and Ideas by Statistical Mechanics (ISM
固体物理英文教材

固体物理英文教材Solid State Physics TextbookThe field of solid state physics is a vast and complex area of study that encompasses the fundamental properties and behaviors of materials in their solid state. This branch of physics is crucial in understanding the behavior of materials at the atomic and molecular level, and has far-reaching applications in fields such as electronics, materials science, and nanotechnology.One of the primary goals of solid state physics is to develop a comprehensive understanding of the structure and properties of solids, including their electronic, magnetic, and thermal characteristics. This involves studying the arrangement and interactions of atoms within a solid material, as well as the various defects and impurities that can influence its behavior.A solid state physics textbook is an essential tool for students and researchers who are interested in this field. These textbooks typically cover a wide range of topics, including crystal structures, lattice vibrations, electronic band theory, semiconductor physics, superconductivity, and more. They provide a detailed and systematicapproach to the subject, guiding readers through the fundamental concepts and mathematical principles that underlie the behavior of solid materials.One of the key features of a solid state physics textbook is its emphasis on the experimental and theoretical foundations of the field. Textbooks often include detailed discussions of the various experimental techniques used to study solid materials, such as X-ray diffraction, neutron scattering, and scanning probe microscopy. They also delve into the mathematical and theoretical frameworks that have been developed to model and predict the behavior of solids, including quantum mechanics, statistical mechanics, and solid state theory.Another important aspect of a solid state physics textbook is its coverage of the diverse applications of solid state materials. Textbooks may explore the use of semiconductors in electronic devices, the role of magnetic materials in data storage and energy conversion, and the potential of superconductors in high-speed computing and power transmission. By highlighting these real-world applications, textbooks help students understand the practical relevance and importance of solid state physics.In addition to providing a comprehensive overview of the field, a solid state physics textbook should also be designed to be user-friendly and accessible to students. This may involve the use of clear and concise language, well-designed illustrations and diagrams, and a logical and organized structure that guides readers through the material in a step-by-step manner.One of the challenges in writing a solid state physics textbook is the need to strike a balance between depth and breadth. On the one hand, the textbook should cover the fundamental principles and concepts in sufficient detail to ensure a thorough understanding of the subject. On the other hand, it should also provide a broad overview of the field, touching on the diverse range of topics and applications that fall under the umbrella of solid state physics.To address this challenge, textbook authors often employ a variety of pedagogical strategies, such as the use of worked examples, problem sets, and case studies. These tools can help students apply the concepts they have learned and develop a deeper understanding of the material.Overall, a solid state physics textbook is an invaluable resource for anyone interested in the study of solid materials and their properties. Whether you are a student, a researcher, or a professional working in a related field, a well-written and comprehensive textbook can provide you with the knowledge and tools you need to explore the fascinating and ever-evolving world of solid state physics.。
纳斯别尔格公式英文

纳斯别尔格公式英文The Nasserberg Formula is a mathematical expression that has been widely used in various fields, particularly in the study of thermodynamics and statistical mechanics. This formula, named after the renowned physicist Gerhard Nasserberg, provides a relationship between the entropy of a system and its microstates. Understanding the Nasserberg Formula is crucial for comprehending the fundamental principles of thermodynamics and the behavior of complex systems.At its core, the Nasserberg Formula states that the entropy of a system is proportional to the natural logarithm of the number of accessible microstates. Mathematically, this can be expressed as S = k ln W, where S represents the entropy of the system, k is the Boltzmann constant, and W is the number of accessible microstates. This simple yet powerful equation has profound implications in our understanding of the physical world.One of the primary applications of the Nasserberg Formula is in the field of statistical mechanics, where it provides a bridge between themicroscopic behavior of individual particles and the macroscopic properties of a system. By considering the distribution of particles among the available microstates, we can derive important thermodynamic quantities such as temperature, pressure, and internal energy. This connection between the microscopic and macroscopic realms is a fundamental aspect of our understanding of the physical universe.Furthermore, the Nasserberg Formula has implications for our understanding of information theory and the nature of information itself. In this context, the entropy of a system can be interpreted as a measure of the uncertainty or information content of that system. This relationship between entropy and information has led to the development of fields such as information theory and communication theory, which have had a profound impact on our ability to store, transmit, and process information.One of the fascinating aspects of the Nasserberg Formula is its ability to describe the behavior of complex systems, such as those found in biology, ecology, and even social sciences. In these domains, the concept of entropy can be used to understand the organization and dynamics of complex systems, from the intricate networks of living organisms to the collective behavior of human societies. By analyzing the distribution of microstates in these systems, researchers can gain insights into their underlying principles and potentially predict theirfuture behavior.Moreover, the Nasserberg Formula has played a crucial role in the development of our understanding of the universe as a whole. In cosmology, the concept of entropy has been used to study the evolution of the universe, from the early stages of the Big Bang to the eventual fate of the cosmos. The Nasserberg Formula has provided a framework for understanding the arrow of time, the directionality of entropy, and the implications for the ultimate fate of the universe.In recent years, the Nasserberg Formula has also found applications in the realm of quantum mechanics and the study of quantum systems. In this context, the formula has been generalized to describe the entropy of quantum states, providing insights into the behavior of quantum systems and the nature of information at the quantum level.Despite its mathematical simplicity, the Nasserberg Formula has had far-reaching implications and has shaped our understanding of the physical world in profound ways. It continues to be a subject of active research and exploration, as scientists and researchers seek to push the boundaries of our knowledge and uncover the deeper mysteries of the universe.In conclusion, the Nasserberg Formula is a fundamental equation that has had a profound impact on our understanding of the physical world. From its applications in thermodynamics and statistical mechanics to its implications in information theory, cosmology, and quantum mechanics, this equation has proven to be a cornerstone of our scientific understanding. As we continue to explore the frontiers of knowledge, the Nasserberg Formula will undoubtedly continue to play a crucial role in shaping our understanding of the universe and the fundamental laws that govern it.。
英语短文科学家故事
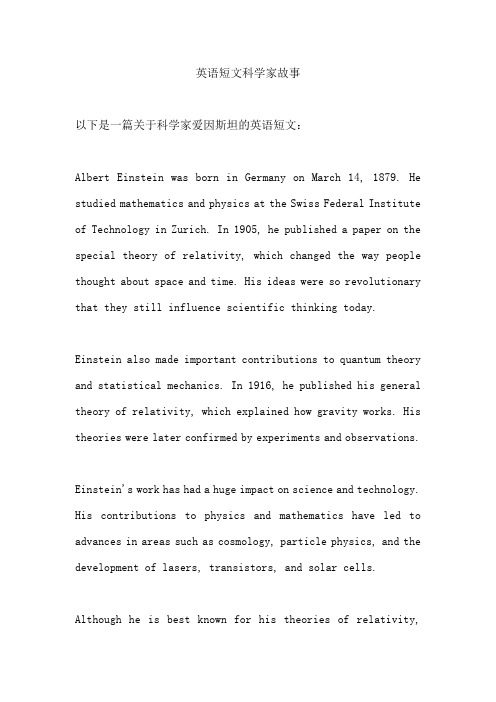
英语短文科学家故事以下是一篇关于科学家爱因斯坦的英语短文:Albert Einstein was born in Germany on March 14, 1879. He studied mathematics and physics at the Swiss Federal Institute of Technology in Zurich. In 1905, he published a paper on the special theory of relativity, which changed the way people thought about space and time. His ideas were so revolutionary that they still influence scientific thinking today.Einstein also made important contributions to quantum theory and statistical mechanics. In 1916, he published his general theory of relativity, which explained how gravity works. His theories were later confirmed by experiments and observations.Einstein's work has had a huge impact on science and technology. His contributions to physics and mathematics have led to advances in areas such as cosmology, particle physics, and the development of lasers, transistors, and solar cells.Although he is best known for his theories of relativity,Einstein's contributions to science went beyond physics. He also wrote about social issues, philosophy, and the importance of education. He believed that everyone should be able to understand science and that it should be used to make the world a better place.Einstein died on April 18, 1955, in Princeton, New Jersey. He was a brilliant scientist and a great thinker whose ideas continue to influence our understanding of the universe.。
Physica A Statistical Mechanics and its Applications

International Journal of Project Management , Volume 28, Issue 3,April 2010, Pages 285-295Paul Bowen, Peter Edwards, Keith Cattell, Ian JayShow preview | Related articles | Related reference work articlesPurchase85Dynamics of R&D networked relationships and mergers and acquisitions in the smart card field Original ResearchArticleResearch Policy , Volume 38, Issue 9, November 2009,Pages 1453-1467 Zouhaïer M’ChirguiClose preview | Related articles | Related reference work articlesAbstract | Figures/Tables | ReferencesAbstractThis paper analyzes how the structure and the evolution of inter-firmagreements have shaped the development of the smart card industry. The aimis to establish a closer connection between the evolution of inter-firmagreements in the smart card industry and the patterns of change of technologyand demand in this new high-tech industry. Based on a proprietary databasecovering both collaborative agreements and mergers and acquisitions (M&As)occurring in this industry over the period 1992–2006, we find that the evolutionof technology and market demand shapes the dynamics of R&D networks andPurchaseM&As are likely to change the industry structure. We also find that a small group of producers – first-movers – still control the industry and technological trajectories. Their position arises not for oligopolistic reasons of marketstructure, but for technological and organizational reasons.Article Outline1. Introduction2. Theoretical background3. The smart card industry: delineating the boundaries and identifying the actors3.1. Defining the smart card3.2. The differentiated market(s)3.3. The actors3.4. The smart card oligopoly: a dual market structure4. Research methods4.1. Methodology4.2. SCIFA database5. Trends in inter-firm agreements and emergence of networks in the smart cardindustry6. The structure of the network6.1. Network evolution6.2. Major players and centrality7. ConclusionAcknowledgementsReferences86The role of industrial maintenance in the maquiladoraindustry: An empirical analysis Original Research ArticleInternational Journal of Production Economics, Volume 114,Issue 1, July 2008, Pages 298-307Shad DowlatshahiClose preview | Related articles | Related reference work articlesPurchaseAbstract | Figures/Tables | ReferencesAbstractThis study explored the role of industrial maintenance in the maquiladora industry. The maquiladora industry is a manufacturing system that utilizes the Mexican workforce and foreign investment and technology on the border region between the United States and Mexico. The issues related to industrial maintenance were studied through a survey instrument and 11 in-depth and extensive field interviews with experts of eight maquiladora industries in El Paso, TX and Juarez, Mexico. Based on an 86% response rate (with 131 usable questionnaires) and four major survey questions, statistical analyses were performed. The survey questions included: collaboration between the maintenance and other functional areas, likely sources of maintenance problems (equipment, personnel, and management), major common losses of maintenance problems, and the role of ISO certification in maintenance. Finally, additional insights and assessment of the results were provided.Article Outline1. Introduction1.1. Review of literature2. Evolution of and various approaches to maintenance3. Historical, operational characteristics and the importance of the maquiladora industry4. Research design4.1. Data collection4.2. The interviews with maquiladora managers5. Analyses of results5.1. Statistical analysis for question 15.2. Statistical analysis for question 25.3. Statistical analysis for question 3 5.4. Statistical Analysis for question 46.Conclusions and assessmentReferences87 A variable P value rolling Grey forecasting model forTaiwan semiconductor industry production OriginalResearch ArticleTechnological Forecasting and Social Change, Volume 72,Issue 5, June 2005, Pages 623-640Shih-Chi Chang, Hsien-Che Lai, Hsiao-Cheng YuClose preview | Related articles |Related reference work articlesAbstract | Figures/Tables | ReferencesAbstractThe semiconductor industry plays an important role in Taiwan's economy. In thispaper, we constructed a rolling Grey forecasting model (RGM) to predictTaiwan's annual semiconductor production. The univariate Grey forecastingmodel (GM) makes forecast of a time series of data without considering possible correlation with any leading indicators. Interestingly, within the RGM there is aconstant, P value, which was customarily set to 0.5. We hypothesized thatmaking the P value a variable of time could generate more accurate forecasts. Itwas expected that the annual semiconductor production in Taiwan should beclosely tied with U.S. demand. Hence, we let the P value be determined by theyearly percent change in real gross domestic product (GDP) by U.S.manufacturing industry. This variable P value RGM generated better forecaststhan the fixed P value RGM. Nevertheless, the yearly percent change in realGDP by U.S. manufacturing industry is reported after a year ends. It cannotserve as a leading indicator for the same year's U.S. demand. We found out thatthe correlation between the yearly survey of anticipated industrial productiongrowth rates in Taiwan and the yearly percent changes in real GDP by U.S. manufacturing industry has a correlation coefficient of 0.96. Therefore, we usedPurchasethe former to determine the P value in the RGM, which generated very accurate forecasts. Article Outline1.Introduction2. The semiconductor industry in Taiwan3. Rolling GM (1,1)4. Forecast Taiwan semiconductor production with RGM (1,1)5. Forecast Taiwan semiconductor production with variable P value RGM (1,1)6. ConclusionsAppendix A. AppendixA.1. 1998 Production forecast for the semiconductor industry under different PvaluesA.2. 1999 Production forecast for the semiconductor industry under different PvaluesA.3. 2000 Production forecast for the semiconductor industry under different PvaluesA.4. 2001 Production forecast for the semiconductor industry under different PvaluesA.5. 2002 Production forecast for the semiconductor industry under different PvaluesReferencesVitae88 Energy demand estimation of South Korea using artificial neural network Original Research ArticleEnergy Policy , Volume 37, Issue 10, October 2009, Pages4049-4054Zong Woo Geem, William E. Roper Close preview | Related articles | Related reference work articlesAbstract | Figures/Tables | ReferencesPurchaseAbstractBecause South Korea's industries depend heavily on imported energy sources (fifth largest importer of oil and second largest importer of liquefied natural gas in the world), the accurate estimating of its energy demand is critical in energy policy-making. This research proposes an artificial neural network model (a structure with feed-forward multilayer perceptron, error back-propagation algorithm, momentum process, and scaled data) to efficiently estimate the energy demand for South Korea. The model has four independent variables, such as gross domestic product (GDP), population, import, and export amounts. The data are obtained from diverse local and international sources. The proposed model better estimated energy demand than a linear regression model (a structure with multiple linear variables and least square method) or an exponential model (a structure with mixed integer variables, branch and bound method, and Broyden–Fletcher–Goldfarb–Shanno (BFGS) method) in terms of root mean squared error (RMSE). The model also forecasted better than the other two models in terms of RMSE without any over-fitting problem. Further testing with four scenarios based upon reliable source data showed unanticipated results. Instead of growing permanently, the energy demands peaked at certain points, and then decreased gradually. This trend is quite different from the results by regression or exponential model.Article Outline1. Introduction2. Artificial neural network model3. Case study of South Korea4. Results of linear regression model5. Results of exponential model6. Results of ANN model7. Validation of the ANN model8.Future estimation with different scenarios9. ConclusionsReferences89 Catching up through developing innovation capability: evidence from China's telecom-equipmentindustry Original Research ArticleTechnovation , Volume 26, Issue 3, March 2006,Pages359-368 Peilei FanShow preview | Related articles | Related reference work articlesPurchase90 Optimization of material and production to develop fluoroelastomer inflatable seals for sodium cooled fastbreeder reactor Original Research ArticleNuclear Engineering and Design , In Press, Corrected Proof, Available online 16 February 2011N.K. Sinha, Baldev RajShow preview | Related articles | Related reference work articlesPurchase Research highlights► Production of thin fluoroelastomer profiles by cold feed extrusion and continuous cure involving microwave and hot air heating. ► Use of peroxide curing in air during production . ► Use offluoroelastomers based on advanced polymer architecture (APA) for the production of profiles. ► Use of the profiles in inflatable seals for critical application of Prototype Fast Breeder Reactor. ► Tailoring of material formulation by synchronized optimization of material and production technologies to ensure that the produced seal ensures significant gains in terms of performance and safety in reactor under synergistic influences of temperature, radiation, air and sodium aerosol.91 The dynamic transfer batch-size decision for thin film transistor –liquid crystal display array manufacturing by artificialneural-network Original Research ArticleComputers & Industrial。
统计力学 Statistical Mechanics
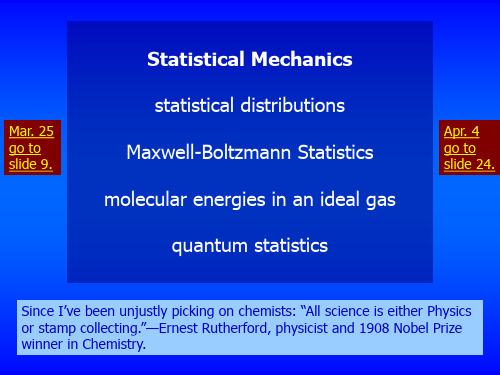
• It doesn’t break the logical chain because we find we can’t explain hydrogen without invoking wave properties.
*The most valuable kid in school that day.
What does this have to do with statistical mechanics? Physics faculty tend to think of thermodynamics (including statistical mechanics) as the stewed spinach of college physics courses. The wacko faculty member who actually likes teaching that stuff is a treasured friend whenever it comes time to give out teaching assignments.
The nuns made us ―clean our plate‖ at lunch. It had something to do with the starving children in China. They would inspect our trays as we passed through the ―dump the trash‖ line. What to do on spinach day?
专业英语(土木工程 路桥方向)李嘉 第三版 翻译
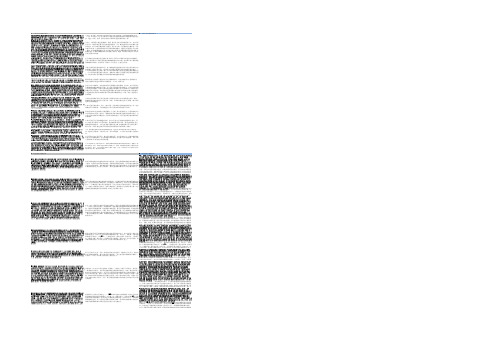
1、土木工程中的各种业务1、土木工程中的各种业务Engineering is a prof ession, which means that an engineer must have a specialized university education. Many government jurisdictions also have licensing procedures which require engineering graduates to pass an examination, similar to the examination f or a lawyer, bef ore they can actively start on their careers.工程是一种专业,这就是说工程师必须受过专业大学教育。
许多政府管辖部门还有(一套)认证程序,这一程序要求工科毕业生在他们能积极地开始他们的职业生涯之前,通过(认证)考试, 这种考试类似于律师职业里的律师考试一样。
In the university, mathematics, physics, and chemistry are heavily emphasized throughout theengineering curriculum, but particularly in the f irst two or three years. Mathematic is very important in all branches of engineering, so it is greatly stressed. Today, mathematics includes courses in statistics, which deals with gathering, classif ying, and using numerical data, or pieces of inf ormation. An important aspect of statistical mathematics is probability, which deals with what may happen when there are dif f erent f actors, or variables, that can change the results of a problem. Bef ore the construction of a bridge is undertaken, f or example, a statistical study is made of the amount of traf f ic the bridge will be expected to handle. In the design of the bridge, variable such as water pressure on the f oundation, impact, the ef f ects of dif f erent wind f orces, and many other f actors must be considered.大学里,工科课程中着重强调数学、物理,和化学,尤其在开始的两到三年。
大学专业英语

大学专业英语化学类翻译命名:H: hydrogen C:carbon N: nitrogen O: oxygen Na: sodium Mg: magnesium S: Sulphur Cl: chlorine K: Potassium Ca: Calcium Fe:iron Cu:copper Zn:zink Ag:silver命名按示例,元素,结构名称灵活更换,不能一一列出。
CaSO4: Calcium sulphate NaSO4:sodium sulphate Na2SO3: sodium sulphite NaNO2:Sodium nitrite Fe(NO3)3 :iron(III) nitrate NaClO:Sodium Hypochlorite KMnO4:Potassium permanganate NaCO3:sodium无机含氧酸命名:H2SO4:sulphuric acid H2SO3:sulphurous acid HNO3: nitric acid HNO2:nitrous acid H2CO3:Carbonic acid无氧酸:HCl: hydrochloric acid H2S:hydrosulphuric acidH2S:hydrosulphide carbonate HCl:hydrogen chloride氧化物命名:CO:carbon oxide CO2:Carbon dioxide Cu2O copper (I) oxide CuO copper(II) oxide碱的命名:金属名称+ hydroxide如:NaOH:Sodium hydroxideFe(OH)3 :iron(III) hydroxide Fe(OH)2: iron(II) hydroxideMg(OH)2: magnesium hydroxideCu(OH)2:Copper(II) hydroxide有机物命名:希腊数字1-10:mono- di- tri- but(a) pent(a) hex(a) hept(a) oct(a) non(a) dec(a)拉丁数字1-10:meth- eth- prop- but(a) pent(a) hex(a) hept(a) oct(a) non(a) dec(a) 烷:ane 烯:ene 炔:yne例甲烷:methane 丙烷:propane 环丙烷:cyclopropane 环丁烷: cyclobutane 环戊烷: cyclopentane 环己烷:cyclohexane 乙烯:ethene丙炔:propyne 甲醇:methaol 乙醇:ethaol 甲酸:methoic acid 乙酸:ethoic acid仪器器材命名:1. 烧杯beaker2. 量筒measuring cylinder3. 滴定管burette4. 移液管transfer pipette5. 刻度吸量管graduated pipette6. 布氏漏斗Büchner funnel7. 滴液漏斗dropping filter8. 圆底烧瓶round bottom flask 10. 干燥塔drying tower 11.塞子stopper 12. 坩埚钳crucile tong 13.ring stand 铁架台14. 托盘天平tray balance 15. 煤气灯Bunsen burner 16. 通风柜fume hoods物理化学四部分:thermodynamics 热力学quantum chemistry量子化学statistical mechanics 统计力学kinetics动力学反应名称:substitution取代nucleophilic亲核nuleophile 亲核试剂electrophilic亲电addition reaction加成反应elimination action消去反应rearrangement action 重排反应electrophilic addition reaction亲电加成反应nucleophilic substitution 亲核取代分解:decomposition置换:displacement复分解:partner exchange沉淀反应:precipitation reaction汉译英或者填空,选择:1. We often hear about toxic chemical or chemical pollution without hearing about the absolutely central role that chemistry play in human well being.有毒化学物质…..2. Chemistry is the science that tries to understand the properties of substance and the changes that substances undergo.化学试图了解物质的性质和物质进行的变化的科学。
物理经典书籍教材推介

物理经典书籍教材推介个人以为国内好的物理教材不太多,很多教材内容严谨可是易读性不强。
因此那个书单特意精选了一些适宜自学且架构严谨的国外大学热点教材分享~~最重要的是,这套教材组成一个完整的物理教材体系,都是教得专门深切浅出的专著,专门适合自学提高。
学物理是一件宝贵的乐事,什么缘故不学得乐在其中呢?这些教材在保留了趣味的情形下不失学术水平,因此专门推荐。
以下是依照学习推荐进度排序的《费曼物理学讲义》卷一、卷二、卷3及《习题解答》诺奖大师费曼乐趣横生的经典之作,读起来津津有趣,没有大段令人畏惧的公式和推导,几乎全文都在说明物理思想和有趣的现象,关于明白得物理思想的本质有极大帮忙。
《Mathematical methods for Physics and engineering》by Riley涵盖了几乎所有物理研究需要的数学知识,没有过度苛求证明严格性且说明形象有趣读起来超级有成绩感,不像国内的数理方式写得过于太抽象,用户界面不太友好呵呵。
《All the Mathematics you missed but need to know》by Garrity轻松的读物,高屋建瓴地整合了之前学过的数学知识,使读者很容易看透其中的数学本质。
举重假设轻地谈了很多深刻的数学领域,例如拓扑和“形式(form)”。
能够给大三看,也能够给研一看,必然会有专门大收成。
《Classical Mechanics- Systems of Particles and Hamiltonian Dynamics》by Greiner清楚地讲述了理论力学的内容,尽管书厚,但说明清楚没有冗余,超级适合自学。
《Introduction to Electrodynamics》及《习题解答》by Griffiths深切浅出的一本书,把人轻松地从电磁学带进电动力学的世界。
《Classical Electrodynamics》及《习题解答》by JacksonJackson那个词几乎已经专指这本神作了,难度高但循序渐进不失自学性。
- 1、下载文档前请自行甄别文档内容的完整性,平台不提供额外的编辑、内容补充、找答案等附加服务。
- 2、"仅部分预览"的文档,不可在线预览部分如存在完整性等问题,可反馈申请退款(可完整预览的文档不适用该条件!)。
- 3、如文档侵犯您的权益,请联系客服反馈,我们会尽快为您处理(人工客服工作时间:9:00-18:30)。
Ideas by Statistical Mechanics (ISM)†Lester IngberLester Ingber Research (LIR)<ingber@> <ingber@> []AbstractIdeas by Statistical Mechanics (ISM) is a generic program to model evolution and propagation of ideas/patterns throughout populations subjected to endogenous and exogenous interactions.The program is based on the author’s work in Statistical Mechanics of Neocortical Interactions (SMNI), and uses the author’s Adaptive Simulated Annealing (ASA) code for optimizations of training sets, as well as for importance-sampling to apply the author’s copula financial risk-management codes, Trading in Risk Dimensions (TRD), for assessments of risk and uncertainty.This product can be used for decision support for projects ranging from diplomatic, information, military,and economic (DIME) factors of propagation/evolution of ideas, to commercial sales, trading indicators across sectors of financial markets, advertising and political campaigns, etc.It seems appropriate to base an approach for propagation of ideas on the only system so far demonstrated to develop and nurture ideas, i.e., the neocortical brain.A statistical mechanical model of neocortical interactions, developed by the author and tested successfully in describing short-term memory and EEG indicators, is the proposed model.ISM develops subsets of macrocolumnar activity of multivariate stochastic descriptions of defined populations, with macrocolumns defined by their local parameters within specific regions and with parameterized endogenous inter-regional and exogenous external connectivities. Parameters of subsets of macrocolumns will be fit using ASA to patterns representing ideas. Parameters of external and inter-regional interactions will be determined that promote or inhibit the spread of these ideas.Tools of financial risk management, developed by the author to process correlated multivariate systems with differing non-Gaussian distributions using modern copula analysis, importance-sampled using ASA, will enable bona fide correlations and uncertainties of success and failure to be calculated. Marginal distributions will be evolved to determine their expected duration and stability using algorithms developed by the author,i.e., PATHTREE and PATHINT codes.Keywords:statistical mechanics, neocortical interactions, simulated annealing, risk management†L. Ingber,“Ideas by statistical mechanics (ISM),”Report 2006:ISM, Lester Ingber Research, Ashland,OR, 2006.URL /smni06_ism.pdf. Early drafts can be downloaded from/pdf/cs.CE/0607103 and /abstract=918860.$Id: smni06_ism,v 1.28 2007/12/20 21:09:35 ingber Exp ingber $ContentsAbstract (1)Contents (2)1. Significance of Problem (4)1.1. Bottom-Up versus Top-Down (4)1.2. Cost Functions for Ideas (4)1.3. Inclusion of non-Gaussian Correlated Systems (5)1.4. Other Alternatives. . . . . . . . . . . . . . . . . . . . . . .52. Technical Objectives. . . . . . . . . . . . . . . . . . . . . . . . .52.1. Architecture for Selected Model (5)3. Work Plan (5)3.1. Application of SMNI Model (5)3.2. Application of TRD Analysis (6)3.3. Example of Use of ISM (6)4. Related Work (7)4.1. Statistical Mechanics of Neocortical Interactions (SMNI) (7)4.1.1. Application to ISM Project (7)4.1.2. SMNI Tests on STM and EEG (7)4.1.3. SMNI Description of STM (7)4.1.4. SMNI Description of EEG (8)4.1.5. Generic Mesoscopic Neural Networks (9)4.1.6. On Chaos in Neocortex. . . . . . . . . . . . . . . . . . .94.1.7. Mathematical Development (9)4.1.7.1. Inclusion of Macroscopic Circuitry (10)4.1.8. Portfolio of Physiological Indicators (PPI) (10)4.1.8.1. Multiple Imaging Data (10)4.1.8.2. Local Versus Global Influences (11)4.1.8.3. Binocular-Rivalry Example (11)4.1.8.4. Application to ISM (11)4.2. Computational Physics (11)4.2.1. Application to ISM Project (11)4.2.2. Adaptive Simulated Annealing (ASA) (11)4.2.3. PATHINT and PATHTREE (12)4.2.3.1. Real Options for Project Schedules (ROPS) (12)4.3. Statistical Mechanics of Combat (SMC) (12)4.3.1. Application to ISM Project (12)4.3.2. Janus Project (12)4.3.3. Portfolio of Combat Indicators (PCI) (13)4.3.3.1. Application to ISM (13)4.4. Trading in Risk Dimensions (TRD) (13)4.4.1. Statistical mechanics of Financial Markets (SMFM) (13)4.4.2. Application to ISM Project (13)4.4.2.1. Standard Code For All Platforms (14)4.4.2.2. Gaussian Copula (14)4.4.3. Exponential Marginal Distribution Models (14)4.4.4. Copula Transformation (15)4.4.4.1. Transformation to Gaussian Marginal Distributions (15)4.4.4.2. Including Correlations (15)4.4.4.2.1. Stable Covariance Matrices (16)4.4.4.3. Copula of Multivariate Correlated Distribution (16)4.4.5. Portfolio Distribution (17)4.4.5.1. Recursive Risk-Management in Trading Systems (17)4.4.6. Risk Management (17)4.4.7. Sampling Multivariate Normal Distribution for Events (18)4.4.7.1. Transformation to Independent Variables (18)4.4.8. Numerical Development of Portfolio Returns (18)4.4.8.1.ΧFrom Sampled Events Into Bins (18)4.4.9. Multiple Trading Systems (19)4.4.10. Value-Weighting (19)4.4.11. Post-Processing of Multiple Optima/“Mutations” (19)5. Future Research (20)5.1. Anticipated Results (20)5.2. Significance of Initial Efforts (20)6. Commercialization (20)REFERENCES (20)1. Significance of ProblemA briefing [2]demonstrates the breadth and depth complexity required to address real diplomatic, information, military,economic (DIME) factors for the propagation/evolution of ideas through defined populations. An open mind would conclude that it is possible that multiple approaches may be required for multiple decision makers in multiple scenarios.However, it is in the interests of multiple decision-makers to as much as possible rely on the same generic model for actual computations.Many users would have to trust that the coded model is faithful to process their inputs.Similar to DIME scenarios, sophisticated competitive marketing requires assessments of responses of populations to new products.Many large financial institutions are now trading at speeds barely limited by the speed of light.They co-locate their servers close to exchange floors to be able to turn quotes into orders to be executed within msecs. Clearly,trading at these speeds require automated algorithms for processing and making decisions. These algorithms are based on “technical” information derived from price, volume and quote (Level II) information.The next big hurdle to automated trading is to turn “fundamental” information into technical indicators, e.g., to include new political and economic news into such algorithms.Ideas by Statistical Mechanics (ISM) can be developed to address these issues.[ism (noun): A belief (or system of beliefs) accepted as authoritative by some group or school.A doctrine or theory; especially,a wild or visionary theory.A distinctive doctrine, theory,system, or practice.]1.1. Bottom-Up versus Top-DownThe concept of “memes” is an example of an approach to deal with DIME factors [72].The meme approach, using a reductionist philosophy of evolution among genes, is reasonably contrasted to approaches emphasizing the need to include relatively global influences of evolution [73].A best selling book on propagation of ideas documents many contexts where the spreading of information often follows a diffusion process [9].It seems appropriate to base an approach for propagation of ideas on the only system so far demonstrated to develop and nurture ideas, i.e., the neocortical brain.In the present context, the author’s approach, using guidance from his statistical mechanics of human neocortical interactions (SMNI), developed in a series of about 30 published papers from 1981-2001[14-16,20,29,33,35,41,43,44,46], also addresses long-standing issues of information measured by electroencephalography(EEG) as arising from bottom-up local interactions of clusters of thousands to tens of thousands of neurons interacting via short-ranged fibers), or top-down influences of global interactions (mediated by long-ranged myelinated fibers). SMNI does this by including both local and global interactions as being necessary to develop neocortical circuitry.1.2. Cost Functions for IdeasComputational approaches developed to process different approaches to modeling phenomena must not be confused with the models of these phenomena.For example, the meme approach lends it self well to a computational scheme in the spirit of genetic algorithms (GA).The cost/objective function that describes the phenomena of course could be processed by any other sampling technique such as simulated annealing (SA).One comparison[60] demonstrated the superiority of SA over GA on cost/objective functions used in a GA database.That study used Very Fast Simulated Annealing (VFSR), created by the author for military simulation studies[26], which has evolved into Adaptive Simulated Annealing (ASA) [30].However, it is the author’s experience that the Art and Science of sampling complex systems requires tuning expertise of the researcher as well as good codes, and GA or SA likely would do as well on cost functions for this study.A very important issue is for this project is to develop cost functions for this study,not only how to fit or process them.1.3. Inclusion of non-Gaussian Correlated SystemsThis approach includes application of methods of portfolio risk analysis to such statistical systems.There are often two kinds of errors committed in multivariate risk analyses: (E1) Although the distributions of variables being considered are not Gaussian (or not tested to see how close they are to Gaussian), standard statistical calculations appropriate only to Gaussian distributions are employed. (E2)Either correlations among the variables are ignored, or the mistakes committed in (E1) — incorrectly assuming variables are Gaussian — are compounded by calculating correlations as if all variables were Gaussian.The harm in committing errors E1 and E2 can be fatal — fatal to the analysis and/or fatal to people acting in good faith on the basis of these risk assessments.Risk is measured by tails of distributions. So,if the tails of some variables are much fatter or thinner than Gaussian, the risk in committing E1 can be quite terrible. Many times systems are pushed to and past desired levels of risk when several variables become highly correlated, leading to extreme dependence of the full system on the sensitivity of these variables. It is very important not to commit E2 errors.The Trading in Risk Dimensions (TRD) project addresses these issues in the context of financial risk management, but the tools and codes are generic.1.4. Other AlternativesThere are multiple other alternative works being conducted world-wide that must be at least kept in mind while developing and testing models of evolution/propagation of ideas in defined populations: A study on a(too) simple algebraic model of opinion formation concluded that the only final opinions are extremal ones [1].A study of the influence on chaos on opinion formation, using a simple algebraic model, concluded that contrarian opinion could persist and be crucial in close elections, albeit the authors were careful to note that most real populations probably do not support chaos[5]. A limited review of work in social networks illustrates that there are about as many phenomena to be explored as there are disciplines ready to apply their network models [71].2. Technical Objectives2.1. Architecture for Selected ModelThe primary objective is to deliver a computer model that contains the following features: (1) A multivariable space will be defined to accommodate populations.(2) A cost function over the population variables in (1) will be defined to explicitly define a pattern that can be identified as an Idea.(3) Subsets of the population will be used to fit parameters — e.g, coefficients of variables, connectivities to patterns, etc. — to an Idea, using the cost function in (2).(4) Connectivity of the population in (3) will be made to the rest of the population.Investigations will be made to determine what endogenous connectivity is required to stop or promote the propagation of the Idea into other regions of the population.(5) External forces, e.g., acting only on specific regions of the population, will be introduced, to determine how these exogenous forces may stop or promote the propagation of an Idea.3. Work Plan3.1. Application of SMNI ModelA statistical mechanical model of neocortical interactions, developed by the author and tested successfully in describing short-term memory (STM) and in training and testing EEG indicators, is the model used here to address DIME factors for the propagation/evolution of Ideas through defined populations.The approach here is to develop subsets of Ideas/macrocolumnar activity of multivariate stochastic descriptions of defined populations (of a reasonable but small population samples, e.g., of 100−1000), with macrocolumns defined by their local parameters within specific regions (larger samples of populations) and with parameterized long-ranged inter-regional and external connectivities. Parameters of a given subset of macrocolumns will be fit using ASA to patterns representing Ideas, akin to acquiring hard-wired long-term memory (LTM) patterns.Parameters of external and inter-regional interactions will be determined that promote or inhibit the spread of these Ideas, by determining the degree of fits andoverlaps of probability distributions relative to the seeded macrocolumns.That is, the same Ideas/patterns may be represented in other than the seeded macrocolumns by local confluence of macrocolumnar and long-ranged firings, akin to STM, or by different hard-wired parameter LTM sets that can support the same local firings in other regions (possible in nonlinear systems).SMNI also calculates how STM can be dynamically encoded into LTM [15].Small populations in regions will be sampled to determine if the propagated Idea(s) exists in its pattern space where it did exist prior to its interactions with the seeded population.SMNI derives nonlinear functions as arguments of probability distributions, leading to multiple STM, e.g.,7±2for auditory memory capacity.Some investigation will be made into nonlinear functional forms other than those derived for SMNI, e.g., to have capacities of tens or hundreds of patterns for ISM.3.2. Application of TRD AnalysisTools of financial risk management, developed by the author to process correlated multivariate systems with differing non-Gaussian distributions using modern copula analysis, importance-sampled using ASA, will enable bona fide correlations and uncertainties of success and failure to be calculated[52]. Marginal distributions will be evolved to determine their expected duration and stability using algorithms developed by the author,i.e., PATHTREE [55] and PATHINT [45] codes.3.3. Example of Use of ISMFor an example of problems that can be approached by ISM, for specificity consider some data that might be drawn from opinions, or internet sampling, etc., and mapped onto several variables. Say that the mapped/projected data has a range that is dense enough (e.g., 10 or more divisions) so that it makes sense to build histograms -- which are essentially marginal probability distributions for each variable. If the system is such that it is changing in time, e.g., due to volatility of the system or changing trends/contexts, then this really is a multivariate stochastic process.For a large class of distributions, the time development can be used to better fit the models, e.g., using PATHINT/PATHTREE code to evolve the model and fit the model over a time domain as well.ASA can fit the data to some forms of reasonable distributions. TRD copula codes can transform these distributions (which likely are not Gaussian) into a space where it makes sense to develop correlations and covariances -- the point of using modern copula analysis for risk management, etc.In most systems it is important to properly treat correlations.Now, consider introducing a product or an idea into a city or two within one of two countries. There can be “long-ranged” interactions/influences between cities within one country and cities within the other country.Within a country,there are relatively “short-ranged” (different from long-ranged, e.g., due to cultural distances, etc.) interactions.The problem posed is how to(a) model this total system composed of two(or more) countries with their inner cities, (b) how to promote or retard the development of certain products or ideas, e.g., how to seed the process to enhance a desired goal.This is very much like the real brain modeled by SMNI in detail to fit EEG data across regions of neocortex(where here different neocortical regions are like different countries, e.g., like auditory,visual, somatic, associative,frontal cortical regions), and the within each region there are macrocolumnar interactions (like within cities).Somewhat more specifically,the distributions likely have multiple states to support several to many ideas or themes, akin to states of short-term memory (STM).These can be maintained by stochastic activity of the underlying model which was not not set prior to hold such states.These states might even be competing preferences or ideas.After time, the parameters of the model may be modified/adapted to store these activity patterns more solidly into the parameters of the system, e.g., into a long-term memory (LTM) storage, which likely would be harder to modify with the long-ranged interactions.The long-ranged interactions, which can exist endogenously in the total system, probably can be more easily modified (than the short-ranged interactions) by introduction of exogenous activity,permitting some kind of control over the system.The use of multivariate stochastic processes at just these few lev e ls, albeit requiring relatively sophisticated computational algebra and numerical algorithms, suffice to explain a lot of brain processes,and this also would suffice to explain some useful tactics and strategies for a lot of businesses.4. Related Work4.1. Statistical Mechanics of Neocortical Interactions (SMNI)4.1.1. Application to ISM ProjectNeocortex has evolved to use minicolumns of neurons interacting via short-ranged interactions in macrocolumns, and interacting via long-ranged interactions across regions of macrocolumns.This common architecture processes patterns of information within and among different regions of sensory, motor,associative cortex, etc.Therefore, the premise of this approach is that this is a good model to describe and analyze evolution/propagation of Ideas among defined populations.Relevant to this study is that a spatial-temporal lattice-field short-time conditional multiplicative-noise (nonlinear in drifts and diffusions) multivariate Gaussian-Markovian probability distribution is developed faithful to neocortical function/physiology.Such probability distributions are a basic input into the approach used here.The SMNI model was the first physical application of a nonlinear multivariate calculus developed by other mathematical physicists in the late 1970’s to define a statistical mechanics of multivariate nonlinear nonequilibrium systems [10,65].4.1.2. SMNI Tests on STM and EEGThe author has developed a statistical mechanics of neocortical interactions (SMNI) for human neocortex, building from synaptic interactions to minicolumnar,macrocolumnar,and regional interactions in neocortex. Since1981, a series of papers on the statistical mechanics of neocortical interactions (SMNI) has been developed to model columns and regions of neocortex, spanning mm to cm of tissue.Most of these papers have dealt explicitly with calculating properties of STM and scalp EEG in order to test the basic formulation of this approach [13-16,19,20,22,28,29,33,35,36,38,39,41,43,58,59].The SMNI modeling of local mesocolumnar interactions (convergence and divergence between minicolumnar and macrocolumnar interactions) was tested on STM phenomena.The SMNI modeling of macrocolumnar interactions across regions was tested on EEG phenomena.4.1.3. SMNI Description of STMSMNI studies have detailed that maximal numbers of attractors lie within the physical firing space of M G, where G={Excitatory,Inhibitory}minicolumnar firings, consistent with experimentally observed capacities of auditory and visual STM, when a “centering” mechanism is enforced by shifting background noise in synaptic interactions, consistent with experimental observations under conditions of selective attention [16,20,33,59,69].This leads to all attractors of the short-time distribution lying along a diagonal line in M G space, effectively defining a narrow parabolic trough containing these most likely firing states. This essentially collapses the 2 dimensional M G space down to a one-dimensional space of most importance. Thus,the predominant physics of STM and of (short-fiber contribution to) EEG phenomena takes place in a narrow“parabolic trough” in M G space, roughly along a diagonal line [16].These calculations were further supported by high-resolution evolution of the short-time conditional-probability propagator using PATHINT [59].SMNI correctly calculated the stability and duration of STM, the primacy versus recency rule, random access to memories within tenths of a second as observed, and the observed 7±2capacity rule of auditory memory and the observed 4±2capacity rule of visual memory.SMNI also calculates how STM patterns (e.g., from a given region or even aggregated from multiple regions) may be encoded by dynamic modification of synaptic parameters (within experimentally observed ranges) into long-term memory patterns (LTM) [15].Figure 1.Illustrated are three biophysical scales of neocortical interactions: (a)-(a*)-(a’)microscopic neurons; (b)-(b’) mesocolumnar domains; (c)-(c’) macroscopic regions. SMNIhas developed appropriate conditional probability distributions at each level, aggregating upfrom the smallest levels of interactions.In (a*)synaptic inter-neuronal interactions, averagedover by mesocolumns, are phenomenologically described by the mean and variance of adistributionΨ.Similarly,in(a) intraneuronal transmissions are phenomenologicallydescribed by the mean and variance ofΓ.Mesocolumnar averaged excitatory (E)andinhibitory (I)neuronal firings M are represented in (a’).In (b) the vertical organization ofminicolumns is sketched together with their horizontal stratification, yielding a physiologicalentity,the mesocolumn.In (b’) the overlap of interacting mesocolumns at locations r and r′from times t and t+τis sketched. In(c) macroscopic regions of neocortex are depicted asarising from many mesocolumnar domains.(c’) sketches how regions may be coupled bylong−ranged interactions.4.1.4. SMNI Description of EEGUsing the power of this formal structure, sets of EEG and evoked potential data from a separate NIH study,collected to investigate genetic predispositions to alcoholism, were fitted to an SMNI model on a lattice of regional electrodes to extract brain “signatures” of STM[41,43]. Each electrode site was represented by an SMNI distribution of independent stochastic macrocolumnar-scaled M G variables, interconnected by long-ranged circuitry with delays appropriate to long-fiber communication in neocortex. The global optimization algorithm ASA was used to perform maximum likelihood fits of Lagrangians defined by path integrals of multivariate conditional probabilities.Canonical momenta indicators (CMI) were thereby derived for individual’s EEG data.The CMI give better signal recognition than the raw data, and were used to advantage as correlates of behavioral states.In-sample data was used for training [41], and out-of-sample data was used for testing [43] these fits.These results gav e strong quantitative support for an accurate intuitive picture, portraying neocortical interactions as having common algebraic physics mechanisms that scale across quite disparate spatial scales and functional or behavioral phenomena, i.e., describing interactions among neurons, columns ofneurons, and regional masses of neurons.4.1.5. Generic Mesoscopic Neural NetworksSMNI was applied to a parallelized generic mesoscopic neural networks (MNN)[29], adding computational power to a similar paradigm proposed for target recognition [18].Figure 2.Scales of interactions among minicolumns are represented, within macrocolumns,across macrocolumns, and across regions of macrocolumns.“Learning” takes place by presenting the MNN with data, and parametrizing the data in terms of the firings, or multivariate firings. The “weights,”or coefficients of functions of firings appearing in the drifts and diffusions, are fit to incoming data, considering the joint “effective”Lagrangian (including the logarithm of the prefactor in the probability distribution) as a dynamic cost function.This program of fitting coefficients in Lagrangian uses methods of ASA.“Prediction” takes advantage of a mathematically equivalent representation of the Lagrangian path-integral algorithm, i.e., a set of coupled Langevin rate-equations.A coarse deterministic estimate to “predict” the evolution can be applied using the most probable path, but PATHINT has been used.PATHINT,even when parallelized, typically can be too slow for “predicting” evolution of these systems.However, PATHTREE is much faster.The present project uses the same concepts, having sets of multiple variables define macrocolumns with a region, with long-ranged connectivity to other regions. Each macrocolumn has its own parameters, which define sets of possible patterns.Ultimately,ISM of course would not use functional relationships developed solely in neocortex, but rather those more appropriate to a given population.4.1.6. On Chaos in NeocortexThere are many papers on the possibility of chaos in neocortical interactions.While this phenomena may have some merit when dealing with small networks of neurons, e.g., in some circumstances such as epilepsy,these papers generally have considered only too simple models of neocortex.The author took a model of chaos that might be measured by EEG, developed and published by some colleagues, but adding background stochastic influences and parameters that were agreed to better model neocortical interactions.The resulting multivariate nonlinear conditional probability distribution was propagated many thousands of epochs, using the authors PATHINT code, to see if chaos could exist and persist under such a model [61]. There was absolutely no measurable instance of chaos surviving in this more realistic context.4.1.7. Mathematical DevelopmentSome of the algebra behind SMNI depicts variables and distributions that populate each representative macrocolumn in each region.A derived mesoscopic Lagrangian L M defines the short-time probability distribution of firings in a minicolumn, composed of ∼102neurons, given its just previous interactions with all other neurons in its macrocolumnar surround.G is used to represent excitatory (E )and inhibitory (I )contributions.G designates contributions from both E and I .P M =GΠP G M [M G (r ;t +τ)|M G (r ′;t )]=σj Σδ⎛⎜⎝jE Σσj −M E (r ;t +τ)⎞⎟⎠δ⎛⎜⎝jI Σσj −M I (r ;t +τ)⎞⎟⎠N jΠp σj≈GΠ(2πτg GG)−1/2exp(−NτL G M),P M≈(2πτ)−1/2g1/2exp(−NτL M),L M=L E M+L I M=(2N)−1(˙M G−g G)g GG′(˙M G′−g G′)+M G J G/( 2Nτ)−V′,V′=GΣV′′G G′(ρ∇M G′)2,g G=−τ−1(M G+N G tanh F G),g GG′=(g GG′)−1=δG′Gτ−1N G sech2F G,g=det(g GG′),F G=(V G−a|G|G′v|G|G′N G′−12A|G|G′v|G|G′M G′)(π[(v|G|G′)2+(φ|G|G′)2](a|G|G′N G′+12A|G|G′M G′)))1/2,a G G′=12A G G′+B G G′,(1)where A G G′and B G G′are minicolumnar-averaged inter-neuronal synaptic efficacies,v G G′andφG G′are averaged means and variances of contributions to neuronal electric polarizations.M G′and N G′in F G are afferent macrocolumnar firings, scaled to efferent minicolumnar firings by N/N*∼10−3,where N*is the number of neurons in a macrocolumn,∼105.Similarly,A G′G and B G′G have been scaled by N*/N∼103to keep F G invariant.V′are mesocolumnar nearest-neighbor interactions.4.1.7.1. Inclusion of Macroscopic CircuitryThe most important features of this development are described by the Lagrangian L G in the negative of the argument of the exponential describing the probability distribution, and the “threshold factor”F G describing an important sensitivity of the distribution to changes in its variables and parameters.To more properly include long-ranged fibers, when it is possible to numerically include interactions among macrocolumns, the J G terms can be dropped, and more realistically replaced by a modified threshold factor F G,F G=(V G−a|G|G′v|G|G′N G′−12A|G|G′v|G|G′M G′−a‡E E′v E E′N‡E′−12A‡EE′v E E′M‡E′)(π[(v|G|G′)2+(φ|G|G′)2](a|G|G′N G′+12A|G|G′M G′+a‡E E′N‡E′+12A‡EE′M‡E′)))1/2,a‡E E′=12A‡EE′+B‡EE′.(2)Here, afferent contributions from N‡E long-ranged excitatory fibers, e.g., cortico-cortical neurons, have been added, where N‡E might be on the order of 10% of N∗:Of the approximately1010to 1011neocortical neurons, estimates of the number of pyramidal cells range from 1/10 to 2/3.Nearly every pyramidal cell has an axon branch that makes a cortico-cortical connection; i.e., the number of cortico-cortical fibers is of the order1010.4.1.8. Portfolio of Physiological Indicators (PPI)The ISM project uses the SMNI distributions as a template for distributions of populations.The TRD project illustrates how such distributions can be developed as a Portfolio of Physiological Indicators (PPI), to calculate risk and uncertainty of functions, e.g., functions of Ideas, dependent on events that impact such populations [53].4.1.8.1. Multiple Imaging DataIt is clear that the SMNI distributions also can be used to process different imaging data beyond EEG, e.g., also MEG, PET,SPECT,fMRI, etc., where each set of imaging data is used to fit it own set of parameterized SMNI distributions using a common regional circuitry.(Different imaging techniques may。