A Note on Tests for Nonlinearity in a Vector Time Series
测试的准确性英语作文

测试的准确性英语作文The Importance of Accuracy in Testing。
Testing is an essential part of any process that involves the evaluation of performance or quality. It is used to determine whether a product or service meets the required standards, and to identify any areas for improvement. However, for testing to be effective, it must be accurate. Inaccurate testing can lead to false conclusions, wasted resources, and even harm to individuals or society. Therefore, it is crucial that testing is conducted with the utmost care and precision.One of the main reasons why accuracy is so important in testing is that it ensures that the results are reliable. Reliable results are those that can be trusted to be consistent and repeatable, regardless of who is conducting the test or when it is being conducted. This isparticularly important in fields such as medicine, where inaccurate test results can have serious consequences forpatients' health and wellbeing. Inaccurate results can also lead to incorrect diagnoses, unnecessary treatments, and wasted resources.Another reason why accuracy is important in testing is that it helps to identify any potential errors or biases in the testing process. Errors can occur at any stage of the testing process, from the design of the test to the interpretation of the results. Biases can also creep in, particularly if the tester has a vested interest in the outcome of the test. Therefore, it is important to be aware of these potential sources of error and to take steps to minimize their impact.Finally, accuracy is important in testing because it helps to ensure that the results are meaningful. Meaningful results are those that provide useful information that can be used to make informed decisions or to improve thequality of a product or service. If the results of a test are inaccurate, then they are unlikely to provide any meaningful information. This can lead to a waste of resources and a missed opportunity to improve the qualityof the product or service.In conclusion, accuracy is essential in testing. It ensures that the results are reliable, helps to identify potential errors or biases, and ensures that the results are meaningful. Therefore, it is important to conduct testing with the utmost care and precision, and to take steps to minimize the impact of potential sources of error or bias. By doing so, we can ensure that testing is effective and provides useful information that can be used to improve the quality of products and services and to make informed decisions.。
音频测试参数
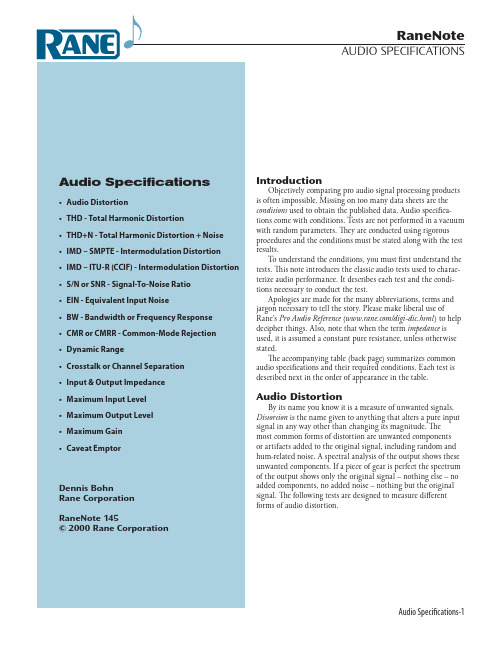
Audio Specifications-Audio Specifications• Audio Distortion• THD - Total Harmonic Distortion• THD+N - Total Harmonic Distortion + Noise • IMD – SMPTE - Intermodulation Distortion • IMD – ITU-R (CCIF) - Intermodulation Distortion • S/N or SNR - Signal-To-Noise Ratio • EIN - Equivalent Input Noise• BW - Bandwidth or Frequency Response • CMR or CMRR - Common-Mode Rejection • Dynamic Range• Crosstalk or Channel Separation • Input & Output Impedance • Maximum Input Level • Maximum Output Level • Maximum Gain • Caveat EmptorDennis BohnRane CorporationRaneNote 145© 2000 Rane CorporationIntroductionObjectively comparing pro audio signal processing products is often impossible. Missing on too many data sheets are the conditions used to obtain the published data. Audio specifica-tions come with conditions. Tests are not performed in a vacuum with random parameters. They are conducted using rigorous procedures and the conditions must be stated along with the test results.To understand the conditions, you must first understand the tests. This note introduces the classic audio tests used to charac-terize audio performance. It describes each test and the condi-tions necessary to conduct the test.Apologies are made for the many abbreviations, terms and jargon necessary to tell the story. Please make liberal use ofRane’s Pro Audio Reference (/digi-dic.html ) to help decipher things. Also, note that when the term impedance is used, it is assumed a constant pure resistance, unless otherwise stated.The accompanying table (back page) summarizes common audio specifications and their required conditions. Each test is described next in the order of appearance in the table.Audio DistortionBy its name you know it is a measure of unwanted signals. Distortion is the name given to anything that alters a pure input signal in any way other than changing its magnitude. The most common forms of distortion are unwanted components or artifacts added to the original signal, including random and hum-related noise. A spectral analysis of the output shows these unwanted components. If a piece of gear is perfect the spectrum of the output shows only the original signal – nothing else – no added components, no added noise – nothing but the original signal. The following tests are designed to measure different forms of audio distortion.THD. Total Harmonic DistortionWhat is tested? A form of nonlinearity that causes unwant-ed signals to be added to the input signal that are harmonically related to it. The spectrum of the output shows added frequency components at 2x the original signal, 3x, 4x, 5x, and so on, but no components at, say, 2.6x the original, or any fractional multi-plier, only whole number multipliers.How is it measured? This technique excites the unit with a single high purity sine wave and then examines the output for evidence of any frequencies other than the one applied. Perform-ing a spectral analysis on this signal (using a spectrum, or FFT analyzer) shows that in addition to the original input sine wave, there are components at harmonic intervals of the input fre-quency. Total harmonic distortion (THD) is then defined as the ratio of the rms voltage of the harmonics to that of the funda-mental component. This is accomplished by using a spectrum analyzer to obtain the level of each harmonic and performing an rms summation. The level is then divided by the fundamental level, and cited as the total harmonic distortion (expressed in percent). Measuring individual harmonics with precision is dif-ficult, tedious, and not commonly done; consequently, THD+N (see below) is the more common test. Caveat Emptor: THD+N is always going to be a larger number than just plain THD. For this reason, unscrupulous (or clever, depending on your viewpoint) manufacturers choose to spec just THD, instead of the more mean-ingful and easily compared THD+N.Required Conditions. Since individual harmonic ampli-tudes are measured, the manufacturer must state the test signal frequency, its level, and the gain conditions set on the tested unit, as well as the number of harmonics measured. Hopefully, it’s obvious to the reader that the THD of a 10 kHz signal at a +20 dBu level using maximum gain, is apt to differ from the THD of a 1 kHz signal at a -10 dBV level and unity gain. And more different yet, if one manufacturer measures two harmonics while another measures five.Full disclosure specs will test harmonic distortion overthe entire 20 Hz to 20 kHz audio range (this is done easily by sweeping and plotting the results), at the pro audio level of +4 dBu. For all signal processing equipment, except mic preamps, the preferred gain setting is unity. For mic pre amps, the standard practice is to use maximum gain. Too often THD is spec’d only at 1 kHz, or worst, with no mention of frequency at all, and nothing about level or gain settings, let alone harmonic count.Correct: THD (5th-order) less than 0.01%, +4 dBu, 20–20 kHz, unity gainWrong:THD less than 0.01%THD+N. Total Harmonic Distortion + NoiseWhat is tested? Similar to the THD test above, except instead of measuring individual harmonics this tests measures everything added to the input signal. This is a wonderful test since everything that comes out of the unit that isn’t the pure test signal is measured and included – harmonics, hum, noise, RFI, buzz – everything.How is it measured? THD+N is the rms summation ofall signal components (excluding the fundamental) over some prescribed bandwidth. Distortion analyzers make this measure-ment by removing the fundamental (using a deep and narrow notch filter) and measuring what’s left using a bandwidth filter (typically 22 kHz, 30 kHz or 80 kHz). The remainder contains harmonics as well as random noise and other artifacts.Weighting filters are rarely used. When they are used, too often it is to hide pronounced AC mains hum artifacts. An exception is the strong argument to use the ITU-R (CCIR) 468 curve because of its proven correlation to what is heard. However, since it adds 12 dB of gain in the critical midband (the whole point) it makes THD+N measurements bigger, so marketeers prevent its widespread use.[Historical Note: Many old distortion analyzers labeled “THD” actually measured THD+N.]Required Conditions. Same as THD (frequency, level & gain settings), except instead of stating the number of harmon-ics measured, the residual noise bandwidth is spec’d, along with whatever weighting filter was used. The preferred value is a 20 kHz (or 22 kHz) measurement bandwidth, and “flat,” i.e., no weighting filter.Conflicting views exist regarding THD+N bandwidth mea-surements. One argument goes: it makes no sense to measure THD at 20 kHz if your measurement bandwidth doesn’t include the harmonics. Valid point. And one supported by the IEC, which says that THD should not be tested any higher than 6 kHz, if measuring five harmonics using a 30 kHz bandwidth, or 10 kHz, if only measuring the first three harmonics. An-other argument states that since most people can’t even hear the fundamental at 20 kHz, let alone the second harmonic, thereis no need to measure anything beyond 20 kHz. Fair enough. However, the case is made that using an 80 kHz bandwidth is crucial, not because of 20 kHz harmonics, but because it reveals other artifacts that can indicate high frequency problems. All true points, but competition being what it is, standardizing on publishing THD+N figures measured flat over 22 kHz seems justified, while still using an 80 kHz bandwidth during the design, development and manufacturing stages.Correct: THD+N less than 0.01%, +4 dBu, 20–20 kHz, unity gain, 20 kHz BWWrong:THD less than 0.01%IMD – SMPTE. Intermodulation Distortion – SMPTE MethodWhat is tested? A more meaningful test than THD, inter-modulation distortion gives a measure of distortion products not harmonically related to the pure signal. This is important since these artifacts make music sound harsh and unpleasant.Intermodulation distortion testing was first adopted in the U.S. as a practical procedure in the motion picture industry in 1939 by the Society of Motion Picture Engineers (SMPE – no “T” [television] yet) and made into a standard in 1941.How is it measured? The test signal is a low frequency (60 Hz) and a non-harmonically related high frequency (7 kHz) tone, summed together in a 4:1 amplitude ratio. (Other frequencies and amplitude ratios are used; for example, DIN favors 250 Hz & 8 kHz.) This signal is applied to the unit, and the output signal is examined for modulation of the upper frequency by the low frequency tone. As with harmonic distortion measurement, this is done with a spectrum analyzer or a dedicated intermodulationAudio Specifications-distortion analyzer. The modulation components of the upper signal appear as sidebands spaced at multiples of the lower fre-quency tone. The amplitudes of the sidebands are rms summed and expressed as a percentage of the upper frequency level.[Noise has little effect on SMPTE measurements because the test uses a low pass filter that sets the measurement bandwidth, thus restricting noise components; therefore there is no need for an “IM+N” test.]Required Conditions. SMPTE specifies this test use 60 Hz and 7 kHz combined in a 12 dB ratio (4:1) and that the peak value of the signal be stated along with the results. Strictly speaking, all that needs stating is “SMPTE IM” and the peak value used. However, measuring the peak value is difficult. Alternatively, a common method is to set the low frequency tone (60 Hz) for +4 dBu and then mixing the 7 kHz tone at a value of –8 dBu (12 dB less).Correct: IMD (SMPTE) less than 0.01%, 60Hz/7kHz, 4:1, +4 dBuWrong:IMD less than 0.01%IMD – ITU-R (CCIF). Intermodulation Distortion – ITU-R MethodWhat is tested? This tests for non-harmonic nonlinearities, using two equal amplitude, closely spaced, high frequency tones, and looking for beat frequencies between them. Use of beat fre-quencies for distortion detection dates back to work first docu-mented in Germany in 1929, but was not considered a standard until 1937, when the CCIF (International Telephonic Consulta-tive Committee) recommend the test. [This test is often mistakenly referred to as the CCI R method (as opposed to the CCI F method).A mistake compounded by the many correct audio references to the CCI R 468 weighting filter.] Ultimately, the CCIF became the radiocommunications sector (ITU-R) of the ITU (International Telecommunications Union), therefore the test is now known as the IMD (ITU-R).How is it measured? The common test signal is a pair of equal amplitude tones spaced 1 kHz apart. Nonlinearity in the unit causes intermodulation products between the two signals. These are found by subtracting the two tones to find the first location at 1 kHz, then subtracting the second tone from twice the first tone, and then turning around and subtracting the first tone from twice the second, and so on. Usually only the first two or three components are measured, but for the oft-seen case of 19 kHz and 20 kHz, only the 1 kHz component is measured.Required Conditions. Many variations exist for this test. Therefore, the manufacturer needs to clearly spell out the two frequencies used,and their level. The ratio is understood to be 1:1.Correct: IMD (ITU-R) less than 0.01%, 19 kHz/20 kHz, 1:1, +4 dBuWrong: IMD less than 0.01%S/N or SNR. Signal-To-Noise RatioWhat is tested? This specification indirectly tells you how noisy a unit is. S/N is calculated by measuring a unit’s output noise, with no signal present, and all controls set to a prescribed manner. This figure is used to calculate a ratio between it and a fixed output reference signal, with the result expressed in dB.How is it measured? No input signal is used, however the input is not left open, or unterminated. The usual practice isto leave the unit connected to the signal generator (with its low output impedance) set for zero volts. Alternatively, a resistor equal to the expected driving impedance is connected between the inputs. The magnitude of the output noise is measured us-ing an rms-detecting voltmeter. Noise voltage is a function of bandwidth – wider the bandwidth, the greater the noise. This is an inescapable physical fact. Thus, a bandwidth is selected for the measuring voltmeter. If this is not done, the noise voltage measures extremely high, but does not correlate well with what is heard. The most common bandwidth seen is 22 kHz (the extra 2 kHz allows the bandwidth-limiting filter to take affect without reducing the response at 20 kHz). This is called a “flat” measure-ment, since all frequencies are measured equally.Alternatively, noise filters, or weighting filters, are used when measuring noise. Most often seen is A-weighting, but a more accurate one is called the ITU-R (old CCIR) 468 filter. This filter is preferred because it shapes the measured noise in a way that relates well with what’s heard.Pro audio equipment often lists an A-weighted noise spec– not because it correlates well with our hearing – but because it can “hide” nasty hum components that make for bad noise specs. Always wonder if a manufacturer is hiding something when you see A-weighting specs. While noise filters are entirely appropri-ate and even desired when measuring other types of noise, it is an abuse to use them to disguise equipment hum problems. A-weighting rolls off the low-end, thus reducing the most annoying 2nd and 3rd line harmonics by about 20 dB and 12 dB respective-ly. Sometimes A-weighting can “improve” a noise spec by 10 dB.The argument used to justify this is that the ear is not sensi-tive to low frequencies at low levels (´ la Fletcher-Munson equal loudness curves), but that argument is false. Fletcher-Munson curves document equal loudness of single tones. Their curve tells us nothing of the ear’s astonishing ability to sync in and lock onto repetitive tones – like hum components – even when these tones lie beneath the noise floor. This is what A-weighting can hide. For this reason most manufacturers shy from using it; instead they spec S/N figures “flat” or use the ITU-R 468 curve (which actually makes their numbers look worse, but correlate better with the real world).However, an exception has arisen: Digital products using A/D and D/A converters regularly spec S/N and dynamic range using A-weighting. This follows the semiconductor industry’s practice of spec’ing delta-sigma data converters A-weighted. They do this because they use clever noise shaping tricks to create 24-bit con-verters with acceptable noise behavior. All these tricks squeeze the noise out of the audio bandwidth and push it up into the higher inaudible frequencies. The noise may be inaudible, but it is still measurable and can give misleading results unless limited. When used this way, the A-weighting filter rolls off the high frequency noise better than the flat 22 kHz filter and compares better with the listening experience. The fact that the low-end also rolls off is irrelevant in this application. (See the RaneNote Digital Dharma of Audio A/D Converters)Required Conditions. In order for the published figure to have any meaning, it must include the measurement bandwidth, including any weighting filters and the reference signal level.Audio Specifications-Stating that a unit has a “S/N = 90 dB” is meaningless without knowing what the signal level is, and over what bandwidth the noise was measured. For example if one product references S/N to their maximum output level of, say, +20 dBu, and another product has the same stated 90 dB S/N, but their reference level is + 4 dBu, then the second product is, in fact, 16 dB quieter. Likewise, you cannot accurately compare numbers if one unit is measured over a BW of 80 kHz and another uses 20 kHz, or if one is measured flat and the other uses A-weighting. By far how-ever, the most common problem is not stating any conditions.Correct: S/N = 90 dB re +4 dBu, 22 kHz BW, unity gainWrong: S/N = 90 dBEIN. Equivalent Input Noise or Input Referred NoiseWhat is tested? Equivalent input noise, or input referred noise, is how noise is spec’d on mixing consoles, standalone mic preamps and other signal processing units with mic inputs. The problem in measuring mixing consoles (and all mic preamps)is knowing ahead of time how much gain is going to be used. The mic stage itself is the dominant noise generator; therefore, the output noise is almost totally determined by the amount of gain: turn the gain up, and the output noise goes up accordingly. Thus, the EIN is the amount of noise added to the input signal. Both are then amplified to obtain the final output signal.For example, say your mixer has an EIN of –130 dBu. This means the noise is 130 dB below a reference point of 0.775 volts (0 dBu). If your microphone puts out, say, -50 dBu under normal conditions, then the S/N at the input to the mic preamp is 80 dB (i.e., the added noise is 80 dB below the input signal). This is uniquely determined by the magnitude of the input signal and the EIN. From here on out, turning up the gain increases both the signal and the noise by the same amount.How is it measured? With the gain set for maximum and the input terminated with the expected source impedance, the output noise is measured with an rms voltmeter fitted with a bandwidth or weighting filter.Required Conditions. This is a spec where test conditions are critical. It is very easy to deceive without them. Since high-gain mic stages greatly amplify source noise, the terminating input resistance must be stated. Two equally quiet inputs will measure vastly different if not using the identical input imped-ance. The standard source impedance is 150 Ω. As unintuitive as it may be, a plain resistor, hooked up to nothing, generates noise, and the larger the resistor value the greater the noise. It is called thermal noise or Johnson noise (after its discoverer J. B. Johnson, in 1928) and results from the motion of electron charge of the atoms making up the resistor. All that moving about is called thermal agitation (caused by heat – the hotter the resistor, the noisier).The input terminating resistor defines the lower limit of noise performance. In use, a mic stage cannot be quieter than the source.A trick which unscrupulous manufacturers may use is to spec their mic stage with the input shorted – a big no-no, since it does not represent the real performance of the preamp.The next biggie in spec’ing the EIN of mic stages is band-width. This same thermal noise limit of the input terminating resistance is a strong function of measurement bandwidth. For example, the noise voltage generated by the standard 150 Ω input resistor, measured over a bandwidth of 20 kHz (and room temperature) is –131 dBu, i.e., you cannot have an operating mic stage, with a 150 Ω source, quieter than –131 dBu. However, if you use only a 10 kHz bandwidth, then the noise drops to –134 dBu, a big 3 dB improvement. (For those paying close attention: it is not 6 dB like you might expect since the bandwidth is half. It is a square root function, so it is reduced by the square root of one-half, or 0.707, which is 3 dB less).Since the measured output noise is such a strong functionof bandwidth and gain, it is recommended to use no weighting filters. They only complicate comparison among manufacturers. Remember: if a manufacturer’s reported EIN seems too good to be true, look for the details. They may not be lying, only using favorable conditions to deceive.Correct: EIN = -130 dBu, 22 kHz BW, max gain, Rs = 150 ΩWrong: EIN = -130 dBuBW. Bandwidth or Frequency Response What is tested? The unit’s bandwidth or the range of frequencies it passes. All frequencies above and below a unit’s Frequency Response are attenuated – sometimes severely.How is it measured? A 1 kHz tone of high purity and precise amplitude is applied to the unit and the output measured using a dB-calibrated rms voltmeter. This value is set as the 0 dB reference point. Next, the generator is swept upward in frequen-cy (from the 1 kHz reference point) keeping the source ampli-tude precisely constant, until it is reduced in level by the amount specified. This point becomes the upper frequency limit. The test generator is then swept down in frequency from 1 kHz until the lower frequency limit is found by the same means.Required Conditions. The reduction in output level is relative to 1 kHz; therefore, the 1 kHz level establishes the 0 dB point. What you need to know is how far down is the response where the manufacturer measured it. Is it 0.5 dB, 3 dB, or (among loudspeaker manufacturers) maybe even 10 dB?Note that there is no discussion of an increase, that is, no mention of the amplitude rising. If a unit’s frequency response rises at any point, especially the endpoints, it indicates a funda-mental instability problem and you should run from the store. Properly designed solid-state audio equipment does not ever gain in amplitude when set for flat response (tubes or valve designs using output transformers are a different story and are not dealt with here). If you have ever wondered why manufacturers state a limit of “+0 dB”, that is why. The preferred condition here is at least 20 Hz to 20 kHz measured +0/-0.5 dB.Correct: Frequency Response = 20–20 kHz, +0/-0.5 dBWrong: Frequency Response = 20-20 kHzCMR or CMRR. Common-Mode Rejection or Common-Mode Rejection RatioWhat is tested? This gives a measure of a balanced input stage’s ability to reject common-mode signals. Common-mode is the name given to signals applied simultaneously to both inputs. Normal differential signals arrive as a pair of equal voltages that are opposite in polarity: one applied to the positive input and the other to the negative input. A common-mode signal drives both inputs with the same polarity. It is the job of a well designed bal-Audio Specifications-anced input stage to amplify differential signals, while simulta-neously rejecting common-mode signals. Most common-mode signals result from RFI (radio frequency interference) and EMI (electromagnetic interference, e.g., hum and buzz) signals induc-ing themselves into the connecting cable. Since most cables con-sist of a tightly twisted pair, the interfering signals are induced equally into each wire. The other big contributors to common-mode signals are power supply and ground related problems between the source and the balanced input stage.How is it measured? Either the unit is adjusted for unity gain, or its gain is first determined and noted. Next, a generator is hooked up to drive both inputs simultaneously through two equal and carefully matched source resistors valued at one-half the expected source resistance, i.e., each input is driven from one-half the normal source impedance. The output of the bal-anced stage is measured using an rms voltmeter and noted. A ratio is calculated by dividing the generator input voltage by the measured output voltage. This ratio is then multiplied by the gain of the unit, and the answer expressed in dB.Required Conditions. The results may be frequency-depen-dent, therefore, the manufacturer must state the frequency tested along with the CMR figure. Most manufacturers spec this at 1 kHz for comparison reasons. The results are assumed constant for all input levels, unless stated otherwise.Correct: CMRR = 40 dB @ 1 kHzWrong: CMRR = 40 dBDynamic RangeWhat is tested? First, the maximum output voltage and then the output noise floor are measured and their ratio expressed in dB. Sounds simple and it is simple, but you still have to be care-ful when comparing units.How is it measured? The maximum output voltage is mea-sured as described below, and the output noise floor is measured using an rms voltmeter fitted with a bandwidth filter (with the input generator set for zero volts). A ratio is formed and the result expressed in dB.Required Conditions. Since this is the ratio of the maxi-mum output signal to the noise floor, then the manufacturer must state what the maximum level is, otherwise, you have no way to evaluate the significance of the number. If one company says their product has a dynamic range of 120 dB and another says theirs is 126 dB, before you jump to buy the bigger number, first ask, “Relative to what?” Second, ask, “Measured over what bandwidth, and were any weighting filters used?” You cannot know which is better without knowing the required conditions.Again, beware of A-weighted specs. Use of A-weighting should only appear in dynamic range specs for digital products with data converters (see discussion under S/N). For instance, us-ing it to spec dynamic range in an analog product may indicate the unit has hum components that might otherwise restrict the dynamic range.Correct: Dynamic Range = 120 dB re +26 dBu, 22 kHz BW Wrong: Dynamic Range = 120 dB Crosstalk or Channel SeparationWhat is tested? Signals from one channel leaking into another channel. This happens between independent channels as well as between left and right stereo channels, or between all six channels of a 5.1 surround processor, for instance.How is it measured? A generator drives one channel and this channel’s output value is noted; meanwhile the other chan-nel is set for zero volts (its generator is left hooked up, but turned to zero, or alternatively the input is terminated with the expect source impedance). Under no circumstances is the measured channel left open. Whatever signal is induced into the tested channel is measured at its output with an rms voltmeter and noted. A ratio is formed by dividing the unwanted signal by the above-noted output test value, and the answer expressed in dB. Since the ratio is always less than one(crosstalk is always less than the original signal) the expression results in negative dB ratings. For example, a crosstalk spec of –60 dB is interpreted to mean the unwanted signal is 60 dB below the test signal.Required Conditions. Most crosstalk results from printed circuit board traces “talking” to each other. The mechanism is capacitive coupling between the closely spaced traces and layers. This makes it strongly frequency dependent, with a characteristic rise of 6 dB/octave, i.e., the crosstalk gets worst at a 6 dB/octave rate with increasing frequency. Therefore knowing the frequency used for testing is essential. And if it is only spec’d at 1 kHz (very common) then you can predict what it may be for higher frequencies. For instance, using the example from above of a –60 dB rating, say, at 1 kHz, then the crosstalk at 16 kHz probably degrades to –36 dB. But don’t panic, the reason this usually isn’t a problem is that the signal level at high frequencies is also reduced by about the same 6 dB/octave rate, so the overall S/N ratio isn’t affected much.Another important point is that crosstalk is assumed level independent unless otherwise noted. This is because the parasitic capacitors formed by the traces are uniquely determined by the layout geometry, not the strength of the signal.Correct: Crosstalk = -60 dB, 20-20kHz, +4 dBu, channel-to-channelWrong: Crosstalk = -60 dBInput & Output ImpedanceWhat is tested? Input impedance measures the load that the unit represents to the driving source, while output impedance measures the source impedance that drives the next unit.How is it measured? Rarely are these values actually mea-sured. Usually they are determined by inspection and analysis of the final schematic and stated as a pure resistance in Ωs. Input and output reactive elements are usually small enough to be ignored. (Phono input stages and other inputs designed for specific load reactance are exceptions.)Required Conditions. The only required information is whether the stated impedance is balanced or unbalanced (bal-anced impedances usually are exactly twice unbalanced ones). For clarity when spec’ing balanced circuits, it is preferred to state whether the resistance is “floating” (exists between the two lines) or is ground referenced (exists from each line to ground).The impedances are assumed constant for all frequencies within the unit’s bandwidth and for all signal levels, unlessAudio Specifications-。
Rmetrics - 非线性和混沌时间序列建模说明书
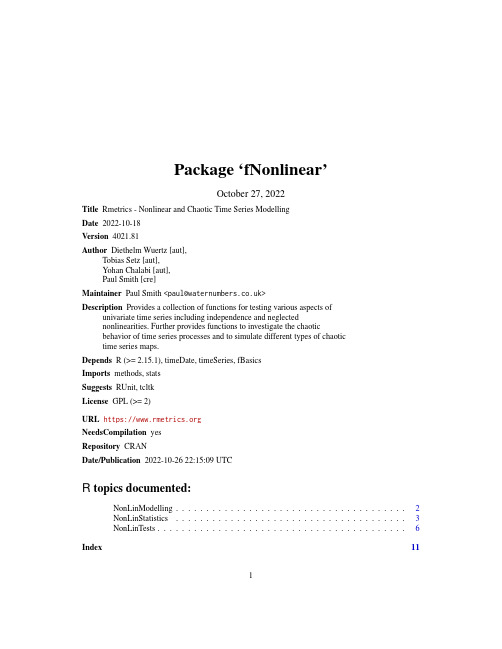
Package‘fNonlinear’October27,2022Title Rmetrics-Nonlinear and Chaotic Time Series ModellingDate2022-10-18Version4021.81Author Diethelm Wuertz[aut],Tobias Setz[aut],Yohan Chalabi[aut],Paul Smith[cre]Maintainer Paul Smith<********************.uk>Description Provides a collection of functions for testing various aspects ofunivariate time series including independence and neglectednonlinearities.Further provides functions to investigate the chaoticbehavior of time series processes and to simulate different types of chaotictime series maps.Depends R(>=2.15.1),timeDate,timeSeries,fBasicsImports methods,statsSuggests RUnit,tcltkLicense GPL(>=2)URL https://NeedsCompilation yesRepository CRANDate/Publication2022-10-2622:15:09UTCR topics documented:NonLinModelling (2)NonLinStatistics (3)NonLinTests (6)Index1112NonLinModelling NonLinModelling Chaotic Time Series ModellingDescriptionA collection and description of functions to simulate different types of chaotic time series maps.Chaotic Time Series Maps:tentSim Simulates data from the Tent Map,henonSim simulates data from the Henon Map,ikedaSim simulates data from the Ikeda Map,logisticSim simulates data from the Logistic Map,lorentzSim simulates data from the Lorentz Map,roesslerSim simulates data from the Roessler Map.UsagetentSim(n=1000,n.skip=100,parms=c(a=2),start=runif(1), doplot=FALSE)henonSim(n=1000,n.skip=100,parms=c(a=1.4,b=0.3),start=runif(2),doplot=FALSE)ikedaSim(n=1000,n.skip=100,parms=c(a=0.4,b=6.0,c=0.9), start=runif(2),doplot=FALSE)logisticSim(n=1000,n.skip=100,parms=c(r=4),start=runif(1), doplot=FALSE)lorentzSim(times=seq(0,40,by=0.01),parms=c(sigma=16,r=45.92, b=4),start=c(-14,-13,47),doplot=TRUE,...)roesslerSim(times=seq(0,100,by=0.01),parms=c(a=0.2,b=0.2,c=8.0), start=c(-1.894,-9.920,0.0250),doplot=TRUE,...)Argumentsdoplot a logicalflag.Should a plot be displayed?n,n.skip[henonSim][ikedaSim][logisticSim]-the number of chaotic time series points to be generated and the number of initialvalues to be skipped from the series.parms the named parameter vector characterizing the chaotic map.start the vector of start values to initiate the chaotic map.times[lorentzSim][roesslerSim]-the sequence of time series points at which to generate the map....arguments to be passed.Value[*Sim]-All functions return invisible a vector of time series data.Author(s)Diethelm Wuertz for the Rmetrics R-port.ReferencesBrock,W.A.,Dechert W.D.,Sheinkman J.A.(1987);A Test of Independence Based on the Correla-tion Dimension,SSRI no.8702,Department of Economics,University of Wisconsin,Madison.Eckmann J.P.,Oliffson Kamphorst S.,Ruelle D.(1987),Recurrence plots of dynamical systems, Europhys.Letters4,973.Hegger R.,Kantz H.,Schreiber T.(1999);Practical implementation of nonlinear time series meth-ods:The TISEAN package,CHAOS9,413–435.Kennel M.B.,Brown R.,Abarbanel H.D.I.(1992);Determining embedding dimension for phase-space reconstruction using a geometrical construction,Phys.Rev.A45,3403.Rosenstein M.T.,Collins J.J.,De Luca C.J.(1993);A practical method for calculating largest Lyapunov exponents from small data sets,Physica D65,117.See AlsoRandomInnovations.Examples##logisticSim-set.seed(4711)x=logisticSim(n=100)plot(x,main="Logistic Map")NonLinStatistics Chaotic Time Series StatisticsDescriptionA collection and description of functions to investigate the chaotic behavior of time series processes.Functions to Analyse Chaotic Time Series:mutualPlot Returns mutual information,falsennPlot returns false nearest neigbours,recurrencePlot returns a recurrence plot,separationPlot returns a space-time separation plot,lyapunovPlot computes maximum lyapunov exponent.UsagemutualPlot(x,partitions=16,lag.max=20,doplot=TRUE,...)falsennPlot(x,m,d,t,rt=10,eps=NULL,doplot=TRUE,...)recurrencePlot(x,m,d,end.time,eps,nt=10,doplot=TRUE,...)separationPlot(x,m,d,mdt,idt=1,doplot=TRUE,...)lyapunovPlot(x,m,d,t,ref,s,eps,k=1,doplot=TRUE,...)Argumentsd an integer value setting the value of the time delay.eps[falsennPlot]-a numeric value setting the value of the neighbour diameter.If NULL,which isthe default value,then the value will be automatically setted to eps=sd(x)/10.[lyapunovPlot]-the radius where tofind nearest neighbours.[recurrencePlot]-the neighbourhood threshold.doplot a logicalflag.Should a plot be displayed?end.time[recurrencePlot]-ending time as number of observations.idt[separationPlot]-an integer value setting the number of observation steps in each iterations.Bydefault1.k[lyapunovPlot]-an integer setting th enumber of considered neighbours.By default1.lag.max[mutualPlot]-an integer value setting the number of maximum lags,by default20.m[*Plot]-an integer value setting the value of the maximum embedding dimension.mdt[separationPlot]-an integer value setting the number of iterations.nt[recurrencePlot]-observations in each step which will be plotted,by default10.Increasing nt re-duces number of points plotted which is usefule especially with highly sampleddata.rt[falsennPlot]-an integer value setting the value for the escape factor.By default10.partitions[mutualPlot]-an integer value setting the number of bins,by default16.ref[lyapunovPlot]-the number of points to take into account.s[lyapunovPlot]-the iterations along which follow the neighbours of each point.t[*Plot]-an integer value setting the value for the Theiler window.x[*Plot]-a numeric vector,or an object either of class’ts’or of class’timeSeries’....arguments to be passed.DetailsPhase Space Representation:The function mutualPlot estimates and plots the mutual information index of a given time se-ries for a specified number of lags.The joint probability distribution function is estimated with a simple bi-dimensional density histogram.The function falsennPlot uses the Method of false nearest neighbours to help deciding the opti-mal embedding dimension.Non-Stationarity:The funcdtion recurrencePlot creates a recurrence plot as proposed by Eckmann et al.[1987].The function separationPlot creates a space-time separation plot qs introduced by Provenzale et al.[1992].It plots the probability that two points in the reconstructed phase-space have distance smaller than epsilon in function of epsilon and of the time between the points,as iso-lines at levels 10,20,...,100percent levels.The plot can be used to decide the Theiler time window.Lyapunov Exponents:The function lyapunovPlot evaluates and plots the largest Lyapunov exponent of a dynamic sys-tem from a univariate time series.The estimate of the Lyapunov exponent uses the algorithm of Kantz.In addition,the function computes the regression coefficients of a user specified segment of the sequence given as input.Dimensions and Entropies:The function C2computes the sample correlation integral on the provided time series for the speci-fied length scale and Theiler window.It uses a naiv algorithm:simply returns the fraction of points pairs nearer than eps.It is prefarable to use the function d2,which takes roughly the same time,but computes the correlation sum for multiple length scales and embedding dimensions at once.The function d2computes the sample correlation integral over given length scales neps for embed-ding dimensions1:m for a given Theiler window.The slope of the linear segment in the log-log plot gives an estimate of the correlation dimension.Author(s)Diethelm Wuertz for the Rmetrics R-port.ReferencesBrock,W.A.,Dechert W.D.,Sheinkman J.A.(1987);A Test of Independence Based on the Correla-tion Dimension,SSRI no.8702,Department of Economics,University of Wisconsin,Madison.Eckmann J.P.,Oliffson Kamphorst S.,Ruelle D.(1987),Recurrence plots of dynamical systems, Europhys.Letters4,973.Hegger R.,Kantz H.,Schreiber T.(1999);Practical implementation of nonlinear time series meth-ods:The TISEAN package,CHAOS9,413–435.Kennel M.B.,Brown R.,Abarbanel H.D.I.(1992);Determining embedding dimension for phase-space reconstruction using a geometrical construction,Phys.Rev.A45,3403.Rosenstein M.T.,Collins J.J.,De Luca C.J.(1993);A practical method for calculating largest Lyapunov exponents from small data sets,Physica D65,117.See AlsoRandomInnovations.Examples##mutualPlot-mutualPlot(logisticSim(1000))##recurrencePlot-lorentz=lorentzSim(times=seq(0,40,by=0.01),parms=c(sigma=16,r=45.92,b=4),start=c(-14,-13,47),doplot=FALSE)recurrencePlot(lorentz[,2],m=3,d=2,end.time=800,eps=3,nt=5,pch= . ,cex=2)NonLinTests Time Series TestsDescriptionA collection and description of functions for testing various aspects of univariate time series,in-cluding independence,and neglected nonlinearities.The family of time series tests includes the following hypothesis tests:bdsTest Brock--Dechert--Scheinkman test for iid seriestnnTest Teraesvirta NN test for neglected nonlinearitywnnTest White NN test for neglected nonlinearityrunsTest Runs test for detecting non-randomnessUsagebdsTest(x,m=3,eps=NULL,title=NULL,description=NULL)tnnTest(x,lag=1,title=NULL,description=NULL)wnnTest(x,lag=1,qstar=2,q=10,range=4,title=NULL,description=NULL) runsTest(x)Argumentsdescription optional description string,or a vector of character strings.eps[bdsTest]-a numeric vector of epsilon values for close points.The BDS test is computedfor each element of eps.It should be set in terms of the standard deviation of x.If eps is NULL,then the four default values seq(0.5*sd(x),2*sd(x),length=4)are used.lag[tnnTest][wnnTest]-an integer which specifies the model order in terms of lags.m[bdsTest]-an integer indicating that the BDS test statistic is computed for embedding di-mensions2,...,m.q[wnnTest]-an integer representing the number of phantom hidden units used to compute thetest statistic.qstar[wnnTest]-the test is conducted using qstar principal components of the phantom hiddenunits.Thefirst principal component is omitted since in most cases it appears tobe collinear with the input vector of lagged variables.This strategy preservespower while still conserving degrees of freedom.range[wnnTest]-the input to hidden unit weights are initialized uniformly over[-range/2,range/2].title an optional title string,if not specified the inputs data name is deparsed.x a numeric vector or an object of class"timeseries".DetailsBrock–Dechert–Sheinkman Test:The bdsTest test examines the spatial dependence of the observed series.To do this,the series is embedded in m-space and the dependence of x is examined by counting near points.Points for which the distance is less than eps are called near.The BDS test statistic is asymptotically standard Normal.Note,that missing values are not allowed.There is a special print method for objects of class"bdsTest"which by default uses4digits to format real numbers.[tseries:bds.test]Teraesvirta Neural Network Test:The null is the hypotheses of linearity in mean.This test uses a Taylor series expansion of the activation function to arrive at a suitable test statistic.If type equals"F",then the F-statistic in-stead of the Chi-Squared statistic is used in analogy to the classical linear regression.Missing values are not allowed.[tseries:teraesvirta.test]White Neural Network Test:The null is the hypotheses of linearity in“mean”.This type of test is consistent against arbitrary nonlinearity in mean.If type equals"F",then the F-statistic instead of the Chi-Squared statistic is used in analogy to the classical linear regression.[tseries:white.test]Runs Test:The runs test can be used to decide if a data set is from a random process.A run is defined asa series of increasing values or a series of decreasing values.The number of increasing,or decreas-ing,values is the length of the run.In a random data set,the probability that the(i+1)-th value is larger or smaller than the i-th value follows a binomial distribution,which forms the basis of the runs test.[tseries:runs.test]ValueIn contrast to R’s output report from S3objects of class"htest"a different output report is pro-duced.The tests here return an S4object of class"fHTEST".The object contains the following slots:@call the function call.@data the data as specified by the input argument(s).@test a list whose elements contail the results from the statistical test.The information provided is similar to a list object of"htest".@title a character string with the name of the test.This can be overwritten specifyinga user defined input argument.@description a character string with an optional user defined description.By default just the current date when the test was applied will be returned.The slot@test returns an object of class"list"containing the following(otionally empty)ele-ments:statistic the value(s)of the test statistic.p.value the p-value(s)of the test.parameters a numeric value or vector of parameters.estimate a numeric value or vector of sample estimates.conf.int a numeric two row vector or matrix of95method a character string indicating what type of test was performed. a character string giving the name(s)of the data.NoteThe output of the various hypothesis tests is an object of class htest.The associated print method gives an unique report about the test results.Author(s)Adrian Trapletti for the tests from R’s tseries package,Blake LeBaron for the bds C program,Diethelm Wuertz for the Rmetrics R-port.ReferencesBrock,W.A.,Dechert W.D.,Sheinkman J.A.(1987);A Test of Independence Based on the Correla-tion Dimension,SSRI no.8702,Department of Economics,University of Wisconsin,Madison.Conover,W.J.(1980);Practical Nonparametric Statistics,New York,Wiley.Cromwell J.B.,Labys W.C.,Terraza M.(1994);Univariate Tests for Time Series Models,Sage, Thousand Oaks,CA,pages32–36.Lee T.H.,White H.,Granger C.W.J.(1993);Testing for neglected nonlinearity in time series models, Journal of Econometrics56,269–290.Teraesvirta T.,Lin C.F.,Granger C.W.J.(1993);Power of the Neural Network Linearity Test,Journal of Time Series Analysis14,209–220.Examples##bdsTest-#iid Time Series:par(mfrow=c(3,1))x=rnorm(100)plot(x,type="l",main="iid Time Series")bdsTest(x,m=3)#Non Identically Distributed Time Series:x=c(rnorm(50),runif(50))plot(x,type="l",main="Non-iid Time Series")bdsTest(x,m=3)#Non Independent Innovations from Quadratic Map:x=rep(0.2,100)for(i in2:100)x[i]=4*(1-x[i-1])*x[i-1]plot(x,type="l",main="Quadratic Map")bdsTest(x,m=3)##tnnTest-#Time Series Non-linear in"mean"regressionpar(mfrow=c(2,1))n=1000x=runif(1000,-1,1)tnnTest(x)#Generate time series which is nonlinear in"mean"x[1]=0.0for(i in(2:n)){x[i]=0.4*x[i-1]+tanh(x[i-1])+rnorm(1,sd=0.5)} plot(x,main="Teraesvirta Test",type="l")tnnTest(x)##wnnTest-#Time Series Non-Linear in"mean"Regressionpar(mfrow=c(2,1))n=1000x=runif(1000,-1,1)wnnTest(x)#Generate time series which is nonlinear in"mean"x[1]=0.0for(i in(2:n)){x[i]=0.4*x[i-1]+tanh(x[i-1])+rnorm(1,sd=0.5)} plot(x,main="White Test",type="l")wnnTest(x)Index∗htestNonLinTests,6∗modelsNonLinModelling,2NonLinStatistics,3bdsTest(NonLinTests),6falsennPlot(NonLinStatistics),3 henonSim(NonLinModelling),2ikedaSim(NonLinModelling),2logisticSim(NonLinModelling),2 lorentzSim(NonLinModelling),2 lyapunovPlot(NonLinStatistics),3 mutualPlot(NonLinStatistics),3 NonLinModelling,2NonLinStatistics,3NonLinTests,6recurrencePlot(NonLinStatistics),3 roesslerSim(NonLinModelling),2runsTest(NonLinTests),6separationPlot(NonLinStatistics),3 tentSim(NonLinModelling),2tnnTest(NonLinTests),6tsTest(NonLinTests),6wnnTest(NonLinTests),611。
THETESTINGOFLINEN
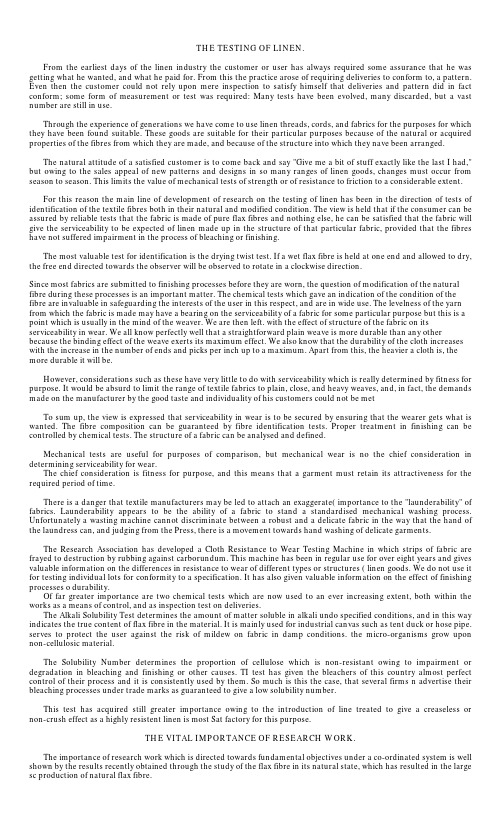
THE TESTING OF LINEN.From the earliest days of the linen industry the customer or user has always required some assurance that he was getting what he wanted, and what he paid for. From this the practice arose of requiring deliveries to conform to, a pattern. Even then the customer could not rely upon mere inspection to satisfy himself that deliveries and pattern did in fact conform; some form of measurement or test was required: Many tests have been evolved, many discarded, but a vast number are still in use.Through the experience of generations we have come to use linen threads, cords, and fabrics for the purposes for which they have been found suitable. These goods are suitable for their particular purposes because of the natural or acquired properties of the fibres from which they are made, and because of the structure into which they nave been arranged.The natural attitude of a satisfied customer is to come back and say "Give me a bit of stuff exactly like the last I had," but owing to the sales appeal of new patterns and designs in so many ranges of linen goods, changes must occur from season to season. This limits the value of mechanical tests of strength or of resistance to friction to a considerable extent.For this reason the main line of development of research on the testing of linen has been in the direction of tests of identification of the textile fibres both in their natural and modified condition. The view is held that if the consumer can be assured by reliable tests that the fabric is made of pure flax fibres and nothing else, he can be satisfied that the fabric will give the serviceability to be expected of linen made up in the structure of that particular fabric, provided that the fibres have not suffered impairment in the process of bleaching or finishing.The most valuable test for identification is the drying twist test. If a wet flax fibre is held at one end and allowed to dry, the free end directed towards the observer will be observed to rotate in a clockwise direction.Since most fabrics are submitted to finishing processes before they are worn, the question of modification of the natural fibre during these processes is an important matter. The chemical tests which gave an indication of the condition of the fibre are invaluable in safeguarding the interests of the user in this respect, and are in wide use. The levelness of the yarn from which the fabric is made may have a bearing on the serviceability of a fabric for some particular purpose but this is a point which is usually in the mind of the weaver. We are then left. with the effect of structure of the fabric on its serviceability in wear. We all know perfectly well that a straightforward plain weave is more durable than any other because the binding effect of the weave exerts its maximum effect. We also know that the durability of the cloth increases with the increase in the number of ends and picks per inch up to a maximum. Apart from this, the heavier a cloth is, the more durable it will be.However, considerations such as these have very little to do with serviceability which is really determined by fitness for purpose. It would be absurd to limit the range of textile fabrics to plain, close, and heavy weaves, and, in fact, the demands made on the manufacturer by the good taste and individuality of his customers could not be metTo sum up, the view is expressed that serviceability in wear is to be secured by ensuring that the wearer gets what is wanted. The fibre composition can be guaranteed by fibre identification tests. Proper treatment in finishing can be controlled by chemical tests. The structure of a fabric can be analysed and defined.Mechanical tests are useful for purposes of comparison, but mechanical wear is no the chief consideration in determining serviceability for wear.The chief consideration is fitness for purpose, and this means that a garment must retain its attractiveness for the required period of time.There is a danger that textile manufacturers may be led to attach an exaggerate( importance to the "launderability" of fabrics. Launderability appears to be the ability of a fabric to stand a standardised mechanical washing process. Unfortunately a wasting machine cannot discriminate between a robust and a delicate fabric in the way that the hand of the laundress can, and judging from the Press, there is a movement towards hand washing of delicate garments.The Research Association has developed a Cloth Resistance to Wear Testing Machine in which strips of fabric are frayed to destruction by rubbing against carborundum. This machine has been in regular use for over eight years and gives valuable information on the differences in resistance to wear of different types or structures ( linen goods. We do not use it for testing individual lots for conformity to a specification. It has also given valuable information on the effect of finishing processes o durability.Of far greater importance are two chemical tests which are now used to an ever increasing extent, both within the works as a means of control, and as inspection test on deliveries.The Alkali Solubility Test determines the amount of matter soluble in alkali undo specified conditions, and in this way indicates the true content of flax fibre in the material. It is mainly used for industrial canvas such as tent duck or hose pipe. serves to protect the user against the risk of mildew on fabric in damp conditions. the micro-organisms grow upon non-cellulosic material.The Solubility Number determines the proportion of cellulose which is non-resistant owing to impairment or degradation in bleaching and finishing or other causes. TI test has given the bleachers of this country almost perfect control of their process and it is consistently used by them. So much is this the case, that several firms n advertise their bleaching processes under trade marks as guaranteed to give a low solubility number.This test has acquired still greater importance owing to the introduction of line treated to give a creaseless or non-crush effect as a highly resistent linen is most Sat factory for this purpose.THE VITAL IMPORTANCE OF RESEARCH WORK.The importance of research work which is directed towards fundamental objectives under a co-ordinated system is well shown by the results recently obtained through the study of the flax fibre in its natural state, which has resulted in the large sc production of natural flax fibre.The invention of a new type of machine for breaking flax straw before scutching, by the Research Association,has led to the production of linen fabrics from flax fibre which has not undergone the age-old retting or steeping process. Such fabrics consist of flax fibres still in their naturally-occurring state. Ordinarily, retting the flax straw brings about modifications in the plant stem which render the fibre difficult to purify without having recourse to severe bleaching processes.In dealing with retted flax, the bleacher has to destroy or remove a group of substances which are degradation products of the natural constituents of the flax plant, but in dealing with natural or unretted flax the bleacher has to deal with an entirely different set of substances which are themselves natural constituents of the flax plant, and he has not to do more than remove these substances from the fibre, thus making the task of avoiding injury to the cellulose comparatively simple. The true natural fibre in all its dazzling lustre and whiteness is readily obtained.Having been able to produce with the aid of the new machine several tons of natural flax fibre, the Research Association has been actively engaged in determining with the assistance of its member firms, the range of yarns and fabrics which can be produced from natural flax.The advantages of linen made from natural flax are, ready bleaching, exceptional launderability, high absorbency, and a cheapening of the manufacturing processes.In another direction the Research Association has been able to open up wider possibilities for the use of linen by a special mechanical process which gives a soft yarn with many of the characteristics of wool.There would appear to be a wide field for the application of this yarn, both in conjunction with wool, and in pure linen materials. Examples of this new type of linen are shown, but this new result of research must still take some time to reach commercial development.。
CJAS2013年第一卷第一期
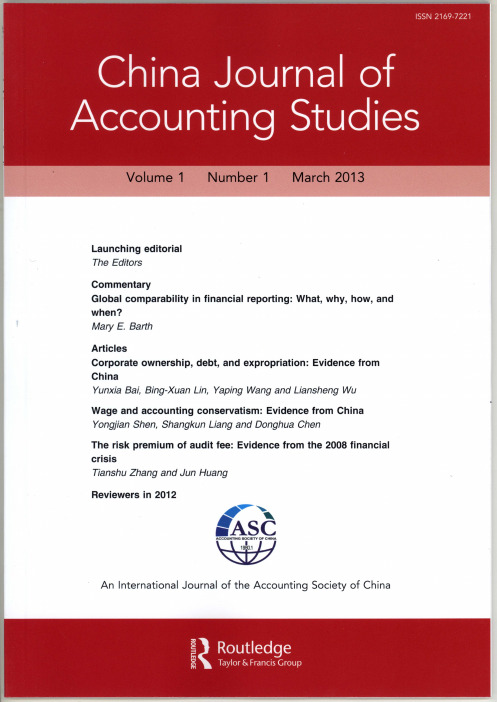
Launching editorialFollowing the adoption of economic reform and open door policies over the last two decades,China ’s economy is now signi ficantly integrated with international economies.Concurrently,Chinese accounting/auditing standards have made substantial strides towards convergence with International Financial Reporting Standards as well as Interna-tional Standards of Auditing.However,China ’s economy is still in a transitional phase with strong remnants of previous central planning regimes.Consequently,state owner-ship still has a strong presence in many sectors and enterprises and the government plays a signi ficant role in business affairs,accounting and finance regulation,and enforcement.With the strong trend towards economic globalisation and accounting convergence,accounting and finance research in China can and should no longer be undertaken in isolation.At the same time,the transitional nature of China ’s economy offers numerous opportunities for identifying unique accounting and finance issues and solutions.To promote and support signi ficant scholarship in this environment,we are pleased to launch The China Journal of Accounting Studies (CJAS)as a forum for knowledge exchange between and among Chinese and international academics,research students,policy makers and others interested in accounting and finance research and develop-ments in China and elsewhere.CJAS is the of ficial international research journal of the Accounting Society of China.The Society was established in 1980and has been the largest accounting associ-ation in China,with over 2400individual members and over 250institutional members.Even with strict admission criteria,the numbers of both individual and institutional members continue to increase from year to year.As an association journal,CJAS will adhere to a principle of openness and inclusive-ness.This means that it welcomes high-quality papers in financial accounting,manage-ment accounting,auditing,corporate finance,corporate governance,public sector accounting,social and environmental accounting,accounting education,accounting his-tory,accounting information systems,and related areas.The Journal will embrace a wide range of theoretical paradigms based on economics,sociology,psychology and other related sciences and social sciences and research methodologies (e.g.analytical,archival,experimental,survey and qualitative case methods).In addition,it will publish original papers on Chinese as well as non-Chinese accounting and finance theories,methods and issues.In particular,the Journal welcomes submissions that investigate Chinese and inter-national issues in comparative terms,whether comparing China with advanced economies or with other emerging and transitional economies.It expects such comparative studies to offer opportunities for identifying unique accounting and finance issues and solutions in individual countries,and also to provide settings for testing established theories and para-digms as well as developing new ones.It welcomes submissions in English or Chinese and will evaluate them on their origi-nality,rigor,relevance and quality of exposition.The EditorsV ol.1,No.1,1,/10.1080/21697221.2013.781768Ó2013Accounting Society of China D o w n l o a d e d b y [124.207.132.50] a t 19:00 04 J u n e 2013COMMENTARYGlobal comparability in financial reporting:What,why,how,and when?Mary E.Barth*Graduate School of Business,Stanford University,Stanford,CA 94305,USAThe Conceptual Framework identi fies comparability as a qualitative characteristic ofuseful financial reporting information.This paper explains what comparability is,whycomparability is desirable,how comparability is achieved,and when we mightachieve it.In particular,comparability is the qualitative characteristic that enablesusers to identify and understand similarities in,and differences among,items;compa-rability aides investors,lenders and other creditors in making informed capital alloca-tion decisions;and achieving comparability depends on firms applying a common setof financial reporting standards and on requirements in the standards,especially mea-surement requirements.The paper discusses research showing that greater compara-bility can lower costs of comparing investment opportunities and improving financialreporting information quality.When comparability might be achieved is uncertain,although much progress has been made recently.Keywords:Comparability;global financial reporting;International Financial Report-ing Standards;Conceptual FrameworkThe Conceptual Framework of the International Accounting Standards Board (IASB,2010)speci fies comparability as one of the qualitative characteristics of financial report-ing information,which enable that information to achieve the objective of financial reporting.That objective is to provide investors,lenders and other creditors with infor-mation that helps them in making their capital allocation decisions.Because capital is a scare resource,comparability is a crucial characteristic of financial reporting informa-tion.If investors,lenders and other creditors cannot make informed comparisons of alternative investment opportunities,their capital allocation decisions will be subopti-mal.In fact,some believe that enabling investors to compare investment opportunitiesis the main reason we need financial accounting standards to prescribe the contents of financial reports.Without such standards,firms could portray and provide information about their financial position and performance in any way they choose.Given that financial reporting does not derive from a law of nature,there are innumerable ways firms could do that,and comparability would be lost.Thus,comparability is crucial to high quality financial reporting.This discussion seeks to explain what comparability is,why comparability creates bene fits for investors and the firms in which they invest,how comparability can be achieved,and when we might achieve it.*Email:mbarth@Paper accepted by Jason Xiao.V ol.1,No.1,2–12,/10.1080/21697221.2013.781765Ó2013Accounting Society of ChinaD o w n l o a d e d b y [124.207.132.50] a t 19:15 04 J u n e 2013What is comparability?Although the word ‘comparability ’has a meaning in the English language,financial accounting standard setters have a precise de finition in mind.The Conceptual Frame-work explains that comparability is the qualitative characteristic of financial reporting information that enables users to identify and understand similarities in,and differences among,items.That is,comparability results in like things looking alike and different things looking different.The Conceptual Framework goes on to explain that compara-bility makes financial reporting information useful because the information can be com-pared to similar information about other entities or about the same entity at a different parability does not relate to a single item;comparability requires at least two items that are being compared.To avoid misunderstanding,it is important to clarify what comparability is parability is not consistency.Consistency refers to the use of the same accounting methods or principles by a firm for the same items over parability is a goal of consistency and,thus,consistency helps achieve comparability.In itself,however,consistency does not ensure parability also is not uniformity.This is a source of confusion for parability results in like things looking alike and different things looking different.Uniformity requires treating all things in the same way.As a result,uniformity can make unlike things look alike,which impairs,not enhances,comparability.For example,consider an accounting rule specifying that all buildings be depreciated on a straight-line basis using a 30-year useful life and assuming a 10%residual value.Thus,the depreciation method for all buildings would be the same.What if some buildings last 20years and others last 200years?What if some buildings have a 5%residual value and others have a 25%residual value?What if some buildings deteriorate more rapidly at first and others deteriorate more rapidly nearer the end of their lives?Unless all buildings have a 30-year useful life,a 10%residual value,and economic bene fits that are consumed in a straight-line pattern,using the same depreciation method achieves uniformity,but not comparability.It makes all buildings look alike,when in fact they are different.That is not compara-bility.The Conceptual Framework also explains that some degree of comparability can be achieved by faithful representation,which is one of the fundamental qualitative charac-teristics of financial reporting information.That is,if financial statements faithfully rep-resent an item –e.g.,an asset or a liability –then comparability should follow.This is because a faithful representation would re flect the characteristics of the item.In the building example,if the residual value of a particular building is 25%,not 10%,then depreciating the building assuming a 10%residual value would not result in a faithful representation of the building.Comparability:why?Why is comparability so crucial to financial reporting?The primary reason –as with all qualitative characteristics of financial reporting information –is to help meet the objec-tive of financial reporting.That objective is to provide financial information about the entity that is useful to existing and potential investors,lenders and other creditors in making decisions about providing resources to the entity.The Conceptual Framework explains that decisions to buy,sell,or hold equity and debt instruments require alloca-tion of capital,and financial reporting is aimed at those who cannot demand the infor-mation they need to make those capital allocation decisions.Thus,comparability in China Journal of Accounting Studies 3D o w n l o a d e d b y [124.207.132.50] a t 19:15 04 J u n e 2013financial reporting across entities and over time is crucial to enabling investors,lenders and other creditors to make more informed capital allocation decisions.Comparability:how?Having established the importance of comparability,the next question is how can it be achieved?This,of course,is not a simple task.Global standardsWhen many people think about comparability in financial reporting,they think about the increasing use of International Financial Reporting Standards (IFRS).This is,in part,because the stated vision for IFRS is:…one single set of high quality global standards ed on the global capital markets.Many equate use of the same set of standards with achieving comparability.This vision for IFRS is based on the belief that use of global standards will improve the functioning of global capital markets.This should occur by increasing comparability and the quality of information,and by decreasing the costs of preparing financial reports,particularly for multinational firms,and information rmation risk is the risk that investors perceive when they know that they do not fully understand the information they are given,which would be the case if they are not ‘fluent ’in the accounting standards on which a firm ’s financial statements are based.Decreasing information risk should decrease the cost of capital.It is dif ficult to imagine how comparability can be achieved without the use of glo-bal financial reporting standards.However,use of global standards is only a necessary step –not a suf ficient step –to achieving comparability because,for example,the stan-dards need to be rigorously applied and enforced.Ensuring any set of financial report-ing standards achieves its potential ultimately depends on firms applying the standards as written,auditors auditing the resulting financial statements to ensure compliance with the standards,and regulators enforcing the standards.Requirements in standards Although a focus over the last decade or so has been on the widespread adoption ofIFRS as a means of achieving comparability,even strict adherence to a single set of standards does not ensure comparability.The requirements in the standards also affect comparability and,thus,should not be overlooked.Let me explain how and why.The Conceptual Framework considers financial statement elements,e.g.,assets,lia-bilities,income,and expense,item by item.Examples of financial statement elements are accounts receivable,inventory,and long-term debt.The aim of focusing on financial statement elements item by item is to provide investors with comparable information about the entity ’s assets and claims against those assets.Pro fit or loss is the change in the assets and claims that do not arise from other assets,liabilities,or transactions with equity holders in their capacity as equity holders.The assumption underlying this focus is that comparability results from portraying financial statement elements in the same way,for example by recognizing the same (sub)set of assets and liabilities and measur-ing them in the same way.However,does recognizing the same (sub)set of assets and liabilities achieve compa-rability?What if different assets and liabilities are important for some firms versus oth-4BarthD o w n l o a d e d b y [124.207.132.50] a t 19:15 04 J u n e 2013ers?For example,what about intellectual property assets of knowledge-based firms;property,plant,and equipment for durable manufacturers;and insurance liabilities for insurers?Do we achieve comparability if some assets –e.g.,intangibles –or particular types of claims –e.g.,claims with uncertain outcomes –are omitted?Do we achieve comparability if we omit intellectual property assets for all firms?Unrecognized assets and liabilities also have direct consequences for comparability of pro fit or loss because pro fit or loss depends on changes in recognized asset and liability amounts.Thus,if an asset is omitted,by construction the change in its recognized amount is zero and,as a result,it has no effect on pro fit or loss.If we omit intellectual property assets for all firms,do the financial statements of a knowledge-based firm and a durable manufacturer re flect their similarities and differences in a way that enables investors,lenders and other creditors to make informed capital allocation decisions?I cannot see how they can.MeasurementMeasurement also plays a crucial role in comparability that is often overlooked.Because financial reporting standards focus on financial statement elements item by item,one is lulled into thinking that measuring the same asset in the same way helps achieve comparability.But,does it?What if the measure is modi fied historical cost?1Although the method is the same,the resulting amounts likely differ.For example,the same asset purchased at different times will likely have a different measure.More dif-ferences can emerge over the life of the asset,e.g.,if the asset is impaired or is part of a fair value hedge and,thus,its carrying amount is adjusted for the change in fair value attributable to the risk identi fied in the fair value hedge.How can modi fied historical cost achieve comparability?What if the measure is fair value?IFRS 13Fair Value Measurement de fines fair value as the price that would be obtained to sell an asset or transfer a liability between market participants at the measurement date.Fair value has the potential to achieve com-parability because one would expect economic differences and similarities to be re flected in value.Thus,using fair values makes like things look alike and different things look different.However,a concern about using fair value is the potential effects of discretion in estimating the fair values.Although some assets and liabilities have readily determin-able market values,others do not,which means that their fair values must be estimated by managers.Whenever estimates are used in financial reporting –which is almost everywhere –there is concern that managers will use their discretion opportunistically to affect the estimates.There is a vast academic literature that finds evidence of manag-ers ’opportunistic exercise of discretion relating to many accounting amounts,regardless of whether they are based on modi fied historical cost or on fair value.What if the measure were something else?Perhaps another measure exists that over-comes the undesirable features of both modi fied historical cost and fair value and pos-sesses desirable features.Unfortunately,standard setters have yet to identify such an alternative measure.As an example to illustrate the effects on comparability of using cost or fair value to measure all assets,consider three entities:Entity A,Entity B,and Entity C.Entities A,B,and C each owns one share of common stock in Entity Z.The acquisition cost is 20for Entity A,40for Entity B,and 60for Entity C,and the current fair value of a share of common stock in Entity Z is 45.Are the financial statements of Entities A,B,and C comparable if each measures its investment at cost?The answer is ‘no ’because the three cost amounts –20,40,and 60–make the asset look different when it is the same.Thus,China Journal of Accounting Studies 5D o w n l o a d e d b y [124.207.132.50] a t 19:15 04 J u n e 2013cost makes like things look different,thereby failing to achieve comparability.Are the financial statements of Entities A,B,and C comparable if each measures its investment at fair value?The answer is ‘yes ’because all three entities would measure the asset at 45,which means that the same asset held by different entities looks the same.That achieves comparability.However,this conclusion is only clear because the fair value was speci fied in this example.To the extent that Entities A,B,and C need to estimate the fair value,they might estimate different amounts.In such a case,whether cost or fair value provides more comparability depends on whether the range of fair value estimates is smaller than 40,i.e.,60minus 20.That is,it depends on whether fair value results in making the same asset held by the three entities look more alike than cost.Some might argue that comparability is best achieved by reporting both amounts,for example if each entity measures the investment at cost and discloses the fair value.However,the Conceptual Framework is clear that disclosure is not a substitute for rec-ognition and the limited academic research that exists on recognition versus disclosure tends to support that view.What about measuring different assets of the entity in different ways?Can this achieve comparability?For example,presently we measure many financial assets at fair value and property,plant,and equipment at modi fied historical cost.We impair accounts receivable for incurred credit losses;property,plant,and equipment to recoverable amount;and inventory to lower of cost or fair value less costs to sell.We measure most long-term debt at amortized cost and derivative liabilities at fair value.There are many measurement methods used in financial reporting and most often the different measures apply to differ-ent assets.The question is whether this approach can achieve comparability.As an example to illustrate this issue,consider the assets of Entity A and Entity B.A B Cash 500500Accounts receivable 10001000Property,plant,and equipment 15001500Total assets 30003000The reported assets of these two entities –3000–make the assets look the same,and it appears that each entity ’s accounts receivable represents one-third of its assets and prop-erty,plant,and equipment represents one-half of its assets.If this reporting achieves comparability,then the two entities should have the same assets and these proportions should re flect the economics of the assets each entity holds.What if Entity A measures accounts receivable at fair value and property,plant,and equipment at modi fied historical cost,with all amounts in US dollars?Assume Entity A ’s property,plant,and equipment was purchased at various times over the last ten years.What if Entity B measures all assets at fair value and cash is stated in US dol-lars,accounts receivable is stated in euros,and property,plant,and equipment is stated in Swiss francs?Are these two entities comparable?Do the proportions of total assets each reports for the three assets re flect the economics of the assets?The answer is ‘no ’.How does a financial statement user compare Entity A ’s property,plant,and equip-ment with Entity B ’s?In addition,what do the 3000in total assets for Entities A and B represent?Are these amounts comparable to each other?Are they comparable to any-thing?Each 3000is the sum of three numbers derived on different bases.Like the sum of apples and oranges,its meaning is unclear.Many might react to this example by say-6BarthD o w n l o a d e d b y [124.207.132.50] a t 19:15 04 J u n e 2013ing that ‘we would never account for Entity B ’s assets using different currencies and that would be true ’.However,the distinction between using different currencies for Entity B ’s assets and using different measurements for different assets for Entity A is not clear.Why do we recoil at one and accept the other without question?One likely reason is that we are not accustomed to one but are accustomed to the other.Is there any other reason?Can we achieve comparability if we measure the same asset in different ways –either for the same entity or different entities?For example,presently we treat comput-ers as inventory for some entities (e.g.,Apple)and as equipment for others (e.g.,Gen-eral Electric).We treat warranty obligations relating to sales of goods by retailers differently from insurance contracts issued by insurance companies even though they are both insurance contracts.We treat real estate as investment property for some enti-ties (and measure it at fair value)and as property,plant,and equipment for others (and measure it at amortized cost).We treat the gain or loss on an item designated as hedged in a fair value hedge differently from the same gain or loss on an item that is not so designated (i.e.,in a designated fair value hedge,we adjust the carrying amount of the hedged item for its change in value attributable to the hedged risk).In addition,we per-mit optional asset revaluation,application of fair value,and hedge accounting itself.All of these differences result in differences in amounts in the financial statements for what seem to be the same assets and liabilities.Consider an example.Assume Entity A and Entity B each buys a piece of construc-tion equipment for 200.Entity A classi fies the equipment as inventory and Entity B classi fies it as property,plant,and equipment.Six months later,both entities still own the equipment;Entity A holds it in inventory and B has depreciated it because it is available for use,but Entity B has not used it.Entities A and B both dispose of the equipment for a gain.In its income statement,Entity A displays revenue and expense,which net to the amount of the gain,and Entity B displays the gain net.The asset –a piece of construction equipment –is the same for both entities.Yet,the asset is mea-sured at different amounts and the gain on disposal of the asset is presented differently.The question is whether the financial statements of Entities A and B are comparable.Consider another example,which is often used to illustrate this issue.Assume that Bank A and Bank B each buys US Treasury securities at a cost of US$1million.At the reporting date,the fair value of the securities is US$1.2million.Bank A classi fies the securities as trading (or fair value through pro fit or loss)and recognizes US$1.2million in assets and a gain of US$0.2million.Bank B classi fies the securities as held to maturity (or amortized cost)and recognizes US$1.0million in assets and no gain or loss.In both cases,the bank owns the same asset –US Treasury securities –purchased for the same amount –US$1million –and now worth the same amount –US$1.2million.Yet the financial statements of Bank A and Bank B are quite different.Does this financial report-ing make like things look alike?That is,are the financial statements of Entities A and B comparable?The answer is ‘no ’.Does ‘use ’of an asset affect its economics?A question relating to comparability to which we do not have a good answer is whether two assets that seem the same (e.g.,computers)are economically the same if they are used differently (e.g.,as inventory or property,plant,and equipment).If the use of an asset affects its economics,we need to identify how the economics are affected so that we can determine whether and how the differences in economics should be re flected China Journal of Accounting Studies 7D o w n l o a d e d b y [124.207.132.50] a t 19:15 04 J u n e 2013when accounting for the asset.Accountants have been treating seemingly similar assets differently for a long time –the inventory versus property,plant,and equipment exam-ple is not new.However,we have never articulated why.Without knowing why differ-ent uses of an asset affect the economics of the asset that should be re flected in the accounting,it is not possible to determine when and how to re flect such differences.In addition,we need to know whether the notion of different uses of assets applies to all assets.In particular,does it apply to financial assets and liabilities?IFRS 13concludes ‘no ’and explains why.But more thought needs to be devoted to this notion as it applies to non-financial assets.Recently,the notion that the use of assets should affect the accounting for the assets has been characterized as re flecting an entity ’s ‘business model ’.Thus,the question can be rephrased as whether different ‘use ’of an asset depends on the entity ’s ‘business model ’.Unfortunately,the Conceptual Framework has no concepts about the role of business model in financial reporting.In addition,there is no de finition of a business model and,thus,it is unclear what the term means.Some question whether an entity ’s business model differs in any substantive way from management intent (Leisenring,Lins-meier,Schipper,&Trott,2012).Is the business model something that management is doing,plans to do,or only hopes to do?Is an entity ’s business model veri fiable?Is it auditable?The answers to these questions are not obvious,which only adds to the lack of clarity about why and how a business model or intent should affect financial reporting.What does research say?Academic research provides evidence that global financial reporting with greater compa-rability can be bene ficial to investors,by lowering costs of comparing cross-border investment opportunities and,for some countries and firms,by improving the quality of their financial reporting information.Research also shows that comparability can be ben-e ficial to firms by increasing cross-border investment and by lowering cost of capital,presumably from reducing information risk and,for some,by increasing financial report-ing quality.However,research also provides evidence that these potential bene fits are tempered by cross-country differences in implementation,incentives,and enforcement.One example of a study in this literature is ‘Market Reaction to the Adoption of IFRS in Europe ’(Armstrong,Barth,Jagolinzer,&Riedl,2010).The questions motivat-ing this study are:(1)did investors perceive net bene fits to adoption of IFRS in Eur-ope?and (2)if there were net bene fits,are the net bene fits associated with increased comparability or increased quality of financial reporting information?As with any sin-gle research study,this study cannot directly answer these questions.However,these motivating questions lead to two research questions that the study can answer.The first is:did the European stock market react positively (negatively)to regulatory events that increased (decreased)the likelihood of IFRS adoption in Europe?An af firmative answer to this question indicates that investors perceived net bene fits to IFRS adoption in Eur-ope.The second is:were there differences across firms depending on their pre-adoption information environment?Identifying differences across firms in the market reaction to the regulatory events provides insights into what firm characteristics are associated with the perceived bene fits of IFRS adoption.The study focuses on 16regulatory events,which begin with the 2002European Par-liament resolution requiring all listed firms in the European Union to apply IFRS by 2005.The events end with the 2005European Commission endorsement of the revised fair value option.The events and predicted signs of the effect on the likelihood of IFRS adop-8BarthD o w n l o a d e d b y [124.207.132.50] a t 19:15 04 J u n e 2013。
(2000). ‘Investment-cash flow sensitivities are useful. A comment on Kaplan and Zingales’

INVESTMENT-CASH FLOW SENSITIVITIES ARE USEFUL:A COMMENT ON KAPLAN AND ZINGALES*S TEVEN M.F AZZARIR.G LENN H UBBARDB RUCE C.P ETERSENpaper in this Journal by Kaplan and Zingales reexamines a subset of firms ofFazzari,Hubbard,and Petersen and criticizes the usefulness of investment-cash flow sensitivities for detecting financing constraints.We show that the Kaplan and Zingales theoretical model fails to capture the approach employed in the literature and thus does not provide an effective critique.Moreover,we describe why their empirical classification system is flawed in identifying both whether firms are constrained and the relative degree of constraints across firm groups.We conclude that their results do not support their conclusions about the usefulness of investment-cash flow sensitivities.In a recent paper in this Journal Kaplan and Zingales [1997,hereinafter KZ]argue that investment-cash flow sensitivities do not provide useful evidence about the presence of financing constraints.Because KZ use a subset of the same firms and the same regressions as Fazzari,Hubbard,and Petersen [1988,hereinafter FHP]and claim [page 176]that FHP ‘‘can legitimately be considered the parent of all papers in this literature,’’it is appropriate that we respond.Based on a simple theoretical model,KZ reach the provocative conclusion [page 211]that ‘‘the invest-ment-cash flow sensitivity criterion as a measure of financial constraints is not well-grounded in theory.’’In Section I we show that the KZ model does not capture the theoretical approach employed in FHP and many subsequent studies.Most of the KZ paper attempts to show that empirical investment-cash flow sensitivities do not increase monotonically with the degree of financing constraints within the 49low-dividend firms from the FHP sample.In Section II we explain why the KZ classification of the degree of constraints is flawed in identifying both whether or not firms are constrained (absolute constraints)as well as the relative degree of constraints across firms.As a argue in Section III that there is no expected ex ante for the *WethankMichaelAthey,Charles Calomiris,Robert Carpenter,RobertChirinko,Mark Gertler,Simon Gilchrist,Kevin Hassett,Charles Himmelberg,Anil Kashyap,Ronald King,Wende Reeser,Joachim Winter,and two referees,one of the editors (Andrei Shleifer),and participants in seminars at the London Schoolof Economics and the NBER Summer Institute Conference on Corporate Finance for comments and suggestions.2000by the President and Fellows of Harvard College and the Massachusetts Institute of Technology.The Quarterly Journal of Economics,May 2000695 at Glasgow University Library on January 20, 2012/Downloaded frominvestment-cash flow sensitivities across the KZ categories,mak-ing their empirical results uninformative about the usefulness of investment-cash flow sensitivities.1I.T HE KZ M ODEL AND T ESTS OF F INANCING C ONSTRAINTSThe one-period KZ model consists of a return on investment F (I ),internal financing (W )with constant opportunity cost,external financing (E ),and a premium for external funds C (E ,k ),where k measures the cost wedge between internal and external funds.KZ show that the investment-cash flowsensitivity is(1)dI dW ϭC 11C 11ϪF 11,where C 11is the slope of the supply curve for external finance and F 11is of the investment demand curve.KZ focus on firm heterogeneity in dI /dW as measured by the level of W .To analyze dI /dW at different levels of W they compute(2)d 2I dW 2ϭ3F 111F 11ϪC 111C 114F 112C 112(C 11ϪF 11)3.KZ note that d 2I /dW 2is negative only if the term in brackets is negative.They then point out that the bracketed term could be positive if F 111Ͼ0or C 111Ͻ0.This leads KZ to conclude that the theoretical foundation of previous research is weak because dI /dW may not fall as the degree of financing constraints declines (with larger W ).Before we assess this conclusion,it is helpful to consider the intuition (which does not appear in KZ)behind why d 2may be positive.In Figure I investment is on the horizontal F 1is investment demand,W L or W H indicates the quantity financing (with constant marginal cost as indicated by the horizon-tal line segment),and C 1is the supply of external funds.In the left panel of Figure I,F 111ϭ0and C 111Ͻ0(i.e.,linear demand and concave supply).Investment is more sensitive to small internal finance fluctuations (⌬W )at high internal finance (W H )than at low internal finance (W L )because a firm at W H uses less external 1.Extensive empirical research since FHP (surveyed by Hubbard [1998])also addresses many of the issues raised in KZ.QUARTERLY JOURNAL OF ECONOMICS696 at Glasgow University Library on January 20, 2012/Downloaded fromfinancing,and thereforethe concavity of supply causes its C 11to be larger (see equation (1)).Alternatively,consider F111Ͼ0and C 111ϭ0(i.e.,convex demand and linear supply)as in the right panel of Figure I.Again,investment is more sensitive to W at W H than at W L because investment demand is more sensitive to the cost of capital as W rises.This focus in KZ on d 2I /dW 2does not provide an effective critique of the literature (including the FHP theoretical approach)because most studies do not use the level of W to classify firms.2Instead,FHP and much of the literature classify firms according to a priori criteria designed to give large differences in the slope of the external financing schedule,C 11,across groups.The obvious testable implication of this approach,using equation (1),is that constrained firms with a large C 11should have a larger dI /dW than (relatively)unconstrained firms with a small (or zero)C 11,other things equal.3The necessary condition for dI /dW to be larger for constrained firms is(3)C 11Constrained /C 11Unconstrained ϾF 11Constrained /F 11Unconstrained .2.In fact,KZ never reference any specific study,including FHP ,to demon-strate the relevanceofd 2I /dW 2.3.To appreciate the intuition graphically,consider the effect of a small changein W on two firms with linear demand curves.If the ‘‘constrained’’firmfacesrelatively steepand the ‘‘unconstrained’’firm relatively flat supply,the result isobvious.KZ implicitly assume away this possibility by positing that all firms face the same C 11for a given level of E . at Glasgow University Library on January 20, 2012/Downloaded fromWhile F 11may differ across firms,we can think of no reasons why F 11Constrainedshould be systematically greater thanF 11Unconstrained ,and KZ provide no reasons.Thus,as long as researchers separatefirms by a priori criteria in C 11Constrained ϾC 11Unconstrained inthe relevant range,the of dI /dW across firm groupshas a solid theoretical We also point out that as C 11Unconstrained approaches zero,as we argue below is the case in many studies,(3)almost certainly holds.In addition,if (3)holds,the issues that KZ raise about curvature and nonlinearity are not likely to be relevant.4The only remaining question is whether previous research has effectively classified firms in ways that generate large differ-ences in C 11.Consider the model and discussion in FHP [pages 146–157and Appendix A].In the supply of funds schedule in FHP Figure I,C 11equals zero for internal financing (as in KZ)and C 11is greater than zero for external financing.One group of firms faces C 11of zero at the margin because investment demand is less than internal financing.In contrast,constrained firms exhaust inter-nal funds and finance marginal investment with external funds,and thus face a positive C 11.Operationally,as implied by the model,unconstrained firms are those with large dividend payouts,and constrained firms are those with low or zero dividends.Since FHP ,many other researchers have devised different approaches for separating firms into groups with low and high C 11.5A common separating criterion is access to public debt.Calomiris,Himmelberg,and Wachtel [1995]report that firms with debt ratings are very different from firms without rated debt.Firms that issue public debt,especially commercial paper,are far larger on average,have much lower volatility of sales and income,and therefore pose relatively little,possibly negligible,default risk.The case can be made that firms with commercial paper or high bond ratings face a C 11close to zero.Almost surely,firms that issue public debt tend to have a substantially lower C 11than those 4.KZ also mention the possibility of ‘‘nonmonotonicity’’with the wedge k as a proxy for the degree of financing constraints.Thisapproach is not relevant to theFHP model,discussed in the next paragraph,because high-dividend firms,intheory,face no wedge at the margin.In general,if researchers effectively split theirsamples withcriteria that generate large differences in k that lead to large differences in C 11,the condition in equation (3)is likely to be satisfied.5.See Calomiris,Himmelberg,and Wachtel [1995];Gilchrist and Himmel-berg [1995,1998];Kashyap,Lamont,and Stein [1994];and Whited [1992].Hubbard [1998]provides many other references.Hoshi,Kashyap,and Scharfstein [1991]use association with a large bank to identify firms with a relatively low C 11.In addition,many studies split samples by firm size which is highly correlated with both dividend payout and access to public debt.QUARTERLY JOURNAL OF ECONOMICS698 at Glasgow University Library on January 20, 2012/Downloaded fromthat do not.In contrast,manyfirms without public debt also havearelikelyforcasecan be made that thesefirms face a high C11for externalfinancing.Empirical evidence from most studies is consistent with equation(1)in the sense thatfirms likely to have a priori high C11,(e.g.,firms with low dividends,no public debt,or small size)almost always have a larger dI/dW thanfirms likely to have a lowC11.Furthermore,many studies cannot reject dI/dW equals zerofor control groups selected to have a low(or zero)C11(e.g.,Gilchrist and Himmelberg[1995,1998]).6Thus,the implicationsof the theoretical approach in much previous research are sup-ported by the evidence.II.P ROBLEMS WITH THE KZ E MPIRICAL C LASSIFICATION A PPROACH KZ employ managerial statements and quantitative mea-sures fromfirms’financial statements to sort the49FHP low-dividendfirms into one offive groups:7Not Financially Con-strained(NFC),Likely Not Financially Constrained(LNFC), Possibly Financially Constrained(PFC),Likely Financially Con-strained(LFC),and Financially Constrained(FC).This section summarizes our concerns about the effectiveness of their ap-proach for determining both absolute and relative constraintsacrossfirms.on Managers’Statements and Regulation S-KTo justify use of managerial statements to identify the degreeoffinancing constraints,KZ[p.180]rely on Securities and Exchange Commission Regulation S-K which they claim‘‘explic-itly requiresfirms to disclose whether or not they are havingdifficultyfinancing their investments.’’It is not obvious,however,that this regulation forces afirm to revealfinancing constraints.We contacted Robert Lipe,Academic Fellow in the Office of theChief Accountant of the SEC and asked whether afirm that is6.See also Kashyap,Lamont,and Stein[1994].Some Euler equations studiescannot reject C11equal to zero for control groups offirms[Gilchrist1991;Hubbard, Kashyap,and Whited1995;Whited1992].7.KZ do not explain how these diverse criteria are specifically combined toclassifyfirms into thefive groups.COMMENT ON KAPLAN AND ZINGALES699at Glasgow University Library on January 20, 2012/Downloaded fromunable to undertake a new,positive NPV project due to financing constraints would be obliged to reveal this information.Lipe responded that this is not the case.Rather,he explained,Regula-tion S-K requires the firm to reveal the inability to invest due to financing constraints only when the firm fails to act on a previ-ously announced investment commitment.As a result,we doubt the relevance of self-serving managers’statements as evidence of the absence of financing constraints in most situations.B.Problems with the Quantitative Classification CriteriaThe classification criteria in KZ include cash stocks,unused lines of credit,and leverage.They report summary measures for these variables in Table III [KZ,pages 185–187]and argue that they support the success of their relative ranking of the degree of financing constraints and their finding that the firms face abso-lute financing constraints (PFC,LFC,or FC )in only 15percent of the firm-years.We begin by explaining why the summary statistics in KZ do not support their surprising finding about the infrequency of absolute constraints in the FHP sample.KZ suggest that both the cash flow and the cash stock positions for NFC and LNFC firm-years are so large relative to fixed investment that these firms could not be financially constrained.Their numbers in Table III,however,are misleading because they implicitly assume that firms use sources of financing only for fixed investment when,in fact,growing companies invest heavily in both inventories and accounts receivable (see Fazzari and Petersen [1993,pages 330–331]).We recomputed the KZ figures with the proper comparison of cash flow and cash stocks relative to total investment (fixed investment plus the changes in inventories and accounts receiv-able).These new statistics change some of the KZ conclusions.For example,KZ [page 184]note that the median value of cash flow less fixed investment is positive for NFC firm-years and write ‘‘[t]his suggests that NFC firms could have increased their invest-ment without tapping external sources of capital.’’In sharp contrast,in our computations the median value of cash flow less total investment is negative at the seventy-fifth percentile for even the NFC and LNFC firms.Thus,most NFC and LNFC firms exhaust all internal finance for investment purposes.Further-more,while the median cash stock-fixed investment ratio for NFC and LNFC firm-years is 0.66(similar to the statistics in KZ Table QUARTERLY JOURNAL OF ECONOMICS700 at Glasgow University Library on January 20, 2012/Downloaded fromIII)the median ratio of cash stocks to total investment is only 0.27.8ouropinion,this cash stock too small tosupport the in KZ of thefinancing constraints.constrained firms will maintain some buffer stock of cash to protect against having to cancel invest-ment projects as well as to avoid the costs with financial distress.It is well-known that cash volatile in manufacturing,frequently declining by 50percent or more and often becoming negative during a recession.Suppose,for example,that cash flow declined to zero.Our computations indicate that NFC and LNFC firms could maintain only about three months of median total investment from cash stocks,and then only if these stocks were (implausibly)driven to zero.We believe these statis-tics are consistent with the view that these firms face absolute financing constraints.are to provide them with credit,perhaps due to lack low-debt firms may therefore face more severe example,small high-tech companies—much of to have little collateral value,and little debt,possibly because their assets are intangible or firm-specific (see,for example,Himmelberg and Petersen [1994]).In addition,comparatively large cash positions or unused lines of credit may indicate relatively severe constraints.As argued in recent papers[Fazzari and Petersen 1993;Carpenter,Fazzari,and Petersen 1994;Calomiris,Himmelberg,and Wachtel 1995],it is costly for constrained firms to adjust fixed investment when internal funds fluctuate.Forward-looking firms will therefore partially protect themselves with buffer stocks of cash or unused debt capacity.The more financially constrained a firm is,the greater is its incentive to accumulate liquid buffer stocks.Such a firm may be able to invest more at the margin at a moment in time,but the firm is nonetheless financially constrained.This dynamic perspective contrasts with the static view of financing constraints employed by KZ,which creates problems in their classification approach.8.This statistic excludes observations for which totalinvestment is less than or equalto zero.KZ also point out that unused lines of credit are larger for NFC and LNFC firms.We do not have these data,but the ratios of slack to investment reported by KZ on page 188would be similarly reduced by recognizing a broader measure of MENT ON KAPLAN AND ZINGALES 701at Glasgow University Library on January 20, 2012/Downloaded fromC.The Absence of Heterogeneity in the KZ ClassificationOne striking finding in KZ is that only 19of 719observations (2.6percent)are FC and another 34observations (4.8percent)are LFC.Given so few FC and LFC observations,how do KZ obtain enough FC firms for their regressions?KZ placed firms in the FC category if they had just a single year (out of 15)with an FC or LFC rating.In the FC category,14of the 22firms had an FC or LFC rating only one or two times,while six firms had FC or LFC ratings in just three or four of the fifteen years.For this reason,the difference in cash flow coefficients across the KZ regressions may have little to do with their relative ranking of financing constraints.III.T HE KZ R EGRESSION R ESULTSKZ find that the investment of NFC and LNFC firms displays a greater sensitivity to cash flow than FC firms.Space does not permit a detailed discussion of this pattern of results.One possibility is that the FC firms include some years of financial distress.KZ describe firms in FC years as having ‘‘liquidity problems,’’which is not surprising given that their criteria for receiving the FC classification include violation of debt covenants and renegotiation of debt payments [page 182].The KZ summary statistics in Table III also strongly suggest that the FC firm-years are periods of financial distress.9During years of financial dis-tress,firms,possibly at the insistence of their creditors,are likely to use cash flow to enhance liquidity and avoid bankruptcy resulting in little change in fixed investment as measured in Compustat.A broader measure of investment,however,is likely to respond much more to cash flow for such firms.10Financial distress is one possible explanation for the low cash flow coefficient of the FC firms.Regardless of how one explains the 9.The mean cash flow-net plant ratio for these observations is Ϫ0.047and the mean of interest coverage is only 1.650.While KZ recognizethepossibility offinancial distressin FC observations [page 208],the defense they offer is notconvincing.They note that firms increase rather than repay debt in the PFC,LFC,and FC years.This observation,however,may be due to creditors permitting illiquid,but growing,firms to rebuild liquidity.10.Financially distressed firms (with low or negativecash flow)often disinvest assets with low adjustment costs such as working capital (see Fazzari and Petersen [1993]).In addition,such firms likely sell off existing fixed assets.Neither oftheseresponsesare included in the Compustat measure of fixedinvestment and ignoring them causes a downward bias in the cash flow coefficient,especially at times of financial distress.QUARTERLY JOURNAL OF ECONOMICS702 at Glasgow University Library on January 20, 2012/Downloaded frompattern of results in KZ,however,we argue that this pattern is not informative.As discussed in the previous section,the firms in the NFC and LNFC categories likely are financially constrained and the relative degree of constraints across the KZ categories is far from clear.If there is no clear a priori difference in financing constraints across the firm groups in KZ,their strategy does not meet the criterion (summarized by equation (3))necessary for meaningful tests of financing constraints with firm heterogeneity.Finally,KZ [page 196]present additional tests with group-ings based on ‘‘quantitative/objective data.’’The only one of these tests consistent with their main findings shows that firms with high interest coverage have higher cash flow coefficients than firms with low coverage.KZ imply that the pattern should be the opposite,but this need not be the case.As we discussed earlier,either low levels of debt or high interest coverage may indicate an inability to obtain debt financing,possibly signaling relatively severe financial constraints.KZ [page 211]themselves note that some studies use high leverage as an indicator of more severe financing constraints,while other studies argue the opposite.Thus,these tests do little to bolster the KZ conclusions.11IV .C ONCLUSIONKZ argue that investment-cash flow sensitivities do not provide useful evidence about the presence of financing con-straints.We believe that this conclusion does not follow from their analysis for two reasons.First,their theoretical model fails to capture the approach of most previous research,making their theoretical analysis irrelevant as a criticism of FHP and most subsequent research.Second,the KZ empirical findings are difficult to interpret.The 49low-dividend FHP firms are a poor choice for such a study because they are relatively homogeneous for purposes of testing for capital-market imperfections,making it extremely difficult to classify these firms finely by degree of financing constraints.Furthermore,some of the KZ classification 11.Two new studies are relevant to the KZ results.In a sample of large,dividend-paying firms,Cleary [1999]argues that the ‘‘most financially con-strained’’firms have the lowest investment-cashflow sensitivity.These FC firms,however,appear to be financially distressed.Their mean net income is Ϫ4.8percent of sales compared with 9.6percent for NFC firms.Mean sales growth forFC firmsis Ϫ2.3percent versus 23.5percent for the NFC firms.Winter [1998],using the KZ sample,includes the KZ indicator offinancial constraint status inregressions for investment and firm exit.He finds that the KZ indicator is either statistically insignificant or,when significant,has the wrong MENT ON KAPLAN AND ZINGALES 703at Glasgow University Library on January 20, 2012/Downloaded fromcriteria (e.g.,stock of cash and degree of leverage),may indicate high or low levels of constraints.We therefore believe their finding of nonmonotonic investment-cash flow sensitivities is not informative.While the sweeping critical conclusions in KZ do not follow from their results,we believe their paper makes a contribution.Empirical work in this area has not always clearly identified the theoretical model under investigation.While FHP provided a model of investment behavior that described the criteria for separating firms into ‘‘constrained’’and ‘‘unconstrained’’catego-ries,not all papers have done so.In addition,while commonly used separating criteria have a solid theoretical foundation,not all criteria are as defensible.KZ (and we hope this comment)will lead future researchers to clearly state their model and to carefully choose the criteria used for defining constrained and unconstrained groupings.W ASHINGTON U NIVERSITY AND J EROME L EVY E CONOMICS I NSTITUTEC OLUMBIA U NIVERSITY AND N ATIONAL B UREAU OFE CONOMIC R ESEARCHW ASHINGTON U NIVERSITYR EFERENCESCalomiris,Charles W.,Charles P .Himmelberg,and Paul Wachtel,‘‘Commercial Paper andCorporateFinance:A MicroeconomicPerspective,’’Carnegie-Rochester Conference Series on Public Policy,XLI (1995),203–250.Carpenter,Robert E.,Steven M.Fazzari,and Bruce C.Petersen,‘‘InventoryInvestment,Internal-Finance Fluctuations,and the Business Cycle,’’Brook-ings Papers on Economic Activity (1994:2),75–138.Cleary,Sean,‘‘The Relationship between Firm Investment and Financial Status,’’Journal of Finance,LIV (1999),673–692.Fazzari,Steven M.,R.Glenn Hubbard,and BruceC.Petersen,‘‘Financing Constraints and Corporate Investment,’’Brookings Papers on EconomicActivity (1988:1),141–195.Fazzari,Steven M.,and Bruce C.Petersen,‘‘Working Capital and Fixed Invest-ment:New Evidence on Finance Constraints,’’RAND Journal of Economics,XXIV (1993),328–342.Gilchrist,Simon,‘‘An Empirical Analysis of Corporate Investment and FinancingHierarchies Using Firm-Level Panel Data,’’mimeograph,Board of Governorsof the Federal Reserve System,1991.Gilchrist,Simon,and Charles P .Himmelberg,‘‘Evidence on the Role of Cash Flowfor Investment,’’Journal of Monetary Economics,XXXVI (1995),541–572.Gilchrist,Simon,and Charles P .Himmelberg,‘‘Investment,Fundamentals,andFinance,’’NBER Macroeconomics Annual,XIII (Cambridge,MA:MIT Press,1998).Himmelberg,Charles P .,and Bruce C.Petersen,‘‘R&D and Internal Finance:A Panel Study of Small Firms in High-Tech Industries,’’Review of Economicsand Statistics,LVI (1994),38–51.Hoshi,Takeo,Anil K.Kashyap,and David Scharfstein,‘‘Corporate Structure,Liquidity,and Investment:Evidence from Japanese Panel Data,’’QuarterlyJournal of Economics,CVI (1991),33–60.Hubbard,R.Glenn,‘‘Capital-Market Imperfections and Investment,’’Journal of Economic Literature,XXXV (March 1998),193–225.QUARTERLY JOURNAL OF ECONOMICS704 at Glasgow University Library on January 20, 2012/Downloaded fromHubbard,R.Glenn,Anil K.Kashyap,and Toni M.Whited,‘‘Internal Finance and Firm Investment,’’Journal of Money,Credit and Banking,XXVII (1995),683–701.Kaplan,Steven N.,and Luigi Zingales,‘‘Do Investment-Cash Flow Sensitivities Provide Useful Measures of Financing Constraints?’’Quarterly Journal ofEconomics,CXII(1997),169–215.Kashyap,Anil K,Owen mont,and Jeremy C.Stein,‘‘Credit Conditions andthe Cyclical Behavior of Inventories,’’Quarterly Journal of Economics,CIX(1994),565–592.Whited,Toni M.,‘‘Debt,Liquidity Constraints,and Corporate Investment:Evi-dencefrom PanelData,’’Journal of Finance,XLVII (1992),1425–1460.Winter,Joachim K.,‘‘Does Firms’Financial Status Affect Plant-Level Investment and Exit Decisions?’’mimeograph,University of Mannheim,MENT ON KAPLAN AND ZINGALES 705at Glasgow University Library on January 20, 2012/Downloaded from。
模拟器件ADVFC32电压转频 频率转电压转换器使用手册说明书
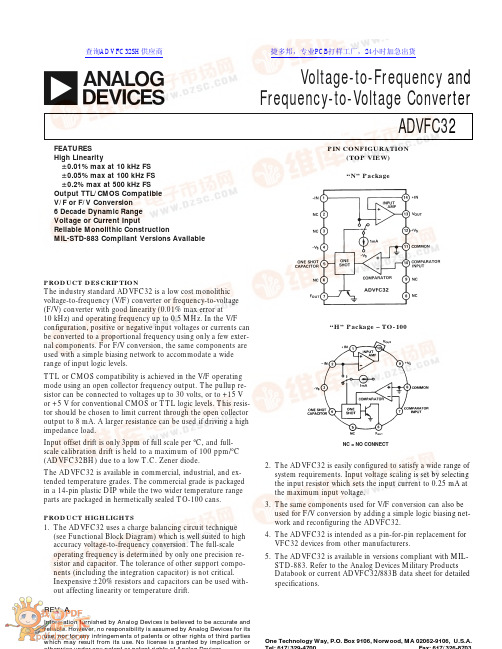
REV.AInformation furnished by Analog Devices is believed to be accurate and reliable. However, no responsibility is assumed by Analog Devices for its use, nor for any infringements of patents or other rights of third parties which may result from its use. No license is granted by implication oraVoltage-to-Frequency and Frequency-to-Voltage ConverterADVFC32One Technology Way, P.O. Box 9106, Norwood, MA 02062-9106, U.S.A.FEATURES High Linearity؎0.01% max at 10 kHz FS ؎0.05% max at 100 kHz FS ؎0.2% max at 500 kHz FS Output TTL/CMOS Compatible V/F or F/V Conversion 6 Decade Dynamic Range Voltage or Current InputReliable Monolithic ConstructionMIL-STD-883 Compliant Versions AvailablePRODUCT DESCRIPTIONThe industry standard ADVFC32 is a low cost monolithic voltage-to-frequency (V/F) converter or frequency-to-voltage (F/V) converter with good linearity (0.01% max error at 10 kHz) and operating frequency up to 0.5 MHz. In the V/F configuration, positive or negative input voltages or currents can be converted to a proportional frequency using only a few exter-nal components. For F/V conversion, the same components are used with a simple biasing network to accommodate a wide range of input logic levels.TTL or CMOS compatibility is achieved in the V/F operating mode using an open collector frequency output. The pullup re-sistor can be connected to voltages up to 30 volts, or to +15 V or +5 V for conventional CMOS or TTL logic levels. This resis-tor should be chosen to limit current through the open collector output to 8 mA. A larger resistance can be used if driving a high impedance load.Input offset drift is only 3ppm of full scale per °C, and full-scale calibration drift is held to a maximum of 100 ppm/°C (ADVFC32BH) due to a low T.C. Zener diode.The ADVFC32 is available in commercial, industrial, and ex-tended temperature grades. The commercial grade is packaged in a 14-pin plastic DIP while the two wider temperature range parts are packaged in hermetically sealed TO-100 cans.PRODUCT HIGHLIGHTS1.The ADVFC32 uses a charge balancing circuit technique (see Functional Block Diagram) which is well suited to high accuracy voltage-to-frequency conversion. The full-scale operating frequency is determined by only one precision re-sistor and capacitor. The tolerance of other support compo-nents (including the integration capacitor) is not critical.Inexpensive ±20% resistors and capacitors can be used with-out affecting linearity or temperature drift.PIN CONFIGURATION(TOP VIEW)“N” Package“H” Package – TO-100NC = NO CONNECT2.The ADVFC32 is easily configured to satisfy a wide range of system requirements. Input voltage scaling is set by selecting the input resistor which sets the input current to 0.25 mA at the maximum input voltage.3.The same components used for V/F conversion can also be used for F/V conversion by adding a simple logic biasing net-work and reconfiguring the ADVFC32.4.The ADVFC32 is intended as a pin-for-pin replacement for VFC32 devices from other manufacturers.5.The ADVFC32 is available in versions compliant with MIL-STD-883. Refer to the Analog Devices Military Products Databook or current ADVFC32/883B data sheet for detailed specifications.查询ADVFC32SH供应商捷多邦,专业PCB打样工厂,24小时加急出货ADVFC32–SPECIFICATIONS (typical @ +25؇C with V S= ؎15 V unless otherwise noted)Specifications shown in boldface are tested on all production units at final electrical test. Results from those tests are used to calculate outgoing quality levels. All min and max specifications are guaranteed,although only those shown in boldface are tested on all production units.ADVFC32KADVFC32BADVFC32SModelMin TypMax Min TypMax Min TypMax Units DYNAMIC PERFORMANCE Full Scale Frequency Range 050005000500kHz Nonlinearity 1f MAX = 10 kHz –0.01±0.01–0.01+0.01–0.01+0.01%f MAX = 100 kHz –0.05+0.05–0.05+0.05–0.05+0.05%f MAX = 0.5 MHz–0.20±0.05+0.20–0.20±0.05+0.20–0.20±0.05+0.20%Full-Scale Calibration Error (Adjustable to Zero)±5±5±5%vs. Supply(Full Scale Frequency = 100 kHz)–0.015+0.015–0.015+0.015–0.015+0.015%of FSR%vs. Temperature(Full Scale Frequency = 10 kHz)±75–100+100+150+150ppm/°CDYNAMIC RESPONSEMaximum Settling Time for Full Scale Step Input1 Pulse of New Frequency Plus 1 µs 1 Pulse of New Frequency Plus 1 µs 1 Pulse of New Frequency Plus 1 µs Overload Recovery Time 1 Pulse of New Frequency Plus 1 µs 1 Pulse of New Frequency Plus 1 µs 1 Pulse of New Frequency Plus 1 µsANALOG INPUT AMPLIFIER (V/F Conversion)Current Input Range 0+0.250+0.250+0.25mA Voltage Input Range–100–100–10V 20.250.250.25mA× R IN 3× R IN 3× R IN 3Differential Impedance 300 k Ω||10 pF 2 M Ω||10 pF 300 k Ω||10 pF 2 M Ω||10 pF 300 k Ω||10 pF 2 M Ω||10 pF Common-Mode Impedance 300 M Ω||3 pF750 M Ω||3 pF 300 M Ω||3 pF 750 M Ω||10 pF300 M Ω||3 pF 750 M Ω||10 pFInput Bias Current Noninverting Input 402504025040250nA Inverting Input –100±8+100–100±8+100 –100±8+100nA Input Offset Voltage(Trimmable to Zero)2, 3444mV vs. Temperature (T MIN to T MAX )303030µV/°CSafe Input Voltage±V S±V S±V SCOMPARATOR (F/V Conversion)Logic “0” Level –V S –0.6–V S –0.6–V S –0.6V Logic “1” Level +1+V S+1+V S+1+V S V Pulse Width Range 40.10.15/f MAX0.10.15/f MAX0.10.15/f MAX µsInput Impedance 50 k Ω||10 pF250 k Ω50 k Ω||10 pF250 k Ω50 k Ω||10 pF250 k ΩOPEN COLLECTOR OUTPUT (V/F Conversion)Output Voltage in Logic “0”I SINK = 8 mA0.40.40.4V Output Leakage Current in Logic “1”111µA Voltage Range+300+300+30V Fall Times (Load = 500 pF and I SINK = 5 mA)400400400ns AMPLIFIER OUTPUT (F/V Conversion)Voltage Range (0 mA ≤I O ≤7 mA)0+100+100+10V Source Current (0≤V O ≤7 V)101010mA Capacitive Load (Without Oscillation)100100100pF Closed Loop Output Impedance 111ΩPOWER SUPPLY Rated Voltage ±15±15±15V Voltage Range ±9±18±9±18±9±18V Quiescent Current 686868mA TEMPERATURE RANGE Specified Range 0+70–25+85–55+125°C Operating Range –25+85–55+125–55+125°C Storage –25+85–65+150–65+150°CPACKAGE OPTIONS Plastic DIP (N-14)ADVFC32KNTO–100 (H-10A)ADVFC32BH ADVFC32SHNOTES 1Nonlinearity defined as deviation from a straight line from zero to full scale, expressed as a percentage of full scale.2See Figure 3.3See Figure 1.4f MAX expressed in units of MHz.Specifications subject to change without notice.ADVFC32UNIPOLAR V/F, POSITIVE INPUT VOLTAGEWhen operated as a V/F converter, the transformation from voltage to frequency is based on a comparison of input signal magnitude to the 1 mA internal current source.A more complete understanding of the ADVFC32 requires a close examination of the internal circuitry of this part. Consider the operation of the ADVFC32 when connected as shown inFigure 1. At the start of a cycle, a current proportional to theFigure 1.Connection Diagram for V/F Conversion,Positive Input Voltageinput voltage flows through R3 and R1 to charge integration capacitor C2. As charge builds up on C2, the output voltage of the input amplifier decreases. When the amplifier output volt-age (Pin 13) crosses ground (see Figure 2 at time t 1), thecomparator triggers a one shot whose time period is determinedFigure 2.Voltage-to-Frequency Conversion Waveformsby capacitor C1. Specifically, the one shot time period (in nano-seconds) is:t OS ≅ (C l + 44 pF ) × 6.7 k ΩDuring this period, a current of (1 mA – I IN ) flows out of the in-tegration capacitor. The total amount of charge depleted during one cycle is, therefore (1 mA – I IN ) × t OS . This charge is replacedduring the remainder of the cycle to return the integrator to its original voltage. Since the charge taken out of C2 is equal to the charge that is put on C2 every cycle,(1 mA – I IN ) × t OS = I IN ×1FOUT–t OSor, rearranging terms,F OUT =I IN1mA ×t OS The complete transfer equation can now be derived by substi-tuting I IN = V IN /R IN and the equation relating C1 and t OS . The final equation describing ADVFC32 operation is:V II N /R IN1mA ×C 1+44pF ()×6.7k ΩComponents should be selected to optimize performance over the desired input voltage and output frequency range using the equations listed below:3.7×107pF /secF OUT FS –44pFC 2 =10–4Farads /secF OUT FS1000pF minimum ()R IN =V IN FS 0.25mA R 2 ≥+V LOGIC 8mABoth R IN and C 1 should have very low temperature coefficients as changes in their values will result in a proportionate change in the V/F transfer function. Other component values and tem-perature coefficients are not critical.CAUTIONESD (electrostatic discharge) sensitive device. Electrostatic charges as high as 4000V readily accumulate on the human body and test equipment and can discharge without detection.Although the ADVFC32 features proprietary ESD protection circuitry, permanent damage may occur on devices subjected to high energy electrostatic discharges. Therefore, proper ESD precautions are recommended to avoid performance degradation or loss of functionality.ORDERING GUIDEPartGain Tempco Temp Range Package Number 1ppm/؇C ؇C Option ADVFC32KN ±75 typ 0 to +7014-Pin Plastic DIP ADVFC32BH ±100 max –25 to +85TO-100ADVFC32SH±150 max–55 to +125TO-100NOTE 1For details on grade and package offerings screened in accordance withMIL-STD-883, refer to the Analog Devices Military Products Databook or current ADVFC32/883B data sheet.Table I. Suggested Values for C 1, R IN and C 2V IN FS F OUT FS C 1R IN C 21 V 10 kHz 3650 pF 4.0 k Ω0.01 µF 10 V 10 kHz 3650 pF 40 k Ω0.01 µF 1 V 100 kHz 330 pF 4.0 k Ω1000 pF 10 V100 kHz330 pF40 k Ω1000 pFADVFC32F/V CONVERSIONAlthough the mathematics of F/V conversion can be very com-plex, the basic principle is easy to understand. Figure 4 shows the connection diagram for F/V conversion with TTL input logic levels. Each time the input signal crosses the comparator threshold going negative, the one shot is activated and switches 1 mA into the integrator input for a measured time period (de-termined by C1). As the frequency increases, the amount of charge injected into the integration capacitor increases propor-tionately. The voltage across the integration capacitor is stabi-lized when the leakage current through R1 and R3 equals the average current being switched into the integrator. The net re-sult of these two effects is an average output voltage which is proportional to the input frequency. Optimum performance can be obtained by selecting components using the same guidelinesand equations listed in the V/F conversion section.Figure 4.Connection Diagram for F/V Conversion, TTL InputDECOUPLINGDecoupling power supplies at the device is good practice in any system, but absolutely imperative in high resolution applica-tions. For the ADVFC32, it is important to remember where the voltage transients and ground currents flow. For example,the current drawn through the output pulldown transistor origi-nates from the logic supply, and is directed to ground through Pin 11 (Pin 8 of TO-100). Therefore, the logic supply should be decoupled near the ADVFC32 to provide a low impedance re-turn path for switching transients. Also, if there is a separate digital ground it should be connected to the analog ground at the ADVFC32. This will prevent ground offsets that could be created by directing the full 8 mA output current into the analog ground, and subsequently back to the logic supply.Although some circuits may operate satisfactorily with the power supplies decoupled at only one location on each board,this practice is not recommended for the ADVFC32. For best results, each supply should be decoupled with 0.1 µF capacitor at the ADVFC32. In addition, a larger board level decoupling capacitor of 1 µF to 10 µF should be located relatively close to the ADVFC32 on each power supply.COMPONENT TEMPERATURE COEFFICIENTSThe drift specifications of the ADVFC32 do not include tem-perature effects of any of the supporting resistors or capacitors.The drift of the input resistors R1 and R3 and the timing ca-pacitor C1 directly affect the overall temperature stability. In theInput resistance R IN is composed of a fixed resistor (R1) and a variable resistor (R3) to allow for initial gain error compensa tion.To cover all possible situations, R3 should be 20% of R IN , and R1 should be 90% of R IN . This allows a ±10% gain adjustment to compensate for the ADVFC32 full-scale error and the toler-ance of C1.If more accurate initial offset is required, the circuit of R4 and R5 can be added. R5 can have a value between 10 k Ω and 100 k Ω, and R4 should be approximately 10 M Ω. The amount of current required to trim zero offset will be relatively small, so the temperature coefficients of these resistors are not critical. If large offsets are added using this circuit, temperature drift of both of these resistors is much more important.BIPOLAR V/FBy adding another resistor from Pin 1 (Pin 2 of TO-100 can) to a stable positive voltage, the ADVFC32 can be operated with a bipolar input voltage. For example, an 80 k Ω resistor to +10 V causes an additional current of 0.125 mA to flow into the inte-grator so that the net current flow to the integrator is positive even for negative input voltages. At negative full-scale input voltage, 0.125 mA will flow into the integrator from V IN cancel-ling out the 0.125 mA from the offset resistor, resulting in an output frequency of zero. At positive full scale, the sum of the two currents will be 0.25 mA and the output will be at its maxi-mum frequency.UNIPOLAR V/F, NEGATIVE INPUT VOLTAGEFigure 3 shows the connection diagram for V/F conversion of negative input voltages. In this configuration full-scale output frequency occurs at negative full-scale input, and zero outputfrequency corresponds to zero input voltage.Figure 3.Connection Diagram for V/F Conversion,Negative Input VoltageA very high impedance signal source may be used since it only drive the noninverting integrator input. Typical input imped-ance at this terminal is 250 M Ω or higher. For V/F conversion of positive input signals the signal generator must be able to source 0.25 mA to properly drive the ADVFC32, but for nega-tive V/F conversion the 0.25 mA integration current is drawn from ground through R1 and R3.Circuit operation for negative input voltages is very similar to positive input unipolar conversion described in the previous sec-tion. For best operating results use component equations listedADVFC32100 ppm/°C capacitor may result in a maximum overall circuit gain drift of:100 ppm/°C (ADVFC32BH) + 100 ppm/°C (C1)+ 10 ppm/°C (R IN ) = 210 ppm/°CAlthough R IN and C1 have the most pronounced effect on tem-perature stability, the offset circuit of resistors R4 and R5 may also have a slight effect on the offset temperature drift of the cir-cuit. The offset will change with variations in the resistance of R4 and supply voltage changes. In most applications the offset adjustment is very small, and the offset drift attributable to this circuit will be negligible. In the bipolar mode, however, both the positive reference and the resistor used to offset the signal range will have a pronounced effect on offset drift. A high quality reference and resistor should be used to minimize offset drift errors.Other circuit components do not directly influence temperature performance as long as their actual values are not so different from nominal value as to preclude operation. This includes integration capacitor C2. A change in the capacitance value of C2 results in a different rate of voltage change across C2, but this is compensated by an equal effect when C2 is discharged by the switched 1 mA current source so that no net effect occurs.The temperature effects of the components described above are the same when the ADVFC32 is configured for negative or bi-polar input ranges, or F/V conversion.OTHER CIRCUIT CONSIDERATIONSThe input amplifier connected to Pins 1, 13, and 14 is not a standard operational amplifier. Although it operates like an op amp in most applications, two key differences should be noted.First, the bias current of the positive input is typically 40 nAwhile the bias current of the inverting input is ±8 nA. Therefore,any attempt to cancel input offset voltage due to bias currents by matching input resistors will create worse offsets. Second, the output of this amplifier will sink only 1 mA, even though it will source as much as 10 mA. When used in the F/V mode, the am-plifier must be buffered if large sink currents are required.MICROPROCESSOR OPERATED A/D CONVERTERWith the addition of a few external components the ADVFC32can be used as a ±10 V A/D microprocessor front end. Although the nonlinearity of the ADVFC32 is only 0.05% maximum (0.01% typ), the resolution is much higher, allowing it to be used in 16-bit measurement and control systems where a mono-tonic transfer function is essential. The resolution of the circuit shown in Figure 5 is dependent on the amount of time allowed to count the ADVFC32 frequency output. Using a full scale fre-quency of 100 kHz, an 8-bit conversion can be made in about 10 ms, and a 2 second time period allows a 16-bit measurement,including offset and gain calibration cycles.As shown in Figure 5, the input signal is selected via the AD7590input multiplexer. Positive and negative references as well as a ground input are provided to calibrate the A/D. This is very im-portant in systems subject to moderate or extreme temperature changes since the gain temperature coefficient of the ADVFC32is as high as ±150 ppm/°C. By using the calibration cycles, the A/D conversion will be as accurate as the references provided.The AD542 following the input multiplexer provides a high im-pedance input (1012 ohms) and buffers the switch resistance from the relatively low impedance ADVFC32 input.If higher linearity is required, the ADVFC32 can be operated at 10 kHz, but this will require a proportionately longer conver-sion, time. Conversely, the conversion time can be decreased at the expense of nonlinearity by increasing the maximum fre-quency to as high as 500 kHz.HIGH NOISE IMMUNITY, HIGH CMRR ANALOG DATA LINKIn many applications, a signal must be sensed at a remote site and sent through a very noisy environment to a central location for further processing. In these cases, even a shielded cable may not protect the signal from noise pickup. The circuit of Figure 6provides a solution in these cases. Due to the optocoupler andFigure 5.High Resolution, Self-Calibrating, Microprocessor Operated A/D Convertervoltage-to-frequency conversion, this data link is extremelyinsensitive to noise and common-mode voltage interference. For even more protection, an optical fiber link substituted for the HCPL2630 will provide common-mode rejection of more than several hundred kilovolts and virtually total immunity to electri-cal noise. For most applications, however, the frequency modu-lated signal has sufficient noise immunity without using an opticalADVFC32C 754c –5–2/89P R I N T E D I N U .S .A.Figure 6.High Noise Immunity Data Linkfiber link, and the optocoupler provides common-mode isolation up to 3000 V dc.The data link input voltage is changed in a frequency modulated signal by the first ADVFC32. A 42.2 k Ω input resistor and a 100 k Ω offset resistor set the scaling so that a 0 V input signal corresponds to 50 kHz, and a 10 V input results in the maxi-mum output frequency of 500 kHz. A high frequency opto-coupler is then used to transmit the signal across any common-mode voltage potentials to the receiving ADVFC32. The opto-coupler is not necessary in systems where common-mode noise is either very small or a constant low level dc voltage. In systems where common-mode voltage may present a problem, the con-nection between the two locations should be through the opto-coupler; no power or ground connections need to be made.The output of the optocoupler drives an ADVFC32 hooked up in the F/V configuration. Since the reconstructed signal at Pin 10 has a considerable amount of carrier feedthrough, it is desir-able to filter out any frequencies in the carrier range of 50 kHz to 500 kHz. The frequency response of the F/V converter is only 3 kHz due to the pole made by the integrator, so a second 3 kHz filter will not significantly limit the bandwidth. With the simple one pole filter shown in Figure 6, the input to output 3 dB point is approximately 2 kHz, and the output noise is less than15 mV. If a lower output impedance drive is needed, a two pole active filter is recommended as an output stage.Although the F/V conversion technique used in this circuit is quite simple, it is also very limited in terms of its frequency re-sponse and output ripple. The frequency response is limited by the integrator time constant and while it is possible to decrease that time constant, either signal range or output ripple must be sacrificed. The performance of the circuit of Figure 6 is shown in the photograph below. The top trace is the input signal, the middle trace is the frequency-modulated signal at theoptocoupler’s output, and the bottom trace is the recoveredsignal at the output of the F/V converter.OUTLINE DIMENSIONSDimensions shown in inches and (mm).14-Pin Plastic DIP PackageTO-100 Package。
A note on reward-risk portfolio selection and two-fund separation
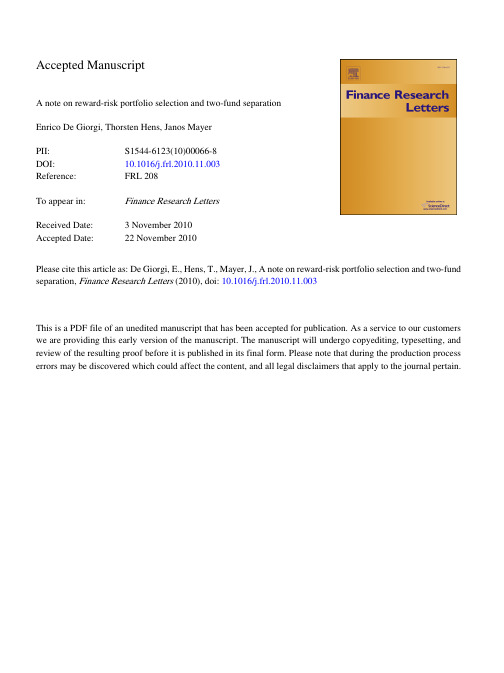
Accepted ManuscriptA note on reward-risk portfolio selection and two-fund separationEnrico De Giorgi, Thorsten Hens, Janos MayerPII:S1544-6123(10)00066-8DOI:10.1016/j.frl.2010.11.003Reference:FRL 208To appear in:Finance Research LettersReceived Date: 3 November 2010Accepted Date:22 November 2010Please cite this article as: De Giorgi, E., Hens, T., Mayer, J., A note on reward-risk portfolio selection and two-fund separation, Finance Research Letters (2010), doi: 10.1016/j.frl.2010.11.003This is a PDF file of an unedited manuscript that has been accepted for publication. As a service to our customers we are providing this early version of the manuscript. The manuscript will undergo copyediting, typesetting, and review of the resulting proof before it is published in its final form. Please note that during the production process errors may be discovered which could affect the content, and all legal disclaimers that apply to the journal pertain.A no te o n rew a rd-risk po rtfo lio selectio n a ndtw o-fund sepa ra tio nEnrico De G io rg i a,∗,T ho rsten Hens b,Ja no s M a y er ca Grou p for M athem atics and Statistics,University of St.GallenBodanstrasse6,C H-9000St.Gallen.b Sw iss Banking Institu te,University of Zu richPlattenstrasse32,C H-8032Z¨u rich.c Institu te for Operations R esearch,University of Zu richM ou ssonstrasse15,C H-8044Z¨u rich.15th Decem b er2010AbstractThis p ap er p resen ts a gen eral rew ard-risk p ortfolio selection m od el an d d eriv es su f-ficien t con d ition s for tw o-fu n d sep aration.In p articu lar w e show that m an y rew ard-riskm od els p resen ted in the literatu re satisfy these con d ition s.K eyw ords:Tw o-fu n d sep aration,rew ard-risk p referen ces.J EL Code:G11.1Corresp onding author.T el.+41+712242430,F ax.+41+712242894.Email ad d resses:enrico.degiorgi@unisg.ch(E nrico De G iorgi),thens@isb.uzh.ch(T horsten H ens) m ay er@ior.uzh.ch(Janos May er).11Intro ductio nThe modern portfolio theory of M arkowitz(1952)is a rich source of intuition and also the basis for many practical decisions.M ean-variance agents may differ with respect to the degree they are willing to trade offmean against variance but all will choose from the set of efficient portfolios,those which max imize mean given a constraint on variance.M oreover, under certain conditions,the mean-variance model of portfolio selection leads to two-fund separation(Tobin1958),i.e.,all agents hold a combination of the same portfolio of risky assets combined with the risk-free asset.Two-fund separation greatly simplifies the advice one should give to a heterogenous set of agents since the proportion of risky assets in the optimal portfolio is independent from agent’s risk aversion.M oreover,it implies a simple asset pricing structure in which a single risk factor ex plains the rewards agents get in equilibrium.W e derive sufficient conditions for two-fund separation in a general reward-risk model, where agents’preferences are assumed to be increasing functions of a reward measure and decreasing functions of a risk measure.W e show that two-fund separation holds if reward and risk measures can be transformed by means of strictly increasing functions into positive homogeneous,translation invariant or translation equivariant functionals.In this case,the efficient frontier is a straight line in the transformed reward-risk diagram.Several reward and risk measures introduced in the literature satisfy the conditions for two-fund separation.M ean and variance,semi-variance(M arkowitz1959),lower partial mo-ments(B awa and L indenberg1977,Fishburn1977,Harlow and Rao1989),the Gini measure (Yitzhaki1982),general deviation measures(Rockafellar,Uryasev,and Zabarankin2006), etc.Since many of these measures are defined based only on few principles of rational-ity,it follows that two-fund separation is a common property to many rational mean-risk models,including even those which are consistent with second order stochastic dominance (De Giorgi2005).This result is surprising because strong conditions on agents’utility func-tions are needed in order to obtain two-fund separation within ex pected utility theory(C ass and Stiglitz1970).In Section2we introduce the general reward-risk model and derive our main result.E x amples are discussed in Section2.All proofs are given in the Appendix.2Ge ne ral re w ard-risk m o de l and tw o-fund se paratio nW e consider a two-period economy.The set S of states of the world in the second period is endowed with a sigma-algebraΣ.An element s∈S is an individual state of the world, while A∈Σis an event.A random variable on(S,Σ)is a real-valued function X:S→R such that X−1(J)∈Σfor all intervals J⊂R.The space of random variables on(S,Σ)is denoted by L0(S,Σ).W e don’t assume ex istence of a probability measure on(S,Σ),since our conditions for two-fund separation do not require any assumption on the distribution of2random variables.There are K +1assets with random payoffs A k ∈L 0(S,Σ)and prices q k ,k =0,...,K .Asset 0is the risk-free asset with A 0=1,q 0>0,and gross return R 0=1/q 0.We assume that assets can be traded without restrictions:the marketed subspace is denoted by X ={ K k =0θk A k |(θ0,...,θK ) ∈R K +1}.An element X ∈X is called a portfolio.For X = K k =0θk A k ∈X we denote by q (X )= K k =0θk q k the price of portfolio X .We introduce two real-valued functions µ:X →R and ρ:X →R .We call µa reward measure and ρa risk measure.It is plausible to assume that µ(X )≥µ(Y )and ρ(X)≤ρ(Y )when X (s )≥Y (s )for all s ∈S .We don’t make these monotonicity assumptions here since they are not necessary for our main result and they would exclude from our framework the mean-variance model (variance violates monotonicity).The basic properties of reward and risk measures we consider are the following.Definition 1.L et ζ:X →R be a real-valued function on X (ζis a reward or a risk measure).W e say that ζis:(i)(positive)homogeneous of degree γifζ(κX )=|κ|γζ(X )for all κ∈R (κ≥0)and X ∈X ,(ii)translation invariant ifζ(X +a )=ζ(X )for all a ∈R and X ∈X ,(iii)translation equivariant ifζ(X +a )=ζ(X )+afor all a ∈R and X ∈X ,orζ(X +a )=ζ(X )−afor all a ∈R and X ∈G .In the first case,we say that ζis positive translation equivariant,while in the second case it is negative translation equivariant.When reward or risk measures are translation invariant,then adding a risk-free position to the portfolio doesn’t change risk or reward.By contrast,translation equivariant implies that adding a risk-free position increases (positive translation equivariant)or decreases (negative translation equivariant)reward or risk by the same amount as the risk-free addition.Negative translation equivariance is often assumed for risk measures that are interpreted as risk capital requirement;see F¨o llmer and Schied (2002).P ositive translation equivariance and translation3invariance are satisfied by many reward and risk measures used in reward-risk models for portfolio selection.An agent has initial wealth w0and wants to choose from X.She possesses a utility function U:X→R which satisfies the following assumption:Assum ption1((µ,ρ)-preferences).U(X)=v(µ(X),ρ(X))for all∈X where v:R2→R,(µ,ρ)→v(ρ,µ)is continuously differentiable,strictly increas-ing inµ,strictly decreasing inρ,and concave.Assumption1simply states that agents evaluate random payoffs only through the reward measureµand the risk measureρ,respectively,and are risk averse.Preferences according to Assumption1are called general reward-risk preferences(or simply(µ,ρ)-preferences).The agent solves the following decision problemU(X)such that q(X)≤w0,(1)maxX∈Xi.e.,she maximizes her utility given the budget constraint.Given the purpose of this paper to characterize solutions of the maximization of reward-risk preferences we make the following additional assumption:Assum ption2(Existence of solutions with bounded reward and risk).Problem(1)possesses a solution X ∈X withµ(X )<∞andρ(X )<∞.The following holds:Proposition1.Under Assumptions1and2,X solves Problem(1)if and only if there exists¯ρ∈R such that X solvesµ(X)such thatρ(X)≤¯ρand q(X)≤w0.(2) maxX∈XWe are now ready to prove our main result:Theorem1(Two-fund separation).Letµ:X→R andρ:X→R be a reward and risk measure,respectively,on X.Suppose that strictly increasing transformations Tµ:R→R, Tρ:R→R+exist such that˜µ=Tµ◦µand˜ρ=Tρ◦ρare positive homogeneous of degree 1,and translation invariant or translation equivariant.T hen(µ,ρ)-preferences satisfy two-fund separation,i.e.,there exists X ∈X,such that for all utility functions U that satisfy Assumptions1and2with reward measureµand risk measureρ,there exist parameters αU,αU0∈R,αU≥0,such thatαU X +αU0solves Problem(1).4Theorem1states that two-fund separation holds in general reward-risk models when the efficient frontier is a straight line in the reward-risk diagram,after transforming reward and risk measures by means of strictly increasing transformations.Increasing transformations change the risk-reward trade-offbut not the ranking of asset payoffs,thus the transformed reward-risk model delivers the same efficient frontier as the original reward-risk model.Many reward and the risk measures are themselves positive homogeneous so that it makes sense to emphasize this case.Corollary1.Letµ:X→R andρ:X→R+be reward and risk measures,respectively,on X.Suppose that one of the following two properties holds forµandρ:(i)nonnegative on X,translation invariant and(positive)homogeneous of degreeγ>0, (ii)translation equivariant and(positive)homogeneous of degree1.Then under Assumption2,(µ,ρ)-preferences satisfy two-fund separation.2ExamplesIn this section we provide examples of reward-risk models that satisfies two-fund separation. We will assume that(S,Σ)is endowed with a probability measure P and a X∈L0(S,Σ)has cumulative distribution function F X(x)=P X≤x for all x.Example1(Mean-variance model(Markowitz1952)).We observe that the mean is posi-tive homogenous and positive translation equivariant,and that the variance is nonnegative, translation invariant,and positive homogeneous of degree2.Example2(Mean-Semi-variance model(Markowitz1959)).F or a random variable X∈L0(S,Σ)the semi-variance is defined assv(X)= R min(0,x−E X ) 2d F X(x).Semi-variance is nonnegative,translation invariant,and positive homogenous of degree2. Example3(Mean-lower partial moment model(Bawa and Lindenberg1977)).F or a random variable X∈L0(S,Σ)the lower partial moment with target wealthτ:X→R is defined by:LPM(X;α,τ)= R[max(0,τ(X)−x)]αd F x(x)(4)whereα≥1.Here we assume that the targetτ(X)might depend on X.One example of target function is the risk-free return,i.e.,τ(X)=R0q(X)corresponds to thefinal wealth when the price q(X)of portfolio X is invested on the risk-free asset instead of X.Another5The piecew ise-pow er fu nction su ggested by Tversky and Kahneman(1992)v(x)= xα,x≥0−λ(−x)β,x<06forλ≥1andα,β∈(0,1),is positive homogenous both on x<0and x>0.M oreover, the reference pointτ(X)=R0q(X)(see Example3is positive homogeneous and positive translation equivariant.Thus,the behavioral reward-risk model where v is the piecewise-power function of Tversky and Kahneman(1992)and the reference point is the risk-free return,satisfies two-fund separation.This observation generalizes to any distribution the result of B arberis and Huang(2008),who show that two-fund separation holds with cumulative prospect theory preferences,when all assets are assumed to be normally distributed. Ackno w le dg e m e ntFinancial support from the Foundation for Research and Development of the University of Lugano and the National C enter of C ompetence in Research“Financial V aluation and Risk Management”is gratefully acknowledged.W e are grateful to T hierry P ost,Hersh S hefrin, Meir S tatman and S iegfried T rautmann for their useful comments.Proof AA.1P ro o f o f P ro p o sitio n1Let X b e a solution to P rob lem(1)withρ(X )<∞and set¯ρ=ρ(X ).S uppose that X∈X exists with q(X)≤w0,ρ(X)≤¯ρandµ(X)>µ(X ).S ince U is strictly increasing inµand strictly decreasing inρ,then U(X)>U(X ),a contradiction to the optimality of X for P rob lem(1).On the other hand,let X (¯ρ)b e the optimal solution to P rob lem(2),when the upper b ound forρis¯ρ∈R.Assume that wefind X such that q(X )≤w0and U(X )>U(X (¯ρ)) for all¯ρ∈R.T henρ(X )=∞,a contradiction to Assumption2.If wefind¯ρ∈R,such that ρ(X )=¯ρ,thenµ(X (¯ρ))≥µ(X ).Moreover,without loss of generality,we can assume thatρ(X (¯ρ))=¯ρ.T herefore,since U strictly increases inµand strictly decreases inρ,we must have U(X (¯ρ))=U(X ),a contradiction.A.2P ro o f o f T he o re m1P roposition1implies that P rob lems(1)and(2)are eq uivalent.W e thus consider solutions to P rob lem(2).S ince Tµand Tρare strictly increasing,P rob lem(2)is eq uivalent tomax˜µ(X)such that˜ρ(X)≤ˆρand q(X)≤w0(3) X∈Xwhereˆρ=Tρ(¯ρ)≥0.78Markowitz,H.,1952.Portfolio selection.Journal of Finance7,77–91.Rockafellar,R.T.,Uryasev,S.,Zabarankin,M.,2006.Generalized deviations in risk analysis.Finance and Stochastics10,51–74.9Tobin,J.,1958.Liquidity preference as behaviour towards risk.Review of Economic Studies 25,65–86.Tversky,A.,K ahneman,D.,1992.Advances in prospect theory:Cumulative representation of uncertainty.Journal of Risk and Uncertainty5,297–323.Yitzhaki,S.,1982.Stochastic dominance,mean variance,and gini’s mean difference.The American Economic Review72,178–185.10。
- 1、下载文档前请自行甄别文档内容的完整性,平台不提供额外的编辑、内容补充、找答案等附加服务。
- 2、"仅部分预览"的文档,不可在线预览部分如存在完整性等问题,可反馈申请退款(可完整预览的文档不适用该条件!)。
- 3、如文档侵犯您的权益,请联系客服反馈,我们会尽快为您处理(人工客服工作时间:9:00-18:30)。
1 Introduction
Nonlinear time series models have been used extensively in recent years to model complex dynamics that cannot be adequately represented using a linear model. Their use has been confined mainly to individual series, due to the additional complexity involved in modeling multivariate, correlated systems. There are examples, however, in which the relationship between series appears to be nonlinear. Hipel and McLeod (1994) hypothesize that, although a linear model may be adequate to describe average annual river flows, the relationship between daily river flows and precipitation may be nonlinear. Tong (1990) provides a nice review of nonlinear time series modeling, primarily in the univariate setting. Chen and Tsay (1993, 1996) and Lewis and Ray (1997) investigate techniques for obtaining semi-multivariate nonlinear models, in the sense that a single output series is modeled using several input series. Although briefly alluded to by Tong (1990, p.429) and Hipel and McLeod (1994, p.758), techniques for vector nonlinear time series modeling have only recently begun to be investigated. Granger and Tera¨svirta (1993) mention vector nonlinear autoregressive processes, vector nonlinear moving average processes, and multiple bilinear time series models in passing, but concentrate on statistical inference for univariate nonlinear models. A recent paper by Tsay (1997) discusses testing and modeling multivariate threshold autoregressive models; Wong and Li (1997) discuss vector processes having conditional heteroscedasticity.
2∞
2∞
fi( 1,t−1, 2,t−1ukl s,t−k u,t−l
(2)
s=1 k=1
s,u=1 k,l=1
2
∞
+
bi,suvklm s,t−k u,t−l v,t−m + · · · .
s,u,v=1 k,l,m=1
This representation is a generalization of the Volterra representation of a nonlinear stationary univariate time series. See, for example, Priestley (1988, p.26). In practice, a truncated representation involving a finite number of parameters is used to approximate the above structure. A bivariate linear process results if all the coefficients of the second and higher-order terms in (2) equal zero. Extensions of (1) and (2) to a general k-variate time series are clear.
jharvil@ and Bonnie K. Ray Dept. of Mathematical Sciences and Center for Applied Math and Statistics, New Jersey Institute of Technology, Newark, NJ 07102
borayx@
Summary
We investigate tests to detect general nonlinear behavior in a vector time series. The proposed tests are multivariate extensions of the univariate nonlinearity tests of Keenan (1985) and Tsay (1986). Simulation results show that, in general, the multivariate tests are more powerful than their univariate counterparts, especially for series having nonlinear structure that involves several components of the vector process and process error terms that are weakly or moderately cross-correlated. For illustration, we apply the tests to several standard multivariate data sets. We then analyze a set of seasonally adjusted quarterly capital expenditures and appropriations in U.S. manufacturing over the period 1953 to 1974 in more detail, to illustrate how one might build a vector nonlinear model and the gains that may achieved from allowing for nonlinear behavior in a vector series. Some key words: Likelihood Ratio; Multivariate time series; Nonlinearity
Before investigating specific nonlinear models for vector time series, however, it is desirable to have a test for general vector nonlinear structure. Univariate tests for examining particular types of nonlinear structure in individual series have been well studied. See, for example, De Gooijer and Kumar (1992) or Harvill (1998) for a review. But even if the components of a vector series exhibit nonlinear structure individually, a more powerful test of nonlinearity may be obtained if the component series are tested jointly. In this paper, we propose a multivariate test for general nonlinear structure in a vector series and show that, in most cases, it is more
(1)
X2,t = µ2 + f2( 1,t−1, 2,t−1, . . .) + 2,t,
where { 1,t} and { 2,t} are serially uncorrelated, but may be cross-correlated at lag zero, and f1(·), f2(·) are measurable real-valued functions. In general, fi(·), i = 1, 2 can be represented by a Volterra series of the form
2
powerful than the corresponding univariate test applied to each component series individually. Section 2 outlines the multivariate nonlinear framework considered in this paper, while Section 3 gives the new test statistic. Section 4 provides numerical comparisons of power for the univariate and multivariate test statistics based on simulations. Section 5 illustrates the tests on several real data sets and analyzes the multivariate nonlinear structure for one of the data sets in more detail. Some concluding remarks are given in Section 6.