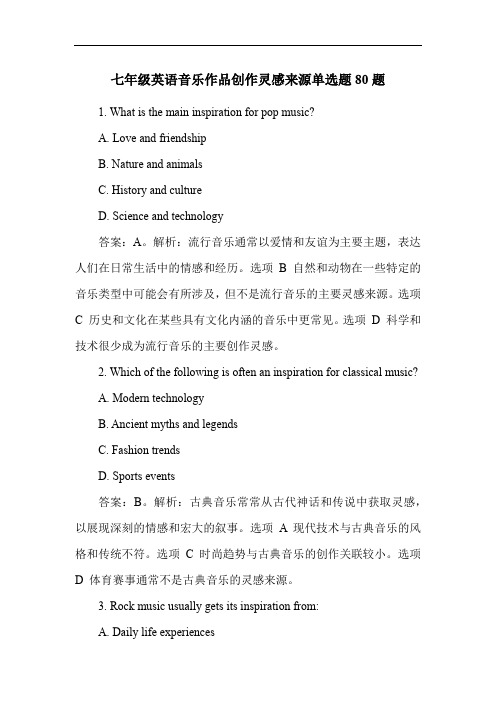
七年级英语音乐作品创作灵感来源单选题80题1. What is the main inspiration for pop music?A. Love and friendshipB. Nature and animalsC. History and cultureD. Science and technology答案:A。
解析:流行音乐通常以爱情和友谊为主要主题,表达人们在日常生活中的情感和经历。
选项B 自然和动物在一些特定的音乐类型中可能会有所涉及,但不是流行音乐的主要灵感来源。
选项C 历史和文化在某些具有文化内涵的音乐中更常见。
选项D 科学和技术很少成为流行音乐的主要创作灵感。
2. Which of the following is often an inspiration for classical music?A. Modern technologyB. Ancient myths and legendsC. Fashion trendsD. Sports events答案:B。
解析:古典音乐常常从古代神话和传说中获取灵感,以展现深刻的情感和宏大的叙事。
选项 A 现代技术与古典音乐的风格和传统不符。
选项C 时尚趋势与古典音乐的创作关联较小。
选项D 体育赛事通常不是古典音乐的灵感来源。
3. Rock music usually gets its inspiration from:A. Daily life experiencesB. Fairy talesC. Political movementsD. Religious ceremonies答案:A。
解析:摇滚音乐常常反映日常生活中的经历、情感和挑战。
选项B 童话故事不是摇滚音乐常见的灵感来源。
选项C 政治运动在一些特定的摇滚作品中可能会有所体现,但不是普遍的灵感来源。
选项D 宗教仪式很少直接成为摇滚音乐的创作灵感。
4. Jazz music is often inspired by:A. City life and urban cultureB. Rural landscapesC. Outer space explorationD. Ancient literature答案:A。
- 1、下载文档前请自行甄别文档内容的完整性,平台不提供额外的编辑、内容补充、找答案等附加服务。
- 2、"仅部分预览"的文档,不可在线预览部分如存在完整性等问题,可反馈申请退款(可完整预览的文档不适用该条件!)。
- 3、如文档侵犯您的权益,请联系客服反馈,我们会尽快为您处理(人工客服工作时间:9:00-18:30)。
First Steps to an Audio Ontology-Based Classifier forTelemedicineCong Phuong Nguyen, Ngoc Yen Pham, Eric CastelliInternational Research Center MICAHUT – CNRS/UMI2954 – INPGrenoble1, Dai Co Viet, Hanoi, Vietnam{Cong-Phuong.Nguyen, Ngoc-Yen.Pham,Eric.Castelli}@.vnAbstract. Our work is within the framework of studying and implementing asound analysis system in a telemedicine project. The task of this system is todetect situations of distress in a patient’s room based on sound analysis. If sucha situation is detected, an alarm will be automatically sent to the medical cen-tre. In this paper we present our works on building domain ontologies of suchsituations. They gather abstract concepts of sounds and these concepts, alongwith their properties and instances, are represented by a neural network. Theontology-based classifer uses outputs of networks to identify classes of audioscenes. The system is tested with a database extracted from films.1 IntroductionIn recent years telemedicine is widely studied and applied. It can be broadly defined as the transfer (e.g. telephone lines, the Internet, satellites, etc) of electronic medical data (e.g. images, sounds, live video, patient records, etc) from one location to an-other. The system that we present is developed for the surveillance of elderly, conva-lescent persons or pregnant women. Its main goal is to detect serious accidents such as falls or faintness at any place in the apartment. If a serious accident is detected, an alarm will be automatically sent to the medical centre. Firstly most people do not like to be supervised by cameras all day long while the presence of microphone can be acceptable. Secondly the supervision field of a microphone is larger than that of a camera. Thirdly, sound processing is much less time consuming than image process-ing, hence a real time processing solution can be easier to develop. Thus, the original-ity of our approach consists in replacing the video camera by a system of multichan-nel sound acquisition. The system analyzes in real time the sound environment of the apartment and detects abnormal sounds (falls of objects or patient, scream, groan) that could indicate a distress situation in the habitat.Fig. 1. The apartment used for telemedicine. Microphones are installed in each room in order to assure the sound surveillance in the whole apartmentThis system is divided into different small modules. In the process of developing it, a sound acquisition module, a sound of everyday’s life classifier, a speech/nonspeech discriminator and a speech/scream-groan discriminator are con-structed [10], [11], [12], [18]. The habitat we use for our experiments is a 30m2 apartment (depicted in Fig. 1) equiped with various sensors, especially microphones. There is one microphone in each room (toilet, kitchen, shower-room, hall and living-room) of the apartment. This installation allows a sound surveillance in the whole apartment. In this apartment, audio signals are acquired by five microphones and feed to a multichannel data acquisition card (National Instruments DAQ) installed on a slave computer. Sound source can be localized through comparison of the soun levels of the microphones. If two simultaneous detections are recorded, only the channel with the maximum signal level is considered. The microphones used are omni-directional, condenser type, small size and low cost. A signal conditional card con-sisting of an amplifier and an anti-aliasing filter is attached to each microphone. The four modules mentioned above, developed in LabWindows/CVI, process acquiredsignals to detect situations of distress.The combination of these four modules seems to be an audio classification system. It can be seen that audio classification has been studied for many years. They are applied to speech recognition, audio content-based analysis, audio segmentation, audio retrieval, broadcast news transcription, etc. Works described in [22], [4], [16], [28] are four examples among many systems. But there is a difference between the problem of audio classification and our problem. Audio classification in the literature is applied to classify different homogeneous audio segments, i.e. each segment con-sists of a unique type of audio signal (e.g. speech). Meanwhile, our system is intended to classify an audio scene containing different types (e.g. a segment of scream and a segment of fallen chair). In other words, an audio scene’s category is determined by its types of segment. In order to complete the system, we propose sound ontologies which can be used in an ontology-based classifier. Each ontology is an abstract con-cept of an audio scene representing a situation of distress in the house. It can be used to detect situations of distress, to classify audio scenes, to share informations of audio scenes among people and software, to analyze domain knowledge, or to save (as metadata) audio scenes in a database for further usages.This article is structured as follows. Sect. 2 discusses works related to ontology-based audio applications. Sect. 3 describes the proposed ontology of audio scenes and the neural network used to represent ontologies and the ontology-based classifier. Sect. 4 presents the database of audio scene and the evaluation of ontologies. Sect. 5 outlines our conclusion and next steps in future to complete this ontology-based sys-tem.2 Related WorkOntology has been researched and developed for years. In audio applications, it is applied probably for the first time by Nakatani and Okuno [19]. They propose a sound ontology and its three usages: ontology-based integration (for sound stream segregation), interfacing between speech processing systems, and integration of bot-tom-up and top-down processing. Their sound stream segregation means generating an instance of a sound class and extracting its attributes from an input sound mixture. Khan and McLeod [13] utilize a domain-specific ontology for the generation of meta-data for audio and the selection of audio information in a query system. In this work, an audio ontology is defined by its identifier, start time, end time, description (a set of tags or labels) and the audio data. MPEG-7 Description Definition Language and a taxonomy of sound categories are employed by Casey [5] for sound recognitions. The audio content is described by qualitative descriptors (taxonomy of sound categories) and quantitative descriptors (set of features). Amatriain and Herrera [1] use semantic descriptors for sound ontology to transmit audio contents. Their description includes both low-level descriptors (e.g. fundamental frequency) and high-level descriptors (e.g. 'loud’). WordNet, an existing lexical network, is used by Cano et al. in [3] as a ontology-backbone of a sound effects management system. Ontology is applied to the disambiguation of the terms used to label a database in order to define concepts of sound effects, for example, the sound of a jaguar and the sound of a Jaguar car. A system of ontology-based sound retrieval is proposed by Hatala et al. in [9] to servemuseum visitors. Ontology is used to describe concepts and characteristics of sound objects as an interface between users and audio database. In [14], Kim et al. develop an MPEG-7-based audio classification and retrieval system targeted for analysis of film material. They test three structures for sound classification: an one-level, a hier-archical, and a hierarchical with hints. The classification is based on the Euclidean distance. The second structure gives the lowest recognition rate, while the third one gives the highest recognition rate. In most cases, ontology is used to describe an au-dio file (e.g. a sound effect) and to manage the database.Our work is to build a sound ontology applied to classifying an unknown audio sample detected in habitat. We will present in the next section ontologies for abstract concepts of audio scenes and for detecting situations of distress.3 Ontology-Based Sound ClassificationAn ontology-based sound classification includes three problems: defining ontologies, representing them, and applying them to classification. These problems will be pre-sented in this section.3.1 Sound OntologyFrom the classified sounds, we intend to extract the true meaning of the scene. For example, when a sound of a fallen chair and a sound of scream are detected, it should be interpreted as “the patient has tumbled down” (making the chair fall). Or when we detect a sound of groan, we can say that the patient is ill or hurt. This is a mapping between concrete sounds and abstract concepts. In other words, an abstract concept is defined by determined sounds. Sound ontology seems to be the most appropriate to our work.An ontology is a definition of a set of representational terms as defined by Gruber in [8]. In this paper, some situations are defined as ontologies. An ontology consists of concept, concept properties, relations and instances. The hierarchical relations between concepts in text or image applications can be established. But in our applica-tions, such relations between situation are not obvious. So we simply define concepts of situations based on concept properties and their facets. The ontology of the situa-tion of patient falling in house is depicted in Fig. 2 as an example. When the patient falls, he can make a chair or a table fall over, or can break something such as a glass. After the fall, the patient probably screams or groans due to pain or shock. Sounds are divided into two categories. Sounds of fallen chair, fallen table and broken glass are categorized in the “thing_distress” class, sounds of scream and groan are of “hu-man_distress”. So if a sound of “thing_distress” and/or a sound of “human_distress” are successively detected, we can probably say that the patient has fallen. Of course it can also be said that those sounds come from a chair tumbled down by a man and from another man being hurt. But if we suppose that normally the patient lives alone in the house then the “fall” interpretation is the most appropriate. Two other concepts of hurt and sick/ill are listed in Table 1. It is noted that water_in_toilet includes soundof water discharge, of water flowing from shower, water rushing or dripping fromfaucet, etc. In short, the concept of sound can be identified based on its attached con-cept properties and their respective facets. The usage of these concepts will be pre-sented in next paragraph.Fig. 2. An example of the ontology representing the “fall” concept. It has two properties andfive facetsTable 1. Properties and facets of three ontologies. “Hurt” concept has no thing_distress prop-erty. “Water_in_toilet” facet taken into account is due to the fact that when the patient issick/ill in the toilet, sound of water is often detectedThing_distress Human_distressFall Fallen_chair, Fallen_table, Broken_glassScream,GroanHurt Scream,GroanSick/ill Water_in_toilet Cough,Pant,Vomit3.2 Ontology-Based Sound ClassificationOntology-based classifications are mostly applied in text, image and biological appli-cations. The image classification system presented by Breen et al. in [2] uses a neural network to identify objects in a sports image. Category of the image is determined by a combination of detected image objects, each objects being assigned an experimental weight. Image ontology in this case is used to prune the selection of concepts: if the parent and the children are selected, the later will be discarded. In order to automati-cally classify web page, Prabowo et al in [23] apply a feed-forward neural network represent relationships in an ontology. The output of this network is used to estimate similarity between web pages. Mezaris et al. in [18] propose an object ontology for an object-based image retrieval system. In this ontology, each immediate-level descrip-tors is mapped to an appropriate range of values of the corresponding low-level arith-metic feature. Based on low-level features and query keywords, a support vector machine will result the final query output. In [21], Noh et al. classify web pages using an ontology. In this ontology, each class is predefined by a certain keyword and their relations. Classes of web pages are classified by extracting term frequency, document frequency and information gain, and by using several machine learning algorithms. Taghva et al. in [25] construct an email classification system in which an ontology is applied to extract useful feature, these feature are inputs of a Bayesian classifier. The text categorization system of Wu et al. in [27] also employ an ontology predefined by keywords and semantic relationship of word pair. These keywords are chosen by a term frequency / inverse document frequency classifier. The domain of a text is cate-gorized by a “score”. Maillot et al. in [17] introduce their ontology-based application for image retrieval. Each image object in an image is detected by a trained detectors. The relations of detected image object, established in ontology, will determine the category. In [26], Wallace et al. present their multimedia content indexing and re-trieval system. The ontology (basing on MPEG-7 description schemes) of the system includes semantic entities, semantic relations and a theraurus. A text-mining-based ontology enhancement and query-processing system is presented by Dey and Abu-laish [6]. Their key ideas are to learn and to include imprecise concept descriptions into ontology structures. Instead of using “very sweet” and “intensely sweet” in the wine ontology, they assign “sweet” for both wines, “very” for the first and “in-tensely” for the second. The degree of similarity between “very” and “intensely” is computed by a fuzzy reasoner. In [15], Laegreid et al. present a system for biological process classifications using Gene Ontology (GO, stored at Gene Ontology Home page, [7]). Their goal is to model the relationships between gene expression and a biological process, to learn and classify multiple biological process roles, and to use this model to predict the biological participation of unknown genes. Their method uses biological knowledge expressed by GO and then generates the model. In work of Robinson et al. [24], results of cluster analysis of gene expression microarray data is based on GO terms and associations.In ontology-based image applications, an image object is often defined by lower properties, such as form, color, viewing angle, background, etc. The method of defin-ing related lower properties in image applications is hard to apply to audio domain, because an audio object, such as a laugh, is hard to be defined.Text applications use relationships between text objects to classify. They are often known or predefined. For example, in a text application “net” can be interpreted as “a fishing tool” if words such as “fish”, “boat”, “river” are found in the same sentence of paragraph because they have obvious relation; or it can be a “group of connected computers” if there are “port”, “cable”, “TCP/IP”, “wi-fi” nearby.GO is usually applied as a basis of biological applications. It provides a structured and controlled vocabulary describing genes and gene products in organisms. This ontology consists of biological objectives, molecular functions and cellular compo-nents, organized into hierachies. To our knowledge, audio applications can take no advantage of this type of ontology.In our work methods used in text applications are considered because we hope to find the meaning of an audio scene by a group of sounds. The predefined classifying rules of Noh et al. is hard to apply to audio domain because so far we do not know a predefined rule for sounds. The keyword-based ontology of Wu et al. is also difficult to be used in our work because it needs relationship between sounds. The imprecise concept descriptions of Dey and Abulaish demand adjectives and adverbs. It cannot be applied to audio domain since audio signal has no equivalence. The method of using neural netwok to represent ontology of Prabowo et al. seems to be the most appropriate for us since it demands only two levels of concept and properties. There-fore in our work the relationship among concept, concept properties and facets is represented by a feed forward neural network described in [23]. The task of this net-work is to produce an output value that is used to estimate the similarity between the ontology and a new sound situation.Instance Instance InstanceFig. 3. The feed-forward neural network representing the “fall” ontology. Weights of links between layers depend on number of properties, number of facets and number of detected instancesThis model has three layers: input, hidden and output. The input layer of the net-work represents a set of instances. The hidden layer represents a set of concept prop-erties. The number of neurons in the hidden layer equals the number of concept prop-erties. The output layer (consists of one neuron) is the concept representatives. The two reasons to choose sigmoid transfer function,f(x) = 1/(1 + e-x) (1) for neurons, are as follows. Different concepts have different numbers of proper-ties, so their outputs are normalised form 0 to 1. And they vary continously. The neural network representing the “fall” concept is depicted in Fig. 3. In this example there are two hidden neurons and five input neurons. Two properties “thing_distress” and “human_distress” are represented by two hidden neurons. Input neurons are used to model the five instances of the concept. The two other neural network representing “hurt” and “sick/ill” have the same number of layers. “Hurt” has one hidden neuron and two input neurons, “sick/ill” has two hidden neurons and four input neurons.The weights of links between the output and hidden layer depend on the number of hidden neurons. The weights of links between a hidden neuron also depend on the number of its attached input neurons. Details of calculation of those weights can be found in [23]. If n instances are matched, the weight of each instance is the square root of n.During the classification phase, similarities between an input sample and each con-cepts are calculated in order to assign a concept to the sample. Classes of sounds are extracted. If an instance is found, its respective concept properties input is set to 1, otherwise it is zero. According to detected instances and their classes, weights of links of layers are determined. The output of each neural network therefore is a func-tion of weighted properties of the respective concept and is the similarity between the sample and the concept. The input sample will be assigned to the concept with which it has the highest similarity.4 Experimental EvaluationAn audio scene database of situations of distress is difficult to build. Firstly, such a database can be collected in hospitals, but recording this type of audio scenes in hos-pitals is nearly impossible due to the concerns of privacy. Secondly, recording them in a studio is feasible, but situations of distress are hard to be simulated by speakers, making a not true corpus. And finally in fact audio scenes are so numerous that it is hard to build a database (from a sound effect database) that can cover many situa-tions. Therefore we collect manually audio scenes from films. The scenes we target are the ones in which the character is in house and in a situation of distress: falling down, being hurt, sick or ill. From 150 films, 44 scenes of situations of distress with total duration of 680 seconds are collected.This classifier (illustrated in Fig. 4) should function automatically: detects sounds, estimates similarities between the sample and ontologies, and outputs the concept of the scene. But in this first stage of building an ontology-based classification system, the first steps are manually carried out. 44 audio scenes of situations of distress and50 of normal (non distress) situations are tested. Results of the ontology-based classi-fication are presented in Table 2.Fig. 4. The ontology-base classifier. Detected sounds are classified by the sound classifiers. Itsoutput is fed to the audio scene classifierThe misidentified scene of “fall” is the one in which the character falls down un-consciously making the door shut, and the unique sound we get is a shutting door. Itseems to need a redefinition for this concept. But if we add this type of sound into theontology, the system will identify actions of shutting doors as “fall”, and that willlead to wrong classification results. Therefore the fall ontology does not need to beredefined. There is one normal situation that is wrongly identified as a “hurt” one. Inthis situation the character cries when she is too happy. So arises the need of an algo-rithm (should be developed in the future) that is capable of discriminating betweenthe cries coming from happiness and those coming from hurt. Based on results ofclassification, it can be said that the ontologies are appropriate for audio scenes ofsituations of distress.Table 2. Results of ontology-based classification. Number of correctly identified is the numberof audio scenes of situations of distress which are correctly identified as its assigned concept inthe database. Number of wrongly identified is the number of audio scenes of normal situationswhich are identified as a situation of distressHurtSick/ill FallNumber of scene 20 19 5Number of correctly identified 19 19 5Number of wrongly identified 0 1 05 Conclusion and Future WorksWe present in this article an ontology-based audio scene classifier which can be usedto detect audio scenes of situations of distress. The construction of the ontology iswithin the framework of a telemedicine project. Three ontologies of sounds are de-fined. Concept, properties and instances of an ontology are modeled by a feed for-ward neural network. The output of a neural network presenting a concept is the simi-larity between it and the input sample. At first stages of developing the classifier, we defined three domain ontologies and tested them manually. These ontologies work well with our first audio database of situations of distress which is extracted from scenes of films.In the future a fully automatic ontology-based system needs to be built. In order to archieve this, the following tasks must be undertaken. First, more sound classes should be classified to cover a larger range of different types of sound. Second, sounds need to be separated from music because audio scenes collected from film are often mixed with music, making sound classifiers work inexactly. Third a combina-tion of ontologies and sound classifier should be built. Fourth more situations of dis-tress need to be defined. And finally, a bigger database should be acquired to obtain more complete domain ontologies. Besides the extension of this audio database, we also think of acquiring a text database from the Internet. This text database will con-sist of paragraphs that use types of sound in order to describe audio scenes in house. In short it is a text database of audio scenes. Audio object classes, context of the scene, or distribution of audio object can probably be extracted from this database. 6. AcknowledgementsThe authors gratefully acknowledge the receipt of a grant from the Flemish Interuni-versity Council for University Development cooperation (VLIR UOS) which enabled them to carry out this work.The authors also gratefully acknowledge the receipt of grants from French projects RESIDE-HIS and MAE/CORUS.References1. Amatriain, X., Herrera, P.: Transmitting Audio Contents as Sound Objects. Proceedings ofAES 22th International Conference on Virtual, Synthetic and Entertainment Audio, Espoo Finland (2002)2. Breen, C., Khan, L., Kumar, A., Wang, L.: Ontology-Based Image Classification UsingNeural Networks. Proc. SPIE (the International Society for Optical Engineering) Vol. 4862 (2002) 198-2083. Cano, P., Koppenberger, M., Celma, O., Herrera, P., Tarasov, V.: Sound Effects TaxonomyManagement in Production Environments. Proceedings of AES 25th International Confer-ence, London UK (2004)4. Carey, M.J., Parris, E.S., Lloyd-Thomas, H.: A Comparision of Features for Speech, MusicDiscrimination. Proceedings of the International Conference on Acoustics, Speech and Sig-nal Processing, Munich Germany (1997)5. Casey, M.: MPEG-7 Sound-Recognition Tools. IEEE Transaction on Circuits and Systemsfor Video Technology, Vol. 11, No. 6 (2001)6. Dey, L., Abulaish, M.: Ontology Enhancement for Including Newly Acquired Knowledgeabout Concept Descriptions and Answering Imprecise Queries. Web Semantics Ontology, Idea Group (2006) 189 - 2257. Gene Ontology Home: /8. Gruber, T.R.: A Translation Approach to Portable Ontology Specifications. KnowledgeAcquisition 5(2) (1993) 199-2209. Hatala, M., Kalantari, L., Wakkary, R., Newby, K, : Ontology and Rule Based Retrieval ofSound Objects in Augmented Audio Reality System for Museum Visitors. Proceedings of the 2004 ACM Symposium on Applied Computing, Nicosia Cyprus (2004) 1045 – 1050 10. Istrate, D., Vacher, M., Castelli, E., Sérignat, J.F.: Distress Situation Identifcation thoughSound Processing. An Application to Medical Telemonitoring. European Conference on Computational Biology, Paris (2003)11. Istrate, D., Vacher, M., Serignat, J.F., Besacier, J.F., Castelli, E.: Système de Télésurveil-lance Sonore pour la Détection de Situation de Détresse (Sound Telesurveillance System for Distress Situations Detection). ITBM-RBM Elsevier (2006)12. Istrate, D., Castelli, E., Vacher, M., Besacier, L., Serignat, J.F.: Sound Detection and Rec-ognition in Medical Telemonitoring. IEEE Transactions on Information Technology in Bio-medicine (to be published)13. Khan, L., McLeod, D.: Audio Structuring and Personalized Retrieval Using Ontologies.Proceedings of IEEE Advances in Digital Libraries, Library of Congress, Washington DC (2000) 116-12614. Kim, H.G., Moreau, N., Sikora, T.: Audio Classification Based on MPEG-7 Spectral BasisRepresentations. IEEE Transactions on Circuits and Systems for Video Technology, Vol.14, No. 5 (2004)15. Laegreid, A., Hvidsten, T.R., Midelfart, H., Komorowski, J., Sandvik, A.K.: PredictingGene Ontology Biological Process from Temporal Gene Expression Patterns. Genome Res13 (2003) 965-97916. Lu, L., Jiang, H., Zhang, H.J.: A Robust Audio Classification and Segmentation Method.ACM Multimedia (2001) 203-21117. Maillot, N., Thonnat, M., Hudelot, C.: Ontology Based Object Learning and Recognition:Application to Image Retrieval. In Proceedings of the 16th IEEE International Conference on Tools with Artificial Intelligence, Boca Raton FL USA (2004)18. Mezaris, V., Kompatsiaris, I., Strintzis, M.G.: An Ontology Approach to Object-BasedImage Retrieval. In proceedings of the IEEE International Conference on Image Processing (2003)19. Nakatani, T., Okuno, H.G.: Sound Ontology for Computational Auditory Scene Analysis.Proceedings of the fifteenth National Conference on Artificial Intelligence (AAAI-98), Vol.~1 (1998) 30-3520. Nguyen, C.P., Pham, N.Y., Castelli, E.: Toward a Sound Analysis System for Telemedi-cine. 2nd International Conference on Fuzzy Systems and Knowledge Discovery, Chansha China (2005)21. Noh, S., Seo, H., Choi, J., Choi, K., Jung, G.: Classifying Web Pages Using AdaptiveOntology. In Proceedings of the IEEE International Conference on Systems, Man and Cy-bernetics, Washington D.C. (2003) 2144-214922. Pfeiffer, S., Fischer, S., Effelsberg, W.: Automatic Audio Content Analysis. IEEE Multi-media, 3(3) (1996) 27-3623. Prabowo, R., Jackson, M., Burden, P., Knoell, H.D.: Ontology-Based Automatic Classifica-tion for the Web Pages: Design, Implimentation and Evaluation. The 3rd International Con-ference on Web Information Systems Engineering, Singapore (2002)。