Computational Complexity in Non-Turing Models of Computation
人工智能领域中英文专有名词汇总
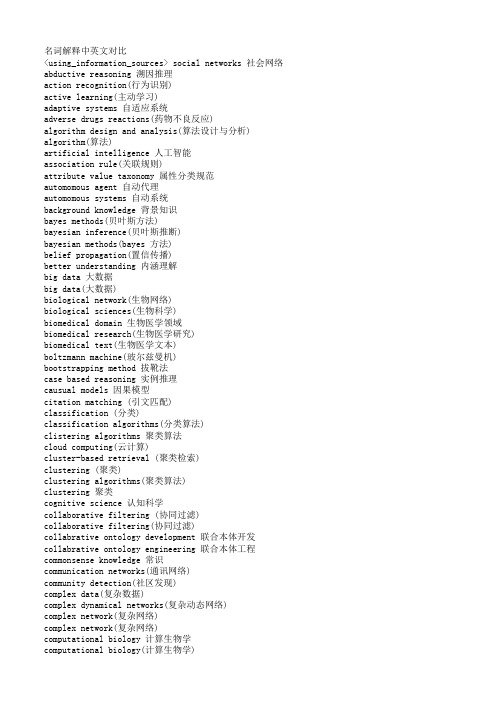
名词解释中英文对比<using_information_sources> social networks 社会网络abductive reasoning 溯因推理action recognition(行为识别)active learning(主动学习)adaptive systems 自适应系统adverse drugs reactions(药物不良反应)algorithm design and analysis(算法设计与分析) algorithm(算法)artificial intelligence 人工智能association rule(关联规则)attribute value taxonomy 属性分类规范automomous agent 自动代理automomous systems 自动系统background knowledge 背景知识bayes methods(贝叶斯方法)bayesian inference(贝叶斯推断)bayesian methods(bayes 方法)belief propagation(置信传播)better understanding 内涵理解big data 大数据big data(大数据)biological network(生物网络)biological sciences(生物科学)biomedical domain 生物医学领域biomedical research(生物医学研究)biomedical text(生物医学文本)boltzmann machine(玻尔兹曼机)bootstrapping method 拔靴法case based reasoning 实例推理causual models 因果模型citation matching (引文匹配)classification (分类)classification algorithms(分类算法)clistering algorithms 聚类算法cloud computing(云计算)cluster-based retrieval (聚类检索)clustering (聚类)clustering algorithms(聚类算法)clustering 聚类cognitive science 认知科学collaborative filtering (协同过滤)collaborative filtering(协同过滤)collabrative ontology development 联合本体开发collabrative ontology engineering 联合本体工程commonsense knowledge 常识communication networks(通讯网络)community detection(社区发现)complex data(复杂数据)complex dynamical networks(复杂动态网络)complex network(复杂网络)complex network(复杂网络)computational biology 计算生物学computational biology(计算生物学)computational complexity(计算复杂性) computational intelligence 智能计算computational modeling(计算模型)computer animation(计算机动画)computer networks(计算机网络)computer science 计算机科学concept clustering 概念聚类concept formation 概念形成concept learning 概念学习concept map 概念图concept model 概念模型concept modelling 概念模型conceptual model 概念模型conditional random field(条件随机场模型) conjunctive quries 合取查询constrained least squares (约束最小二乘) convex programming(凸规划)convolutional neural networks(卷积神经网络) customer relationship management(客户关系管理) data analysis(数据分析)data analysis(数据分析)data center(数据中心)data clustering (数据聚类)data compression(数据压缩)data envelopment analysis (数据包络分析)data fusion 数据融合data generation(数据生成)data handling(数据处理)data hierarchy (数据层次)data integration(数据整合)data integrity 数据完整性data intensive computing(数据密集型计算)data management 数据管理data management(数据管理)data management(数据管理)data miningdata mining 数据挖掘data model 数据模型data models(数据模型)data partitioning 数据划分data point(数据点)data privacy(数据隐私)data security(数据安全)data stream(数据流)data streams(数据流)data structure( 数据结构)data structure(数据结构)data visualisation(数据可视化)data visualization 数据可视化data visualization(数据可视化)data warehouse(数据仓库)data warehouses(数据仓库)data warehousing(数据仓库)database management systems(数据库管理系统)database management(数据库管理)date interlinking 日期互联date linking 日期链接Decision analysis(决策分析)decision maker 决策者decision making (决策)decision models 决策模型decision models 决策模型decision rule 决策规则decision support system 决策支持系统decision support systems (决策支持系统) decision tree(决策树)decission tree 决策树deep belief network(深度信念网络)deep learning(深度学习)defult reasoning 默认推理density estimation(密度估计)design methodology 设计方法论dimension reduction(降维) dimensionality reduction(降维)directed graph(有向图)disaster management 灾害管理disastrous event(灾难性事件)discovery(知识发现)dissimilarity (相异性)distributed databases 分布式数据库distributed databases(分布式数据库) distributed query 分布式查询document clustering (文档聚类)domain experts 领域专家domain knowledge 领域知识domain specific language 领域专用语言dynamic databases(动态数据库)dynamic logic 动态逻辑dynamic network(动态网络)dynamic system(动态系统)earth mover's distance(EMD 距离) education 教育efficient algorithm(有效算法)electric commerce 电子商务electronic health records(电子健康档案) entity disambiguation 实体消歧entity recognition 实体识别entity recognition(实体识别)entity resolution 实体解析event detection 事件检测event detection(事件检测)event extraction 事件抽取event identificaton 事件识别exhaustive indexing 完整索引expert system 专家系统expert systems(专家系统)explanation based learning 解释学习factor graph(因子图)feature extraction 特征提取feature extraction(特征提取)feature extraction(特征提取)feature selection (特征选择)feature selection 特征选择feature selection(特征选择)feature space 特征空间first order logic 一阶逻辑formal logic 形式逻辑formal meaning prepresentation 形式意义表示formal semantics 形式语义formal specification 形式描述frame based system 框为本的系统frequent itemsets(频繁项目集)frequent pattern(频繁模式)fuzzy clustering (模糊聚类)fuzzy clustering (模糊聚类)fuzzy clustering (模糊聚类)fuzzy data mining(模糊数据挖掘)fuzzy logic 模糊逻辑fuzzy set theory(模糊集合论)fuzzy set(模糊集)fuzzy sets 模糊集合fuzzy systems 模糊系统gaussian processes(高斯过程)gene expression data 基因表达数据gene expression(基因表达)generative model(生成模型)generative model(生成模型)genetic algorithm 遗传算法genome wide association study(全基因组关联分析) graph classification(图分类)graph classification(图分类)graph clustering(图聚类)graph data(图数据)graph data(图形数据)graph database 图数据库graph database(图数据库)graph mining(图挖掘)graph mining(图挖掘)graph partitioning 图划分graph query 图查询graph structure(图结构)graph theory(图论)graph theory(图论)graph theory(图论)graph theroy 图论graph visualization(图形可视化)graphical user interface 图形用户界面graphical user interfaces(图形用户界面)health care 卫生保健health care(卫生保健)heterogeneous data source 异构数据源heterogeneous data(异构数据)heterogeneous database 异构数据库heterogeneous information network(异构信息网络) heterogeneous network(异构网络)heterogenous ontology 异构本体heuristic rule 启发式规则hidden markov model(隐马尔可夫模型)hidden markov model(隐马尔可夫模型)hidden markov models(隐马尔可夫模型) hierarchical clustering (层次聚类) homogeneous network(同构网络)human centered computing 人机交互技术human computer interaction 人机交互human interaction 人机交互human robot interaction 人机交互image classification(图像分类)image clustering (图像聚类)image mining( 图像挖掘)image reconstruction(图像重建)image retrieval (图像检索)image segmentation(图像分割)inconsistent ontology 本体不一致incremental learning(增量学习)inductive learning (归纳学习)inference mechanisms 推理机制inference mechanisms(推理机制)inference rule 推理规则information cascades(信息追随)information diffusion(信息扩散)information extraction 信息提取information filtering(信息过滤)information filtering(信息过滤)information integration(信息集成)information network analysis(信息网络分析) information network mining(信息网络挖掘) information network(信息网络)information processing 信息处理information processing 信息处理information resource management (信息资源管理) information retrieval models(信息检索模型) information retrieval 信息检索information retrieval(信息检索)information retrieval(信息检索)information science 情报科学information sources 信息源information system( 信息系统)information system(信息系统)information technology(信息技术)information visualization(信息可视化)instance matching 实例匹配intelligent assistant 智能辅助intelligent systems 智能系统interaction network(交互网络)interactive visualization(交互式可视化)kernel function(核函数)kernel operator (核算子)keyword search(关键字检索)knowledege reuse 知识再利用knowledgeknowledgeknowledge acquisitionknowledge base 知识库knowledge based system 知识系统knowledge building 知识建构knowledge capture 知识获取knowledge construction 知识建构knowledge discovery(知识发现)knowledge extraction 知识提取knowledge fusion 知识融合knowledge integrationknowledge management systems 知识管理系统knowledge management 知识管理knowledge management(知识管理)knowledge model 知识模型knowledge reasoningknowledge representationknowledge representation(知识表达) knowledge sharing 知识共享knowledge storageknowledge technology 知识技术knowledge verification 知识验证language model(语言模型)language modeling approach(语言模型方法) large graph(大图)large graph(大图)learning(无监督学习)life science 生命科学linear programming(线性规划)link analysis (链接分析)link prediction(链接预测)link prediction(链接预测)link prediction(链接预测)linked data(关联数据)location based service(基于位置的服务) loclation based services(基于位置的服务) logic programming 逻辑编程logical implication 逻辑蕴涵logistic regression(logistic 回归)machine learning 机器学习machine translation(机器翻译)management system(管理系统)management( 知识管理)manifold learning(流形学习)markov chains 马尔可夫链markov processes(马尔可夫过程)matching function 匹配函数matrix decomposition(矩阵分解)matrix decomposition(矩阵分解)maximum likelihood estimation(最大似然估计)medical research(医学研究)mixture of gaussians(混合高斯模型)mobile computing(移动计算)multi agnet systems 多智能体系统multiagent systems 多智能体系统multimedia 多媒体natural language processing 自然语言处理natural language processing(自然语言处理) nearest neighbor (近邻)network analysis( 网络分析)network analysis(网络分析)network analysis(网络分析)network formation(组网)network structure(网络结构)network theory(网络理论)network topology(网络拓扑)network visualization(网络可视化)neural network(神经网络)neural networks (神经网络)neural networks(神经网络)nonlinear dynamics(非线性动力学)nonmonotonic reasoning 非单调推理nonnegative matrix factorization (非负矩阵分解) nonnegative matrix factorization(非负矩阵分解) object detection(目标检测)object oriented 面向对象object recognition(目标识别)object recognition(目标识别)online community(网络社区)online social network(在线社交网络)online social networks(在线社交网络)ontology alignment 本体映射ontology development 本体开发ontology engineering 本体工程ontology evolution 本体演化ontology extraction 本体抽取ontology interoperablity 互用性本体ontology language 本体语言ontology mapping 本体映射ontology matching 本体匹配ontology versioning 本体版本ontology 本体论open government data 政府公开数据opinion analysis(舆情分析)opinion mining(意见挖掘)opinion mining(意见挖掘)outlier detection(孤立点检测)parallel processing(并行处理)patient care(病人医疗护理)pattern classification(模式分类)pattern matching(模式匹配)pattern mining(模式挖掘)pattern recognition 模式识别pattern recognition(模式识别)pattern recognition(模式识别)personal data(个人数据)prediction algorithms(预测算法)predictive model 预测模型predictive models(预测模型)privacy preservation(隐私保护)probabilistic logic(概率逻辑)probabilistic logic(概率逻辑)probabilistic model(概率模型)probabilistic model(概率模型)probability distribution(概率分布)probability distribution(概率分布)project management(项目管理)pruning technique(修剪技术)quality management 质量管理query expansion(查询扩展)query language 查询语言query language(查询语言)query processing(查询处理)query rewrite 查询重写question answering system 问答系统random forest(随机森林)random graph(随机图)random processes(随机过程)random walk(随机游走)range query(范围查询)RDF database 资源描述框架数据库RDF query 资源描述框架查询RDF repository 资源描述框架存储库RDF storge 资源描述框架存储real time(实时)recommender system(推荐系统)recommender system(推荐系统)recommender systems 推荐系统recommender systems(推荐系统)record linkage 记录链接recurrent neural network(递归神经网络) regression(回归)reinforcement learning 强化学习reinforcement learning(强化学习)relation extraction 关系抽取relational database 关系数据库relational learning 关系学习relevance feedback (相关反馈)resource description framework 资源描述框架restricted boltzmann machines(受限玻尔兹曼机) retrieval models(检索模型)rough set theroy 粗糙集理论rough set 粗糙集rule based system 基于规则系统rule based 基于规则rule induction (规则归纳)rule learning (规则学习)rule learning 规则学习schema mapping 模式映射schema matching 模式匹配scientific domain 科学域search problems(搜索问题)semantic (web) technology 语义技术semantic analysis 语义分析semantic annotation 语义标注semantic computing 语义计算semantic integration 语义集成semantic interpretation 语义解释semantic model 语义模型semantic network 语义网络semantic relatedness 语义相关性semantic relation learning 语义关系学习semantic search 语义检索semantic similarity 语义相似度semantic similarity(语义相似度)semantic web rule language 语义网规则语言semantic web 语义网semantic web(语义网)semantic workflow 语义工作流semi supervised learning(半监督学习)sensor data(传感器数据)sensor networks(传感器网络)sentiment analysis(情感分析)sentiment analysis(情感分析)sequential pattern(序列模式)service oriented architecture 面向服务的体系结构shortest path(最短路径)similar kernel function(相似核函数)similarity measure(相似性度量)similarity relationship (相似关系)similarity search(相似搜索)similarity(相似性)situation aware 情境感知social behavior(社交行为)social influence(社会影响)social interaction(社交互动)social interaction(社交互动)social learning(社会学习)social life networks(社交生活网络)social machine 社交机器social media(社交媒体)social media(社交媒体)social media(社交媒体)social network analysis 社会网络分析social network analysis(社交网络分析)social network(社交网络)social network(社交网络)social science(社会科学)social tagging system(社交标签系统)social tagging(社交标签)social web(社交网页)sparse coding(稀疏编码)sparse matrices(稀疏矩阵)sparse representation(稀疏表示)spatial database(空间数据库)spatial reasoning 空间推理statistical analysis(统计分析)statistical model 统计模型string matching(串匹配)structural risk minimization (结构风险最小化) structured data 结构化数据subgraph matching 子图匹配subspace clustering(子空间聚类)supervised learning( 有support vector machine 支持向量机support vector machines(支持向量机)system dynamics(系统动力学)tag recommendation(标签推荐)taxonmy induction 感应规范temporal logic 时态逻辑temporal reasoning 时序推理text analysis(文本分析)text anaylsis 文本分析text classification (文本分类)text data(文本数据)text mining technique(文本挖掘技术)text mining 文本挖掘text mining(文本挖掘)text summarization(文本摘要)thesaurus alignment 同义对齐time frequency analysis(时频分析)time series analysis( 时time series data(时间序列数据)time series data(时间序列数据)time series(时间序列)topic model(主题模型)topic modeling(主题模型)transfer learning 迁移学习triple store 三元组存储uncertainty reasoning 不精确推理undirected graph(无向图)unified modeling language 统一建模语言unsupervisedupper bound(上界)user behavior(用户行为)user generated content(用户生成内容)utility mining(效用挖掘)visual analytics(可视化分析)visual content(视觉内容)visual representation(视觉表征)visualisation(可视化)visualization technique(可视化技术) visualization tool(可视化工具)web 2.0(网络2.0)web forum(web 论坛)web mining(网络挖掘)web of data 数据网web ontology lanuage 网络本体语言web pages(web 页面)web resource 网络资源web science 万维科学web search (网络检索)web usage mining(web 使用挖掘)wireless networks 无线网络world knowledge 世界知识world wide web 万维网world wide web(万维网)xml database 可扩展标志语言数据库附录 2 Data Mining 知识图谱(共包含二级节点15 个,三级节点93 个)间序列分析)监督学习)领域 二级分类 三级分类。
Alan Turing

谢谢!
欢迎各位批评指正
提问与回答呈现出下列状况: 问:你会下国际象棋吗? 答:是的。 问:你会下国际象棋吗? 答:是的。 问:请再次回答,你会下国际象棋吗? 答:是的。 如果提问与回答呈现出另一种状态: 问: 你会下国际象棋吗? 答:是的。 问:你会下国际象棋吗? 答:是的,我不是已经说过了吗? 问:请再次回答,你会下国际象棋吗? 答:你烦不烦,干嘛老提同样的问题。 第一种可明显地感到回答者是从 知识库里提取简单的答案,第二种则 具有分析综合的能力,回答者知道观 察者在反复提出同样的问题。因此前 者可能为机器。
—阿兰·麦席森·图灵
Alan Mathison Turing
Alan Turing生平简介 Turing生平简介
1912年6月23日,出生于英国伦敦。 1931年-1934年,在英国剑桥大学国王学院学习。 1932年-1935年,研究量子力学、概率论和逻辑学。 1935年,年仅23岁被选为剑桥大学国王学院院士。 1936年,研究可计算性理论,提出“图灵机”构想。 1936年-1938年,主要在美国普林斯顿大学做博士研究,研究内容涉及 逻辑学、代数和数论等领域。 1938年-1939年,返回剑桥从事研究工作,并应邀加入英国政府破译二战 德军密码的工作。 1940年-1942年,作为主要参与者和贡献者之一,在破译纳粹德国通讯 密码的工作上成就杰出,并成功破译了德军U-潜艇密码,为扭转二 战盟军的大西洋战场战局立下汗马功劳。
• • • • • • • 24岁提出了图灵机理论 31岁参与Colossus的研制(二战时英国 破解德国通讯密码的计算机) 33岁时构思了仿真系统 35岁提出了自动程序设计的概念 38岁设计了“图灵测试” 39岁提出了关于生物增长的非线性理论 有“计算机之父”、“人工智能之父”、 “破译之父”等美誉。有人甚至认为他 的贡献及对未来世界的影响几乎可与牛 顿、爱因斯坦等巨人比肩。
[课件]智能计算理论PPT
![[课件]智能计算理论PPT](https://img.taocdn.com/s3/m/d0b8c3a5bceb19e8b8f6ba97.png)
陕西师范大学 计算机科学学院 9
陕西师范大学 计算机科学学院 14
2018/12/9
从符号主义到连接主义:行为主义
行为主义(actionism),又称为进化主义(evolutionism)或 控制论学派(cyberneticsism),其原理为控制论及感知-动 作型控制系统。 行为主义认为人工智能源于控制论。控制论思想早在20 世纪40~50年代就成为时代思潮的重要部分,影响了早 期的人工智能工作者。维纳(Wiener)和麦克洛克 (McCulloch)等人提出的控制论和自组织系统以及钱学 森等人提出的工程控制论和生物控制论,影响了许多领 域。控制论把神经系统的工作原理与信息理论、控制理 论、逻辑以及计算机联系起来。
陕西师范大学 计算机科学学院 10
2018/12/9
从符号主义到连结主义:符号主义
符号主义者,在1956年首先采用“人工智能”这个术语。 后来又发展了启发式算法->专家系统->知识工程理论与 技术,并在20世纪80年代取得很大发展。符号主义曾长 期一枝独秀,为人工智能的发展作出重要贡献,尤其是 专家系统的成功开发与应用,为人工智能走向工程应用 和实现理论联系实际具有特别重要的意义。在人工智能 的其他学派出现之后,符号主义仍然是人工智能的主流 派别。这个学派的代表人物有纽厄尔(Newell)、西蒙 (Simon)和尼尔逊(Nilsson)等。
Chapter 5 Time Complexity
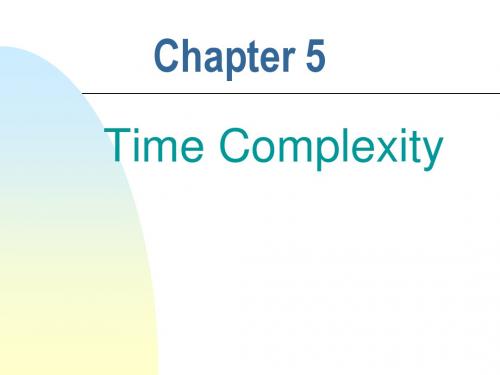
14
Strong Church Turing Thesis: P Class
All reasonable computational models are polynomialtime/space equivalent: It is always possible to simulate one model with a machine from another model with only polynomial time/space overhead. The answer to the question “AP?” does not depend on the model that we favor.
Clearly, n2 O(n3), since n2 n3, for n N, n0 = 0, and C = 1.
n3 C n2, n n0.
Let n1 = max(n0 + 1, C + 1). Then n13 = n1 n12 > C n12 , since n1 > C, contradicting the inequality that n13 C n12. Thus n3 O(n2).
2
Time Constructible Function
A function T(n) is said to be timeconstructible if there exists a T(n) timebounded, deterministic Turing machine that for each n has an input of length n on which it makes exactly T(n) moves. The function is said to be fully timeconstructible if there exists a deterministic Turing machine that makes exactly T(n) moves on each input of length n.
ON THE COMPUTATIONAL COMPLEXITY OF ALGORITHMS
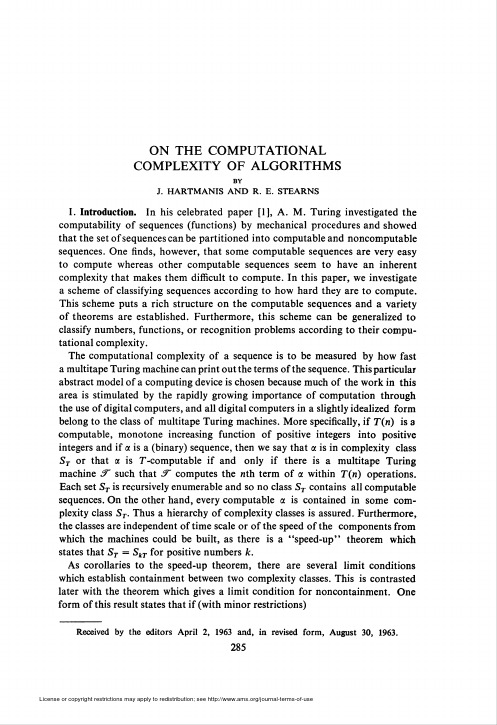
ON THE COMPUTATIONALCOMPLEXITY OF ALGORITHMSBYJ. HARTMANIS AND R. E. STEARNSI. Introduction. In his celebrated paper [1], A. M. Turing investigated the computability of sequences (functions) by mechanical procedures and showed that the setofsequencescanbe partitioned into computable and noncomputable sequences. One finds, however, that some computable sequences are very easy to compute whereas other computable sequences seem to have an inherent complexity that makes them difficult to compute. In this paper, we investigate a scheme of classifying sequences according to how hard they are to compute. This scheme puts a rich structure on the computable sequences and a variety of theorems are established. Furthermore, this scheme can be generalized to classify numbers, functions, or recognition problems according to their compu-tational complexity.The computational complexity of a sequence is to be measured by how fast a multitape Turing machine can print out the terms of the sequence. This particular abstract model of a computing device is chosen because much of the work in this area is stimulated by the rapidly growing importance of computation through the use of digital computers, and all digital computers in a slightly idealized form belong to the class of multitape Turing machines. More specifically, if Tin) is a computable, monotone increasing function of positive integers into positive integers and if a is a (binary) sequence, then we say that a is in complexity class ST or that a is T-computable if and only if there is a multitape Turing machine 3~ such that 3~ computes the nth term of a. within Tin) operations. Each set ST is recursively enumerable and so no class ST contains all computable sequences. On the other hand, every computable a is contained in some com-plexity class ST. Thus a hierarchy of complexity classes is assured. Furthermore, the classes are independent of time scale or of the speed of the components from which the machines could be built, as there is a "speed-up" theorem which states that ST = SkT f or positive numbers k.As corollaries to the speed-up theorem, there are several limit conditions which establish containment between two complexity classes. This is contrasted later with the theorem which gives a limit condition for noncontainment. One form of this result states that if (with minor restrictions)Received by the editors April 2, 1963 and, in revised form, August 30, 1963.285286J. HARTMANIS AND R. E. STEARNS[May»*«, U(n)then S,; properly contains ST. The intersection of two classes is again a class. The general containment problem, however, is recursively unsolvable.One section is devoted to an investigation as to how a change in the abstract machine model might affect the complexity classes. Some of these are related by a "square law," including the one-tape-multitape relationship: that is if a is T-computable by a multitape Turing machine, then it is T2-computable by a single tape Turing machine. It is gratifying, however, that some of the more obvious variations do not change the classes.The complexity of rational, algebraic, and transcendental numbers is studied in another section. There seems to be a good agreement with our intuitive notions, but there are several questions still to be settled.There is a section in which generalizations to recognition problems and functions are discussed. This section also provides the first explicit "impossibility" proof, by describing a language whose "words" cannot be recognized in real-time [T(n) = n] .The final section is devoted to open questions and problem areas. It is our conviction that numbers and functions have an intrinsic computational nature according to which they can be classified, as shown in this paper, and that there is a good opportunity here for further research.For background information about Turing machines, computability and related topics, the reader should consult [2]. "Real-time" computations (i.e., T(n) = n) were first defined and studied in [3]. Other ways of classifying the complexity of a computation have been studied in [4] and [5], where the complexity is defined in terms of the amount of tape used.II. Time limited computations. In this section, we define our version of a multitape Turing machine, define our complexity classes with respect to this type of machine, and then work out some fundamental properties of these classes.First, we give an English description of our machine (Figure 1) since one must have a firm picture of the device in order to follow our paper. We imagine a computing device that has a finite automaton as a control unit. Attached to this control unit is a fixed number of tapes which are linear, unbounded at both ends, and ruled into an infinite sequence of squares. The control unit has one reading head assigned to each tape, and each head rests on a single square of the assigned tape. There are a finite number of distinct symbols which can appear on the tape squares. Each combination of symbols under the reading heads together with the state of the control unit determines a unique machine operation. A machine operation consists of overprinting a symbol on each tape square under the heads, shifting the tapes independently either one square left, one square1965]ON THE COMPUTATIONAL COMPLEXITY OF ALGORITHMS287ti 1111 i n cm U I I i I I I ID mm.Tn T| in i i i i i i i m-m Î2II I I I I I I I I m II I I I I I I IIP TnTAPESFINITE STATECOMPUTEROUTPUT TAPEFigure 1. An «-tape Turing machineright, or no squares, and then changing the state of the control unit. The machine is then ready to perform its next operation as determined by the tapes and control state. The machine operation is our basic unit of time. One tape is signaled out and called the output tape. The motion of this tape is restricted to one way move-ment, it moves either one or no squares right. What is printed on the output tape and moved from under the head is therefore irrevocable, and is divorced from further calculations.As Turing defined his machine, it had one tape and if someone put k successive ones on the tape and started the machine, it would print some f(k) ones on the tape and stop. Our machine is expected to print successively /(l),/(2), ••• on its output tape. Turing showed that such innovations as adding tapes or tape symbols does not increase the set of functions that can be computed by machines. Since the techniques for establishing such equivalences are common knowledge, we take it as obvious that the functions computable by Turing's model are the same as those computable by our version of a Turing machine. The reason we have chosen this particular model is that it closely resembles the operation of a present day computer; and being interested in how fast a machine can compute, the extra tapes make a difference.To clear up any misconceptions about our model, we now give a formal definition.Definition 1. An n-tape Turing machine, &~, is a set of (3n + 4)-tuples, {(q¡; Stl, Sh, — , Sin ; Sjo, Sjl, — , Sh ; m0, mx, —, m… ; qf)},where each component can take on a finite set of values, and such that for every possible combination of the first n + 1 entries, there exists a unique (3zi-t-4)-tupIe in this set. The first entry, q¡, designates the present state; the next n entries, S(l,-",S,B, designate the present symbols scanned on tapes Tx, •■•, T…,respectively; the next n + 1 symbols SJa, ••-, Sjn, designate the new symbols to be printed on288J. HARTMANIS AND R. E. STEARNS[May tapes T0, •■», T…, respectively; the next n entries describe the tape motions (left, right, no move) of the n + 1 tapes with the restriction m0 # left ; and the last entry gives the new internal state. Tape T0 is called the output tape. One tuple with S¡. = blank symbol for 1 = j = n is designated as starting symbol.Note that we are not counting the output tape when we figure n. Thus a zero-tape machine is a finite automaton whose outputs are written on a tape. We assume without loss of generality that our machine starts with blank tapes.For brevity and clarity, our proofs will usually appeal to the English description and will technically be only sketches of proofs. Indeed, we will not even give a formal definition of a machine operation. A formal definition of this concept can be found in [2].For the sake of simplicity, we shall talk about binary sequences, the general-ization being obvious. We use the notation a = axa2 ••• .Definition 2. Let Tin) be a computable function from integers into integers such that Tin) ^ Tin + 1) and, for some integer k, Tin) ^ n/ k for all n. Then we shall say that the sequence a is T-computable if and only if there exists a multitape Turing machine, 3~, which prints the first n digits of the sequence a on its output tape in no more than Tin) operations, n = 1,2, ••», allowing for the possibility of printing a bounded number of digits on one square. The class of all T-computable binary sequences shall be denoted by ST, and we shall refer to T(n) as a time-function. Sr will be called a complexity class.When several symbols are printed on one square, we regard them as components of a single symbol. Since these are bounded, we are dealing with a finite set of output symbols. As long as the output comes pouring out of the machine in a readily understood form, we do not regard it as unnatural that the output not be strictly binary. Furthermore, we shall see in Corollaries 2.5, 2.7, and 2.8 that if we insist that Tin) ^ n and that only (single) binary outputs be used, then the theory would be within an e of the theory we are adopting.The reason for the condition Tin) ^ n/fc is that we do not wish to regard the empty set as a complexity class. For if a is in ST and F is the machine which prints it, there is a bound k on the number of digits per square of output tape and T can print at most fcn0 d igits in n0 operations. By assumption, Tikn0) ^ n0 or (substituting n0 = n/ k) Tin) à n/ k . On the other hand, Tin) ^ n/ k implies that the sequence of all zeros is in ST because we can print k zeros in each operation and thus ST is not void.Next we shall derive some fundamental properties of our classes.Theorem 1. TAe set of all T-computable binary sequences, ST, is recursively enumerable.Proof. By methods similar to the enumeration of all Turing machines [2] one can first enumerate all multitape Turing machines which print binary sequences. This is just a matter of enumerating all the sets satisfying Definition 1 with the1965] ON THE COMPUTATIONAL C OMPLEXITY O F ALGORITHMS 289 added requirement that Sjo is always a finite sequence of binary digits (regarded as one symbol). Let such an enumeration be &~x, 3~2, ••• . Because T(n) is comput-able, it is possible to systematically modify each ^"¡ to a machine &"'t w ith the following properties : As long as y¡ prints its nth digit within T(n) operations (and this can be verified by first computing T(n) and then looking at the first T(n) operations of ^"¡), then the nth digit of &~'t will be the nth output of &~¡. If &~¡ s hould ever fail to print the nth digit after T(n) operations, then ^"¡'will print out a zero for each successive operation. Thus we can derive a new enumeration •^"'u &~2> "•• If' &\ operates within time T(n), then ^", and ^"¡'compute the same T-computable sequence <x¡. O therwise, &~{ c omputes an ultimately constant sequence a¡ and this can be printed, k bits at a time [where T(n) — n / fc] by a zero tape machine. In either case, a¡ is T-computable and we conclude that {«,} = ST.Corollary 1.1. There does not exist a time-function T such that ST is the set of all computable binary sequences.Proof. Since ST is recursively enumerable, we can design a machine !T which, in order to compute its ith output, computes the z'th bit of sequence a, and prints out its complement. Clearly 3~ produces a sequence a different from all <Xj in ST.Corollary 1.2. For any time-function T, there exists a time-function U such that ST is strictly contained in Sv. Therefore, there are infinitely long chainsSTl cr STl cz •••of distinct complexity classes.Proof. Let &" compute a sequence a not in ST (Corollary 1.1). Let V(n) equal the number of operations required by ^"to compute the nth digit of a. Clearly V is computable and a e Sr. Lett/(n) = max [Tin), V(n)] ,then Vin) is a time-function and clearlyOrí ^3 Oj1 *Since a in Sv and a not in ST, we haveCorollary 1.3. The set of all complexity classes is countable.Proof. The set of enumerable sets is countable.Our next theorem asserts that linear changes in a time-function do not change the complexity class. // r is a real number, we write [r] to represent the smallest integer m such that m = r.290J. HARTMANIS AND R. E. STEARNS[MayTheorem 2. If the sequence cc is T-computable and k is a computable, positive real number, then a is [kT~\-computable; that is,ST = S[kTX.Proof. We shall show that the theorem is true for k = 1/2 and it will be true for fc = 1/ 2m b y induction, and hence for all other computable k since, given k, k ^ 1 /2'" for some m. (Note that if k is computable, then \kT~\ is a computable function satisfying Definition 2.)Let ¡F be a machine which computes a in time T. If the control state, the tape symbols read, and the tape symbols adjacent to those read are all known, then the state and tape changes resulting from the next two operations of &~ are determined and can therefore be computed in a single operation. If we can devise a scheme so that this information is always available to a machine 5~', then &' can perform in one operation what ST does in two operations. We shall next show how, by combining pairs of tape symbols into single symbols and adding extra memory to the control, we can make the information available.In Figure 2(a), we show a typical tape of S" with its head on the square marked 0. In Figure 2(b), we show the two ways we store this information in &~'. Each square of the ^"'-tape contains the information in two squares of the ^-tape. Two of the ^"-tape symbols are stored internally in 3r' and 3~' must also remember which piece of information is being read by 9~. In our figures, this is indicated by an arrow pointed to the storage spot. In two operations of &~, t he heads must move to one of the five squares labeled 2, 1,0, — l,or —2. The corresponding next position of our ^"'-tape is indicated in Figures 2(c)-(g). It is easily verified that in each case, &"' can print or store the necessary changes. In the event that the present symbol read by IT is stored on the right in ¡T' as in Figure 2(f), then the analogous changes are made. Thus we know that ST' can do in one operation what 9~ does in two and the theorem is proved.Corollary 2.1. If U and T are time-functions such that«-.«> Vin)then Svçz ST.Proof. Because the limit is greater than zero, Win) ^ Tin) for some k > 0, and thus Sv = SlkVj çz sT.Corollary 2.2. If U and T are time-functions such thatTin)sup-TTT-r- < 00 ,n-»a> O(n)then SV^ST.Proof. This is the reciprocal of Corollary 2.1.1965] ON THE COMPUTATIONAL COMPLEXITY OF ALGORITHMSE37291/HO W2|3l4[5l(/ZEEI33OÏÏT2Ï31/L-2_-iJ(c]¿m W\2I3I4I5K/(b)ZBE o2|3|4l5|\r2Vi!¿En on2l3l4l5|/l-T-i](d)¿BE2 34[5|6|7ir\10 l|(f)¿m2 34|5l6l7l /L<Dj(g)Figure 2. (a) Tape of ^" with head on 0. (b) Corresponding configurations of 9"'. (c) 9~' if F moves two left, (d) 9~> i f amoves to -1. (e) 9~' if ^~ moves to 0. (f)^"' if amoves to 1.(g) 9~' if 3~ moves two rightCorollary 2.3. If U and T are time-functions such thatTin)0 < hm ) ; < oo ,H-.« Uin)then Srj = ST .Proof. This follows from Corollaries 2.1 and 2.2.Corollary 2.4. // Tin) is a time-function, then Sn^ST . Therefore, Tin) = n is the most severe time restriction.Proof. Because T is a time-function, Tin) = n/ k for some positive k by Definition 2; hence292j. hartmanis and r. e. stearns[Maymf m à 1 > O…-»o, n kand S… çz s T by Corollary 2.1.Corollary 2.5. For any time-function T, Sr=Sv where t/(n)=max \T(n),n\. Therefore, any complexity class may be defined by a function U(n) ^ n. Proof. Clearly inf (T/ Í7) > min (1,1/ k) and sup (T/ U) < 1 .Corollary 2.6. If T is a time-function satisfyingTin) > n and inf -^ > 1 ,…-co nthen for any a in ST, there is a multitape Turing machined with a binary (i.e., two symbol) output which prints the nth digit of a in Tin) or fewer operations. Proof. The inf condition implies that, for some rational e > 0, and integer N, (1 - e) Tin) > n or Tin) > eTin) + n for all n > N. By the theorem, there is a machine 9' which prints a in time \zT(ri)\. 9' can be modified to a machine 9" which behaves like 9' except that it suspends its calculation while it prints the output one digit per square. Obviously, 9" computes within time \i.T(ri)\ + n (which is less than Tin) for n > N). $~" can be modified to the desired machine9~ by adding enough memory to the control of 9~" to print out the nth digit of a on the nth operation for n ^ N.Corollary 2.7. IfT(n)^nandoieST,thenforanys >0, there exists a binary output multitape Turing machine 9 which prints out the nth digit of a in [(1 + e) T(n)J or fewer operations.Proof. Observe that. [(1 + e) T(n)]inf —--——■— — 1 + enand apply Corollary 2.6.Corollary 2.8. // T(n)^n is a time-function and oteST, then for any real numbers r and e, r > e > 0, /Aere is a binary output multitape Turing machine ¡F which, if run at one operation per r—e seconds, prints out the nth digit of a within rT(n) seconds. Ifcc$ ST, there are no such r and e. Thus, when considering time-functions greater or equal to n, the slightest increase in operation speed wipes out the distinction between binary and nonbinary output machines.Proof. This is a consequence of the theorem and Corollary 2.7.Theorem 3. // Tx and T2 are time-functions, then T(n) = min [T^n), T2(n)~] is a time-function and STí O ST2 = ST.1965] ON THE COMPUTATIONAL COMPLEXITY OF ALGORITHMS 293 Proof. T is obviously a time-function. If 9~x is a machine that computes a in time T, and 9~2 computes a in time T2, then it is an easy matter to construct a third device &~ i ncorporating both y, and 3T2 which computes a both ways simul-taneously and prints the nth digit of a as soon as it is computed by either J~x or 9~2. Clearly this machine operates inTin) = min \Txin), T2(n)] .Theorem 4. If sequences a and ß differ in at most a finite number of places, then for any time-function T, cceST if and only if ße ST.Proof. Let ,T print a in time T. Then by adding some finite memory to the control unit of 3", we can obviously build a machine 3~' which computes ß in time T.Theorem 5. Given a time-function T, there is no decision procedure to decide whether a sequence a is in ST.Proof. Let 9~ be any Turing machine in the classical sense and let 3Tx be a multitape Turing machine which prints a sequence ß not in ST. Such a 9~x exists by Theorem 1. Let 9~2 be a multitape Turing machine which prints a zero for each operation $~ makes before stopping. If $~ should stop after k operations, then 3~2 prints the /cth and all subsequent output digits of &x. Let a be the sequence printed by 9"2, Because of Theorem 4, a.eST if and only if 9~ does not stop. Therefore, a decision procedure for oceST would solve the stopping problem which is known to be unsolvable (see [2]).Corollary 5.1. There is no decision procedure to determine if SV=ST or Sv c STfor arbitrary time-functions U and T.Proof. Similar methods to those used in the previous proof link this with the stopping problem.It should be pointed out that these unsolvability aspects are not peculiar to our classification scheme but hold for any nontrivial classification satisfying Theorem 4.III. Other devices. The purpose of this section is to compare the speed of our multitape Turing machine with the speed of other variants of a Turing machine. Most important is the first result because it has an application in a later section.Theorem 6. If the sequence a is T-computable by multitape Turing machine, !T, then a is T2-computable by a one-tape Turing machine 3~x .Proof. Assume that an n-tape Turing machine, 3~, is given. We shall now describe a one-tape Turing machine Px that simulates 9~, and show that if &" is a T-computer, then S~x is at most a T2-computer.294j. hartmanis and r. e. stearns[May The S~ computation is simulated on S'y as follows : On the tape of & y will be stored in n consecutive squares the n symbols read by S on its n tapes. The symbols on the squares to the right of those symbols which are read by S~ on its n tapes are stored in the next section to the right on the S'y tape, etc., as indicated in Figure 3, where the corresponding position places are shown. The1 TAPE T|A 1 TAPE T2I?TAPE Tn(a)J-"lo(b)Figure 3. (a) The n tapes of S. (b) The tape of S~\machine Tx operates as follows: Internally is stored the behavioral description of the machine S", so that after scanning the n squares [J], [o], ■■■, [5]»-^"îdetermines to what new state S~ will go, what new symbols will be printed by it on its n tapes and in which direction each of these tapes will be shifted. First,¡Fy prints the new symbols in the corresponding entries of the 0 block. Then it shifts the tape to the right until the end of printed symbols is reached. (We can print a special symbol indicating the end of printed symbols.) Now the machine shifts the tape back, erases all those entries in each block of n squares which correspond to tapes of S~ which are shifted to the left, and prints them in the corresponding places in the next block. Thus all those entries whose corresponding S~ tapes are shifted left are moved one block to the left. At the other end of the tape, the process is reversed and returning on the tape 9y transfers all those entries whose corresponding S~ tapes are shifted to the right one block to the right on the S'y tape. When the machine S', reaches the rigAz most printed symbol on its tape, it returns to the specially marked (0) block which now contains1965] ON THE COMPUTATIONAL COMPLEXITY OF ALGORITHMS 295 the n symbols which are read by &~ o n its next operation, and #", has completed the simulation of one operation of 9~. It can be seen that the number of operations of Tx is proportional to s, the number of symbols printed on the tape of &"¡. This number increases at most by 2(n + 1) squares during each operation of &. Thus, after T(fc) operations of the machine J~, the one-tape machine S"t will perform at most7(*)T,(fc) =C0+ T Cxii = loperations, where C0 and C, are constants. But thenr,(fe) g C2 £ i^C [T(fc)]2 .¡ =iSince C is a constant, using Theorem 2, we conclude that there exists a one tape machine printing its fcth output symbol in less than T(fc)2 tape shifts as was to be shown.Corollary 6.1. The best computation time improvement that can be gained in going from n-tape machines to in + l)-tape machines is the square root of the computation time.Next we investigate what happens if we allow the possibility of having several heads on each tape with some appropriate rule to prevent two heads from occupy-ing the same square and giving conflicting instructions. We call such a device a multihead Turing machine. Our next result states that the use of such a model would not change the complexity classes.Theorem 7. Let a. be computable by a multihead Turing machine 3T which prints the nth digit in Tin) or less operations where T is a time-function; then a is in ST .Proof. We shall show it for a one-tape two-head machine, the other cases following by induction. Our object is to build a multitape machine Jr' which computes a within time 4T which will establish our result by Theorem 2. The one tape of !T will be replaced by three tapes in 9"'. Tape a contains the left-hand information from 9", tape b contains the right-hand information of 9~, and tape c keeps count, two at a time, of the number of tape squares of ST which are stored on both tapes a and b_. A check mark is always on some square of tape a to indicate the rightmost square not stored on tape b_ and tape b has a check to indicate the leftmost square not stored on tape a.When all the information between the heads is on both tapes a and b. then we have a "clean" position as shown in Figure 4(a). As &" operates, then tape296j. hartmanis and r. e. stearns [May7/Fio TTzTTR" 5 "6Ï7M I 4T5T6" 7 8TT77' ^f(a) rT-Tô:TT2l3l4l?l \J ¿Kh.1y(b) J I l?IM2!3|4 5.6T7 /I |?|4,|5|6 7 8TT7(c) f\7~ /\V\/\A7\7M J M/l/yTITTTTTTJ(a) (b)Figure 4. (a) .^"' in clean position, (b) S' in dirty positiona performs like the left head of S~, tape A behaves like the right head, and tape c reduces the count each time a check mark is moved. Head a must carry the check right whenever it moves right from a checked square, since the new symbol it prints will not be stored on tape A; and similarly head A moves its check left.After some m operations of S~' corresponding to m operations of S~, a "dirty"position such as Figure 4(b) is reached where there is no overlapping information.The information (if any) between the heads of S~ must be on only one tape of S~',say tape A as in Figure 4(b). Head A then moves to the check mark, the between head information is copied over onto tape a, and head amoves back into position.A clean position has been achieved and S~' is ready to resume imitating S~. The time lost is 3/ where I is the distance between the heads. But / ^ m since headA has moved / squares from the check mark it left. Therefore 4m is enough time to imitate m operations of S~ and restore a clean position. Thusas was to be shown.This theorem suggests that our model can tolerate some large deviations without changing the complexity classes. The same techniques can be applied to other changes in the model. For example, consider multitape Turing ma-chines which have a fixed number of special tape symbols such that each symbol can appear in at most one square at any given time and such that the reading head can be shifted in one operation to the place where the special symbol is printed, no matter how far it is on the tape. Turing machines with such "jump instructions^ are similarly shown to leave the classes unchanged.Changes in the structure of the tape tend to lead to "square laws." For example,consider the following :Definition 3. A two-dimensional tape is an unbounded plane which is sub-divided into squares by equidistant sets of vertical and horizontal lines as shown in Figure 5. The reading head of the Turing machine with this two-dimensional tape can move either one square up or down, or one square left or right on each operation. This definition extends naturally to higher-dimensional tapes.。
量子计算复杂性理论综述_张焕国
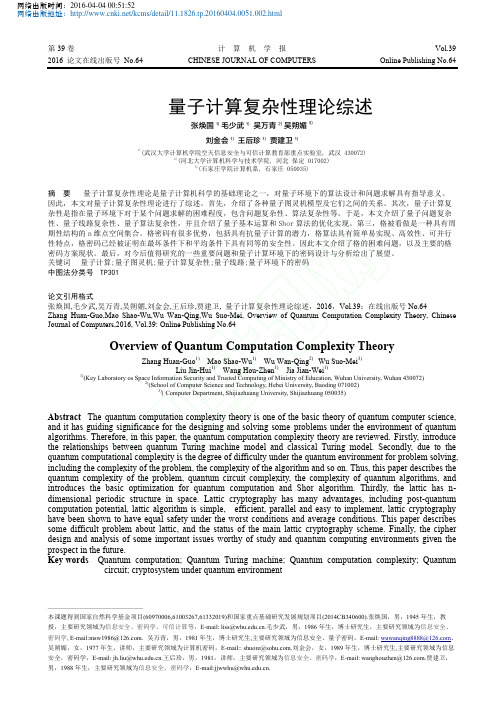
摘 要 量子计算复杂性理论是量子计算机科学的基础理论之一,对量子环境下的算法设计和问题求解具有指导意义。 因此,本文对量子计算复杂性理论进行了综述。首先,介绍了各种量子图灵机模型及它们之间的关系。其次,量子计算复 杂性是指在量子环境下对于某个问题求解的困难程度,包含问题复杂性、算法复杂性等。于是,本文介绍了量子问题复杂 性、量子线路复杂性、量子算法复杂性,并且介绍了量子基本运算和 Shor 算法的优化实现。第三,格被看做是一种具有周 期性结构的 n 维点空间集合。格密码有很多优势,包括具有抗量子计算的潜力,格算法具有简单易实现、高效性、可并行 性特点,格密码已经被证明在最坏条件下和平均条件下具有同等的安全性。因此本文介绍了格的困难问题,以及主要的格 密码方案现状。最后,对今后值得研究的一些重要问题和量子计算环境下的密码设计与分析给出了展望。 关键词 量子计算;量子图灵机;量子计算复杂性;量子线路;量子环境下的密码 中图法分类号 TP301 论文引用格式 张焕国,毛少武,吴万青,吴朔媚,刘金会,王后珍,贾建卫, 量子计算复杂性理论综述,2016,Vol.39:在线出版号 No.64 Zhang Huan-Guo,Mao Shao-Wu,Wu Wan-Qing,Wu Suo-Mei, Overview of Quantum Computation Complexity Theory, Chinese Journal of Computers,2016, Vol.39: Online Publishing No.64
大 D-Wave 公司推出世界上首台 128 量子位商用量 子计算机 D-Wave One 系统。著名军火商洛克希德 马丁公司以 1000 万美元/台购买了 D-Wave One 用 于 F35 战机分析,新武器开发和航天航空器系统 测试等。2013 年初,加拿大 D-Wave 公司又推出 512 量子位的 D-Wave Two。谷歌公司以 1500 万美 元/台购买了 D-Wave Two 用于加速信息搜索的速 度和人工智能。但是加拿大 D-Wave 公司推出的 DWave 系列量子计算机是专用型的量子计算机,不 是通用型的,只能处理某些特定的问题。2001 年 IBM 公司率先研制成功了 7qbit 的示例性通用量子 计算机。证明了量子计算机原理的正确性和可行 性。2011 年 9 月,Nature 撰文指出 UCSB 团队通 过量子电路成功实现了冯诺依曼结构的 9 个量子 位的量子计算机。2012 年 IBM 在美国物理年会上 公布声称找到了可以大规模提升量子计算机规模 的一种关键技术。2013 年美国 MIT 报告指出微软 公司早在十年前就与加州大学圣巴巴拉分校合作 开始研究量子计算机。2014 年 4 月,科学家获得 110 量子位的纠缠态远高于以前的 11 量子位。 2014 年 9 月 3 日谷歌公司宣布投资 50 亿美元与 UCSB 的研究团队联合研制量子计算机。 综上所述,虽然量子计算机离大规模使用还 有很长的距离。但是,一旦大规模的量子计算机 成为现实,现有的许多公钥密码将不再安全。量 子计算时代我们使用什么密码,是摆在我国面前 的一个十分紧迫的重大战略问题!这样就迫切需 要研究能抵御量子计算的新型密码。抗量子计算 [12] 的密码主要包括以下几类 :(1) 基于量子物理的 [14-19] [20-22] 量子密码 ;(2)基于生物学的 DNA 密码 ; (3) 基于量子计算不擅长计算的数学问题构建的 [23-26] 密码 。量子计算复杂性理论是量子密码体制安 全性的理论基础,也是构造现代量子密码体制的 理论依据。它给出求解一个问题是容易还是困难 的依据,并由此对问题和算法的复杂性进行分 类,进而根据困难问题设计量子计算环境下的安 全密码。 [27] 由于量子计算机具有并行性 ,所以它在许 [28] 多方面具有比电子计算机更强大的计算能力 。 这使得现在广泛应用的许多密码在量子计算环境 [4,11,39,30] 下将不再安全 。量子计算复杂性理论是量 [31] 子计算机科学的基础理论之一 ,对量子环境下 的算法设计和问题求解具有指导意义。因此,量 子计算复杂性理论成为量子环境下密码安全的理 论基础。本文对量子计算复杂性理论进行了综 述。介绍了各种量子图灵机模型及它们之间的关 系,并进行了量子线路模型与量子图灵机的比 较。详细讨论了量子计算复杂性,包括量子算法 复杂性,问题复杂性和量子线路复杂性。特别提 出了一种新的量子计算数据复杂性。最后,对今
基于非精确单调与非单调线搜索的全波形反演
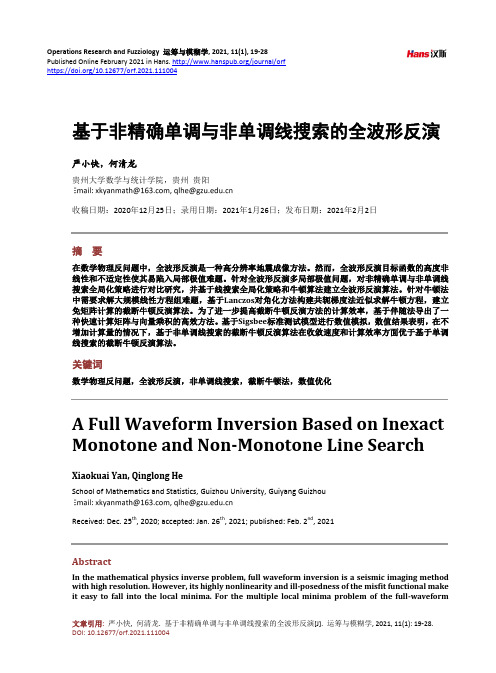
return mk
endif
经典 FWI 的目标函数定义为
∑ = f (m)
( ) 1 Ns
2 s=1
us
m
− ds
2.
2
(1)
其中, ⋅ 2 表示 L2 范数,m 为反演参数(例如波速、介质密度等),Ns 为震源个数,ds 表示实际观测数据,
us (m) 为合成波场,且满足波动方程
A(m)us = qs.
(2)
其中, A(m) 为波动微分算子。因此,FWI 问题为一个标准的偏微分方程(PDE)约束的优化问题。
Keywords
Mathematical Physics Inverse Problem, Full Waveform Inversion, Non-Monotone Line Search, Truncated Newton Method, Numerical Optimization
Copyright © 2021 by author(s) and Hans Publishers Inc. This work is licensed under the Creative Commons Attribution International License (CC BY 4.0). /licenses/by/4.0/
δ m = −Hk−1∇f (mk ).
于是牛顿迭代公式为
mk=+1 mk + αkδ m,
(4)
其中, αk 表示迭代步长, δ m 称为牛顿下降方向。 FWI 是大规模反演问题,采用矩阵分解方法直接求解牛顿方程(3)是不现实的。一方面,Hessian
矩阵 Hk 通常无法显式计算;另一方面,即便获得了 Hk ,采用直接法求解(3)也是不切实际的。因此, 通常用 CG 法来近似求解牛顿方程(3),该方法的最大优点是在求解过程中只需要计算 Hessian 矩阵与 向量的乘积。该类方法称为截断牛顿法(或不精确牛顿法)。算法 1 所示为一般截断牛顿型方法的算法 描述。
现代密码学中英文翻译(部分)

Table of ContentsModern Cryptography: Theory and PracticeBy Wenbo Mao Hewlett-Packard CompanyPublisher: Prentice Hall PTRPub Date: July 25, 2003ISBN: 0-13-066943-1Pages: 648Many cryptographic schemes and protocols, especially those based onpublic-keycryptography,have basic or so-called "textbook crypto" versions, as these versionsare usually the subjects formany textbooks on cryptography. This book takes adifferent approach to introducingcryptography: it pays much more attention tofit-for-application aspects of cryptography. Itexplains why "textbook crypto" isonly good in an ideal world where data are random and badguys behave nicely.It reveals the general unfitness of "textbook crypto" for the real world bydemonstratingnumerous attacks on such schemes, protocols and systems under variousrealworldapplication scenarios. This book chooses to introduce a set of practicalcryptographic schemes, protocols and systems, many of them standards or de factoones, studies them closely,explains their working principles, discusses their practicalusages, and examines their strong(i.e., fit-for-application) security properties, oftenwith security evidence formally established.The book also includes self-containedtheoretical background material that is the foundation formodern cryptography.Table of ContentsModern Cryptography: Theory and PracticeBy Wenbo Mao Hewlett-Packard CompanyPublisher: Prentice Hall PTRPub Date: July 25, 2003ISBN: 0-13-066943-1Pages: 648CopyrightHewlett-Packard® Professional BooksA Short Description of the BookPrefaceScopeAcknowledgementsList of FiguresList of Algorithms, Protocols and AttacksPart I: IntroductionChapter 1. Beginning with a Simple Communication GameSection 1.1. A Communication GameSection 1.2. Criteria for Desirable Cryptographic Systems and Protocols Section 1.3. Chapter SummaryExercisesChapter 2. Wrestling Between Safeguard and AttackSection 2.1. IntroductionSection 2.2. EncryptionSection 2.3. Vulnerable Environment (the Dolev-Yao Threat Model)Section 2.4. Authentication ServersSection 2.5. Security Properties for Authenticated Key Establishment Section 2.6. Protocols for Authenticated Key Establishment Using Encryption Section 2.7. Chapter SummaryExercisesPart II: Mathematical Foundations: Standard NotationChapter 3. Probability and Information TheorySection 3.1. IntroductionSection 3.2. Basic Concept of ProbabilitySection 3.3. PropertiesSection 3.4. Basic CalculationSection 3.5. Random Variables and their Probability DistributionsSection 3.6. Birthday ParadoxSection 3.7. Information TheorySection 3.8. Redundancy in Natural LanguagesSection 3.9. Chapter SummaryExercisesChapter 4. Computational ComplexitySection 4.1. IntroductionSection 4.2. Turing MachinesSection 4.3. Deterministic Polynomial TimeSection 4.4. Probabilistic Polynomial TimeSection 4.5. Non-deterministic Polynomial TimeSection 4.6. Non-Polynomial BoundsSection 4.7. Polynomial-time IndistinguishabilitySection 4.8. Theory of Computational Complexity and Modern Cryptography Section 4.9. Chapter SummaryExercisesChapter 5. Algebraic FoundationsSection 5.1. IntroductionSection 5.2. GroupsSection 5.3. Rings and FieldsSection 5.4. The Structure of Finite FieldsSection 5.5. Group Constructed Using Points on an Elliptic CurveSection 5.6. Chapter SummaryExercisesChapter 6. Number TheorySection 6.1. IntroductionSection 6.2. Congruences and Residue ClassesSection 6.3. Euler's Phi FunctionSection 6.4. The Theorems of Fermat, Euler and LagrangeSection 6.5. Quadratic ResiduesSection 6.6. Square Roots Modulo IntegerSection 6.7. Blum IntegersSection 6.8. Chapter SummaryExercisesPart III: Basic Cryptographic TechniquesChapter 7. Encryption — Symmetric TechniquesSection 7.1. IntroductionSection 7.2. DefinitionSection 7.3. Substitution CiphersSection 7.4. Transposition CiphersSection 7.5. Classical Ciphers: Usefulness and SecuritySection 7.6. The Data Encryption Standard (DES)Section 7.7. The Advanced Encryption Standard (AES)Section 7.8. Confidentiality Modes of OperationSection 7.9. Key Channel Establishment for Symmetric Cryptosystems Section 7.10. Chapter SummaryExercisesChapter 8. Encryption — Asymmetric TechniquesSection 8.1. IntroductionSection 8.2. Insecurity of "Textbook Encryption Algorithms"Section 8.3. The Diffie-Hellman Key Exchange ProtocolSection 8.4. The Diffie-Hellman Problem and the Discrete Logarithm Problem Section 8.5. The RSA Cryptosystem (Textbook Version)Section 8.6. Cryptanalysis Against Public-key CryptosystemsSection 8.7. The RSA ProblemSection 8.8. The Integer Factorization ProblemSection 8.9. Insecurity of the Textbook RSA EncryptionSection 8.10. The Rabin Cryptosystem (Textbook Version)Section 8.11. Insecurity of the Textbook Rabin EncryptionSection 8.12. The ElGamal Cryptosystem (Textbook Version)Section 8.13. Insecurity of the Textbook ElGamal EncryptionSection 8.14. Need for Stronger Security Notions for Public-key CryptosystemsSection 8.15. Combination of Asymmetric and Symmetric CryptographySection 8.16. Key Channel Establishment for Public-key CryptosystemsSection 8.17. Chapter SummaryExercisesChapter 9. In An Ideal World: Bit Security of The Basic Public-Key Cryptographic Functions Section 9.1. IntroductionSection 9.2. The RSA BitSection 9.3. The Rabin BitSection 9.4. The ElGamal BitSection 9.5. The Discrete Logarithm BitSection 9.6. Chapter SummaryExercisesChapter 10. Data Integrity TechniquesSection 10.1. IntroductionSection 10.2. DefinitionSection 10.3. Symmetric TechniquesSection 10.4. Asymmetric Techniques I: Digital SignaturesSection 10.5. Asymmetric Techniques II: Data Integrity Without Source Identification Section 10.6. Chapter SummaryExercisesPart IV: AuthenticationChapter 11. Authentication Protocols — PrinciplesSection 11.1. IntroductionSection 11.2. Authentication and Refined NotionsSection 11.3. ConventionSection 11.4. Basic Authentication TechniquesSection 11.5. Password-based AuthenticationSection 11.6. Authenticated Key Exchange Based on Asymmetric CryptographySection 11.7. Typical Attacks on Authentication ProtocolsSection 11.8. A Brief Literature NoteSection 11.9. Chapter SummaryExercisesChapter 12. Authentication Protocols — The Real WorldSection 12.1. IntroductionSection 12.2. Authentication Protocols for Internet SecuritySection 12.3. The Secure Shell (SSH) Remote Login ProtocolSection 12.4. The Kerberos Protocol and its Realization in Windows 2000Section 12.5. SSL and TLSSection 12.6. Chapter SummaryExercisesChapter 13. Authentication Framework for Public-Key CryptographySection 13.1. IntroductionSection 13.2. Directory-Based Authentication FrameworkSection 13.3. Non-Directory Based Public-key Authentication FrameworkSection 13.4. Chapter SummaryExercisesPart V: Formal Approaches to Security EstablishmentChapter 14. Formal and Strong Security Definitions for Public-Key Cryptosystems Section 14.1. IntroductionSection 14.2. A Formal Treatment for SecuritySection 14.3. Semantic Security — the Debut of Provable SecuritySection 14.4. Inadequacy of Semantic SecuritySection 14.5. Beyond Semantic SecuritySection 14.6. Chapter SummaryExercisesChapter 15. Provably Secure and Efficient Public-Key CryptosystemsSection 15.1. IntroductionSection 15.2. The Optimal Asymmetric Encryption PaddingSection 15.3. The Cramer-Shoup Public-key CryptosystemSection 15.4. An Overview of Provably Secure Hybrid CryptosystemsSection 15.5. Literature Notes on Practical and Provably Secure Public-key Cryptosystems Section 15.6. Chapter SummarySection 15.7. ExercisesChapter 16. Strong and Provable Security for Digital SignaturesSection 16.1. IntroductionSection 16.2. Strong Security Notion for Digital SignaturesSection 16.3. Strong and Provable Security for ElGamal-family SignaturesSection 16.4. Fit-for-application Ways for Signing in RSA and RabinSection 16.5. SigncryptionSection 16.6. Chapter SummarySection 16.7. ExercisesChapter 17. Formal Methods for Authentication Protocols AnalysisSection 17.1. IntroductionSection 17.2. Toward Formal Specification of Authentication ProtocolsSection 17.3. A Computational View of Correct Protocols — the Bellare-Rogaway Model Section 17.4. A Symbolic Manipulation View of Correct ProtocolsSection 17.5. Formal Analysis Techniques: State System ExplorationSection 17.6. Reconciling Two Views of Formal Techniques for SecuritySection 17.7. Chapter SummaryExercisesPart VI: Cryptographic ProtocolsChapter 18. Zero-Knowledge ProtocolsSection 18.1. IntroductionSection 18.2. Basic DefinitionsSection 18.3. Zero-knowledge PropertiesSection 18.4. Proof or Argument?Section 18.5. Protocols with Two-sided-errorSection 18.6. Round EfficiencySection 18.7. Non-interactive Zero-knowledgeSection 18.8. Chapter SummaryExercisesChapter 19. Returning to "Coin Flipping Over Telephone"Section 19.1. Blum's "Coin-Flipping-By-Telephone" ProtocolSection 19.2. Security AnalysisSection 19.3. EfficiencySection 19.4. Chapter SummaryChapter 20. AfterremarkBibliographyCopyrightLibrary of Congress Cataloging-in-Publication DataA CIP catalog record for this book can be obtained from the Library of Congress. Editorial/production supervision: Mary SudulCover design director: Jerry VottaCover design: Talar BoorujyManufacturing manager: Maura ZaldivarAcquisitions editor: Jill HarryMarketing manager: Dan DePasqualePublisher, Hewlett-Packard Books: Walter BruceA Short Description of the BookMany cryptographic schemes and protocols, especially those based on public-key cryptography,have basic or so-called "textbook crypto" versions, as these versions are usually the subjects formany textbooks on cryptography. This book takes a different approach to introducingcryptography: it pays much more attention to fit-for-application aspects of cryptography. Itexplains why "textbook crypto" is only good in an ideal world where data are random and badguys behave nicely. It reveals the general unfitness of "textbook crypto" for the real world bydemonstrating numerous attacks on such schemes, protocols and systems under various realworldapplication scenarios. This book chooses to introduce a set of practical cryptographicschemes, protocols and systems, many of them standards or de facto ones, studies them closely,explains their working principles, discusses their practical usages, and examines their strong(i.e., fit-for-application) security properties, often with security evidence formally established.The book also includes self-contained theoretical background material that is the foundation formodern cryptography.PrefaceOur society has entered an era where commerce activities, business transactions andgovernment services have been, and more and more of them will be, conducted and offered overopen computer and communications networks such as the Internet, in particular, viaWorldWideWeb-based tools. Doing things online has a great advantage of an always-onavailability to people in any corner of the world. Here are a few examples of things that havebeen, can or will be done online:Banking, bill payment, home shopping, stock trading, auctions, taxation, gambling, micropayment(e.g., pay-per-downloading), electronic identity, online access to medical records, virtual private networking, secure data archival and retrieval, certified delivery of documents, fair exchange of sensitive documents, fair signing of contracts,time-stamping,notarization, voting, advertising, licensing, ticket booking, interactive games, digitallibraries, digital rights management, pirate tracing, …And more can be imagined.Many cryptographic schemes and protocols, especially those based onpublic-keycryptography,have basic or so-called "textbook crypto" versions, as these versionsare usually the subjects formany textbooks on cryptography. This book takes adifferent approach to introducingcryptography: it pays much more attention tofit-for-application aspects of cryptography. Itexplains why "textbook crypto" isonly good in an ideal world where data are random and badguys behave nicely.It reveals the general unfitness of "textbook crypto" for the real world bydemonstratingnumerous attacks on such schemes, protocols and systems under variousrealworldapplication scenarios. This book chooses to introduce a set of practicalcryptographic schemes, protocols and systems, many of them standards or de factoones, studies them closely,explains their working principles, discusses their practicalusages, and examines their strong(i.e., fit-for-application) security properties, oftenwith security evidence formally established.The book also includes self-containedtheoretical background material that is the foundation formodern cryptography.PrefaceOur society has entered an era where commerce activities, business transactions andgovernment services have been, and more and more of them will be, conducted and offered overopen computer and communications networks such as the Internet, in particular, viaWorldWideWeb-based tools. Doing things online has a great advantage of an always-onavailability to people in any corner of the world. Here are a few examples of things that havebeen, can or will be done online:Banking, bill payment, home shopping, stock trading, auctions, taxation, gambling, micropayment(e.g., pay-per-downloading), electronic identity, online access to medical records, virtual private networking, secure data archival and retrieval, certified delivery of documents, fair exchange of sensitive documents, fair signing of contracts,time-stamping,notarization, voting, advertising, licensing, ticket booking, interactive games, digitallibraries, digital rights management, pirate tracing, …And more can be imagined.Fascinating commerce activities, transactions and services like these are only possible ifcommunications over open networks can be conducted in a secure manner. An effective solutionto securing communications over open networks is to apply cryptography. Encryption, digitalsignatures, password-based user authentication, are some of the most basic cryptographictechniques for securing communications. However, as we shall witness many times in this book,there are surprising subtleties and serious security consequences in the applicationsof even themost basic cryptographic techniques. Moreover, for many "fancier" applications, such as manylisted in the preceding paragraph, the basic cryptographic techniques are no longer adequate.With an increasingly large demand for safeguarding communications over open networks formore and more sophisticated forms of electronic commerce, business and services[a], anincreasingly large number of information security professionals will be needed for designing,developing, analyzing and maintaining information security systems and cryptographicprotocols. These professionals may range from IT systems administrators, information securityengineers and software/hardware systems developers whose products have securityrequirements, to cryptographers.[a] Gartner Group forecasts that total electronic business revenues for business to business (B2B) andbusiness to consumer (B2C) in the European Union will reach a projected US $2.6 trillion in 2004 (withprobability 0.7) which is a 28-fold increase from the level of 2000 [5]. Also, eMarketer [104] (page 41) reportsthat the cost to financial institutions (in USA) due to electronic identity theft was US $1.4 billion in 2002, andforecasts to grow by a compound annual growth rate of 29%.In the past few years, the author, a technical consultant on information security and cryptographic systems at Hewlett-Packard Laboratories in Bristol, has witnessed the phenomenon of a progressively increased demand for information security professionalsunmatched by an evident shortage of them. As a result, many engineers, who are oriented toapplication problems and may have little proper training in cryptography and informationsecurity have become "roll-up-sleeves" designers and developers for information securitysystems or cryptographic protocols. This is in spite of the fact that designing cryptographicsystems and protocols is a difficult job even for an expert cryptographer.The author's job has granted him privileged opportunities to review many information securitysystems and cryptographic protocols, some of them proposed and designed by "roll-up-sleeves"engineers and are for uses in serious applications. In several occasions, the author observed socalled"textbook crypto" features in such systems, which are the result of applications of cryptographic algorithms and schemes in ways they are usually introduced in many cryptographic textbooks. Direct encryption of a password (a secret number of a smallmagnitude) under a basic public-key encryption algorithm (e.g., "RSA") is a typical example oftextbook crypto. The appearances of textbook crypto in serious applications with a "nonnegligibleprobability" have caused a concern for the author to realize that the general danger oftextbook crypto is not widely known to many people who design and develop informationsecurity systems for serious real-world applications.Motivated by an increasing demand for information security professionals and a belief that theirknowledge in cryptography should not be limited to textbook crypto, the author has written thisbook as a textbook on non-textbook cryptography. This book endeavors to: Introduce a wide range of cryptographic algorithms, schemes and protocols with a particular emphasis on their non-textbook versions.Reveal general insecurity of textbook crypto by demonstrating a large number of attacks onand summarizing typical attacking techniques for such systems.Provide principles and guidelines for the design, analysis and implementation of cryptographic systems and protocols with a focus on standards.Study formalism techniques and methodologies for a rigorous establishment of strong andfit-for-application security notions for cryptographic systems and protocols. Include self-contained and elaborated material as theoretical foundations of modern cryptography for readers who desire a systematic understanding of the subject.ScopeModern cryptography is a vast area of study as a result of fast advances made in the past thirtyyears. This book focuses on one aspect: introducing fit-for-application cryptographic schemesand protocols with their strong security properties evidently established.The book is organized into the following six parts:Part I This part contains two chapters (1—2) and serves an elementary-level introductionfor the book and the areas of cryptography and information security. Chapter 1 begins witha demonstration on the effectiveness of cryptography in solving a subtle communicationproblem. A simple cryptographic protocol (first protocol of the book) for achieving "fair cointossing over telephone" will be presented and discussed. This chapter then carries on toconduct a cultural and "trade" introduction to the areas of study. Chapter 2 uses a series ofsimple authentication protocols to manifest an unfortunate fact in the areas: pitfalls areeverywhere.As an elementary-level introduction, this part is intended for newcomers to the areas.Part II This part contains four chapters (3—6) as a set of mathematical background knowledge, facts and basis to serve as a self-contained mathematical reference guide forthe book. Readers who only intend to "knowhow," i.e., know how to use thefit-forapplicationcrypto schemes and protocols, may skip this part yet still be able to follow most contents of the rest of the book. Readers who also want to "know-why," i.e., know whythese schemes and protocols have strong security properties, may find that this selfcontainedmathematical part is a sufficient reference material. When we present working principles of cryptographic schemes and protocols, reveal insecurity for some of them andreason about security for the rest, it will always be possible for us to refer to a precise pointin this part of the book for supporting mathematical foundations.This part can also be used to conduct a systematic background study of the theoreticalfoundations for modern cryptography.Part III This part contains four chapters (7—10) introducing the most basic cryptographicalgorithms and techniques for providing privacy and data integrity protections. Chapter 7 isfor symmetric encryption schemes, Chapter 8, asymmetric techniques. Chapter 9 considersan important security quality possessed by the basic and popular asymmetric cryptographicfunctions when they are used in an ideal world in which data are random. Finally, Chapter10 covers data integrity techniques.Since the schemes and techniques introduced here are the most basic ones, manyof themare in fact in the textbook crypto category and are consequently insecure. While the schemes are introduced, abundant attacks on many schemes will be demonstrated withwarning remarks explicitly stated. For practitioners who do not plan to proceed with an indepthstudy of fit-for-application crypto and their strong security notions, this textbook crypto part will still provide these readers with explicit early warning signals on the generalinsecurity of textbook crypto.Part IV This part contains three chapters (11—13) introducing an important notion inapplied cryptography and information security: authentication. These chapters provide awide coverage of the topic. Chapter 11 includes technical background, principles, a series ofbasic protocols and standards, common attacking tricks and prevention measures. Chapter12 is a case study for four well-known authentication protocol systems for real world applications. Chapter 13 introduces techniques which are particularly suitable for openfor-application) security properties, oftenwith security evidence formally established.The book also includes self-containedtheoretical background material that is the foundation formodern cryptography.systems which cover up-to-date and novel techniques.Practitioners, such as information security systems administration staff in an enterprise andsoftware/hardware developers whose products have security consequences may find thispart helpful.Part V This part contains four chapters (14—17) which provide formalism and rigoroustreatments for strong (i.e., fit-for-application) security notions for public-key cryptographictechniques (encryption, signature and signcryption) and formal methodologies for theanalysis of authentication protocols. Chapter 14 introduces formal definitions of strongsecurity notions. The next two chapters are fit-for-application counterparts to textbookcrypto schemes introduced in Part III, with strong security properties formally established(i.e., evidently reasoned). Finally, Chapter 17 introduces formal analysismethodologiesand techniques for the analysis of authentication protocols, which we have not been able todeal with in Part IV.Part VI This is the final part of the book. It contains two technical chapters (18—19) and ashort final remark (Chapter 20). The main technical content of this part, Chapter 18, introduces a class of cryptographic protocols called zero-knowledge protocols. Theseprotocols provide an important security service which is needed in various "fancy" electronic commerce and business applications: verification of a claimed property of secretdata (e.g., in conforming with a business requirement) while preserving a strict privacyquality for the claimant. Zero-knowledge protocols to be introduced in this part exemplifythe diversity of special security needs in various real world applications, which are beyondconfidentiality, integrity, authentication and non-repudiation. In the final technical chapterof the book (Chapter 19) we will complete our job which has been left over from the firstprotocol of the book: to realize "fair coin tossing over telephone." That final realization willachieve a protocol which has evidently-established strong security properties yet with anefficiency suitable for practical applications.Needless to say, a description for each fit-for-application crypto scheme or protocol has to beginwith a reason why the textbook crypto counterpart is unfit for application. Invariably, thesereasons are demonstrated by attacks on these schemes or protocols, which, by the nature ofattacks, often contain a certain degree of subtleties. In addition, a description of a fit-forapplicationscheme or protocol must also end at an analysis that the strong (i.e.,fit-forapplication)security properties do hold as claimed. Consequently, some parts of this book inevitably contain mathematical and logical reasonings, deductions and transformations in orderto manifest attacks and fixes.While admittedly fit-for-application cryptography is not a topic for quick mastery or that can bemastered via light reading, this book, nonetheless, is not one for in-depth researchtopics whichwill only be of interest to specialist cryptographers. The things reported and explained in it arewell-known and quite elementary to cryptographers. The author believes that they can also becomprehended by non-specialists if the introduction to the subject is provided with plenty ofexplanations and examples and is supported by self-contained mathematical background andreference material.The book is aimed at the following readers.Students who have completed, or are near to completion of, first degree courses in computer, information science or applied mathematics, and plan to pursue a career ininformation security. For them, this book may serve as an advanced course in appliedcryptography.Security engineers in high-tech companies who are responsible for the design and development of information security systems. If we say that the consequence of textbookcrypto appearing in an academic research proposal may not be too harmful since the worstcase of the consequence would be an embarrassment, then the use of textbook crypto in aninformation security product may lead to a serious loss. Therefore, knowing the unfitness oftextbook crypto for real world applications is necessary for these readers. Moreover, thesereaders should have a good understanding of the security principles behind thefit-forapplicationschemes and protocols and so they can apply the schemes and the principles correctly. The self-contained mathematical foundations material in Part II makes the book asuitable self-teaching text for these readers.Information security systems administration staff in an enterprise andsoftware/hardwaresystems developers whose products have security consequences. For these readers, Part Iis a simple and essential course for cultural and "trade" training; Parts III and IV form asuitable cut-down set of knowledge in cryptography and information security. These threeparts contain many basic crypto schemes and protocols accompanied with plenty of attacking tricks and prevention measures which should be known to and can be grasped by。
- 1、下载文档前请自行甄别文档内容的完整性,平台不提供额外的编辑、内容补充、找答案等附加服务。
- 2、"仅部分预览"的文档,不可在线预览部分如存在完整性等问题,可反馈申请退款(可完整预览的文档不适用该条件!)。
- 3、如文档侵犯您的权益,请联系客服反馈,我们会尽快为您处理(人工客服工作时间:9:00-18:30)。
Computational Complexity inNon-Turing Models of ComputationThe What ,the Why and the HowEd Blakey 1,2Oxford University Computing Laboratory,Wolfson Building,Parks Road,Oxford,OX13QD,United KingdomAbstractWe preliminarily recap what is meant by complexity and non-Turing computation ,by way of explanation of our title,‘Computational Complexity in Non-Turing Models of Computation’.Based on investigation of a motivating example,we argue that traditional complexity theory does not adequately capture the true complexity of certain non-Turing computers,and,hence,that an extension of the theory is needed in order to accommodate such machines.We propose a framework of complexity that is not computation-model-dependent—that,rather,is extensible so as to accommodate diverse computational models—,and that allows meaningful comparison of computers’respective complexities,whether or not the comparison be with respect to different resources ,and whether or not the computers be instances of different models of computation .Whilst,we suggest,complexity theory is—without some modification—of limited applicability to certain non-standard models,we hope that the ideas described here go some way to showing how such modification can be made,and that members of the non-Turing-computation community—not least participants of Quantum Physics and Logic/Development of Computational Models 2008—find these ideas both useful and interesting.Keywords:Computational complexity,non-standard/non-Turing computational models,precision.This work forms part of the author’s ongoing studies for his doctoral degree.A more complete account of this project can be found in [3],which is available at /~quee1871/transfer.pdf .1What......do we mean by complexity and non-Turing computation ?1We thank Bob Coecke and Jo¨e l Ouaknine (at Oxford)for their support,supervision and suggestions;Samson Abramsky and Peter Jeavons (at Oxford)for their useful comments about this project;Viv Kendon and colleagues (at Leeds)for useful discussions regarding the application of this work to quantum computing;Jonathan Mills (at Indiana,USA),Susan Stepney (at York)and others for their comments and suggestions made at Unconventional Computing 2007;and participants of the Second International Workshop on Natural Computing for their encouraging feedback and discussion.2Email:edward.blakey@Electronic Notes in Theoretical Computer Science 270 (2011) 17–281571-0661/$ – see front matter © 2011 Elsevier B.V. All rights reserved./locate/entcsdoi:10.1016/j.entcs.2011.01.003In this section,we recall briefly what is meant by computational complexity ,and note the concept’s (understandable)bias towards the Turing machine.We also note,however,the widespread discussion—and,indeed,practical use—of other models,and ask whether more is needed of complexity theory in order to allow suitable analysis of non-standard computers.1.1ComplexityThe field of computational complexity strives to categorize problems according to the cost of their solution.This cost is the resource (run-time,memory space or similar)consumed during computation;complexity theorists are interested particularly in the increase in resource as the computation’s input grows.So that the notions of complexity theory are well defined,the field has largely been developed relative to a specific computation model :the Turing machine.This choice is rarely seen as problematic:a vast majority of practical computation con-forms to this model (specifically,real-world computations are typically performed by digital computers running programs that implement algorithms);the almost exclu-sive consideration of Turing machines is further bolstered—at least for those who overlook the distinction between computability and complexity—by the Church-Turing thesis 3.Consequently,resource is virtually always taken to be a property—usually run-time—of a Turing machine (or algorithm,random access machine or similar).4Because of the prevalence of digital computers,then,and because of the con-jectured equivalence of the Turing machine to other computational models,this restriction of complexity theory to the exclusively algorithmic is seldom explicitly considered,let alone viewed as cause for concern.Indeed,the field is very successful,not only theoretically,but also practically,offering excellent guidance,for example,on the allocation of computational effort.1.2Non-Standard Computational ModelsHowever,there has long been—and is today—an active community working on non-Turing forms of computer 5:•mechanical means by which differential/integral equations are solved (e.g.,the Differential Analyzer;see [11]);•the formation of soap bubbles between parallel plates,as used to find minimal-length spanning networks (possibly with additional vertices)connecting given vertices (see [17]);•DNA-computing techniques that can tackle the directed Hamiltonian path prob-lem (see [2]),amongst other graph-theoretic/combinatorial problems;3The Church-Turing thesis is introduced in [12]and discussed in [8]and [20],amongst many others.4Having said this,we acknowledge that consideration has been made—though in a largely ad hoc,and,hence,far from unified,way—of complexity in non-standard computational models.We note,however,that the true complexity of such computation is not always captured by such consideration;notably,this is true in the (circuit-model)quantum-computer case—see Sect.3.1.5We note also the growing recognition (see,e.g.,[1]and [14])of such computers’importance.E.Blakey /Electronic Notes in Theoretical Computer Science 270(2011)17–2818E.Blakey/Electronic Notes in Theoretical Computer Science270(2011)17–2819•standard(circuit-model[18])and non-standard(adiabatic[15,16],measurement-based[19],continuous-variable[9],etc.)quantum computers;•optical methods offering novel approaches to the Travelling Salesman problem (see[13]),etc.;•the work of participants of Quantum Physics and Logic/Development of Compu-tational Models2008;•and so on.Despite the dominance of digital computers,non-Turing systems are of increasing importance.Just as we can analyse the complexity of algorithms(and Turing machines, etc.),so we wish to be able to analyse the complexity of non-standard computers, not least so that we can compare the efficiency of our non-standard solutions with that of their digital counterparts.One may hope,then,that the complexity of non-Turing computers can be adequately analysed using the existing tools of traditional complexity theory.We shall see that this is not the case:the Turing-machine-focused measures fail to capture the true complexity of certain systems.2Why......do non-Turing computers warrant different approaches to complexity analysis?We claim above that traditional,Turing-based complexity theory overlooks the true complexity of some computing systems.In this section,we justify this claim by exhibiting just such a system and discussing its complexity.2.1Analogue Factorization SystemWe now outline an analogue system that factorizes natural numbers.The descrip-tion given here is brief;for full details,see[4].(The interested reader should also see[7],the pending US patent,of which the system is the subject,applied for by IBM and with the author as sole inventor,and[6],which describes a modified and in some senses improved version of the system.Finally,the system is used,as here, as a motivating example in[3].)Just as with traditional algorithms,there is a practical limit to the size of num-bers that the system can factorize;in contrast with traditional algorithms,however, the system suffers no increase in calculation time as the input number approaches this limit.Crucially for present purposes,the limit is not imposed by considerations (of run-time,memory space,etc.)made in traditional complexity theory,but rather by the increasing precision demanded of the user of the system.66We see here thefirst hints of a need for an extension of complexity theory.Geometric formulation.Note first that the task of factorizing natural number n has a geometric formu-lation:the task is exactly that of finding points both in the integer grid Z 2and on the curve y =n x (the coordinates of such a point are two of the sought factors).Since factors of n are members of {0,...,n }(in fact,of {1,...,n }),we need search for grid/curve points (x,y )only in the region 0≤x,y ≤n ;and since (x,y )reveals the same factors as (y,x ),we need search only in the region 0≤x ≤y ≤n .The curve y =n x is a conic section (specifically,a hyperbola),and so is the intersection of the (x,y )-plane and a cone ;the factorization method’s implementation exploits this fact.Implementation of the grid.We implement the part of the integer grid that is of interest (i.e.,that lies inthe region identified above)using a source S (of wavelength 2n )of transverse waves,reflected by mirrors M 1,M 2and M 3so as to produce a certain interference pattern;the points of maximal wave activity in this pattern model integer grid points,with maximally active point a n ,b n ,0 modelling grid point (a,b )(where a,b ∈Z ).7Since the wavelength of radiation from S depends on n ,its being set forms part of the computation’s input process.See Fig.1for the apparatus used in implementing the integer grid (in which B is a black body that absorbs some radiation from S ),Fig.2for the route of propagation of a sample ray 8,and Fig.3for the maxima (shown as dots)of the resultant interference pattern within the region R :={(x,y,0)|0≤x ≤y ≤1}that,since a n ,b n ,0models (a,b ),models the region 0≤x ≤y ≤n of interest (this figure takes as its example n =5).Implementation of the cone.The cone is implemented by a source P n of radiation (the vertex of the cone)and a part-circular sensor C n (the circle is a cross section of the cone).9See Fig.4.The subscripts reflect the fact that the positions of P n and C n depend on n ;the positioning of these components forms part of the input process for the computation.Interpreting output.Having described the apparatus and alluded to the input method (namely,set-ting to the appropriate,n -dependent values the wavelength of S and positions of P n and C n ),we turn to the interpretation of output.Radiation arriving from P n at a point on C n displays high-amplitude interference (due to the pattern of waves 7In fact,these maximally active points model those integer-grid points that have coordinates of the same parity .This is sufficient provided that we assume n to be odd,for then any factors of n are odd.(Should a factorization be required of an even number,it is computationally trivial—in the Turing-machine realm—iteratively to divide by two until an odd number,which can be factorized as described here,is obtained.)8Note that the ray is reflected back along itself by M 3;this produces a standing wave .9It is proven in [4]that the curve of C n is the circular arc produced by projecting from P n the curve G n := (x,y,0)∈R |1xy =n onto the plane y =2−x .Hence,radiation arriving from P n at a point onC n passes through the plane z =0at a point (x,y,0)such that 1xy =n .E.Blakey /Electronic Notes in Theoretical Computer Science 270(2011)17–2820E.Blakey/Electronic Notes in Theoretical Computer Science270(2011)17–2821grid.Fig.1.The layout of the apparatus that implements the integer Array.Fig.2.The path of a ray propagating from S via the three mirrors M i back to S Array Fig.3.The maximum amplitude points in the region R,where n=5.Fig.4.The layout of the apparatus that implements the cone.from S )if and only if the point (x,y,0)at which it meets the (x,y )-plane models aninteger point;that is,if and only if 1x ,1y ∈Z .Further,by construction of P n and C n ,1x ·1y =n (see footnote 9).Hence,this radiation displays high-amplitude interferenceif and only if 1x and 1y are factors of n .Thus,to interpret the results,we measure the coordinates of a point (that which displays high-amplitude interference)on C n and convert them into those of a point (that through which the ray passes)in the(x,y )-plane.Explicitly,radiation from P n incident on a point (a,2−a,c )on C n passes through a n (2−a ) 2−a na ,0 ;hence,if the radiation arriving from P n at (a,2−a,c )on C n displays high-amplitude interference,then a n (2−a ), 2−a na ,0 models an integer point on the hyperbola under consideration: n (2−a )a and na 2−a are factors of n ;conversely,all factors have such a point on C n .Having set up the apparatus as described here,then,the factors of n are so found.We have outlined an analogue factorization system.10It offers much-improved run-times when compared with existing,algorithmic solutions,largely because it di-rectly,physically implements the problem rather than converting the problem into a contrived instance of the standard computation model.The polynomial time and space complexities 11serve,however,to highlight not the power of the system but the incompleteness of traditional complexity theory.As n increases,the system does require exponentially more resource (though neither specifically time nor space);in particular,the precision with which n must be input (by setting the wavelength of S and the positions of P n and C n )and its factors read (by measuring the positions of 10We reiterate that [4]offers a more complete description of the system;it also offers proof of the system’s correct functioning.11These are discussed further in the following section,and formalized in [3].E.Blakey /Electronic Notes in Theoretical Computer Science 270(2011)17–2822points on C n )increases exponentially with n .This suggests that,for some comput-ers,traditional,‘algorithmic’complexity theory is inadequate;we aim to introduce notions of complexity more suitable in such contexts.2.2Complexity of the SystemUsing the system.The use of the system to factorize n consists of:(i)calculation of the values 2n (to be used as the wavelength of S )and 2n (to be used as the z -coordinate of P n and of the centre of the circle of C n );(ii)supply of n to the system,by adjusting the wavelength of S and the height(z -coordinate)of P n and C n in accordance with the values found during (i );(iii)interference of the radiation in the system,which entails propagation of the ra-diation over a fixed distance (since the same apparatus is—bar the adjustments made in (ii )—used for all values of n );(iv)measurement of the positions of high-amplitude interference points on the sen-sor C n ;and(v)conversion of the positions measured during (iv )into factors:(a,2−a,c )→ n (2−a )a , na 2−a .We consider now the computational complexity,with respect to several resources (formally defined in [3]),of these steps of the factorization system’s use.Time complexity.Consider first time ;the time complexity of the system,we claim,is polynomial in the size (i.e.,number of digits)of the input value.Note that,in the Turing-machine realm,steps (i )and (v )above take only poly-nomially long (in the size of n ),the former since 2n and 2n need be calculatedonly with sufficient precision that n can be retrieved given that n ∈Z ,and the latter since sought values n (2−a )a and na 2−a are integers.Note further that steps (ii )–(iv )take a constant time:larger values of n take no longer to process in accor-dance with these stages.Notably,step (iii ),during which the actual factorization is performed,takes constant time;compare this with known algorithmic methods,where computation time increases exponentially with the size of n (see,e.g.,[10]).Thus,the time complexity of the system as a whole is,as claimed,polynomial in the size of the input.E.Blakey /Electronic Notes in Theoretical Computer Science 270(2011)17–2823Space complexity.Similarly,when considering the resource of space ,12we see that only the Turing-machine calculations of steps (i )and (v )(which essentially prepare input and in-terpret output)consume an increasing volume as n increases,and these only a polynomially increasing volume (in the size of the input);and for steps (ii )–(iv ),the same,fixed-size apparatus—occupying the same,fixed space—is used for all values of n (though the positions of P n and C n depend on n ,there exists a finite,n -independent,bounding cuboid in which the apparatus lies for all n ).Thus,the space complexity of the system is polynomial in the size of the input.The resources of time and space are arguably of paramount relevance when con-sidering instances (Turing machines,random access machines,etc.)of the standard,algorithmic computational model.Notions of complexity developed with only these instances in mind,however,are understandably poor at capturing the complexity of instances of wildly different models;the factorization system above does indeed have polynomial time and space complexities,and yet does require exponentially increasing resource as n increases.Notably,larger values of n require exponentially increasingly precise manipulation of the input parameters (the wavelength of S and the positions of P n and C n )and exponentially increasingly precise measurement of the output parameters (the coordinates of points on C n ),and there is no reason for which we should not view required precision as a resource.Accordingly,we consider now the precision complexity of the system,which is certainly not polynomial,and hence better captures the system’s true complexity than do the resources of time and space.Precision complexity.The intention of precision complexity is to capture the lack of robustness against input/output imprecision of a ‘physical’(e.g.,analogue/optical/chemical)computer.We consider the example of setting the wavelength in our factorization system;other input parameters (the positions of P n and C n )and output parameters (the positions of points on C n ),which we omit for brevity,13can be analysed similarly.(A formal account/definition of precision complexity is given in [3].)Given n ,which we wish to factorize,we intend to set the wavelength to 2n .In practice,due to technological limitations on our control over the wavelength (we have in mind imprecise use of a variable resistor or similar),we may set the wavelength to 2n ,which we know only to lie in 2n − ,2n + for some real error term .However,we may engineer the system (using standard analogue techniques)so that non-integer input values are rounded offso as to correct ‘small’errors:given wavelength 2x (for arbitrary x ∈R ),the value to be factorized is taken to be x +12 .So,provided that the supplied wavelength 2n ∈ 2n − ,2n + falls in the interval 12Space,as traditionally encountered with Truing machines,etc.,can be viewed as the storage capacity of the memory required by a computation;we consider the analogous notion of required physical volume .13The omission,whilst partly for brevity,also reflects the redundancy of consideration of other parame-ters’precision requirements:once the setting of the wavelength has been shown to require exponentially increasing precision,then we have that the overall precision complexity is exponential,regardless of the contribution from other parameters.E.Blakey /Electronic Notes in Theoretical Computer Science 270(2011)17–28242 n+12,2n−12of values‘corrected’to n—i.e.,provided that <1n(n+12)—,then thesystem processes the correct input value.Note that this constraint on implies that the precision required of us when setting the wavelength increases quadratically with n,and hence exponentially with the size of n.We see,then,that the system’s precision complexity,which we informally in-troduce here and,we iterate,treat formally in[3],is exponential,and therefore of much greater significance than the system’s polynomial time/space complexity.The significant complexity measure is overlooked by traditional complexity theory.3How......should we measure non-Turing computers’complexity,and how can such complexity be meaningfully compared with that of Turing machines?There are two aspects to the way in which,we suggest,complexity in non-standard computation should be analysed.•First,we advocate consideration of more diverse selections of resources;for exam-ple,we see above that not only traditional,algorithmic measures such as time and space,but also more physical measures such as precision,should be considered. See Sect.3.1.•Secondly,we should like to be able to compare in a meaningful way the respec-tive complexities of instances of different computational models,with respect to different complexity resources;we accordingly advocate use of the notion of dom-inance,which offers a criterion that tells us which of a computation’s resources are‘relevant’;once such are identified,they can be compared within the usual ‘∈O’pre-ordering.See Sect.3.2.3.1Resources in Non-Turing ComputationWe note above the following:•that traditional complexity theory and the resources(time,space,etc.)considered therein are inspired by the Turing-machine model of computation,almost totally to the exclusion of other models;•that,while this is clearly adequate when considering Turing machines,certain non-standard computers(quantum computers being a timely example)are inad-equately catered for by these resources;and•that it is possible(and even natural,once the problem has been acknowledged) to define resources(e.g.,precision)that better capture the true complexity of non-Turing computers.The solution is obvious,then:when working with a non-standard compu-tational model,we should consider which resources—both algorithmic and non-algorithmic—are consumed during computation,and should explicitly measure the complexity of our computation with respect to these resources;this gives a moreE.Blakey/Electronic Notes in Theoretical Computer Science270(2011)17–2825complete picture,and more confidence in our understanding,of the computation’s complexity.The need for consideration of different resources is particularly evident in the quantum-computer case.An arbitrary algorithm (or Turing machine or similar)can,by definition of complete,be expressed as a conversion of input to output via operations exclusively taken from some complete set of what are deemed to be ‘atomic’operations.For a given input value,the number of such operations performed during this conversion is an accurate measure (or,depending on view-point,a definition)of run-time.Similarly,an arbitrary quantum computation can be expressed as the preparation of several quantum bits,followed by a sequence of applications to subsets of these quantum bits of ‘atomic’unitary operations taken from a complete set,followed by a measurement of the system.As in the classical case,an enumeration of the invocations of these atomic operations gives a mea-sure of the system’s complexity;indeed,this is the basis of an existing definition of complexity of circuit-model quantum computing devices (see [18]).Also as in the classical case,however,the result is essentially a measure of run-time,which is not,we suggest,particularly relevant to quantum systems.14By introducing and considering new resources,specifically ones similar to pre-cision,we may,we suggest,better encapsulate the true complexity of a quantum system;this complexity arises,after all,because of our limited ability to take precise measurements from the system.3.2Comparing ComplexityWhereas reasoning directly about the computational complexity of a problem seems inherently difficult,it is relatively easy (once appropriate resources are considered)to ascertain the complexity of specific methods (algorithms,analogue computers,Turing machines,etc.)that solve the problem .Consequently,our sole understand-ing of a problem’s complexity is,in the majority of cases,gleaned via our having determined the complexity of solution methods for the problem;specifically,we have that the problem’s complexity is bounded from above by the complexity of the most efficient known solution method.In order to improve such bounds,it is desired to consider as large a set as is pos-sible of solution methods for a problem.Each set so considered in practice,however,is likely to be of solution methods taken from a single model of computation (often that of the Turing machine).This is a necessary evil of our inability meaningfully to compare the complexity of instances of different computation models.Required,then,is a more general framework in which to study complexity;in particular,we wish to be able to use the framework to consider on a consistent and comparable footing the complexity—with respect to several notions of resource—of instances of diverse models of computation;only when we can meaningfully 14The advantage of quantum computers over their classical counterparts arises primarily from the use of entangled states and the effective parallelism that such use allows;a disadvantage is the strictly constrained way in which information can be read from the quantum system.The run-time of such a system,then,is a reflection of neither the ‘amount of computation’being performed (due to the parallelism)nor the ‘difficulty’in using the system (which chiefly arises during measurement).E.Blakey /Electronic Notes in Theoretical Computer Science 270(2011)17–2826E.Blakey/Electronic Notes in Theoretical Computer Science270(2011)17–2827 compare,say,the respective complexities of a Turing machine and a DNA computer can we begin to consider larger,model-heterogeneous sets of solution methods,and hence obtain improved bounds on the complexity of problems.Accordingly,we introduce the notion of dominance of resource.3.2.1Dominance.Recall that,in the complexity analysis of the factorization system above,we suggest that precision,on which the system has an exponential dependency,is more relevant than either time or space,on each of which it has polynomial dependency.This can be formalized(and a general concept abstracted)by noting that T,S∈O(P), but neither P∈O(T)nor P∈O(S),where P,T and S stand respectively for the precision,time and space complexity functions;we say that,relative to{P,T,S}, precision is dominant.More generally,relative to a set{X1,...,X k}of complexity functions(with respect to respective resources x1,...,x k),resource x i is dominantif and only if X i∈O(X j)⇒X j∈O(X i)for all j.By considering for a given solution method(e.g.,Turing machine/analogue com-puter/quantum system)only the dominant resources,we focus on the relevant measures for the method—dominance formalizes a resource’s relevance:resources that are dominant impose the asymptotically greatest cost,to the extent that non-dominant resources may be disregarded as irrelevant.Further,we can compare solu-tion methods according to their relevant(i.e.,dominant)resources(using the‘∈O’pre-ordering).We therefore have a framework in which can be made meaningful and consistent comparisons of computation-model-heterogeneous sets of computers; the framework can accommodate instances of various models of computation,and provide structure according to cost in terms of various resources.(This framework and the notion of dominance on which it is based are investi-gated in greater detail in[5].)4ConclusionComplexity theory has developed with a bias towards the Turing-machine model. This is readily explained,and poses no problem in‘standard’use;we note,how-ever,that thefield is—without some modification—of limited applicability to non-standard models.We hope that the ideas described here(which are explored more fully in[3])go some way to showing how such modification can be made,and that members of the QPL/DCM2008communityfind these ideas both useful and in-teresting.References[1]Adamatzky,A.(editor),Int.J.of Unconventional Computing,Old City Publishing(2005onwards)[2]Adleman,L.M.,Molecular Computation of Solutions to Combinatorial Problems,Science266(1994),1021–1024[3]Blakey, E.,A Model-Independent Theory of Computational Complexity;Price:From Patience toPrecision(and Beyond),(2008)。