Probabilistic versus Possibilistic Fuzzy Clustering An Unifying Model
英文速读资料金融时报

经济预测,欲望永不眠辞旧迎新的时候,人们为何总期望经济学家做预测呢?预测成绩怎样?这是FT"卧底经济学家"蒂姆·哈福德有趣的解答。
An insatiable desire to peer into the futureThe wonderful thing about forecasts is that they all sound very profound--------------------------It’s that time of year again. Time for you to make your predictions for 2013.You’re kidding, right? You’re asking an economist for predictions?Just my little joke. But surely you’re not a propereconomist if you can’t make a few predictions. Isn’t that the whole point of the economic profession – to make dozens of mutually contradictory forecasts with impunity?Well, the impunity is a topic worth discussing. But the economics profession could do with a few more disagreements, I think. In 1995, FT columnist John Kay examined the record of British economic forecasters from 1987 to 1994. He discovered that they tended to all say much the same thing. The only dissenter was reality: economic growth often fell outside the range of all 34 forecasts.So economists are terrible forecasters. What else is new?It isn’t just economists who are terrible forecasters. Take the quantitative analysts responsible for Goldman Sachs’s notorious “25 standard deviation” episode – presumably physicists or mathematicians.25 standard deviation?At the beginning of the financial crisis, the chief financial officer of Goldman Sachs explained that the firm was seeing “25 standard deviation moves, several days in a row” – a statement that, translated into English, means “according to our models, what we’re seeing is very unlucky”.How unlucky?Oh, the sort of bad luck you see once every 28, 900, 000, 000, 000, 000, 000, 000, 000, 000, 000, 000, 000, 000, 000, 000, 000, 000, 000, 000, 000, 000, 000, 000, 000, 000, 000, 000, 000, 000, 000, 000, 000, 000, 000, 000, 000, 000, 000, 000, 000, 000, 000, 000, 000, 000, 000, 000, 000, 000, 000, 000, 000, 000, 000, 000, 000, 000, 000, 000, 000, 000, 000, 000, 000, 000, 000, 000, 000, 000, 000, 000, 000, 000, 000, 000, 000, 000, 000, 000, 000, 000, 000, 000, 000, 000, 000, 000, 000, 000, 000, 000, 000, 000, 000, 000, 000, 000, 000, 000, 000, 000, 000, 000, 000, 000, 000, 000, 000, 000, 000, 000, 000, 000, 000, 000, 000, 000, 000, 000, 000, 000, 000, 000, 000, 000, 000, 000, 000, 000, 000, 000, 000, 000, 000, 000,000, 000, 000, 000, 000, 000, 000, 000, 000, 000, 000, 000, 000, 000, 000, 000, 000, 000, 000, 000, 000, 000, 000, 000, 000, 000, 000, 000, 000, 000, 000, 000, 000, 000, 000, 000, 000, 000, 000 years, given certain assumptions about what Goldman might have meant. For reference the universe is about 14,000,000,000 years old. The alternative to the “very unlucky” hypothesis, of course, is that the quantitative models didn’t produce very good forecasts.Well, that’s a forecast so bad that I can’t believe an economist wasn’t involved somewhere.You may be right. But I can give you another example: the 300-odd experts recruited by Philip Tetlock, the psychologist, for his epic study of forecasting in political science. Prof Tetlo ck’s conclusions are wide-ranging and painstaking, but if I can be forgiven an excessively brief summary, he finds that all sorts of people with plausible claims to expertise –diplomats, political advisers, journalists and academics –produce lame forecasts of political and economic events.Nate Silver seems to be able to forecast just fine.Well, yes, notwithstanding the politically motivated “Nate Silver can’t add up” school of criticism, Mr Silver, and other statisticians such as Drew Linzer and Sam Wang, successfully forecast the outcome of the US elections in some detail. Contrasted against a background of bloviation, it was impressive. But if psephology is Exhibit A in the Museum of Successful Social Science Forecasts, let’s reflect on how modest our ambitions must have become: US elections are frequently repeated, with behaviour that shows considerable historical persistence, and an astonishing amount of detailed quantitative data are available. The elections take place at a fixed date, according to well-understood rules, and with a narrowly defined space of possible outcomes. It’s easy to see that forecasting a win for Barack Obama, while better than forecasting a win for Mitt Romney, is not quite as hard as successfully predicting if and when Greece will leave the eurozone.You’re pretty quick with the excuses.No excuses. We just can’t see into the future. I don’t thinkthat’s any surprise, nor an embarrassment. The question is why there’s such a hunger for social science predictions, when the practice is so transparently pointless.It’s a test of expertise.If so, then monkeys are as expert as professors of political economy. I wouldn’t want to be quite so cynical. I think forecasting in a complex world is a poor test of expertise because luck is the overwhelming success factor.So why do we love predictions?No idea. Here’s one guess: saying “the UK economy will recover strongly in 2012” or “President Assad will be out of office by June” compresses a vast amount of expertise and analysis into a few words.But the words are probably meaningless.Yes. But it’s Christmas. Actually studying the situation in detail is far too much like hard work真是费神的事. Thewonderful thing about a forecast is that both the forecaster and his audience feel that something profound has been expressed. And nobody will remember the forecast anyway.It’s time of …….time for; but surely you…..if you …….; Isn’t that the whole point of …….Well, but……..I think.So…… what else is new?It isn’t just , presumably……;But if I can be forgivenWell yes,notwithstandingAs hard asIf and whenIf so….共和党旗手埃里克·坎托年轻的国会共和党领袖坎托是个野心勃勃和勤奋异常的人,FT华盛顿分社社长马利德(Richard McGregor)认为他是共和党的新旗手。
Fuzzy Logic - PCU Teaching Staffs模糊逻辑与教学人员
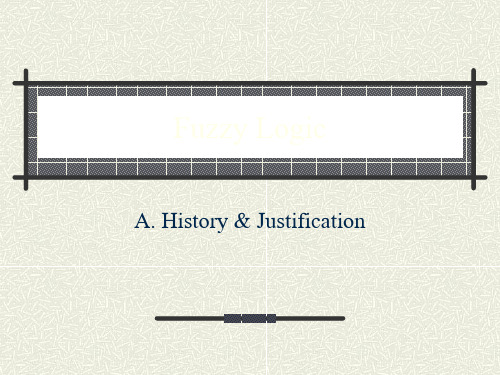
e.g. On a scale of one to 10, how good was
the dive?
10
9
9
9.5
Fuzzy Probability
Example #1
Billy has ten toes. The probability Billy has nine toes is zero. The fuzzy membership of Billy in the set of people with about nine toes, however, is nonzero.
Looking at Fuzzy Logic
4. Degree of Membership (Fuzzy Linguistic Variables)
Fuzzy and “Crisp” Control
Examples include close, heavy, light, big, small, smart, fast, slow, hot, cold, tall and short.
Gödel showed all such theories are either incomplete or inconsistent.
Gödel & Einstein (Princeton: August 1950)
Gödel’s Proof
Inconsistent: Show that 1+1=2 and 1+12. Incomplete: We cannot show that 1+1=2 .
Is such logic more compatible with Asian philosophy?
1Cor 13:12 “For now we see through a glass, darkly; but then face to face: now I know in part; but then shall I know even as also I am known.”
超实用备战高考英语考试易错题——阅读理解:主旨大意题(大陷阱) (解析版)

易错点17 阅读理解主旨大意题目录01 易错陷阱(3大陷阱)02 举一反三【易错点提醒一】标题类易混易错点【易错点提醒二】段落大意类易混易错点【易错点提醒三】文章大意类易混易错点03 易错题通关养成良好的答题习惯,是决定高考英语成败的决定性因素之一。
做题前,要认真阅读题目要求、题干和选项,并对答案内容作出合理预测;答题时,切忌跟着感觉走,最好按照题目序号来做,不会的或存在疑问的,要做好标记,要善于发现,找到题目的题眼所在,规范答题,书写工整;答题完毕时,要认真检查,查漏补缺,纠正错误。
易错陷阱1:标题类易混易错点。
【分析】标题类是对中心思想的加工和提炼,可以是单词、短语、也可以是句子。
她的特点是短小精悍,多为短语;涵盖性、精确性强;不能随意改变语言表达的程度和色彩。
如果是短语类选项,考生容易混淆重点,此时应当先划出选项的关键词。
此类题和文章的中心主题句有很大关系。
中心主题句一般出现在第一段,有时第一段也可能引出话题,此时应当重点关注第二段和最后一段,看看是否会出现首尾呼应。
易错陷阱2:段落大意类易混易错点。
【分析】每个段落都有一个中心思想,通常会在段落的第一句或最后一句体现,这就是段落主题句。
如果没有明显的主题句时,应当根据段落内容概括处段落大意。
有时考生还会找错文章对应位置,盲目选词文中相同的词句,而出现文不对题的现象。
易错陷阱3:文章大意类易混易错点。
【分析】确定文章主旨的方法是:先看首尾段或各段开头再看全文找主题句,若无明显主题句,就通过关键词句来概括。
如,议论文中寻找表达作者观点态度的词语,记叙文中寻找概括情节和中心的动词或反映人物特点的形容词。
文中出现不同观点时,要牢记作者的观点彩色体现全文中心的。
此时,要注意转折词,如:but, however, yet, in spite of, on the contrary等。
【易错点提醒一】标题类易混易错点【例1】(浙江省义乌五校2023-2024学年高三联考试题)The scientist’s job is to figure out how the world works, to “torture (拷问)” Nature to reveal her secrets, as the 17th century philosopher Francis Bacon described it. But who are these people in the lab coats (or sports jackets, or T-shirts and jeans) and how do they work? It turns out that there is a good deal of mystery surrounding the mystery-solvers.“One of the greatest mysteries is the question of what it is about human beings — brains, education, culture etc. that makes them capable of doing science at all,” said Colin Allen, a cognitive scientist at Indiana University.Two vital ingredients seem to be necessary to make a scientist: the curiosity to seek out mysteries and the creativity to solve them. “Scientists exhibit a heightened level of curiosity,” reads a 2007 report on scientific creativity. “They go further and deeper into basic questions showing a passion for knowledge for its own sake.” Max Planck, one of the fathers of quantum physics, once said, the scientist “must have a vivid and intuitive imagination, for new ideas are not generated by deduction (推论), but by an artistically creative imagination.”......ong as our best technology for seeing inside the brain requires subjects to lie nearly motionless while surrounded by a giant magnet, we’re only going to make limited pro gress on these questions,” Allen said.What is a suitable title for the text?A.Who Are The Mystery-solversB.Scientists Are Not Born But MadeC.Great Mystery: What Makes A ScientistD.Solving Mysteries: Inside A Scientist's Mind【答案】C【解析】文章标题。
虚无悖论

上节提及,资产的升值代表着财富累积的增加,因此,所有资产皆可以看为财富累积的仓库。问题是,在费雪的传统中,资产有生产力,带来租值或收入,而这些收入以利率折现是资产的价值,称财富。本身毫无生产力的资产也是资产,但我们无从以其产出的收入折现。这类资产的主要用场是累积财富,当年费雪没有分析过。以「仓库」来描述这类资产是恰当的,虽然其他有生产力的资产也是财富累积的仓库。-
这里顺便一提。在同类的收藏品中,那些所谓「精品」的,在市价一般上升时其升幅的百分率通常比较高,而市价一般下降时其跌幅百分率比较小。不是永远如是,是或然率如是。有两个原因。其一是精品通常不多,其存在市场通常知道。比较平庸的不仅远为量大,其总量究竟有多少市场通常不知道。其二,称得上是精品的,假冒远为困难,出现赝品的机会比较少。-
从上述的四个需要的条件看,一个健全的收藏品仓库的形成可真不易,而正因为得之不易,失之也难。一个健全的收藏仓库可以长存不破。-
印象画派对乾隆皇帝-
要举出收藏仓库的成功例子,西方应该首推法国十九世纪的印象派画作。中国呢?今天看我选十八世纪的乾隆皇帝。乾隆不仅是神州历来最大的收藏家,也可能是人类历史的收藏一哥。此帝也,有点发神经,收藏兴趣广泛,工程之巨属天方夜谭。我个人认为乾隆自己指导炮制的物品有点俗气,但风格明确。(他的书画收藏有他的题跋、玺印风格。)不乱来,乾隆凡事苛求:瓷器华丽,玉雕精绝。魄力雄强,这个皇帝写过逾万首诗;手痒,到处题字,遗留下来的墨宝无数。好印章,据说为他刻的逾八千件。别的我没有研究,但有点研究的印章钮雕,我认为乾隆御用的来来去去是同一组人,不仅风格相同,刀法也差不多。这样的皇帝日理万机,六下江南,竟然活到八十然在第二章我细说了复息利率的杀伤力,持久地收藏不容易斗得过利息代价的蹂躏。然而,收藏品的市值上升不是平稳的,可以有大幅的波动,机缘巧合,市值的上升可以有一段长时期高于利息的代价。上世纪八十年代,因为日本的经济不济,法国印象派的画作下跌得急,但十年后回升。今天回顾,六十年前收藏印象派的画,选择得对,其升值高于以复息算的利息。-
贝叶斯学派的英文

贝叶斯学派的英文The Bayesian school of thought offers a probabilistic approach to statistical inference, emphasizing the updatingof beliefs based on new evidence. It's a way of learning from the world, where each piece of information refines our understanding.This method stands in contrast to the frequentist interpretation, which relies on fixed parameters and long-term frequencies. Bayesians, however, treat probabilities as degrees of belief, which can be subjective and personal.The beauty of Bayesian analysis lies in its ability to incorporate prior knowledge, making it incredibly flexibleand powerful in uncertain situations. It's not just about the data we have, but also about the wisdom we bring to the table.In fields ranging from medicine to machine learning, Bayesian techniques are revolutionizing the way we make decisions. By continuously updating our models with new data, we can navigate through the fog of uncertainty with greater confidence.Yet, the Bayesian approach also comes with challenges. It requires careful consideration of prior assumptions, whichcan significantly influence the conclusions drawn. It's a reminder that even in the realm of mathematics, oursubjective perspectives play a crucial role.As we delve deeper into the Bayesian paradigm, we find a philosophy that resonates with the human condition: our quest for knowledge is an ongoing journey, never a destination.It's a testament to the power of embracing change and learning from the world around us.。
抗帕金森病药

Origin of Parkinson disease
James Parkinson (Britain): 1817 年在Essay on the shaking Palsy 一书中第一次提出“震颤麻痹” 。 Parkinson去世40年后,神经病学家Charcot JM对震 颤麻痹补充了肌强直这一重要体征,完善了震颤麻痹 的诊断. 为纪念James Parkinson遂以其名字命名为
依据:⑴阻断DA-R的抗精神病药、耗竭DA 抑制纹状体摄取储存DA的利血平 ⑵MPTP→选择性破坏黑质-纹状体 DA能神经元 ⑶帕金森病患者中脑纹状体DA↓
⑷病理学改变:大体/组织学
帕金森病/帕 金森氏征
Sketch map of PD
On physiology state
Ach(+)
DA(-)
中枢胆碱能受体阻断药,减弱纹状体中Ach作用
Clinical uses
早期轻症PD患者
不能耐受L-dopa或L-dopa禁忌症患者
抗精神病药物引起的帕金森氏综合征
与复方多巴合用—协同
L-dopa 口服
90%被外周代谢
药物相互作用
脑内
10%进入血液、 外周组织
L-dopa
卡比多巴
多巴脱羧酶 多潘立酮
Relationship with other drugs
1. 禁止同时服用 Vit-B6
因Vit-B6 是L-dopa脱羧酶辅酶,加速L-dopa在外周 脱羧,进入脑循环L-dopa ↓, ↓疗效、↑不良反应
2.不宜合用吩噻嗪类,利血平
因吩噻嗪类、利血平等通过阻断中枢DA受体和耗竭 DA储存而引起药源性帕金森氏综合征,它们可拮抗 L-dopa的作用
第22章模糊理论

❖本章的學習主題 ❖ 1.認識模糊理論 2.模糊合成 3.模糊綜合評判 4.模糊運算 5.模糊推論 6.模糊控制 7.模擬理論之應用範例
企業研究方法 第 22 章 1
22.1 前言
一般可將資訊分為「可量化的資訊」與「不可量 化的資訊」,其中不可量化的資訊又稱為質化的 資訊,如:「這家公司總經理能力很強」、「這項 產品的品牌形象很好」等口語化的描述。 模糊理論(Fuzzy Theory)乃是積極承認主觀性問 題的存在,進而以模糊集合理論來處理不易量化 的問題,以便能適當而可靠的處理人們主觀評估 問題的方法。 模糊理論是為解決真實世界中普遍存在的模糊現 象而發展的一門學問,1965年美國自動控制學家 Lotfi. A. Zadeh首先提出的一種定量表達工具。
企業研究方法 第 22 章 3
22.2 模糊理論發展歷史
3.人類知識可說是用語言來表達的,而語言中存 在的模糊性,特別是因人而異所產生的主觀 性,也各不相同,這些模糊現象無法使用傳統 的數學工具例如機率等解決,故必須尋找另外 的替代途徑。
企業研究方法 第 22 章 4
22.3 模糊理論的基本概念
表 22-1 傳統集合與 Fuzzy 集合基本精神的比較
傳統集合 使用特徵函數 強調非此即彼的關係 只接受精確不模糊的資訊 硬性二分類法
Fuzzy 集合 (fuzzy set) 使用隸屬函數 接受亦此亦彼的關係 可接受精確不模糊的資訊 軟性的分類法
企業研究方法 第 22 章 5
22.3 模糊理論的基本概念
企業研究方法 第 22 章 6
在模糊集合的定義中,對某一元素X而言,是以μ(x) 來表示X屬於某集合的程度,即將X對應到[0,1]的 函數中,等級愈接近1,則表示該集合包合X元素的 程度愈大,此值稱為(degree of membership),所 以μ(x)稱為隸屬函數(membership function)。 隸屬函數的值只有0與1兩種時,該集合就是傳統的 明確集合(crisp set)。以圖22 - 1為例,來說明模糊 集合與明確集合間的不同。μ(x)表示「中年」的模 糊集合,而C (X)則表示傳統的明確集合。
概率逻辑、不确定逻辑和模糊逻辑之比较
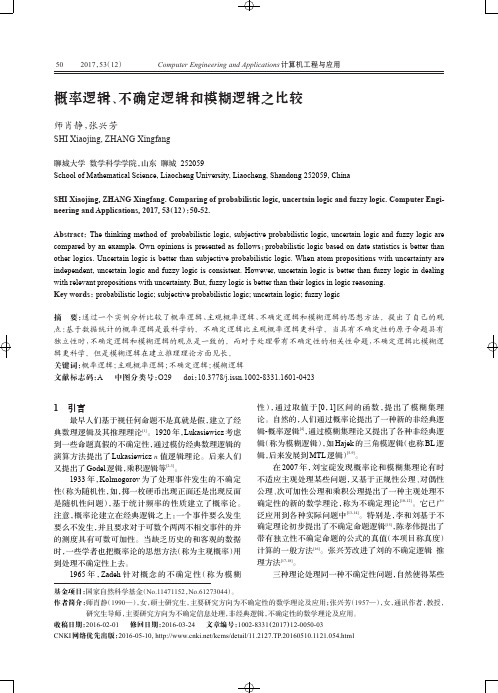
Computer Engineering and Applications 计算机工程与应用2017,53(12)1引言最早人们基于视任何命题不是真就是假,建立了经典数理逻辑及其推理理论[1]。
1920年,Lukasiewicz 考虑到一些命题真假的不确定性,通过模仿经典数理逻辑的演算方法提出了Lukasiewicz n 值逻辑理论。
后来人们又提出了Godel 逻辑,乘积逻辑等[2-3]。
1933年,Kolmogorov 为了处理事件发生的不确定性(称为随机性,如,掷一枚硬币出现正面还是出现反面是随机性问题),基于统计频率的性质建立了概率论。
注意,概率论建立在经典逻辑之上:一个事件要么发生要么不发生,并且要求对于可数个两两不相交事件的并的测度具有可数可加性。
当缺乏历史的和客观的数据时,一些学者也把概率论的思想方法(称为主观概率)用到处理不确定性上去。
1965年,Zadeh 针对概念的不确定性(称为模糊性),通过取值于[0,1]区间的函数,提出了模糊集理论。
自然的,人们通过概率论提出了一种新的非经典逻辑-概率逻辑[4],通过模糊集理论又提出了各种非经典逻辑(称为模糊逻辑),如Hajek 的三角模逻辑(也称BL 逻辑,后来发展到MTL 逻辑)[5-9]。
在2007年,刘宝碇发现概率论和模糊集理论有时不适应主观处理某些问题,又基于正规性公理、对偶性公理、次可加性公理和乘积公理提出了一种主观处理不确定性的新的数学理论,称为不确定理论[10-12]。
它已广泛应用到各种实际问题中[13-14]。
特别是,李和刘基于不确定理论初步提出了不确定命题逻辑[15],陈孝伟提出了带有独立性不确定命题的公式的真值(本项目称真度)计算的一般方法[16]。
张兴芳改进了刘的不确定逻辑推理方法[17-18]。
三种理论处理同一种不确定性问题,自然使得某些概率逻辑、不确定逻辑和模糊逻辑之比较师肖静,张兴芳SHI Xiaojing,ZHANG Xingfang聊城大学数学科学学院,山东聊城252059School of Mathematical Science,Liaocheng University,Liaocheng,Shandong 252059,ChinaSHI Xiaojing,ZHANG paring of probabilistic logic,uncertain logic and fuzzy puter Engi-neering and Applications,2017,53(12):50-52.Abstract :The thinking method of probabilistic logic,subjective probabilistic logic,uncertain logic and fuzzy logic are compared by an example.Own opinions is presented as follows :probabilistic logic based on date statistics is better than other logics.Uncertain logic is better than subjective probabilistic logic.When atom propositions with uncertainty are independent,uncertain logic and fuzzy logic is consistent.However,uncertain logic is better than fuzzy logic in dealing with relevant propositions with uncertainty.But,fuzzy logic is better than their logics in logic reasoning.Key words :probabilistic logic;subjective probabilistic logic;uncertain logic;fuzzy logic摘要:通过一个实例分析比较了概率逻辑、主观概率逻辑、不确定逻辑和模糊逻辑的思想方法。
- 1、下载文档前请自行甄别文档内容的完整性,平台不提供额外的编辑、内容补充、找答案等附加服务。
- 2、"仅部分预览"的文档,不可在线预览部分如存在完整性等问题,可反馈申请退款(可完整预览的文档不适用该条件!)。
- 3、如文档侵犯您的权益,请联系客服反馈,我们会尽快为您处理(人工客服工作时间:9:00-18:30)。
F. Masulli is with the Department of Computer Science, University of Pisa, Italy, and INFM, the National Institute for the Physics of Matter. S. Rovetta is with the Department of Computer and Information Sciences, University of Genoa, Italy, and INFM, the National Institute for the Physics of Matter. Corresponding author: Francesco Masulli INFM, Via Dodecaneso 35 – 16146 Genova, Italy. Fax: +39-10-353 6699. E-mail: masulli@disi.unige.it
ห้องสมุดไป่ตู้
R
[1] [2] [3] [4] [5] [6] [7] [8] [9] [10] [11] [12] [13] [14] [15] Enrique H. Ruspini, “A new approach to clustering”, Information and Control, vol. 15, no. 1, pp. 22–32, 1969. J. C. Dunn, “A fuzzy relative of the ISODATA process and its use in detecting compact well-separated clusters”, Journal of Cybernetics, vol. 3, pp. 32–57, 1974. James C. Bezdek, Pattern recognition with fuzzy objective function algorithms, Plenum, New York, 1981. G.H. Ball and D.J. Hall, “ISODATA, an iterative method of multivariate analysis and pattern classification”, Behavioral Science, vol. 12, pp. 153–155, 1967. Kenneth Rose, Eitan Gurewitz, and Geoffrey Fox, “A deterministic annealing approach to clustering”, Pattern Recognition Letters, vol. 11, pp. 589–594, 1990. Kenneth Rose, Eitan Gurewitz, and Geoffrey Fox, “Statistical mechanics and phase transitions in clustering”, Physical Review Letters, vol. 65, pp. 945–948, 1990. C.K. Chow, “An optimum character recognition system using decision function”, IRE Transactions on Electronic Computers, vol. 6, pp. 247–254, 1957. C.K. Chow, “An optimum recognition error and reject tradeoff ”, IEEE Transactions on Information Theory, vol. 16, pp. 41–46, 1970. Richard O. Duda and Peter E. Hart, Pattern Classification and Scene Analysis, John Wiley and Sons, 1973. Hisao Ishibuchi and Manabu Nii, “Neural networks for soft decision making”, Fuzzy Sets and Systems, vol. 115, no. 1, pp. 121–140, October 2000. Gian Paolo Drago and Sandro Ridella, “Possibility and necessity pattern classification using an interval arithmetic perceptron”, Neural Computing and Applications, vol. 8, no. 1, pp. 40–52, 1999. Sandro Ridella, Stefano Rovetta, and Rodolfo Zunino, “K-winner machines for pattern classification”, IEEE Transactions on Neural Networks, vol. 12, no. 2, pp. 371–385, March 2001. Raghu Krishnapuram and James M. Keller, “A possibilistic approach to clustering”, IEEE Transactions on Fuzzy Systems, vol. 1, no. 2, pp. 98–110, May 1993. Raghu Krishnapuram and James M. Keller, “The possibilistic C -Means algorithm: insights and recommendations”, IEEE Transactions on Fuzzy Systems, vol. 4, no. 3, pp. 385–393, August 1996. Antonio Flores-Sintas, Jos´ e M. Cadenas, and Fernando Martin, “Local geometrical properties application to fuzzy clustering”, Fuzzy Sets and Systems, vol. 100, pp. 245–256, 1998.
Probabilistic versus Possibilistic Fuzzy Clustering: An Unifying Model
Francesco Masulli and Stefano Rovetta
E A H e clustering problem is usually stated as the task of partitioning a set of data vectors or patterns X = { xk }, k ∈ {1, . . . , n}, xk ∈ n by attributing each data point xk to a subset ω j ⊂ X , j ∈ {1, . . . , c}, defined by its centroid y j ∈ n . This attribution is made based on a given distance d (·, ·). A popular clustering method is the Fuzzy c-Means/Fuzzy ISODATA [1][2][3] (FCM) algorithm, which is a “fuzzy relative” to the simple c-Means technique [4]. FCM defines the ω j as fuzzy partitions of the data set X . Variations over this basic scheme try to overcome some of its well-known limitations. The Deterministic Annealing (or Maximum Entropy) approach [5][6] does not minimize a simple cost term, but a compound ˆ and an entropic term −H . The optimization is done cost function which is the sum of a distortion term E by fixing a constant value for one of the two terms and minimizing the other; then this step is iterated for decreasing values of the constant, until a global optimum is reached. This alleviates the false minima problem of standard c-Means and (to a lesser extent) of FCM. In decision-making and classification applications, algorithms should feature several desirable properties in addition to the basic discrimination or decision function. For instance, it is normally required that in certain configurations a decision is not made (pattern rejection). This situation typically occurs in the presence of outliers. This problem is very well-known and well studied (see for instance [7][8][9]), and is tackled in a convenient way within the framework of soft-computing, fuzzy, and neural approaches [10][11][12]. However, the clustering problem as stated above implies that the outlier rejection property cannot be achieved. This is because the membership values are constrained to sum to 1. By giving up the requirement for strict partitioning, and by resorting to a “mode seeking” algorithm, Krishnapuram and Keller proposed the so-called possibilistic approach [13][14], where this constraint is relaxed essentially to u jk ∈ [0, 1] ∀k, ∀ j. With this model outlier rejection can be achieved, but at the expense of a clear cluster attribution and other computational drawbacks. The same issue of analysing the membership interactions on a local basis, as opposed to the global effects induced by the probabilistic model, is considered in [15]. In this paper, we introduce a formalism to provide an alternative, unified perspective on these clustering algorithms derived from the basic c-Means, focused on the memberships rather than on the cost function. Then, we introduce the concept of graded possibility . Its formulation includes as the two extreme cases the “probabilistic” assumption and the “possibilistic” assumption. A possible formulation can be stated as an interval equality constraint enforcing both the normality condition and the required graded possibilistic condition. We outline a basic example of the graded possibilistic clustering algorithm. The experimental demonstrations presented in the paper highlight the properties attainable through appropriate implementation of a suitable graded possibilistic model.