Dependence of shake probability on nuclear charge in Li-, Na- and K-like ions
On the role of shake-off in single-photon double ionization
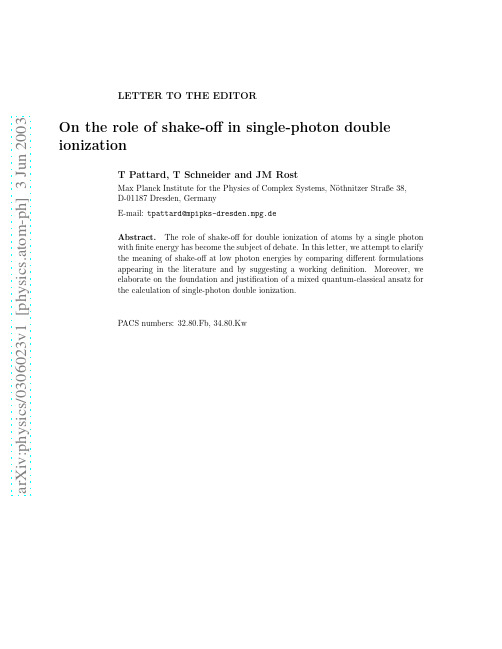
Letter to the Editor
3
evolution (i.e. the redistribution of the energy between the two electrons) in the spirit of an (e,2e)–like process with the additional possibility of shake-off. Such a “half-collision” picture has been originally suggested by Samson [11] and elaborated by Pattard and Burgd¨ orfer [4], allowing for shake-off processes which are not taken into account in Samson’s original model. In the SCR ansatz, the (e,2e)–like (“knock-out”) part of the cross section is calculated using a classical trajectory Monte Carlo method, to which the shake-off as a purely quantum mechanical process is added on top. In this spirit, shake-off is introduced as a more or less ad-hoc quantum correction to an essentially classical treatment. Here, we start from a fully quantum mechanical expression and see which kind of approximations lead to an SCR-like ansatz. In this way, further insight into the validity of the ansatz, concerning both technical details of the calculation as well as the approximate separation of physical mechanisms (shake-off and knock-out), can be obtained. In ref. [4], a Born series for the transition amplitude from the ground state ψi (0) to a final state ψf of a two-electron target following single-photon absorption has been derived. It was shown that, under the assumption of negligible electron–electron correlation in the ionized final state, the transition amplitude can be written as af i = −2πi δ (Ef − Ei − ω )
人们是否对科技产生依赖英语作文
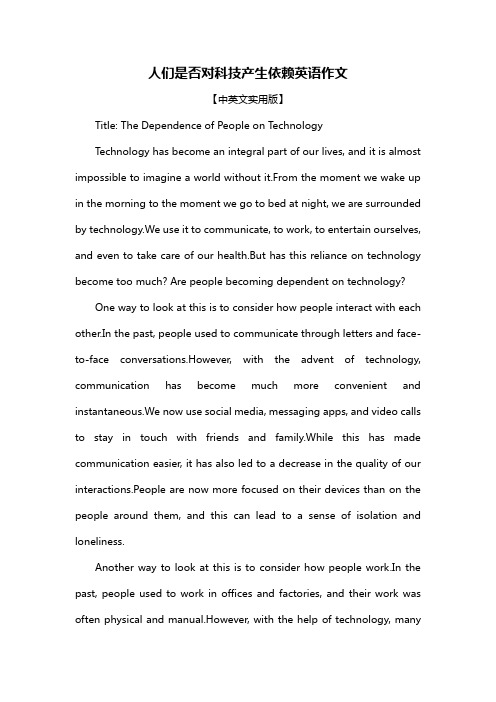
人们是否对科技产生依赖英语作文【中英文实用版】Title: The Dependence of People on TechnologyTechnology has become an integral part of our lives, and it is almost impossible to imagine a world without it.From the moment we wake up in the morning to the moment we go to bed at night, we are surrounded by technology.We use it to communicate, to work, to entertain ourselves, and even to take care of our health.But has this reliance on technology become too much? Are people becoming dependent on technology?One way to look at this is to consider how people interact with each other.In the past, people used to communicate through letters and face-to-face conversations.However, with the advent of technology, communication has become much more convenient and instantaneous.We now use social media, messaging apps, and video calls to stay in touch with friends and family.While this has made communication easier, it has also led to a decrease in the quality of our interactions.People are now more focused on their devices than on the people around them, and this can lead to a sense of isolation and loneliness.Another way to look at this is to consider how people work.In the past, people used to work in offices and factories, and their work was often physical and manual.However, with the help of technology, manyjobs have become more sedentary and less physical.People now spend most of their time sitting in front of a computer screen, and this can lead to a variety of health problems, including obesity, diabetes, and heart disease.In addition to this, technology has also become a crutch for many people.For example, many people rely on GPS to navigate, even when they are in familiar surroundings.Others rely on spell checkers and grammar checkers to write emails and documents, even when they are proficient in the language.This reliance on technology can lead to a decrease in people"s ability to perform basic tasks and can make them more vulnerable to technological failures.In conclusion, people have indeed become dependent on technology, and this reliance can have both positive and negative consequences.While technology has made our lives easier and more convenient, it has also led to a decrease in the quality of our interactions, a more sedentary lifestyle, and a reliance on technology as a crutch.It is important for people to find a balance between using technology to enhance their lives and maintaining their ability to perform basic tasks without the aid of technology.---标题:人们对科技的依赖科技已经成为我们生活的重要组成部分,几乎无法想象一个没有科技的世界。
Probability and Stochastic Processes
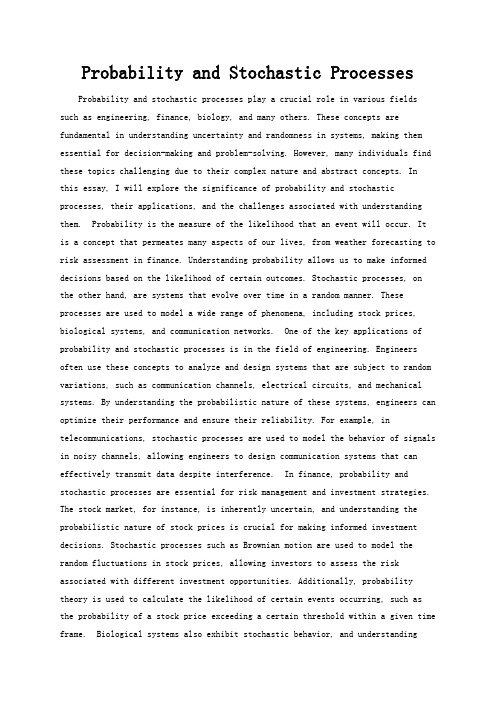
Probability and Stochastic Processes Probability and stochastic processes play a crucial role in various fields such as engineering, finance, biology, and many others. These concepts are fundamental in understanding uncertainty and randomness in systems, making them essential for decision-making and problem-solving. However, many individuals find these topics challenging due to their complex nature and abstract concepts. Inthis essay, I will explore the significance of probability and stochastic processes, their applications, and the challenges associated with understanding them. Probability is the measure of the likelihood that an event will occur. It is a concept that permeates many aspects of our lives, from weather forecasting to risk assessment in finance. Understanding probability allows us to make informed decisions based on the likelihood of certain outcomes. Stochastic processes, on the other hand, are systems that evolve over time in a random manner. These processes are used to model a wide range of phenomena, including stock prices, biological systems, and communication networks. One of the key applications of probability and stochastic processes is in the field of engineering. Engineers often use these concepts to analyze and design systems that are subject to random variations, such as communication channels, electrical circuits, and mechanical systems. By understanding the probabilistic nature of these systems, engineers can optimize their performance and ensure their reliability. For example, in telecommunications, stochastic processes are used to model the behavior of signals in noisy channels, allowing engineers to design communication systems that can effectively transmit data despite interference. In finance, probability and stochastic processes are essential for risk management and investment strategies. The stock market, for instance, is inherently uncertain, and understanding the probabilistic nature of stock prices is crucial for making informed investment decisions. Stochastic processes such as Brownian motion are used to model the random fluctuations in stock prices, allowing investors to assess the risk associated with different investment opportunities. Additionally, probability theory is used to calculate the likelihood of certain events occurring, such as the probability of a stock price exceeding a certain threshold within a given time frame. Biological systems also exhibit stochastic behavior, and understandingthis randomness is essential for fields such as genetics, epidemiology, and ecology. For example, in genetics, stochastic processes are used to model the random inheritance of genetic traits, allowing researchers to study the likelihood of certain genetic disorders being passed on to offspring. In epidemiology, stochastic models are used to simulate the spread of diseases within populations, taking into account factors such as random contact between individuals and the effectiveness of interventions. By understanding the probabilistic nature of these systems, researchers can develop strategies for disease control and prevention. Despite the wide-ranging applications of probability and stochastic processes, many individuals find these topics challenging to grasp. The abstract nature of probability, in particular, can be difficult to understand, as it deals with concepts such as randomness and uncertainty that are not always intuitive. Additionally, the mathematical formalism used to describe stochastic processes, such as Markov chains and random walks, can be daunting for those who are not familiar with advanced mathematical concepts. Another challenge in understanding probability and stochastic processes is the reliance on abstract models and theoretical concepts. While these models are essential for analyzing andpredicting the behavior of complex systems, they can be difficult to relate toreal-world phenomena. This disconnect between theory and practice can make it challenging for students and practitioners to fully grasp the significance of probability and stochastic processes in various fields. In conclusion,probability and stochastic processes are fundamental concepts that play a crucial role in understanding uncertainty and randomness in systems. These concepts have wide-ranging applications in fields such as engineering, finance, and biology, allowing researchers and practitioners to make informed decisions and develop effective strategies. However, the abstract nature of probability and the reliance on theoretical models can make these topics challenging to understand for many individuals. Despite these challenges, a solid understanding of probability and stochastic processes is essential for navigating the complexities of the modern world and making informed decisions in the face of uncertainty.。
probabilistic lane tracking in difficult road scenarios using

Probabilistic Lane Tracking in Difficult Road Scenarios Using StereovisionRadu Danescu and Sergiu Nedevschi,Member,IEEEAbstract—Accurate and robust lane results are of great sig-nificance in any driving-assistance system.To achieve robustness and accuracy in difficult scenarios,probabilistic estimation tech-niques are needed to compensate for the errors in the detection of lane-delimiting features.This paper presents a solution for lane estimation in difficult scenarios based on the particle-filtering framework.The solution employs a novel technique for pitch detection based on the fusion of two stereovision-based cues,a novel method for particle measurement and weighing using mul-tiple lane-delimiting cues extracted by grayscale and stereo data processing,and a novel method for deciding upon the validity of the lane-estimation results.Initialization samples are used for uniform handling of the road discontinuities,eliminating the need for explicit track initialization.The resulting solution has proven to be a reliable and fast lane detector for difficult scenarios.Index Terms—Cue fusion,lane detection,particlefiltering, stereovision,tracking.I.I NTRODUCTIONL ANE/ROAD detection has been a fertile researchfield for decades due to the great significance of accurate and robust road description results in any driving-assistance system.The algorithms have increasingly become complex,as the targeted scenarios increasingly became difficult.From the highway scenario,the lane-detection systems moved to city and country roads.With this move,the initial emphasis on lane-delimiting features,such as lane markings,was replaced by the emphasis on model parameter estimation techniques,which use static and dynamic knowledge-based probabilistic constraints to counteract possible noisy features and smooth the result. These constraints lead to probabilistic reasoning in the form of tracking,which is traditionally achieved by the use of the Kalmanfilter(KF).The use of KF tracking has the advantage of reducing the search space,eliminating the detection outliers, and smoothing the result.The features that make the KF solutions smooth and efficient are the very features that cause problems when the road is not continuous.Sharp turns,lane changes,and atypical road geometries pose problems to a tracker that represents the lane probability density as a Gaussian function,and the reduction of the search space around the past results makes it difficult to han-Manuscript received April10,2008;revised January5,2009and January28, 2009.First published April28,2009;current version published June2,2009. The Associate Editor for this paper was R.Hammoud.The authors are with the Technical University of Cluj-Napoca,400020Cluj-Napoca,Romania(e-mail:Radu.Danescu@cs.utcluj.ro;Sergiu.Nedevschi@ cs.utcluj.ro).Color versions of one or more of thefigures in this paper are available online at .Digital Object Identifier10.1109/TITS.2009.2018328dle new hypotheses and causes detection errors to accumulate if the search regions are drawn toward false delimiters. Particlefiltering is a novel technology for probability-based tracking,allowing multiple-hypothesis tracking,simple mea-surement,and faster handling of road discontinuities.This paper describes a lane-detection system that combines the advantage of particlefiltering,stereovision,and grayscale image processing to achieve robust lane-estimation results in difficult scenarios of city,highway,and country roads.II.P ROBABILISTIC F OUNDATIONS OF L ANE T RACKING While there is no universal definition of tracking,we can regard it as the process of reasoning about the state of a time-evolving entity,given a sequence of observations.In particular, lane tracking can be defined as the process of reasoning about the position and geometry of the lane,given a sequence of image-derived feature sets.The goal of tracking as probabilistic inference is to evaluate P(X i|Y0=y0,...,Y i=y i),i.e.,to compute the conditional probability density of state X i,given the sequence of measure-ments from the past and current frames.Due to the fact that the tracking process must deliver results at each frame and that a tracker should be able to function in mostly the same way for an indefinite period of time,the process of estimation of P(X i|Y0=y0,...,Y i=y i)has to be written in a recursive manner,such that the results of the past frames can be reused in the estimation for the current frame.To achieve this,four concepts are used.1)Dynamic model:P(X i|X i−1)is the probability of reach-ing some value of random variable X i,given the past state X i−1,under the assumption that only the immediate past matters.2)Prediction:Computation of the conditional probabilitydensity of the current state,given the past sequence of measurements,i.e.,P(X i|Y0=y0,...,Y i−1=y i−1).Given the simplification assumption that only the imme-diate past matters,the prediction probability values can be recursively computed,given the past results and the dynamic model,i.e.,P(X i|y0,...,y i−1)=P(X i|X i−1)P(X i−1|y0,...,y i−1)d X i−1.(1)3)Data association:At each frame i,there may be severalmeasurements that are available,and not all of them are equally useful.Denoting by y r i the r th measurement1524-9050/$25.00©2009IEEEFig.1.Analogy between a probability density function and a set of weighted samples.of frame i ,the probability of this measurement beinguseful is expressed as P (Y i =y ri|y 0,...,y i −1).If each measurement is conditionally independent of the others (the measurement independence assumption is taken),the usefulness of each measurement can be computed asP (Y i =y ri|y 0,...y i −1)=P (Y i =y ri |X i )P (X i |y 0,...,y i −1)d X i .(2)4)State update:State probability density P (X i |Y 0=y 0,...,Y i =y i ),which is the end result of the tracking process,is computed using Bayes’rule,i.e.,P (X i |y 0,...,y i )=P (y i |X i )P (X i |y 0,...,y i −1)P (y i |X i )P (X i |y 0,...,y i −1)d X i.(3)The equations of tracking as probabilistic inference are too complex to apply in the general case.Furthermore,the proba-bility densities involved are impossible to analytically represent most of the time and are therefore approximated.Approxi-mating means either coercing them to a known probability density function,such as a Gaussian,or maintaining a discrete numerical representation throughout the whole process.The Gaussian representation leads to the well-known KF solutions,and the representation as discrete samples leads to the particle-filtering solutions.III.P ARTICLE F ILTERINGA practical approach to tracking general probability density functions,i.e.,particle filtering,is described in [3].Instead of trying to analytically approximate an unknown function,their system uses N discrete values called “samples”or “particles.”At each given time t ,a particle i is defined by a value x i t and aweight πit ,with the sum of all weights being 1(see Fig.1).The problem of tracking becomes the problem of evaluating the values and the weights,given a dynamic model and an observation density function.For algorithm optimization purposes,a parameter is added to each particle,changing the particle representation to{x i t ,πi t ,c it ,i =1,...,N }.This parameter is defined as thesumFig.2.Same probability density function approximated by weighted andweightless particles.of the weights of each particle from 1to i (a cumulativehistogram).Each iteration of the CONDENSATION algorithm has the aim of evaluating a new set of particles,given the previous set,the dynamic model,and the measurements.The first step of the algorithm is resampling.A weighted sample set is transformed into a new set of samples of equal weight but uneven concentration through the domain of values of x .This is achieved by performing N random draws from the particle set using the particle weights as probabilities for particle selection.A particle having a larger weight will be selected several times,whereas a particle having a low weight may not be selected at all.The new set of weightless particles and the weighted set approximate the same density function (see Fig.2).Prediction is the next step of the CONDENSATION algo-rithm.In a general form,this is achieved by sampling from the dynamic model density function.This function describes the likelihood of each possible current state,given the assumption that the past state is described by the value of weightless particle i .A more pragmatic approach is to assume that the new state is derived from the past state partly by a deterministic process,which is described by a function or a linear transfor-mation,and partly by a random factor.Each weightless particle that resulted from the resampling step is subjected to a deterministic transformation,which will take into account the state transition equations of the system,and a stochastic diffusion,which will account for the random events that may change the state (see Figs.3and 4).The final step of the algorithm is the measurement/update process.In the general formulation of the tracking problem as probabilistic inference,updating means applying Bayes’rule to get the posterior probability density,given the prior probability density and the measurement.The prior state prob-ability density is,at this point,completely encoded in the distribution of the values of the weightless particles through the domain of possible state values.The posterior probability density function is obtained by simply weighing the particles using the likelihood of observation p (y t |x t =x i t ).Several cues can be combined in this step by multiplication using the cue conditional independence assumption,if applicable (see Fig.5).Fig.3.Deterministic drift using weightlessparticles.Fig.4.Stochastic diffusion using weightlessparticles.Fig.5.Weightless particles are weighed by measurement.IV .R ELATED W ORKLane estimation through Kalman filtering was pioneered by Dickmanns and Mysliwetz [1],and since then,many re-searchers have devised working solutions,such as [2]–[7].The Kalman-filter-based lane tracking relies on the model-based prediction for establishing search regions for detection and uses the detection results to update the state.This approach expectsa continuously varying road situation,and the discontinuities are usually handled by reinitializing the tracking process.The solution presented in [6]handles some particular case of road discontinuities by using two instances of the road model,but it is clear that the KF is not the best choice for tracking discontinuous roads.A shift toward particle filtering for lane estimation is cur-rently taking place.A particle-based lane solution usually starts with particle sampling,followed by drifting and measurement.The measurement step is considerably simpler,in comparison to the KF,because it usually consists of a comparison between the particle and the image data,from which a weight is derived;therefore,no complex detection algorithms are required.How-ever,the measurement step is executed for each particle,and therefore,the simplicity is essential for adequate time perfor-mance.Southall and Taylor [10]presented a lane detector based on a condensation framework,which uses lane-marking points as measurement features.Each point in the image receives a score based on the distance to the nearest lane marking,and these scores are used to compute the matching score of each particle.The system uses partitioned sampling (two-step sampling and measurement using subsets of the state space,achieving a multiresolution effect),importance sampling,and initialization samples (completely random samples from the whole parameter space),which cope faster with lane discon-tinuities.In [4],we find a lane detection system that uses the particle-filtering framework to fuse multiple image cues (color,edges,and Laplacian of Gaussian).For each cue,a comparison method between the image data and the particle is designed,the likelihood is computed,and then,the likelihoods are combined by multiplication.This solution also uses initialization samples for faster lane relocation and additional sampling around the best-weighted particles for improvement of accuracy.The much simpler way in which a particle filter handles the measurement information allows the use of a wider range of cues.Such is the case of the lane detector for country roads,as presented in [5],where the image space is divided into road and nonroad areas and each pixel in these areas contributes to the final weight by its intensity,color,edge,and texture information.The likelihood of each feature value to belong to either road or off-road areas is computed using trained his-tograms,thus allowing a non-Gaussian multimodal probability density not only for the lane state but for the measurement as well.The work presented in [11]also shows the value of the particle-filtering technique for heterogeneous cue fusion,when image information is fused with a Global Positioning System and map information for long-distance lane estimation.In [12],the authors described a system that uses a hybrid approach,i.e.,combining lane border hypotheses generated using a RANdom-SAmple-Consensus (RANSAC)-type algorithm with hypothe-ses from a particle filter and then using further probabilistic reasoning to choose the best border pair to delimit the lane.V .S OLUTION O UTLINEThe system continuously evaluates the state of the lane by means of a set of particles.There is no initialization phase;therefore,each cycle is run exactly in the same way,as shown inne-detection algorithm outline.Fig.6.The cycle starts with particle resampling,which is done partly from the previous particle distribution and partly from a generic distribution that covers all lane geometries to cover the possible discontinuities that may arise.The deterministic drift is applied to all particles,taking into account ego motion param-eters,such as speed,yaw rate,and frame timestamps;then, stochastic diffusion will alter each particle in a random way. Pitch detection is done independently of the particle system using a probabilistic approach.The value of the detected pitch is set to each particle.The pitch value is also used to select the road features,which are then used to weigh the particles.A validation step ensures that the particles are locked on a lane,and if this step succeeds,a lane representation is estimated.VI.A LGORITHM D ESCRIPTIONne ParticlesThe lane state probability density is described at a given time t by a set of N weighted particles p(x)≈{x i t,πi t, i=1,...,N}.Particle value x is a lane state hypothesis in the form of a lane description vector.The coordinate system that is used has the point of origin on the ground in front of the ego vehicle,relatively centered to the width of the car.The X-axis is positive toward the right of the vehicle,the Y-axis is positive toward the ground,and the Z-axis is positive along the forward direction.The lane is a surface that stretches forward and is bounded by two delimiting curves.The X coordinate of the delimiting curves depends on the lane parameters,the chosen distance Z,and the delimiter type t(left or right),i.e.,X=h(Z,t).We will denote the preceding equation as the horizontal profile of the lane.The lane parameters that affect function h will be denoted as horizontal profile parameters(such as the horizontal curvature).In the same way,we can describe the variation of the Y coordinate of each of the delimiters,with the equation of the vertical profile of the lane,i.e.,Y=v(Z,t).The lane-tracking system was designed in a modular fashion, with the equations for the vertical and horizontal profiles being easily configurable.The measurement function is independent of the3-D model,as long as the sets of3-D points for the delimiters are available.We have found that,for the majority of the cases,the following set of parameters was sufficient:x i t=⎡⎢⎢⎢⎢⎢⎢⎢⎣W−lane widthC H−horizontal curvatureC V−vertical curvatureX C−lateral offsetα−pitch angleγ−roll angleψ−yaw angle⎤⎥⎥⎥⎥⎥⎥⎥⎦.Due to the configurable nature of the system,we have been able to experiment with several other models and parameter sets.A model that included a width variation parameter has successfully been tested in highway scenarios(the results sec-tion includes the tests done with this model),but the simpler model previously described has proven to be more reliable in urban scenarios.A quite powerful argument against the use of a very complex lane representation model is that the visibility range is quite limited due to the camera focal distance and the complexity of city traffic.B.PredictionBefore prediction can be applied,the past state described by the particle set has to be resampled into particles of equal weight.A fraction R=0.1N of the particles will be selected from a uniform probability distribution spanning the whole range of possible lane parameters.These particles account for the probability that the currently tracked lane can be erroneous or that a better lane candidate appears,such as in the case of lane change or road forking.Each particle is transformed via prediction,which is achieved by applying the following equation:x i t=A tˆx i t−1+B t u t+w tA t=⎡⎢⎢⎢⎢⎢⎢⎢⎣1000000010000000100000−s2t20100s t000010000000100−s t00001⎤⎥⎥⎥⎥⎥⎥⎥⎦B t=⎡⎢⎢⎢⎢⎢⎢⎢⎣s2t2s t⎤⎥⎥⎥⎥⎥⎥⎥⎦u t=c t.(4)plex city scene with road,cars,and walls and a side view of the reconstructed3-D points.The possible domain of pitch variation is highlighted. Matrix A t is the linear transformation that encodes the way the lane evolves in time in the absence of any input from the driver,and B t is the matrix that relates the driver input to the lane evolution.The input consists of c t,which is the curvature of the vehicle’s trajectory derived from the yaw rate. Matrices A t and B t depend on space s t traveled by the vehicle between measurements.Part A tˆx i t−1+B t u t is the deterministic part of the predic-tion,when motion laws are applied and each possible past lane configuration is clearly mapped onto a present configuration. In addition to the deterministic part,each particle’s position is altered by a random value w t,which is drawn from a Gaussian distribution of zero mean and covariance matrix Q t.Covariance matrix Q t is obtained by scaling afixed matrix Q0,which is calibrated for a time of100ms between frames,with the actual elapsed time between measurements(as the frame rate is notfixed).This is natural,as a longer time between mea-surements allows the lane to deviate more from the predicted configuration.C.Pitch DetectionPitch detection has to be handled somehow differently out-side of the particlefiltering framework due to the following reasons:The pitch does not track well(is not very predictable), and pitch selection influences the measurement data,which are selected from the3-D setpoints knowing the pitch angle (see Fig.7).Assuming that the origin of the center of coordinates is at ground level,immediately in the front of the car,it can be assumed that,for about10–20m,the road seen from one side will be a line passing through this origin.This line is defined by the pitch angle alone.Similarly to our previous version of the stereovision-based lane detection[7],the process of pitch detection starts by building a polar histogram that counts the points along each line passing through the origin in the lateral projection(distance–height plane).The lines correspond to discrete values of the pitch angle,which are spaced at0.1◦and range from−2◦to2◦.The algorithm for polar histogram building is given here.Initialize polar histogram H(index)to0for each index. For each3-D point pIf distance(p)>Limit,go to the next point.Find the angle of the line passing through p and the origin,i.e.,αp=tan−1height(p)distance(p).(5)Ifαp>2◦orαp<−2◦,go to the next point.Find the index ofαp in the polar histogram,i.e.,index p=αp+2◦0.1◦.Increment the polar histogram by a variable amount,tak-ing into account the variability of the point density with the distance,i.e.,H(index p)=H(index p)+distance(p)2K.(6) End ForThe difference from the previous pitch detection method is how we process this polar histogram.Previously,we found the maximum of the histogram and then scan the histogram bottom up until a value that is greater or equal to two thirds of the maximum was found.The reasoning behind this approach is that the road is thefirst structure of a substantial number of points encountered scanning the scene from bottom up,and the “substantial”part is relative to the scene.The problem with the previous approach is that it is hard to justify its correctness,and one can imagine some rare situations when it would fail.For the current lane detection algorithm,a probabilistic approach is used,which describes better relations between the real-world and possible pitch values.This means that,for each of the pitch candidatesαindex,we will approximate probability density p(α=αindex),given the available information. There are several assumptions that will govern the process of probability computation.Thefirst assumption is that pitch history does not matter,as the pitch variation is mostly due to imperfections in the road surface,which are not easy to predict. (One can argue that an oscillatory model of the pitch variation can be used,but it would introduce a constraint that can lead to wrong estimations if not properly calibrated.)This means that the pitch probability density will be derived from current measurements alone,i.e.,p(α|y1,y2,...,y t)=p(α|y t).(7)The second assumption is that there is no prior probability density;therefore,the probability density of the pitch variable is directly proportional to the measurement likelihood,i.e.,p(α|y t)∝p(y t|α).(8) The measurement is composed of two cues,which were derived from two assumptions about the3-D road points seen in the lateral projection.1)The road points should be nearly collinear.2)Most of the points in the3-D space are above the roadsurface.bining the cues for pitch.(a)Polar histogram.(b)Cumulative histogram.(c)Combination.The cue corresponding to thefirst assumption has the like-lihood directly proportional to polar histogram H,and the likelihood for the cue of the second assumption is directly pro-portional to a cumulative histogram derived from H,i.e.,CH.p(y H|α=αindex)∝H(index)(9)p(y CH|α=αindex)∝CH(index)(10)p(α=αindex|y t)∝H(index)CH(index).(11) The pitch candidate with the highest likelihood,which corre-sponds to the highest value of the histogram product,is chosen as the pitch estimate.Fig.8shows the effect of pitch cue fusion,leading to a clear maximum,even if the complex scene leads to multiple strong peaks in the polar histogram.Another estimation method that was taken into consideration was the weighted sum of the pitch candidates,but the maximum leads to better results.The value vectors x of the predicted particles are modified by setting their pitchfield to the estimated pitch value.This pitch value is also used for selecting the road points from the available3-D point set to perform the next stages of the measurement.D.Mapping the Particles in the Image SpacePitch detection is the only part of the measurement process that happens in the3-D space,and for the next stages,the parti-cles have to be compared to image space measurement data.To achieve the comparison,from each particle value of the form x i t=(W,C H,C V,X C,α,γ,ψ)T,a measurement space vector is generated,i.e.,y i t=(v1,...,v P,u L,1,...,u L,P,u R,1,..., u R,P).Values v are coordinates of the image lines,and values u are coordinates of the image columns.The v values are common to the left and right delimiters.P is the number of points chosen to describe each lane delimiter in the image space.To derive y i t from x i t,three steps have to be taken.1)Generate P points in the3-D space for each lane delim-iter.The points will be equally spaced on distance axis Z,and their X and Y coordinates(lateral and height) will be given by the horizontal and vertical profiles of the lane.The nearest points will start at distance Z B,which is the closest distance that allows the road to be visible to our system.The points will span a detection distanceD.Detection distance D is variable,and its adjustmentis based on the vehicle’s speed.The rationale behind this decision is that a longer distance is needed if the vehicle travels at high speeds,which is usually on straight or low-curvature roads,but a shorter distance is needed at slow speeds to handle narrower curvatures.Distance D coversa second of vehicle movement at the current speed,but noshorter than5m and no longer than60m.2)Project the3-D points in the image space using thecamera parameters.For each lane delimiter,a chain of unevenly spaced points will be obtained.3)Intersect the segments obtained by linking the projectedpoints for each side with a set of evenly spaced horizontal lines.The points of intersection are the points that will form the particle representation in the image space y i t. E.Visual CuesAfter the pitch angle has been detected from the3-D point set,a rough approximation of the road geometry can be made based on this angle alone.The rough approximation is used for road point selection.The image edges corresponding to these 3-D points form ourfirst measurement cue.The lane-marking edge points are detected using an algo-rithm based on the tried-and-tested dark–light–dark transition detection principle[8].In addition to the lane markings,another high-priority lane-delimiting feature is the curb,and the curbs are detected using height variations in a dense stereovision map[9]and then converted into image edges.Due to the fact that lane markings and curbs are of similar priorities,they are inserted in a common“special edge”map,which represents the second lane measurement cue.To allow comparison between the particles and the measure-ment,each cue map(road edges or special edges)undergoes distance transformation(see Fig.9).F.Particle Weighing by MeasurementGiven the a priori probability density encoded in the distri-bution of the particle values throughout the state space,it is now time to compute the posterior probability density,which will encode all the knowledge about the lane state that we are able to extract from the sequence of measurements up to the current time t.This is achieved by assigning new weights to theFig.9.Visual information.(a)Original grayscale image.(b)Edges cor-responding to 3-D points contained in the road surface and the associated distance transform image.(c)Markings and curbs and their associated distance transformimage.Fig.10.Positive and negative points.The positive points are lane boundary points,and the negative points are points inside the lane area.predicted particles,which are proportional to the measurement likelihood,given the state hypothesis,i.e.,πit =p y t |x t =x i t .(12)The measurement likelihood is obtained by multiplying theedge likelihood and the marking/curb likelihood under the measurement independence assumption,i.e.,p y t |x t =x i t =p road _edges |x t =x i t·p mark _curb |x t =x i t .(13)To compute the likelihood of the two measurement cues,adistance between the lane state hypothesis and the measurement has to be computed.The distance transformation of the two edge images now becomes very helpful.Ideally,the lane hypothesis boundaries’projections in the image space have to exactly fit on the edges of the visual cues.In addition,the area inside the hypothetic lane projection has to be as free of edges as possible.To test these two conditions,two sets of points are used:1)the positive points,which are points belonging to the lane delimiters’projection in the image space,and 2)the negative points,which are points that are near the borders and reside inside the projected lane area (see Fig.10).The positive points will generate the positive distance;this is obtained by averaging the distance-transformed pixel values at these points’coordinates.The distance corresponding to the negative points is the complement of the distance-transformedimage at these points’coordinates.The two distances are com-bined by weighted averaging,i.e.,DM=αD M (+)+βD M (−)(α+β).(14)The value of weight parameters αand βhas been set to 2and 1,respectively,through trial-and-error experiments.Now,for each measurement M ,the measurement likelihood is computed using a Gaussian distribution to relate the probabil-ity to the distance between the prediction and the visual data,i.e.,pM |x t =x it =1σM √2πe−D 2M 2σ2M.(15)Each particle will receive as weight the product of the two likelihoods.At this step,the particles that show a degenerate lane,such as a lane that is too narrow,too wide,or too far from the vehicle’s position,will receive a null weight,preventing them for spawning new candidates in the next cycle.The final step is to normalize the new weights so that their sum is 1,and the system is ready to perform a new tracking ne ValidationUnlike a KF lane-tracking solution,the particle-filtering system does not need initialization or measurement validation before track update.The particles will freely evolve,eventually clustering around the best lane estimate,if the system is prop-erly designed and the measurements are relevant.However,the system must know when a valid lane is being tracked if it is to be used for practical purposes.The first attempt was to analyze the particle distribution in the state space and validate the situation when the particles were reasonably clustered.However,we have observed that particles tend to cluster,even in the presence of weak measurements,and this clustering does not guarantee the validity of the final estimate.A much more successful solution is to compare the average weight of the predicted (from sampled)particles against the average weight of the completely random particles that are added in the sampled set.Recalling that N denotes the total number of particles and R denotes the number of totally random particles and that the random particles are inserted at the head of the particle list (without altering the probability density),a quality factor is defined asq =R Ni =R +1πit(N −R )Ri =1πi t.(16)If q is higher than a threshold,the lane track is consideredvalid for output,and a lane state vector will be estimated from the particle set.A high quality factor means that the visual cues support the predicted lane to a much higher degree than some completely random lane parameters,which supports the hypothesis that the lane approximated by the particles is correct (agrees with the observation).The threshold that we found to work best is 10.。
Chapter 3 Probability and InformationTheory

CHAPTER 3. PROBABILITY AND INFORMATION THEORY
makes heavy use of probability theory. This is because machine learning must always deal with uncertain quantities, and sometimes may also need to deal with stochastic (non-deterministic) quantities. Uncertainty and stochasticity can arise from many sources. Researchers have made compelling arguments for quantifying uncertainty using probability since at least the 1980s. Many of the arguments presented here are summarized from or inspired by Pearl (1988). Nearly all activities require some ability to reason in the presence of uncertainty. In fact, beyond mathematical statements that are true by definition, it is difficult to think of any proposition that is absolutely true or any event that is absolutely guaranteed to occur. There are three possible sources of uncertainty: 1. Inherent stochasticity in the system being modeled. For example, most interpretations of quantum mechanics describe the dynamics of subatomic particles as being probabilistic. We can also create theoretical scenarios that we postulate to have random dynamics, such as a hypothetical card game where we assume that the cards are truly shuffled into a random order. 2. Incomplete observability. Even deterministic systems can appear stochastic when we cannot observe all of the variables that drive the behavior of the system. For example, in the Monty Hall problem, a game show contestant is asked to choose between three doors and wins a prize held behind the chosen door. Two doors lead to a goat while a third leads to a car. The outcome given the contestant’s choice is deterministic, but from the contestant’s point of view, the outcome is uncertain. 3. Incomplete modeling. When we use a model that must discard some of the information we have observed, the discarded information results in uncertainty in the model’s predictions. For example, suppose we build a robot that can exactly observe the location of every object around it. If the robot discretizes space when predicting the future location of these objects, then the discretization makes the robot immediately become uncertain about the precise position of objects: each object could be anywhere within the discrete cell that it was observed to occupy. In many cases, it is more practical to use a simple but uncertain rule rather than a complex but certain one, even if the true rule is deterministic and our modeling system has the fidelity to accommodate a complex rule. For example, the simple rule “Most birds fly” is cheap to develop and is broadly useful, while a rule of the form, “Birds fly, except for very young birds that have not yet learned to fly, sick or injured birds that have lost the ability to fly, flightless species of birds
英文外刊,抗击疟疾的科学家们,陷入了生物伦理学的争论
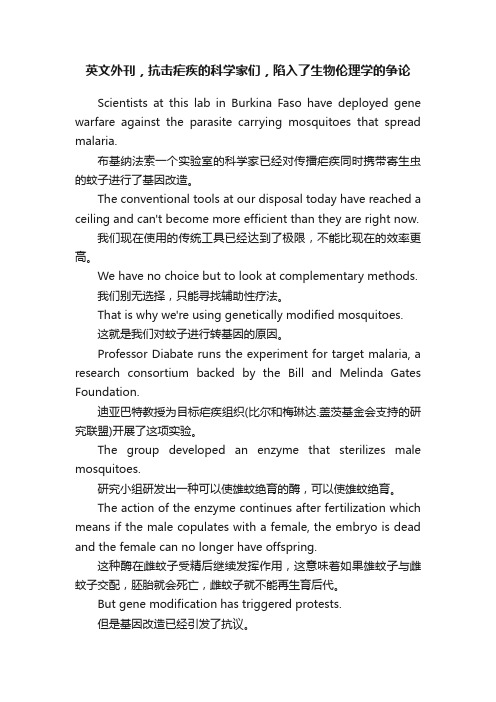
英文外刊,抗击疟疾的科学家们,陷入了生物伦理学的争论Scientists at this lab in Burkina Faso have deployed gene warfare against the parasite carrying mosquitoes that spread malaria.布基纳法索一个实验室的科学家已经对传播疟疾同时携带寄生虫的蚊子进行了基因改造。
The conventional tools at our disposal today have reached a ceiling and can't become more efficient than they are right now.我们现在使用的传统工具已经达到了极限,不能比现在的效率更高。
We have no choice but to look at complementary methods.我们别无选择,只能寻找辅助性疗法。
That is why we're using genetically modified mosquitoes.这就是我们对蚊子进行转基因的原因。
Professor Diabate runs the experiment for target malaria, a research consortium backed by the Bill and Melinda Gates Foundation.迪亚巴特教授为目标疟疾组织(比尔和梅琳达.盖茨基金会支持的研究联盟)开展了这项实验。
The group developed an enzyme that sterilizes male mosquitoes.研究小组研发出一种可以使雄蚊绝育的酶,可以使雄蚊绝育。
The action of the enzyme continues after fertilization which means if the male copulates with a female, the embryo is dead and the female can no longer have offspring.这种酶在雌蚊子受精后继续发挥作用,这意味着如果雄蚊子与雌蚊子交配,胚胎就会死亡,雌蚊子就不能再生育后代。
SCI写作句型汇总

S C I论文写作中一些常用的句型总结(一)很多文献已经讨论过了一、在Introduction里面经常会使用到的一个句子:很多文献已经讨论过了。
它的可能的说法有很多很多,这里列举几种我很久以前搜集的:A.??Solar energy conversion by photoelectrochemical cells?has been intensively investigated.?(Nature 1991, 353, 737 - 740?)B.?This was demonstrated in a number of studies that?showed that composite plasmonic-metal/semiconductor photocatalysts achieved significantly higher rates in various photocatalytic reactions compared with their pure semiconductor counterparts.C.?Several excellent reviews describing?these applications are available, and we do not discuss these topicsD.?Much work so far has focused on?wide band gap semiconductors for water splitting for the sake of chemical stability.(DOI:10.1038/NMAT3151)E.?Recent developments of?Lewis acids and water-soluble organometalliccatalysts?have attracted much attention.(Chem. Rev. 2002, 102, 3641?3666)F.?An interesting approach?in the use of zeolite as a water-tolerant solid acid?was described by?Ogawa et al(Chem.Rev. 2002, 102, 3641?3666)G.?Considerable research efforts have been devoted to?the direct transition metal-catalyzed conversion of aryl halides toaryl nitriles. (J. Org. Chem. 2000, 65, 7984-7989) H.?There are many excellent reviews in the literature dealing with the basic concepts of?the photocatalytic processand the reader is referred in particular to those by Hoffmann and coworkers,Mills and coworkers, and Kamat.(Metal oxide catalysis,19,P755)I. Nishimiya and Tsutsumi?have reported on(proposed)the influence of the Si/Al ratio of various zeolites on the acid strength, which were estimated by calorimetry using ammonia. (Chem.Rev. 2002, 102, 3641?3666)二、在results and discussion中经常会用到的:如图所示A. GIXRD patterns in?Figure 1A show?the bulk structural information on as-deposited films.?B.?As shown in Figure 7B,?the steady-state current density decreases after cycling between 0.35 and 0.7 V, which is probably due to the dissolution of FeOx.?C.?As can be seen from?parts a and b of Figure 7, the reaction cycles start with the thermodynamically most favorable VOx structures(J. Phys. Chem. C 2014, 118, 24950?24958)这与XX能够相互印证:A.?This is supported by?the appearance in the Ni-doped compounds of an ultraviolet–visible absorption band at 420–520nm (see Fig. 3 inset), corresponding to an energy range of about 2.9 to 2.3 eV.B. ?This?is consistent with the observation from?SEM–EDS. (Z.Zou et al. / Chemical Physics Letters 332 (2000) 271–277)C.?This indicates a good agreement between?the observed and calculated intensities in monoclinic with space groupP2/c when the O atoms are included in the model.D. The results?are in good consistent with?the observed photocatalytic activity...E. Identical conclusions were obtained in studies?where the SPR intensity and wavelength were modulated by manipulating the composition, shape,or size of plasmonic nanostructures.?F.??It was also found that areas of persistent divergent surfaceflow?coincide?with?regions where convection appears to be consistently suppressed even when SSTs are above 27.5°C.(二)1. 值得注意的是...A.?It must also be mentioned that?the recycling of aqueous organic solvent is less desirable than that of pure organic liquid.B.?Another interesting finding is that?zeolites with 10-membered ring pores showed high selectivities (>99%) to cyclohexanol, whereas those with 12-membered ring pores, such as mordenite, produced large amounts of dicyclohexyl ether. (Chem. Rev. 2002, 102,3641?3666)C.?It should be pointed out that?the nanometer-scale distribution of electrocatalyst centers on the electrode surface is also a predominant factor for high ORR electrocatalytic activity.D.?Notably,?the Ru II and Rh I complexes possessing the same BINAP chirality form antipodal amino acids as the predominant products.?(Angew. Chem. Int. Ed., 2002, 41: 2008–2022)E. Given the multitude of various transformations published,?it is noteworthy that?only very few distinct?activation?methods have been identified.?(Chem. Soc. Rev., 2009,?38, 2178-2189)F.?It is important to highlight that?these two directing effects will lead to different enantiomers of the products even if both the “H-bond-catalyst” and the?catalyst?acting by steric shielding have the same absolute stereochemistry. (Chem. Soc. Rev.,?2009,?38, 2178-2189)G.?It is worthwhile mentioning that?these PPNDs can be very stable for several months without the observations of any floating or precipitated dots, which is attributed to the electrostatic repulsions between the positively charge PPNDs resulting in electrosteric stabilization.(Adv. Mater., 2012, 24: 2037–2041)2.?...仍然是个挑战A.?There is thereby an urgent need but it is still a significant challenge to?rationally design and delicately tail or the electroactive MTMOs for advanced LIBs, ECs, MOBs, and FCs.?(Angew. Chem. Int. Ed.2 014, 53, 1488 – 1504)B.?However, systems that are?sufficiently stable and efficient for practical use?have not yet been realized.C.??It?remains?challenging?to?develop highly active HER catalysts based on materials that are more abundant at lower costs. (J. Am. Chem.Soc.,?2011,?133, ?7296–7299)D.?One of the?great?challenges?in the twenty-first century?is?unquestionably energy storage. (Nature Materials?2005, 4, 366 - 377?)众所周知A.?It is well established (accepted) / It is known to all / It is commonlyknown?that?many characteristics of functional materials, such as composition, crystalline phase, structural and morphological features, and the sur-/interface properties between the electrode and electrolyte, would greatly influence the performance of these unique MTMOs in electrochemical energy storage/conversion applications.(Angew. Chem. Int. Ed.2014,53, 1488 – 1504)B.?It is generally accepted (believed) that?for a-Fe2O3-based sensors the change in resistance is mainly caused by the adsorption and desorption of gases on the surface of the sensor structure. (Adv. Mater. 2005, 17, 582)C.?As we all know,?soybean abounds with carbon,?nitrogen?and oxygen elements owing to the existence of sugar,?proteins?and?lipids. (Chem. Commun., 2012,?48, 9367-9369)D.?There is no denying that?their presence may mediate spin moments to align parallel without acting alone to show d0-FM. (Nanoscale, 2013,?5, 3918-3930)(三)1. 正如下文将提到的...A.?As will be described below(也可以是As we shall see below),?as the Si/Al ratio increases, the surface of the zeolite becomes more hydrophobic and possesses stronger affinity for ethyl acetate and the number of acid sites decreases.(Chem. Rev. 2002, 102, 3641?3666)B. This behavior is to be expected and?will?be?further?discussed?below. (J. Am. Chem. Soc.,?1955,?77, 3701–3707)C.?There are also some small deviations with respect to the flow direction,?whichwe?will?discuss?below.(Science, 2001, 291, 630-633)D.?Below,?we?will?see?what this implies.E.?Complete details of this case?will?be provided at a?later?time.E.?很多论文中,也经常直接用see below来表示,比如:The observation of nanocluster spheres at the ends of the nanowires is suggestive of a VLS growth process (see?below). (Science, 1998, ?279, 208-211)2. 这与XX能够相互印证...A.?This is supported by?the appearance in the Ni-doped compounds of an ultraviolet–visible absorption band at 420–520 nm (see Fig. 3 inset), corresponding to an energy range of about 2.9 to 2.3 eVB.This is consistent with the observation from?SEM–EDS. (Chem. Phys. Lett. 2000, 332, 271–277)C.?Identical conclusions were obtained?in studies where the SPR intensity and wavelength were modulated by manipulating the composition, shape, or size of plasmonic nanostructures.?(Nat. Mater. 2011, DOI: 10.1038/NMAT3151)D. In addition, the shape of the titration curve versus the PPi/1 ratio,?coinciding withthat?obtained by fluorescent titration studies, suggested that both 2:1 and 1:1 host-to-guest complexes are formed. (J. Am. Chem. Soc. 1999, 121, 9463-9464)E.?This unusual luminescence behavior is?in accord with?a recent theoretical prediction; MoS2, an indirect bandgap material in its bulk form, becomes a direct bandgapsemiconductor when thinned to a monolayer.?(Nano Lett.,?2010,?10, 1271–1275)3.?我们的研究可能在哪些方面得到应用A.?Our ?ndings suggest that?the use of solar energy for photocatalytic watersplitting?might provide a viable source for?‘clean’ hydrogen fuel, once the catalyticef?ciency of the semiconductor system has been improved by increasing its surface area and suitable modi?cations of the surface sites.B. Along with this green and cost-effective protocol of synthesis,?we expect that?these novel carbon nanodots?have potential applications in?bioimaging andelectrocatalysis.(Chem. Commun., 2012,?48, 9367-9369)C.?This system could potentially be applied as?the gain medium of solid-state organic-based lasers or as a component of high value photovoltaic (PV) materials, where destructive high energy UV radiation would be converted to useful low energy NIR radiation. (Chem. Soc. Rev., 2013,?42, 29-43)D.?Since the use of?graphene?may enhance the photocatalytic properties of TiO2?under UV and visible-light irradiation,?graphene–TiO2?composites?may potentially be usedto?enhance the bactericidal activity.?(Chem. Soc. Rev., 2012,?41, 782-796)E.??It is the first report that CQDs are both amino-functionalized and highly fluorescent,?which suggests their promising applications in?chemical sensing.(Carbon, 2012,?50,?2810–2815)(四)1. 什么东西还尚未发现/系统研究A. However,systems that are sufficiently stable and efficient for practical use?have not yet been realized.B. Nevertheless,for conventional nanostructured MTMOs as mentioned above,?some problematic disadvantages cannot be overlooked.(Angew. Chem. Int. Ed.2014,53, 1488 – 1504)C.?There are relatively few studies devoted to?determination of cmc values for block copolymer micelles. (Macromolecules 1991, 24, 1033-1040)D. This might be the reason why, despite of the great influence of the preparation on the catalytic activity of gold catalysts,?no systematic study concerning?the synthesis conditions?has been published yet.?(Applied Catalysis A: General2002, 226, ?1–13)E.?These possibilities remain to be?explored.F.??Further effort is required to?understand and better control the parameters dominating the particle surface passivation and resulting properties for carbon dots of brighter photoluminescence. (J. Am. Chem. Soc.,?2006,?128?, 7756–7757)2.?由于/因为...A.?Liquid ammonia?is particularly attractive as?an alternative to water?due to?its stability in the presence of strong reducing agents such as alkali metals that are used to access lower oxidation states.B.?The unique nature of?the cyanide ligand?results from?its ability to act both as a σdonor and a π acceptor combined with its negativecharge and ambidentate nature.C.?Qdots are also excellent probes for two-photon confocalmicroscopy?because?they are characterized by a very large absorption cross section?(Science ?2005,?307, 538-544).D.?As a result of?the reductive strategy we used and of the strong bonding between the surface and the aryl groups, low residual currents (similar to those observed at a bare electrode) were obtained over a large window of potentials, the same as for the unmodified parent GC electrode. (J. Am. Chem. Soc. 1992, 114, 5883-5884)E.?The small Tafel slope of the defect-rich MoS2 ultrathin nanosheets is advantageous for practical?applications,?since?it will lead to a faster increment of HER rate with increasing overpotential.(Adv. Mater., 2013, 25: 5807–5813)F. Fluorescent carbon-based materials have drawn increasing attention in recent years?owing to?exceptional advantages such as high optical absorptivity, chemical stability, biocompatibility, and low toxicity.(Angew. Chem. Int. Ed., 2013, 52: 3953–3957)G.??On the basis of?measurements of the heat of immersion of water on zeolites, Tsutsumi etal. claimed that the surface consists of siloxane bondings and is hydrophobicin the region of low Al content. (Chem. Rev. 2002, 102, 3641?3666)H.?Nanoparticle spatial distributions might have a large significance for catalyst stability,?given that?metal particle growth is a relevant deactivation mechanism for commercial catalysts.?3. ...很重要A.?The inhibition of additional nucleation during growth, in other words, the complete separation?of nucleation and growth,?is?critical(essential, important)?for?the successful synthesis of monodisperse nanocrystals. (Nature Materials?3, 891 - 895 (2004))B.??In the current study,?Cys,?homocysteine?(Hcy) and?glutathione?(GSH) were chosen as model?thiol?compounds since they?play important (significant, vital, critical) roles?in many biological processes and monitoring of these?thiol?compounds?is of great importance for?diagnosis of diseases.(Chem. Commun., 2012,?48, 1147-1149)C.?This is because according to nucleation theory,?what really matters?in addition to the change in temperature ΔT?(or supersaturation) is the cooling rate.(Chem. Soc. Rev., 2014,?43, 2013-2026)(五)1. 相反/不同于A.?On the contrary,?mononuclear complexes, called single-ion magnets (SIM), have shown hysteresis loops of butterfly/phonon bottleneck type, with negligiblecoercivity, and therefore with much shorter relaxation times of magnetization. (Angew. Chem. Int. Ed., 2014, 53: 4413–4417)B.?In contrast,?the Dy compound has significantly larger value of the transversal magnetic moment already in the ground state (ca. 10?1?μB), therefore allowing a fast QTM. (Angew. Chem. Int. Ed., 2014, 53: 4413–4417)C.?In contrast to?the structural similarity of these complexes, their magnetic behavior exhibits strong divergence.?(Angew. Chem. Int. Ed., 2014, 53: 4413–4417)D.?Contrary to?other conducting polymer semiconductors, carbon nitride ischemically and thermally stable and does not rely on complicated device manufacturing. (Nature materials, 2009, 8(1): 76-80.)E.?Unlike?the spherical particles they are derived from that Rayleigh light-scatter in the blue, these nanoprisms exhibit scattering in the red, which could be useful in developing multicolor diagnostic labels on the basis not only of nanoparticle composition and size but also of shape. (Science 2001,? 294, 1901-1903)2. 发现,阐明,报道,证实可供选择的词包括:verify, confirm, elucidate, identify, define, characterize, clarify, establish, ascertain, explain, observe, illuminate, illustrate,demonstrate, show, indicate, exhibit, presented, reveal, display, manifest,suggest, propose, estimate, prove, imply, disclose,report, describe,facilitate the identification of?举例:A. These stacks appear as nanorods in the two-dimensional TEM images, but tilting experiments?confirm that they are nanoprisms.?(Science 2001,? 294, 1901-1903)B. Note that TEM?shows?that about 20% of the nanoprisms are truncated.?(Science 2001,? 294, 1901-1903)C. Therefore, these calculations not only allow us to?identify?the important features in the spectrum of the nanoprisms but also the subtle relation between particle shape and the frequency of the bands that make up their spectra.?(Science 2001,? 294, 1901-1903)D. We?observed?a decrease in intensity of the characteristic surface plasmon band in the ultraviolet-visible (UV-Vis) spectroscopy for the spherical particles at λmax?= 400 nm with a concomitant growth of three new bands of λmax?= 335 (weak), 470 (medium), and 670 nm (strong), respectively. (Science 2001,? 294, 1901-1903)E. In this article, we present data?demonstrating?that opiate and nonopiate analgesia systems can be selectively activated by different environmental manipulationsand?describe?the neural circuitry involved. (Science 1982, 216, 1185-1192)F. This?suggests?that the cobalt in CoP has a partial positive charge (δ+), while the phosphorus has a partial negative charge (δ?),?implying?a transfer of electron density from Co to P.?(Angew. Chem., 2014, 126: 6828–6832)3. 如何指出当前研究的不足A. Although these inorganic substructures can exhibit a high density of functional groups, such as bridging OH groups, and the substructures contribute significantly to the adsorption properties of the material,surprisingly little attention has been devoted to?the post-synthetic functionalization of the inorganic units within MOFs. (Chem. Eur. J., 2013, 19: 5533–5536.)B.?Little is known,?however, about the microstructure of this material. (Nature Materials 2013,12, 554–561)C.?So far, very little information is available, and only in?the absorber film, not in the whole operational devices. (Nano Lett.,?2014,?14?(2), pp 888–893)D.?In fact it should be noted that very little optimisation work has been carried out on?these devices. (Chem. Commun., 2013,?49, 7893-7895)E. By far the most architectures have been prepared using a solution processed perovskite material,?yet a few examples have been reported that?have used an evaporated perovskite layer. (Adv. Mater., 2014, 27: 1837–1841.)F. Water balance issues have been effectively addressed in PEMFC technology through a large body of work encompassing imaging, detailed water content and water balance measurements, materials optimization and modeling,?but very few of these activities have been undertaken for?anion exchange membrane fuel cells,? primarily due to limited materials availability and device lifetime. (J. Polym. Sci. Part B: Polym. Phys., 2013, 51: 1727–1735)G. However,?none of these studies?tested for Th17 memory, a recently identified T cell that specializes in controlling extracellular bacterial infections at mucosal surfaces. (PNAS, 2013,?111, 787–792)H. However,?uncertainty still remains as to?the mechanism by which Li salt addition results in an extension of the cathodic reduction limit. (Energy Environ. Sci., 2014,?7, 232-250)I.?There have been a number of high profile cases where failure to?identify the most stable crystal form of a drug has led to severe formulation problems in manufacture. (Chem. Soc. Rev., 2014,?43, 2080-2088)J. However,?these measurements systematically underestimate?the amount of ordered material. ( Nature Materials 2013, 12, 1038–1044)(六)1.?取决于a.?This is an important distinction, as the overall activity of a catalyst will?depend on?the material properties, synthesis method, and other possible species that can be formed during activation.?(Nat. Mater.?2017,16,225–229)b.?This quantitative partitioning?was determined by?growing crystals of the 1:1 host–guest complex between?ExBox4+?and corannulene. (Nat. Chem.?2014,?6177–178)c.?They suggested that the Au particle size may?be the decisive factor for?achieving highly active Au catalysts.(Acc. Chem. Res.,?2014,?47, 740–749)d.?Low-valent late transition-metal catalysis has?become indispensable to?chemical synthesis, but homogeneous high-valent transition-metal catalysis is underdeveloped, mainly owing to the reactivity of high-valent transition-metal complexes and the challenges associated with synthesizing them.?(Nature2015,?517,449–454)e.?The polar effect?is a remarkable property that enables?considerably endergonic C–H abstractions?that would not be possible otherwise.?(Nature?2015, 525, 87–90)f.?Advances in heterogeneous catalysis?must rely on?the rational design of new catalysts. (Nat. Nanotechnol.?2017, 12, 100–101)g.?Likely, the origin of the chemoselectivity may?be also closely related to?the H?bonding with the N or O?atom of the nitroso moiety, a similar H-bonding effect is known in enamine-based nitroso chemistry. (Angew. Chem. Int. Ed.?2014, 53: 4149–4153)2.?有很大潜力a.?The quest for new methodologies to assemble complex organic molecules?continues to be a great impetus to?research efforts to discover or to optimize new catalytic transformations. (Nat. Chem.?2015,?7, 477–482)b.?Nanosized faujasite (FAU) crystals?have great potential as?catalysts or adsorbents to more efficiently process present and forthcoming synthetic and renewablefeedstocks in oil refining, petrochemistry and fine chemistry. (Nat. Mater.?2015, 14, 447–451)c.?For this purpose, vibrational spectroscopy?has proved promising?and very useful.?(Acc Chem Res. 2015, 48, 407–413.)d.?While a detailed mechanism remains to be elucidated and?there is room for improvement?in the yields and selectivities, it should be remarked that chirality transfer upon trifluoromethylation of enantioenriched allylsilanes was shown. (Top Catal.?2014,?57: 967.?)e.?The future looks bright for?the use of PGMs as catalysts, both on laboratory and industrial scales, because the preparation of most kinds of single-atom metal catalyst is likely to be straightforward, and because characterization of such catalysts has become easier with the advent of techniques that readily discriminate single atoms from small clusters and nanoparticles. (Nature?2015, 525, 325–326)f.?The unique mesostructure of the 3D-dendritic MSNSs with mesopore channels of short length and large diameter?is supposed to be the key role in?immobilization of active and robust heterogeneous catalysts, and?it would have more hopeful prospects in?catalytic applications. (ACS Appl. Mater. Interfaces,?2015,?7, 17450–17459)g.?Visible-light photoredox catalysis?offers exciting opportunities to?achieve challenging carbon–carbon bond formations under mild and ecologically benign conditions. (Acc. Chem. Res.,?2016, 49, 1990–1996)3. 因此同义词:Therefore, thus, consequently, hence, accordingly, so, as a result这一条比较简单,这里主要讲一下这些词的副词词性和灵活运用。
Computation of interprocedural control dependence
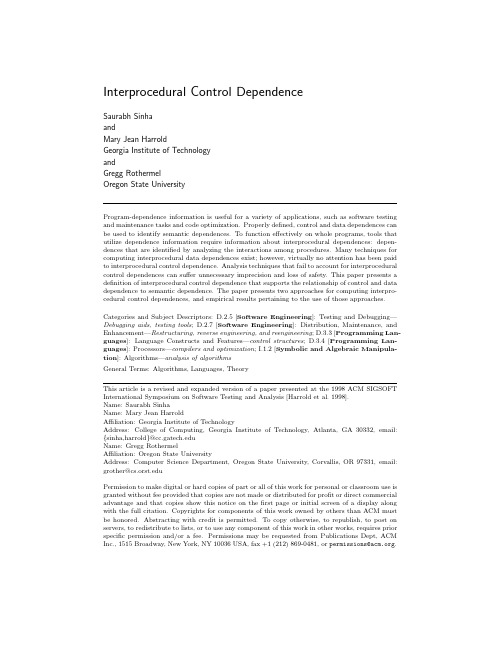
Interprocedural Control DependenceSaurabh SinhaandMary Jean HarroldGeorgia Institute of TechnologyandGregg RothermelOregon State UniversityThis article is a revised and expanded version of a paper presented at the1998ACM SIGSOFT International Symposium on Software Testing and Analysis[Harrold et al.1998].Name:Saurabh SinhaName:Mary Jean HarroldAffiliation:Georgia Institute of TechnologyAddress:College of Computing,Georgia Institute of Technology,Atlanta,GA30332,email: {sinha,harrold}@Name:Gregg RothermelAffiliation:Oregon State UniversityAddress:Computer Science Department,Oregon State University,Corvallis,OR97331,email: grother@Permission to make digital or hard copies of part or all of this work for personal or classroom use is granted without fee provided that copies are not made or distributed for profit or direct commercial advantage and that copies show this notice on thefirst page or initial screen of a display along with the full citation.Copyrights for components of this work owned by others than ACM must be honored.Abstracting with credit is permitted.To copy otherwise,to republish,to post on servers,to redistribute to lists,or to use any component of this work in other works,requires prior specific permission and/or a fee.Permissions may be requested from Publications Dept,ACM Inc.,1515Broadway,New York,NY10036USA,fax+1(212)869-0481,or permissions@.2·S.Sinha et al.Additional Key Words and Phrases:Interprocedural control dependence,interprocedural analysis, semantic dependence,program slicing,software maintenanceInterprocedural Control Dependence·3 and their potential effects on interprocedural analysis techniques.The main con-tributions of the paper are:—A description of several ways in which control dependences computed intraproce-durally inaccurately model the control dependences that exist in whole programs.—A precise definition of interprocedural control dependence.Unlike the previously presented definition[Loyall and Mathisen1993],this definition supports the re-lationship between syntactic and semantic dependence[Podgurski and Clarke 1990]that must hold if analyses based on dependence information are to model conservatively the semantic dependences in programs.—Two approaches for computing interprocedural control dependences:one ap-proach computes precise interprocedural control dependences but may be inor-dinately expensive;the other approach summarizes control dependences,and efficiently obtains a conservative(safe)estimate of those dependences at the cost of some precision.The paper provides empirical results pertaining to the effec-tiveness and efficiency of these approaches.The remainder of this paper is organized as follows.The next section provides background information necessary to support our definition of interprocedural con-trol dependence.Section3demonstrates several effects related to interprocedural control dependence,and then provides our definition of interprocedural control de-pendence.Section4presents our algorithms for calculating interprocedural control dependence,and Section5presents empirical results obtained in the use of the second algorithm.Section6reviews related work,and illustrates the drawbacks of the existing definition of interprocedural control dependence.Finally,Section7 presents conclusions and outlines possible future work.2.BACKGROUNDTo demonstrate the semantic basis for uses of program dependences,and to evaluate some of those uses,Podgurski and Clarke[Podgurski and Clarke1990]present a for-mal model of program dependences.They distinguish several types of control and data dependences,and describe conditions under which identification of such(syn-tactic)dependences may or may not imply identification of semantic dependences (cases where the behavior of a statement can indeed affect the execution behavior of another statement).Their results show that a maintenance tool,such as a slicer, that uses control and data dependences to identify a superset of the statements that could semantically affect another statement,can omit semantic dependences if it utilizes inappropriate definitions or computations of data-or control-dependence information.Our definition of interprocedural control dependence builds on this previous work. This section presents definitions drawn directly from,or based on,those given in Reference[Podgurski and Clarke1990]that are prerequisite to that definition. Control dependences are typically defined in terms of control-flow graphs,paths in those graphs,and the postdominance relation.Definition1.A control-flow graph(CFG)G=(N,E)for procedure P is a di-rected graph in which N contains one node for each statement in P,and E contains edges that represent possibleflow of control between statements in P.N contains4·S.Sinha et al.two distinguished nodes,n e and n x,representing entry to and exit from P,re-spectively,where n e has no predecessors,and n x has no successors.If P contains multiple exit points,E contains an edge from each node that represents an exit point to n x.Each call site in P is represented by a call node and a return node in G,and there is an edge from each call node to its associated return node.Each node in N is reachable from n e,and n x is reachable from each node in N.Each node in N that represents a predicate statement is called a predicate node and has exactly two successors;all other nodes in N except n x have exactly one successor. Definition2.An n1–n k path in a CFG G=(N,E)is a sequence of nodes W= n1,n2,...,n k such that k≥0,and such that,if k≥2,then for i=1,2,...,k−1, (n i,n i+1)∈E.1Definition3.Let G=(N,E)be a CFG.A node u∈N postdominates a node v∈N if and only if every v–n x path in G contains u.Several forms of control dependence have been identified in the research literature. We restrict our attention to the form of control dependence found most commonly in the literature,described as“control dependence”[Ferrante et al.1987],as“direct, strong control dependence”[Podgurski and Clarke1990],and as“classical control dependence”[Bilardi and Pingali1996].Definition4.Let G=(N,E)be a CFG,and let u,v∈N.Node u is control dependent on node v if and only if v has successors v and v such that u postdom-inates v but u does not postdominate v .For control-dependence computation,a CFG G is augmented with a unique pred-icate node n s,and edges(n s,n e),labeled‘true’,and(n s,n x),labeled‘false’[Ferrante et al.1987].By this mechanism,nodes in G that are not control dependent on any predicate nodes are control dependent on entry to the procedure.The following definitions extend the CFG to model data elements,and use this extension to define data dependence.Definition5.A def/use graph is a quadruple G du=(G,Σ,D,U),where G= (N,E)is a CFG,Σis afinite set of symbols called variables,and D:N→P(Σ), U:N→P(Σ)are functions.Definition6.Let G du=(G,Σ,D,U)be a def/use graph with G=(N,E),and let u,v∈N.Node u is data dependent on node v if and only if there exists a path vW uD(n i). in G du such that(D(v)∩U(u))−D(W)=φ,where D(W)=∪ni∈W(n i/∈{u,v})The next definition captures the notion that two nodes in a def/use graph may be connected by a chain of data and control dependences,resulting in a syntactic dependence.Definition7.Let G du=(G,Σ,D,U)be a def/use graph with G=(N,E),and let u,v∈N.Node u is syntactically dependent on node v if and only if there is a sequence n1,n2,...,n k of nodes,k≥2,such that v=n1,u=n k,and forInterprocedural Control Dependence·5 i=1,2,...,k−1either n i+1is control dependent on n i or n i+1is data dependent on n i.2Podgurski and Clarke[Podgurski and Clarke1990]define semantic dependence, and relate it to syntactic rmally,when the semantics of statement s may affect the execution of statement s ,s is semantically dependent on s.A more formal definition is based on notions of interpretations,computation sequences,and execution histories,defined as follows[Podgurski and Clarke1990].Definition8.Let G du=(G,Σ,D,U)be a def/use graph with G=(N,E).An interpretation of G du is an assignment of partial computable functions to the ver-tices of G du.The function assigned to a vertex v∈N is the function computed by the program statement that v represents;it maps values for the variables in U(v) to values for the variables in D(v)or,if v is a decision vertex,to a successor of v. Definition9.A computation sequence of a program is the sequence of states (pairs consisting of a statement and a function assigning values to all the variables in the program)induced by executing the program with a particular input.Definition10.Let G du=(G,Σ,D,U)be a def/use graph with G=(N,E). An execution history of a vertex v∈N is the sequence whose i th element is the assignment of values held by the variables of U(v)just before the i th time v is visited during a computation.Given these definitions,Podgurski and Clarke[Podgurski and Clarke1990]define semantic dependence as follows:Definition11.A node u in a def/use graph G du is semantically dependent on a node v in G du if there are interpretations I1and I2of G du that differ only in the function assigned to v,such that for some input,the execution history of u induced by I1differs from that induced by I2.Semantic dependence of u on v can be demonstrated in either of two ways:(1) if for some pair of interpretations,the execution histories of u differ in some pair of corresponding entries,or(2)if for some pair of interpretations,the execution histories of u have different lengths.When case(1)holds,or when case(2)holds with respect tofinite portions of computations,the semantic dependence is said to befinitely demonstrated:this necessarily occurs when programs halt,but can also occur for non-halting programs.There is no algorithm to determine,for arbitrary statements s and s ,whether s is semantically dependent on s;however,Podgurski and Clarke[Podgurski and Clarke1990]demonstrate that given appropriate definitions of control and data dependence,there exist useful relationships between syntactic and semantic depen-dence.In this paper,we restrict our attention to the relationship stated in the following theorem:36·S.Sinha et al.begin M procedure M 1procedure B begin B 9begin C 16procedure C Fig.1.Program Sum with alternative versions of two of its statements.Theorem 1.Let G du =(G,Σ,D,U )be a def/use graph with G =(N,E ),and let u,v ∈N .If u is semantically dependent on v and this semantic dependence is finitely demonstrated,then u is syntactically dependent on v .4Theorem 1is significant because it shows that,given appropriate definitions of control and data dependence,syntactic dependence is a necessary condition for (finitely demonstrated)semantic dependence.Thus,the theorem provides justifi-cation for algorithms that use syntactic dependence to approximate semantic de-pendence.We refer to this desirable relationship between syntactic and semantic dependence as the syntactic –semantic relationship .53.INTERPROCEDURAL CONTROL DEPENDENCEIn this section,we illustrate three effects that impact interprocedural control de-pendences.We then define interprocedural control dependence.3.1Effects that impact interprocedural control dependencesFig.1presents a program Sum that consists of three procedures:M (the entry procedure),B ,and C .The two insets in the figure provide alternative versions of two lines of the program;we use these alternatives to illustrate specific points.Intraprocedural control-dependence analysis operates independently on individual procedures,ignoring both the context in which each procedure is invoked,and the side-effects on control dependence that may be caused by a called procedure.Table 1illustrates the intraprocedural control dependences for Sum .Considering Sum as a whole,however,we can observe three ways in which control dependences that are computed intraprocedurally inaccurately model the semantic dependences that exist between statements in the program.Interprocedural Control Dependence·7 Table1.Intraprocedural control dependences for Sum.ControlStatements Dependent Onentry M4,5entry B12,13entry C188·S.Sinha et al.Table2.Interprocedural control dependences for Sum.ControlStatements Dependent Onentry M5,6,10,171712,13Number ofProgramsAristotle10Eli11Empire1GCC19Omega1Siemens3XVCG1736See /projects/omega/omega.html for information about the Omega project.Interprocedural Control Dependence·9 in main we were able to exclude“non-embedded”exit()statements,used uncon-ditionally at the ends of the programs,that cannot affect control dependences.)For programs that Aristotle could not completely analyze,we determined this information by manual inspection of the source code.As Table3illustrates,over63%of the programs we examined,and at least42%of the programs in each groupof programs,contained exit()statements.Although further study is necessary to determine the extent to which these results generalize,the results do supporthypotheses that(1)in C programs,embedded halts are used frequently,and(2) this frequent use is consistent over a range of programs.Embedded halts are not the only cause of return-dependence effects.Otherlanguage constructs,such as setjump–longjump statements in C,and exception-handling constructs in Java and C++,also cause these effects.In this paper,we restrict our attention to embedded halts.3.2Definition of interprocedural control dependenceThe entry-dependence,multiple-context,and return-dependence effects constitutethree ways in which intraprocedural control dependence computation fails to pre-serve the syntactic–semantic relationship with respect to control dependences for whole programs.Other effects may also exist.To preserve the syntactic–semanticrelationship,a definition of interprocedural control dependence must account forall such effects;this section provides such a definition.Our definition relies on an interprocedural inlinedflow graph(IIFG).An IIFG isthe graph,possibly infinite,that results when we inline all procedures at their callsites and construct a controlflow graph from the resulting program;as such,an IIFG represents the controlflow in a program that has been rolled out[Binkley1992].Like the invocation graph[Emami et al.1994]and the context graph[Atkinsonand Griswold1996],the IIFG is fully context-sensitive:it accounts for the calling sequence that leads to each call.However,unlike the invocation and context graphs,the IIFG is alsoflow-sensitive:it accounts for the controlflow of the individualprocedures.We define the IIFG more formally as follows:Definition12.Let P be a program,and letΓbe a collection of CFGs,G k,k>0,that contains,for each procedure P i,i>0,in P,one copy of the CFG for eachcall site in P that calls P i.Furthermore,let E be the set of edges and N be the set of nodes in the G k,k>0.An interprocedural inlinedflow graph(IIFG) G I=(N I,E I)for P is a directed graph:N I=N∪{n stop},E I=(E−CR−HX)∪CE∪XR∪HS;n stop is a unique node that represents exit from P;CR is the set of edges from call nodes to the corresponding return nodes;HX isthe set of edges from nodes that represent embedded halts to the exit nodes of the respective CFGs;CE is the set of edges from call nodes to the entry nodes of the G k;XR is the set of edges from the exit nodes of the G k to the return nodes;and HS is the set of edges from nodes that represent embedded halts to n stop.Note that a given statement in P corresponds to a set of IIFG nodes—one for eachcalling context in which the statement can be executed.We denote the set of nodes in G I to which a given statement s in P corresponds by NodeSet(s).Fig.2depicts the IIFG for program Sum.Each call site is represented by calland return nodes;the CFG for the called procedure is inlined at each call node.10·S.Sinha et al.Fig.2.Interprocedural inlinedflow graph for program Sum.The CFGs are connected by(call node,entry node)and(exit node,return node) edges,shown as dashed lines.The IIFG in Fig.2contains two copies of the CFG for procedure B,corresponding to call nodes5a and6a;each inlined CFG for B contains a call to C,and therefore the IIFG contains two copies of the CFG for C as well.Nodes from which control can exit the program(nodes8and18)are connected to a unique exit node.The definitions of paths,postdominance,control dependence,def/use graphs, data dependence,syntactic dependence,and semantic dependence presented in Sec-tion2apply to IIFGs as follows:Definition13.A path in an IIFG G I=(N I,E I)is a sequence of nodes W= n1,n2,...,n k,such that k≥0,and such that,if k≥2,then for i=1,2,...,k−1, (n i,n i+1)∈E I.Definition14.Let G I=(N I,E I)be an IIFG.A node u∈N I postdominates a node v∈N I if and only if every v–n stop path in G I contains u.Definition15.Let G I=(N I,E I)be an IIFG,and let u,v∈N I.Node u is control dependent on node v if and only if v has successors v and v such that u postdominates v but u does not postdominate v .Definition16.A def/use IIFG is a quadruple G I−du=(G I,Σ,D,U),where G I= (N I,E I)is an IIFG,Σis afinite set of symbols called variables,and D:N I→P(Σ),U:N I→P(Σ)are functions.Definition17.Let G I−du=(G I,Σ,D,U)be a def/use IIFG with G I=(N I,E I), and let u,v∈N I.Node u is data dependent on node v if and only if there existsInterprocedural Control Dependence·11 a path vW u in G I−du such that(D(v)∩U(u))−D(W)=φ,where D(W)=∪nD(n i).i∈W(n i/∈{u,v})Definition18.Let G I−du=(G I,Σ,D,U)be a def/use IIFG with G I=(N I,E I), and let u,v∈N I.Node u is syntactically dependent on node v if and only if there is a sequence n1,n2,...,n k of nodes,k≥2,such that v=n1,u=n k,and for i=1,2,...,k−1either n i+1is control dependent on n i or n i+1is data dependent on n i.Definition19.Let G I−du=(G I,Σ,D,U)be a def/use IIFG with G I=(N I,E I). An interpretation of G I−du is an assignment of partial computable functions to the vertices of G I−du.The function assigned to a vertex v∈N I is the function com-puted by the program statement that v represents;it maps values for the variables in U(v)to values for the variables in D(v)or,if v is a decision vertex,to a successor of v.Definition20.Let G I−du=(G I,Σ,D,U)be a def/use IIFG with G I=(N I,E I). An execution history of a vertex v∈N I is the sequence whose i th element is the assignment of values held by the variables of U(v)just before the i th time v is visited during a computation.Definition21.A node u in a def/use IIFG G I−du is semantically dependent on a node v in G I−du if there are interpretations I1and I2of G I−du that differ only in the function assigned to v,such that for some input,the execution history of u induced by I1differs from that induced by I2.Given these definitions,the following theorem holds:Theorem 2.Let G I−du=(G I,Σ,D,U)be a def/use IIFG with G I=(N I,E I), and let u,v∈N I.If u is semantically dependent on v and this semantic dependence isfinitely demonstrated,then u is syntactically dependent on v.The proof of the theorem follows from Podgurski and Clarke’s proof of Theorem 1,and the relationship between the graphs used by Podgurski and Clarke in that proof and the IIFG.See Appendix A for further discussion.Given this theorem,the syntactic–semantic relationship holds for the IIFG-based definition of control dependence(Definition15).The theorem is significant for reasons similar to those that render Theorem1significant:it asserts that given appropriate definitions of control and data dependence,syntactic dependence is a necessary condition for(finitely demonstrated)semantic dependence.However, Theorem2applies in the interprocedural context,and thus,provides justification for(and a measure of success of)interprocedural algorithms that use syntactic dependence to approximate semantic dependence.PUTING INTERPROCEDURAL CONTROL DEPENDENCESIn this section,we present two approaches to computing interprocedural control dependences.Thefirst approach computes precise interprocedural control depen-dences,but may be inordinately expensive.The second approach summarizes in-terprocedural control dependences,and computes a conservative estimate of those dependences more efficiently than thefirst approach,but at the cost of some pre-cision.12·S.Sinha et al.Table4.Programs used for the empirical studies reported in the paper.Subject LOC armenu5835Interprocedural regression test selector[Rothermel and Harrold1997]diff1447 Lexical analyzer generatormpegplayer5380 3-D maze combat gamespace5889 Zipfile extract utility7This set of programs differs from the set we used for our study of the occurrence of embedded halts,reported in Table3.The objective of the embedded-halt study was to motivate our research, and the study required only limited processing of the programs.Thus,we were able to examine a large number of programs and conclude that embedded halts do occur often in practice.However, the empirical studies reported in this section required extensive processing of the programs with our prototype tools,and examination of the results for correctness.Thus,we selected a subset of the programs for study.Future work includes more experimentation with additional subjects, including those from Table3.Interprocedural Control Dependence ·13Table 5.IIFG sizes for programs with each recursion expanded once.Increase per Recursion ExpansionCFGs CFGs CFGs931642515444dejavu 34856872–41232–flex 37281092898580310518528–netmaze 458515602–1361552–unzip 20386074270u n z i p f l e x d e j a v u a r m e n u s p a c e d i f f n et m a z e m p e g p l a y e r I I F G n o d e s / C F G n o d e s Fig.3.Factors of increase in IIFG sizes over the sizes of the CFGs.programs,mpegplayer exhibits the largest increase in size—by a factor of 59—from 4,705nodes in the CFGs to 278,267nodes in the IIFG.These results suggest that using the IIFG and a traditional algorithm [Bilardi and Pingali 1996;Cytron et al.1991;Ferrante et al.1987;Pingali and Bilardi 1997]to compute interprocedural dependences for whole programs may be inordi-nately expensive.This expense must be weighed,in practice,against the precision requirements of particular applications.Nevertheless,it seems reasonable to seek alternative approaches to computing interprocedural control dependences,that sac-rifice precision for efficiency,while remaining conservative in that they do not omit interprocedural dependences that do exist in a program.We next present one such approach.4.2Efficient,safe computation of interprocedural control dependencesThe IIFG-based approach just presented computes interprocedural control depen-dences between nodes in the IIFG.A program statement may correspond to several nodes in an IIFG—one node for each calling context in which the procedure con-taining the statement can execute.Therefore,the IIFG-based approach computes distinct control dependences for each calling context in which a statement can ex-ecute;we call such dependences context-based interprocedural control dependences .An alternative approach to computing interprocedural control dependences is to14·S.Sinha et al.ignore the context-based distinctions and,instead,compute those dependences by summarizing the control dependences that exist in at least one calling context of execution of a statement;we call such dependences statement-based interprocedural control dependences.A precise definition of statement-based interprocedural control dependence,in terms of IIFG nodes and the NodeSet function,is as follows:Definition22.Let P be a program,let G I be the IIFG for P,and let s1and s2 be statements in P.Statement s1is control dependent on statement s2if and only if there exist nodes u,v∈G I such that u∈NodeSet(s1),v∈NodeSet(s2),and u is control dependent on v.Because statement-based control dependences summarize the control dependences that exist in different calling contexts,they do not encode control-dependence in-formation as precisely as do context-based control dependences.However,because context-based control dependences,defined on the IIFG,preserve the syntactic–semantic relationship,statement-based control dependences,which summarize those control dependences,also preserve that relationship.Theorem 3.Statement-based control dependences preserve the syntactic–semantic relationship.Proof.The proof requires a definition of what it means for a statement to be semantically or syntactically dependent on another rmally,we say such a dependence exists if it exists in some calling context.More formally,we say that a statement s1∈P is(semantically/syntactically)dependent on another statement s2∈P if and only if there exist nodes n1,n2∈G I−du,the def/use IIFGfor P,such that n1∈NodeSet(s1)and n2∈NodeSet(s2),and such that n1is (semantically/syntactically)dependent on n2.The proof then proceeds as follows. Suppose s1is semantically dependent on s2.Then there exist nodes n1,n2∈G I−du, the def/use IIFG for P,such that n1∈NodeSet(s1)and n2∈NodeSet(s2),and such that n1is semantically dependent on n2.But then,by Theorem2,n1is syntactically dependent on n2;thus,s1is syntactically dependent on s2.Given Definition22,it follows that we could compute statement-based interpro-cedural control dependences byfirst computing context-based control dependences on the IIFG,and then transforming them into statement-based control dependences using the NodeSet associations.Such an approach,of course,would be more expen-sive than simply computing context-based control dependences.A more efficient algorithm exists,however,that does not require an IIFG.This algorithm uses a representation that is linear in the size of a program to compute precisely the same statement-based interprocedural control dependences that would be computed us-ing the IIFG.4.2.1The algorithm.The algorithm proceeds in two phases:(1)Phase1iden-tifies call sites to which control may not return due to the presence of embedded halts,and uses this information to compute partial control dependences and con-struct an augmented control-dependence graph for each procedure;(2)Phase2 connects the augmented control-dependence graphs for the procedures to construct an interprocedural control-dependence graph for the program,and traverses the graph to compute interprocedural control dependences.Interprocedural Control Dependence·15Definition23.Let G be a CFG for procedure P in P,let N be the set of nodes in G,let E be the set of edges in G,let CN={CN1,CN2,...,CN j}be nodes in G that represent call sites where control may not return from the called procedures due to the presence of embedded halts,and let RN be the set of return nodes associated with the call nodes in CN.An augmented control-flow graph(ACFG) G A=(N A,E A)is a directed graph:N A=N∪{n sx}∪RP;E A=(E−CR−HX)∪CP∪P E∪SX;n sx is a unique super-exit node that represents all potential exits from P;RP is a set of return-predicate nodes,one for each call node in CN,that represent predicates that are external to P and affect the control dependences of statements in P;CR is the set of edges from each call node in CN to its corresponding return node;HX is the set of edges from nodes that represent embedded halts to the exit node;CP is a set of edges,one from each node n∈CN to the node in RP associated with n;P E is a set of edges,one labeled‘T’from each node n∈RP to the node in RN associated with n,and one labeled‘F’from each node n∈RP to n sx;and SX is a set of edges that connect the exit node and16·S.Sinha et al.Table6.Partial control dependences for Sum computed using the ACFGs.ControlStatements Dependent Onentry M4,5b46b,7,8entry B10b,111116,1717。
- 1、下载文档前请自行甄别文档内容的完整性,平台不提供额外的编辑、内容补充、找答案等附加服务。
- 2、"仅部分预览"的文档,不可在线预览部分如存在完整性等问题,可反馈申请退款(可完整预览的文档不适用该条件!)。
- 3、如文档侵犯您的权益,请联系客服反馈,我们会尽快为您处理(人工客服工作时间:9:00-18:30)。
a r X i v :p h y s i c s /0504105v 1 [p h y s i c s .a t o m -p h ] 15 A p r 2005DEPENDENCE OF SHAKE PROBABILITY ON NUCLEAR CHARGE IN Li-,Na-AND K-LIKE IONSA.Kupliauskien˙e a 1,K.Glemˇz a baVilnius University Institute of Theoretical Physics and Astronomy,A.Goˇs tauto 12,LT-01108Vilnius,LithuaniabVilnius University,Saul˙e tekio 9,LT-10222Vilnius,LithuaniaAbstractIn sudden perturbation approximation,the probability of the shake-up process accompanying inner-shell ionization is calculated for the isoelectronic sequences of Li-,Na-and K-like ions in the ground and excited n p and n d states.Numerical solutions of Hartree-Fock equations and hydrogen-like radial orbitals are used.Very large differences between the results of both approximations for all ions and strong dependences on ion charge are obtained at the beginning of the isoelectronic sequences.PACS :34.80.HdKeywords :Auger decay and inner-shell excitation or ionization1.IntroductionA shake process accompanies inner-shell ionization of atoms and ions.It usually effects photoelectron [1,2]and Auger electron [3,4,5]spectra.In the case of shake-up or shake-offprocess,it describes the excitation [6]or ionization [7]of the second electron,respectively.The shake probability describes the relaxation of passive electrons and is also important for the single electron ionization cross section.It was obtained by Kupliauskien˙e [8,9,10]that the shake probability should be taken into account even in the case of inner-shell ionization when spectator electrons saved their initial states.Then it describes the probability of spectator electrons to stay in their initial states.A strong increase of the relative intensity of shake-up satellites in the inner-shell photoioniza-tion of excited Na atoms [2]was explained by the change of the localization of valence electronin thefinal state with respect to that in the inital state[11,8,9].Very strong dependence of the calculated inner-shell ionization cross sections of alkaline atoms on the excited valence electron state was noticed not only in the case of photoionization[8,9,12]but also for the ionization of atoms by electrons[13,14,15].For neutral atoms and singly charged ions of the second and third rows,the probability of shake process accompanying inner-shell ionization was calculated in[16], and very strong dependence on valence electron state was obtained.Enormous differences were found for low excited ns and np states between shake probabilities that were calculated using numerical solutions of Hartree-Fock equations(HF)and hydrogen-like(H-like)radial orbitals [16].A simple two-parameter interpolation function for Z-dependences of shake-up probabilities per electron was presented by Kochur et al[17]for atoms in the ground state.The aim of the present work is the investigation of the dependence of shake probability for Li-,Na-and K-like ions on the ion charge and the state of valence electron because of the very strong sensitivity of the inner-shell ionization cross sections of Li-,Na-and K-like atoms on the valence electron state[8,9,12,15].The regularities of the shake probability passing from atoms to highly charged ions of these isoelectronic sequences are investigated.The calculated shake probabilities can be used for searching strong relaxation effects in processes dealing with inner-shell ionization of excited atoms and ions by photons and electrons as well as for the Auger decay calculations in sudden perturbation approximation.2.Description of calculationsThe ionizing transitionA N+(nl4l+2n′l′)+α→A(N+1)+(nl4l+1n′′l′)+e−(1)is investigated in the present work.Here nl=1s,2p,3p for Li-,Na-and K-like ions,respectively, n′=n+1for Li-and Na-like ions,n′=4for s-and p-electrons and n′=3,4for d-electrons of K-like ions,l′=0,1,2,n′′=n′,n′+1,N is the charge of an ion,andαstands for a particle or photon.The shake probability can be expressed as:P(i′→f)=| i′|f |2(2)where the wave function i′|describes the ion in the intermediate state following ionization,in which spectator electrons remain in their initial states,with the radial orbitals of an atom,and |f is the wave function of an ion in thefinal state.The calculations of shake probabilities(2)have been performed by using both numerical solutions of HF equations[18]obtained in the average-term approximation and hydrogen-like radial orbitals with effective nuclear charge Z=N+1and Z=N+2for ions in the initial and final states of the valence electron,respectively.2.Results and discussionThe shake probabilities for single electron ionization(SPI)and shake-up(SPS)transition were calculated for Li-,Na-and K-like ions in the configurations nl4l+2n′l′,l′=0,1,2,n′=n+1 for l′=0,1,and n′=n+2for Li-,n′=n+1for Na-and n′=n,n+1for K-like isoelectronic sequences in the case of d-electron.Thefinal state of the ionized ions is n′′=n′+1,n′+2. The values of the shake probabilities calculated by using both HF and H-like radial orbitals are presented in Fig.1–2for Li-and Na-like ions,respectively,and Table1for K-like ions. The values of shake probabilities for d-electrons calculated with H-like radial orbitals are not displayed in Fig.1–2because they are very close to those of HF approximation for Li-and Na-like ions.The results of Fig.1–2and Table1demonstrate that SPI are smaller for d-electrons than those of s-and p-electrons.They increase with the decrease of the orbital quantum number from l′=2down to l′=0.This tendency is independent of the charge of an ion.The values of SPI are much smaller than those at the beginning of all sequences investigated but rapidly increase up to one with growing ion charge N=9,N=8and N=6for Li-,Na-and K-like ions,respectively.At the beginning of each sequence under investigation the values of SPS are much larger than zero and decrease with increasing ion charge.The values of SPS become close to zero for ion charge a little less than the values of SPI which become close to one,i.e.8,7,and5for Li-, Na-and K-like ions,respectively.Thus,the values of SPS decrease more rapidly than those of SPI increase.The values both of SPI and SPS calculated by using H-like radial orbitals with effective ion charge differ very much from those of HF calculation not only at the beginning of isoelectronic sequences but also for highly charged ions.They are similar to each other only for d-electrons in the case of Li-and Na-like ions.In the case of Li-like ions,the differences between the results of both approximations are less in comparison with those of Na-and K-like ions.In the case of K-like ions,the H-like calculations can not be used for the evaluations of shake probabilities even for highly charged ions(see table1).The results of Table1show that the differences betweenthe values calculated with HF and H-like orbitals reach several times or even an order for both SPI and SPS probabilities.Calculated values of the ratios of SPS and SPI for Li-,Na-and K-like isoelectronic sequences are presented in Fig.3.Here white points in black background stand for the experimental ratios of shake-up and single electron photoionization cross sections in the case of Li[19],Na[2]and K [1]atoms.Experimental values of the ratio are chosen for large photon energies where sudden perturbation approximation is valid.The results from Fig.3show that the agreement between calculated ratios of shake proba-bilities and measured shake-up and single electron photoionization cross sections is good.The values of the rations decrease with increasing ion charge.The ratios for s-and p-electrons de-crease while the number of core electrons increases.But the ratios for d-electrons in the case of K-like ions are larger than those of Na-and Li-like sequences.The ratios are larger at the beginning of the sequences,however,their decrease is more rapid for Li-like ions than for K-like ions.References[1]D.Cubaynes,au,T.J.Morgan,B.Carre,F.J.Wuilleumier,in:Y.Petrov(Ed.),Tenth Int.Conf.on Vaccuum Ultraviolet Radiation Physics,Paris,1992,p.Tu79.[2]D.Cubaynes,au,F.J.Wuilleumier,B.Carre,F.Gounand,Phys.Rev.Lett.63(1989)2460.[3]M.Meyer,E.v.Raven,B.Sonntag,J.E.Hansen,Phys.Rev.A43(1991)177.[4]J.Mursu,H.Aksela,O.-P.Sairanen,A.Kivim¨a ki,E.N¨o mmiste,A.Ausmees,S.Svensson,S.Aksela,J.Phys.B:At.Mol.Opt.Phys.29(1996)4387.[5]S.B.Whitfield,J.Tulkki,T.Aberg,Phys.Rev.A44(1991)R6983.[6]T.Aberg,Phys.Rev.156,35(1967).[7]T.A.Carlson,M.O.Krause,Phys.Rev.137A1655(1965).[8]A.Kupliauskien˙e,J.Phys.B:At.Mol.Opt.Phys.27(1994)5647.[9]A.Kupliauskien˙e,Physica Scripta,53(1996)149.[10]A.Kupliauskien˙e,J.Phys.B:At.Mol.Opt.Phys.34(2001)345.[11]Z.Felfli,S.T.Manson,Phys.Rev.Lett.68(1992)1687.[12]N.Rakˇs tikas,A.Kupliauskien˙e,Physica Scripta,58(1998)587.[13]A.Dorn,O.I.Zatsarinny,W.Mehlhorn,J.Phys.B:At.Mol.Opt.Phys.30(1997)2975.[14]J.Nienhaus,O.I.Zatsarinny,A.Dorn,W.Mehlhorn,J.Phys.B:At.Mol.Opt.Phys.30(1997)3611.[15]N.Rakˇs tikas,A.Kupliauskien˙e,Physica Scripta,64(2001)230.[16]A.Kupliauskien˙e,K.Glemˇz a,J.Phys.B:At.Mol.Opt.Phys.35(2002)4637.[17]A.G.Kochur,A.I.Dudenko,D.Petrini,J.Phys.B:At.Mol.Opt.Phys.35(2002)395.[18]C.Froese Fischer,mun.64(1991)369.[19]J.Journel,B.Rouvellou,D.Cubaynes,au,F.J.Wuilleumier,in:T.Andersen,B.Fastup,F.Folkman,H.Knudsen(Eds.),Abstracts XVII Int.Conf.Physcs of Electronicand Atomic Collisions,Aarhus,1993,p.22.Table1.Shake probabilities(2)(SPI and SPS)calculated by using HF and H-like(H)radial orbitals with N+1and N+2efective nuclear charges(1)for K-like ions in the3p-electron ionization4s4s HF0.840.940.970.980.990.990.99H0.020.200.480.750.860.910.93 4s5s HF0.140.050.0270.0120.0070.0050.004H0.670.690.410.170.0850.0510.042 4p4p HF0.730.900.950.980.990.990.99H0.0030.250.530.780.880.920.94 4p5p HF0.270.090.0440.0180.0100.0060.005H0.690.630.370.150.0770.0470.038 3d3d HF0.160.900.980.99 1.00 1.00 1.00H0.440.750.860.940.970.980.98 3d4d HF0.680.080.0140.0030.0020.0010.001H0.540.240.120.050.0260.0160.013 4d4d HF0.0270.600.840.950.970.980.99H0.020.390.640.840.910.940.95 4d5d HF0.470.330.130.040.020.0120.01H0.700.510.290.120.060.0370.030Figure captions:Fig. 1.Shake probability vs nuclear charge for Li isoelectronic sequence in the case of the transition nl4l+2(n+1)l′→nl4l+1n′l′.Filled circles are for single electron ionization(SPI), diamonds are for shake-up process(SPS),solid line stands for l′=0,dashed line is for l′=1 and dotted line is for l′=2,respectively.Triangles-dot up show single electron and triangles-dot down indicate shake-up probabilities calculated using hydrogen-like radial orbitals with effective nuclear charge Z=N+1and Z=N+2(1)for the initial andfinal states,respectively,and dashed-dot and dashed-dot-dot lines are for l′=0and l′=1electrons,respectively.Fig.2.Shake probability vs nuclear charge for Na isoelectronic sequence.Details are as in Fig.1.Fig. 3.The ratios of the probability of shake-up to that of single electron transition for Li-(filled circles),Na-(squares)and K-like(diamonds)isoelectronic sequences.Solid,dashed and dotted lines are for s,p and d electrons,respectively.The white dots in black background are experimental values of the ratios between shake-up and single electron photoionization cross sections for Li[19],Na[2]and K[1]atoms.。