Matrix Derivative_wiki
[全]范德蒙矩阵详解
![[全]范德蒙矩阵详解](https://img.taocdn.com/s3/m/5a662511b14e852459fb577c.png)
范德蒙矩阵从行列式开始范德蒙行列式是长这个样子滴:然后呢,它的转置也叫做范德蒙行列式:为什么呢?因为矩阵转置,行列式的值不会变这里我就不推导了,直接给出范德蒙行列式的值:这是什么意思呢?假设有如下的范德蒙方阵:那么它的行列式等于:(3-2)*(5-2)*(5-3)=6假设4阶范德蒙行列式中有四个数2,3,5,7那么就等于(3-2)(5-2)(7-2)(5-3)(7-3)(7-5)=240 考试中经常出现与范德蒙行列式类似的结构,它们就差了那么一点点,我们需要做的就是将之转化为标准的范德蒙行列式便于计算。
这里我举一个例子。
上面这个行列式长得太像范德蒙矩阵了,只是没有三次项,我们给它补上。
这里除了补上三次项,还有未知数x,为什么呢?当然首先是只有方阵才有行列式。
这样整个行列式的值为:我们仅看x^3 项的系数(负的原行列式的值)就是答案:怎么理解呢?原因就是代数余子式按列展开等于行列式的值。
(1,x,x^2,x^3,x^4)^T按列展开一次得到对应级次的系数,我们这里只取x^3的系数就是对应上上个矩阵行列式的值了。
性质范德蒙矩阵的性质不多,有两条值得说一下:范德蒙矩阵与多项式的最小二乘拟合最后我想谈一谈范德蒙矩阵在多项式的最小二乘拟合中的应用用一个多项式去拟合若干个点:假设有n个采样点,拟合次数为k次,那么方差可以表示为:为求得方差的极小值,对a0,...ak依次求偏导为0。
移项看起来很乱,写成矩阵形式试试:em...这就是个什么矩阵,看起来好复杂滴哈哈,我们观察变形一下:上面的矩阵可以写成范德蒙矩阵相乘的形式哦!我们简记上面的矩阵为:X是竖着的范德蒙矩阵。
这样向量a等于:这里有个很关键的点,范德蒙矩阵不一定是个方阵,即采样点多于拟合多项式的最高次数,不是方阵就没有逆,但是只要不存在相同的两个采样点,那么一定是存在逆矩阵的,多项式的系数向量a也可表示出来了。
这就是基于最小二乘法的多项式拟合原理!。
Wiki技术在图书馆服务中的应用
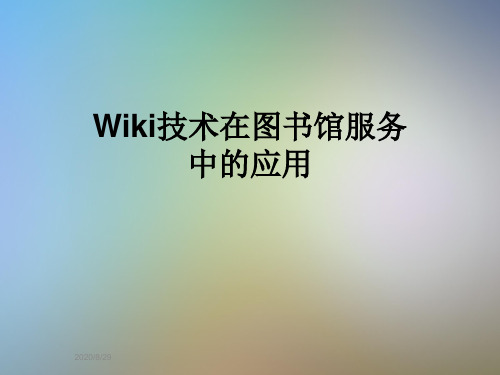
Wiki技术在图书馆中的应用
what
+ Wiki + 维基百科
Wiki技术在图书馆中的应用
what
Wiki技术在图书馆中的应用
what
+ 最早的wiki:wikiwikiweb + 最大的wiki:维基百科(wikipedia)
Wiki技术在图书馆中的应用
what
Wiki ≠维基百科
Wiki技术在图书馆中的应用
– 编辑 – 管理 – 使用技巧
• Microsoft office to wiki
• 所见即所得编辑器
– 内容管理
明尼苏达州大学图书馆职工网站
– 项目管理 – 业务管理及员工内部交流:厦门大学图
书馆“喂鸡” – 文档共笔:上海图书馆的“图书馆2.0”文
档工作组社区
Wiki技术在图书馆中的应用
图书馆专业领域
– 专业机构与组织:ALA wikis – 专业会议wiki:厦门大学图书馆维护的DL-
China 网站
图书馆服务
– 图书馆网站:USC Aiken GreggGraniteville Library
– 主题导览:St. Joseph County Public Library
– 社区wiki:SCRLD Wiki – Wiki化OPAC:Open worldcat
Wiki技术在图书馆中的应用
图书馆业务与管理
免费线上wiki站点
+Pbwiki:bull run library + Wikispaces:Rundlett-Middle-
School-Library
Wiki技术在图书馆中的应用
自己搭建wiki站点
derivative微分
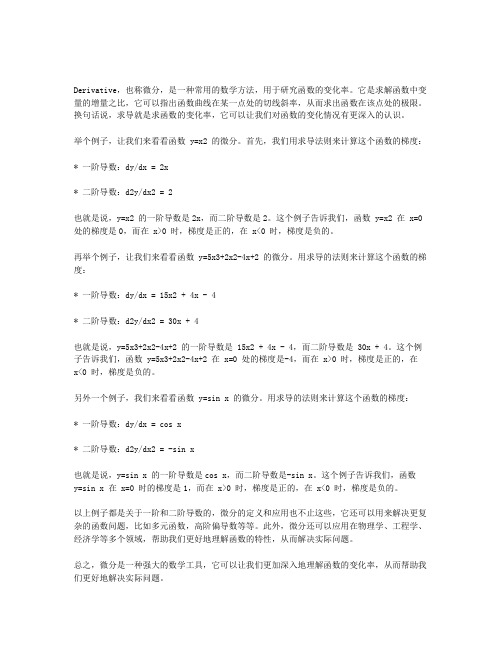
Derivative,也称微分,是一种常用的数学方法,用于研究函数的变化率。
它是求解函数中变量的增量之比,它可以指出函数曲线在某一点处的切线斜率,从而求出函数在该点处的极限。
换句话说,求导就是求函数的变化率,它可以让我们对函数的变化情况有更深入的认识。
举个例子,让我们来看看函数 y=x2 的微分。
首先,我们用求导法则来计算这个函数的梯度:* 一阶导数:dy/dx = 2x* 二阶导数:d2y/dx2 = 2也就是说,y=x2 的一阶导数是2x,而二阶导数是2。
这个例子告诉我们,函数 y=x2 在 x=0 处的梯度是0,而在 x>0 时,梯度是正的,在 x<0 时,梯度是负的。
再举个例子,让我们来看看函数 y=5x3+2x2-4x+2 的微分。
用求导的法则来计算这个函数的梯度:* 一阶导数:dy/dx = 15x2 + 4x - 4* 二阶导数:d2y/dx2 = 30x + 4也就是说,y=5x3+2x2-4x+2 的一阶导数是 15x2 + 4x - 4,而二阶导数是 30x + 4。
这个例子告诉我们,函数 y=5x3+2x2-4x+2 在 x=0 处的梯度是-4,而在 x>0 时,梯度是正的,在x<0 时,梯度是负的。
另外一个例子,我们来看看函数 y=sin x 的微分。
用求导的法则来计算这个函数的梯度:* 一阶导数:dy/dx = cos x* 二阶导数:d2y/dx2 = -sin x也就是说,y=sin x 的一阶导数是cos x,而二阶导数是-sin x。
这个例子告诉我们,函数y=sin x 在 x=0 时的梯度是1,而在 x>0 时,梯度是正的,在 x<0 时,梯度是负的。
以上例子都是关于一阶和二阶导数的,微分的定义和应用也不止这些,它还可以用来解决更复杂的函数问题,比如多元函数,高阶偏导数等等。
此外,微分还可以应用在物理学、工程学、经济学等多个领域,帮助我们更好地理解函数的特性,从而解决实际问题。
三阶矩阵特征根范文
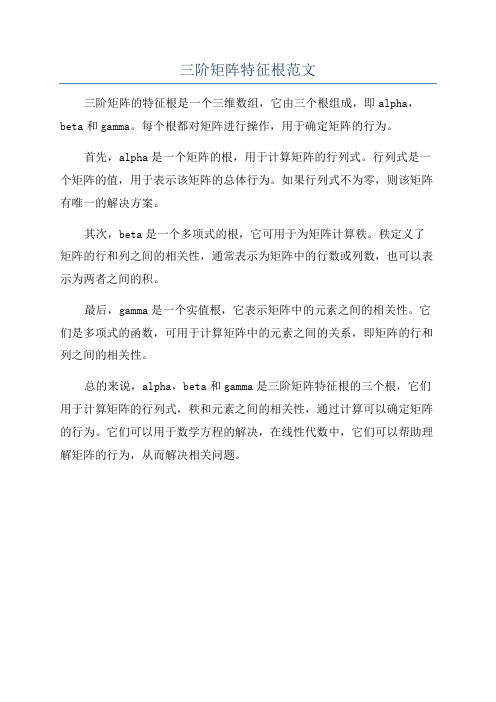
三阶矩阵特征根范文
三阶矩阵的特征根是一个三维数组,它由三个根组成,即alpha,beta和gamma。
每个根都对矩阵进行操作,用于确定矩阵的行为。
首先,alpha是一个矩阵的根,用于计算矩阵的行列式。
行列式是一个矩阵的值,用于表示该矩阵的总体行为。
如果行列式不为零,则该矩阵有唯一的解决方案。
其次,beta是一个多项式的根,它可用于为矩阵计算秩。
秩定义了矩阵的行和列之间的相关性,通常表示为矩阵中的行数或列数,也可以表示为两者之间的积。
最后,gamma是一个实值根,它表示矩阵中的元素之间的相关性。
它们是多项式的函数,可用于计算矩阵中的元素之间的关系,即矩阵的行和列之间的相关性。
总的来说,alpha,beta和gamma是三阶矩阵特征根的三个根,它们用于计算矩阵的行列式,秩和元素之间的相关性,通过计算可以确定矩阵的行为。
它们可以用于数学方程的解决,在线性代数中,它们可以帮助理解矩阵的行为,从而解决相关问题。
3D数学矩阵更多知识(自动保留)

3D数学---- 矩阵的更多知识(2)矩阵的逆另外一种重要的矩阵运算是矩阵的求逆,那个运算只能用于方阵。
运算法那么方阵M的逆,记作M-1,也是一个矩阵。
当M与M-1相乘时,结果是单位矩阵。
表示为公式9.6的形式:并非所有的矩阵都有逆。
一个明显的例子是假设矩阵的某一行或列上的元素都为0,用任何矩阵乘以该矩阵,结果都是一个零矩阵。
若是一个矩阵有逆矩阵,那么称它为可逆的或非奇异的。
若是一个矩阵没有逆矩阵,那么称它为不可逆的或奇异矩阵。
奇异矩阵的行列式为0,非奇异矩阵的行列式不为0,因此检测行列式的值是判定矩阵是不是可逆的有效方式。
另外,关于任意可逆矩阵M,当且仅当v=0时,vM=0。
M的”标准伴随矩阵“记作”adj M“,概念为M的代数余子式矩阵的转置矩阵。
下面是一个例子,考虑前面给出的3x3阶矩阵M:计算M的代数余子式矩阵:M的标准伴随矩阵是代数余子式矩阵的转置:一旦有了标准伴随矩阵,通过除以M的行列式,就能够计算矩阵的逆。
其表示如公式9.7所示:例如为了求得上面矩阵的逆,有:固然还有其他方式能够用来计算矩阵的逆,比如高斯消元法。
很多线性代数书都断定该方式更适合在运算机上实现,因为它所利用的代数运算较少,这种说法实际上是不正确的。
关于大矩阵或某些特殊矩阵来讲,这或许是对的。
但是,关于低阶矩阵,比如几何应用中常见的那些低阶矩阵,标准伴随矩阵可能更快一些。
因为能够为标准伴随矩阵提供无分支(branchless)实现,这种实现方式在现今的超标量体系结构和专用向量处置器上会更快一些。
矩阵的逆的重要性质:几何说明矩阵的逆在几何上超级有效,因为它使得咱们能够计算变换的”反向“或”相反“变换---- 能”撤销“原变换的变换。
因此,若是向量v 用矩阵M来进行变换,接着用M的逆M-1进行变换,将会取得原向量。
这很容易通过代数方式验证:矩阵的行列式在任意方阵中都存在一个标量,称作该方阵的行列式。
线性运算法那么方阵M的行列式记作|M|或“det M”,非方阵矩阵的行列式是未概念的。
Matrix Derivative_wiki
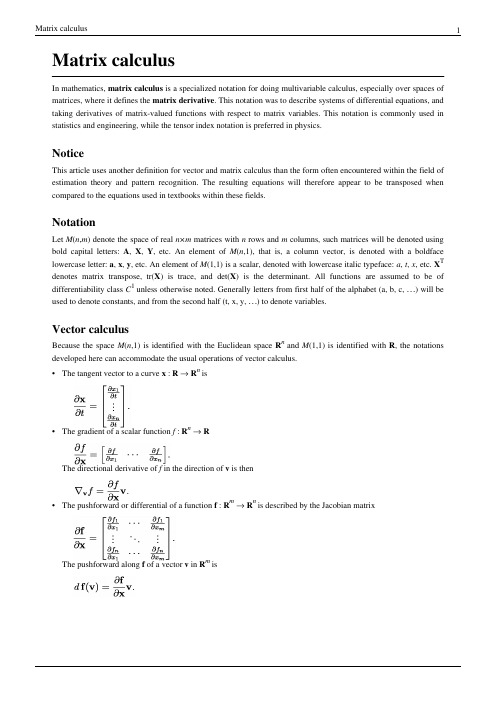
Matrix calculusIn mathematics, matrix calculus is a specialized notation for doing multivariable calculus, especially over spaces of matrices, where it defines the matrix derivative. This notation was to describe systems of differential equations, and taking derivatives of matrix-valued functions with respect to matrix variables. This notation is commonly used in statistics and engineering, while the tensor index notation is preferred in physics.NoticeThis article uses another definition for vector and matrix calculus than the form often encountered within the field of estimation theory and pattern recognition. The resulting equations will therefore appear to be transposed when compared to the equations used in textbooks within these fields.NotationLet M(n,m) denote the space of real n×m matrices with n rows and m columns, such matrices will be denoted using bold capital letters: A, X, Y, etc. An element of M(n,1), that is, a column vector, is denoted with a boldface lowercase letter: a, x, y, etc. An element of M(1,1) is a scalar, denoted with lowercase italic typeface: a, t, x, etc. X T denotes matrix transpose, tr(X) is trace, and det(X) is the determinant. All functions are assumed to be of differentiability class C1 unless otherwise noted. Generally letters from first half of the alphabet (a, b, c, …) will be used to denote constants, and from the second half (t, x, y, …) to denote variables.Vector calculusBecause the space M(n,1) is identified with the Euclidean space R n and M(1,1) is identified with R, the notations developed here can accommodate the usual operations of vector calculus.•The tangent vector to a curve x : R→ R n is•The gradient of a scalar function f : R n→ RThe directional derivative of f in the direction of v is then•The pushforward or differential of a function f : R m→ R n is described by the Jacobian matrix The pushforward along f of a vector v in R m isMatrix calculusFor the purposes of defining derivatives of simple functions, not much changes with matrix spaces; the space of n×m matrices is isomorphic to the vector space R nm. The three derivatives familiar from vector calculus have close analogues here, though beware the complications that arise in the identities below.•The tangent vector of a curve F : R→ M(n,m)•The gradient of a scalar function f : M(n,m) → RNotice that the indexing of the gradient with respect to X is transposed as compared with the indexing of X. The directional derivative of f in the direction of matrix Y is given by•The differential or the matrix derivative of a function F : M(n,m) → M(p,q) is an element of M(p,q) ⊗ M(m,n), a fourth-rank tensor (the reversal of m and n here indicates the dual space of M(n,m)). In short it is an m×n matrix each of whose entries is a p×q matrix.is a p×q matrix defined as above. Note also that this matrix has its indexing and note that each ∂F/∂Xi,jtransposed; m rows and n columns. The pushforward along F of an n×m matrix Y in M(n,m) is thenas formal block matrices.Note that this definition encompasses all of the preceding definitions as special cases.According to Jan R. Magnus and Heinz Neudecker, the following notations are both unsuitable, as the determinants of the resulting matrices would have "no interpretation" and "a useful chain rule does not exist" if these notations are being used:[1]1.2.The Jacobian matrix, according to Magnus and Neudecker,[1] isIdentitiesNote that matrix multiplication is not commutative, so in these identities, the order must not be changed.•Chain rule: If Z is a function of Y which in turn is a function of X, and these are all column vectors, then•Product rule:In all cases where the derivatives do not involve tensor products (for example, Y has more than one row and X has more than one column),ExamplesDerivative of linear functionsThis section lists some commonly used vector derivative formulas for linear equations evaluating to a vector.Derivative of quadratic functionsThis section lists some commonly used vector derivative formulas for quadratic matrix equations evaluating to a scalar.Related to this is the derivative of the Euclidean norm:Derivative of matrix tracesThis section shows examples of matrix differentiation of common trace equations.Derivative of matrix determinantRelation to other derivativesThe matrix derivative is a convenient notation for keeping track of partial derivatives for doing calculations. The Fréchet derivative is the standard way in the setting of functional analysis to take derivatives with respect to vectors. In the case that a matrix function of a matrix is Fréchet differentiable, the two derivatives will agree up to translation of notations. As is the case in general for partial derivatives, some formulae may extend under weaker analytic conditions than the existence of the derivative as approximating linear mapping.UsagesMatrix calculus is used for deriving optimal stochastic estimators, often involving the use of Lagrange multipliers. This includes the derivation of:•Kalman filter•Wiener filter•Expectation-maximization algorithm for Gaussian mixtureAlternativesThe tensor index notation with its Einstein summation convention is very similar to the matrix calculus, except one writes only a single component at a time. It has the advantage that one can easily manipulate arbitrarily high rank tensors, whereas tensors of rank higher than two are quite unwieldy with matrix notation. Note that a matrix can be considered simply a tensor of rank two.Notes[1]Magnus, Jan R.; Neudecker, Heinz (1999 (1988)). Matrix Differential Calculus. Wiley Series in Probability and Statistics (revised ed.).Wiley. pp. 171–173.External links•Matrix Calculus (/engineering/cas/courses.d/IFEM.d/IFEM.AppD.d/IFEM.AppD.pdf) appendix from Introduction to Finite Element Methods book on University of Colorado at Boulder.Uses the Hessian (transpose to Jacobian) definition of vector and matrix derivatives.•Matrix calculus (/hp/staff/dmb/matrix/calculus.html) Matrix Reference Manual , Imperial College London.•The Matrix Cookbook (), with a derivatives chapter. Uses the Hessian definition.Article Sources and Contributors5Article Sources and ContributorsMatrix calculus Source: /w/index.php?oldid=408981406 Contributors: Ahmadabdolkader, Albmont, Altenmann, Arthur Rubin, Ashigabou, AugPi, Blaisorblade,Bloodshedder, CBM, Charles Matthews, Cooli46, Cs32en, Ctacmo, DJ Clayworth, DRHagen, Dattorro, Dimarudoy, Dlohcierekim, Enisbayramoglu, Eroblar, Esoth, Excirial, Fred Bauder,Freddy2222, Gauge, Geometry guy, Giftlite, Giro720, Guohonghao, Hu12, Immunize, Jan mei118, Jitse Niesen, Lethe, Michael Hardy, MrOllie, NawlinWiki, Oli Filth, Orderud, Oussjarrouse, Ozob, Pearle, RJFJR, Rich Farmbrough, SDC, Sanchan89, Stpasha, TStein, The Thing That Should Not Be, Vgmddg, Willking1979, Xiaodi.Hou, Yuzisee, 170 anonymous editsLicenseCreative Commons Attribution-Share Alike 3.0 Unported/licenses/by-sa/3.0/。
如何选择一款合适的3D生物墨水来进行3D生物打印?

如何选择⼀款合适的3D⽣物墨⽔来进⾏3D⽣物打印?3D⽣物打印尚处于初始研发阶段,⽬前尚没有进⼊临床实验的产品。
⽣物墨⽔是3D⽣物打印过程中重要⼀环。
⽬前⽣物墨⽔体系尚没有完全建⽴,很⼤⽐例的3D⽣物打印的Paper都是关于⽣物墨⽔及其材料的研发。
国外有⼏家⽣物公司推出的⽣物墨⽔,也仅⽤于研发。
国内商⽤⽣物墨⽔仅司特易推出了Stemeasy TM蓝光固化细胞相容性⽣物墨⽔系列和StemeasyTM软⾻⽤⽣物墨⽔。
1⽣物墨⽔的基本特性1.1剪切稀化特性,使可打印;1.2粘弹性,保护细胞受剪切⼒作⽤1.3细胞相容性,负载细胞且使具有⾼的细胞活率1.4 ⽔化度,利于营养扩散1.5 凝胶动⼒学,使3D打印结构稳定等2⽣物墨⽔的分类可以从多个⾓度进⾏简单的分类2.1⽣物打印机打印⽅式配制⽣物墨⽔的材料喷墨打印低粘度能够悬浮活细胞⽣物材料;⽣物分⼦;⽣长因⼦挤压式打印⽔凝胶;细胞;蛋⽩质和陶瓷材料;从低到⾼粘度的溶液、糊剂或分散物;PLGA;磷酸三钙(TCP);胶原蛋⽩和壳聚糖;胶原-硅复合材料包覆的HA;和琼脂糖凝胶等激光辅助打印⽔凝胶,培养基,细胞,蛋⽩质和不同粘度的陶瓷材料等⽴体平⾯印刷光敏聚合物材料;固化丙烯酸树脂和环氧树脂等参考⽂献:Recent advances in bioprinting techniques: approaches, applications and future prospects 2.2墨⽔成分功能⽣物墨⽔分类描述墨⽔材料Matrix基质细胞负载的混合物细胞浆,PGD,胶原,纤维蛋⽩,明胶,重组蛛丝蛋⽩,⼈⼯基质胶,甲基丙烯酸酯硫酸软⾻素,透明质酸,海藻酸盐,葡萄聚糖,壳聚糖,吉兰糖胶,琼脂糖,K-卡拉胶,甲基纤维素等Sacrificial⽀架⽣物打印后可以移除的物质明胶,Pluronic F127琼脂糖,碳⽔化合物玻璃等Support ⽀持具有特殊机械性能的物质聚乳酸,聚⼄酸内酯,聚左旋乳酸等参考⽂献:Bioinks for biofabrication: current state and future perspectives2.3 成胶⽅式参考⽂献:Bioinks for biofabrication: current state and future perspectives2.4 按照有⽆⽀架有⽆⽀架举例Scaffold-based Bioink Materials⽔凝胶;dECM(脱细胞外基质成分);微载体Scaffold-free Bioink Materials组织球状体;细胞聚合体;组织链参考⽂献:The bioink: A comprehensive review on bioprintable materials3 商业⽣物墨⽔3.1国外商业⽣物墨⽔公司墨⽔材料特性Bioink Solutions, Inc.Gel4Cell®基于明胶紫外光光交联,细胞活率>90%Gel4Cell®-BMP结合了不同的⽣长因⼦⾻诱导Gel4Cell®-VEGF⾎管形成Gel4Cell®-TGF软管形成CELLINK CELLINK纳⽶纤维素/海藻酸钠混合物剪切稀化,快速交联,⽤于软⾻组织⼯程RegenHU BioInk®基于PEG/明胶/透明质酸良好的细胞粘附性能,可⽣物降解,模仿天然ECM Osteoink™磷酸钙糊剂与⼈⾻相似的成份,利于⾻诱导形成,⽤于硬组织⼯程Biobot Bio127基于Pluronic F127BioGel基于MA化明胶结合引发剂,蓝光引发交联参考⽂献:Bioink properties before, during and after 3D bioprinting3.2国内商业⽣物墨⽔公司名系列墨⽔应⽤特点司特易 StemeasyTM蓝光固化细胞相容性⽣物墨⽔甲基丙烯酸酯化明胶GelMA⾻,软⾻,⼼脏和⾎管等GelMA⽔凝胶与天然细胞外基质(ECM)的基本性质⾮常相似,利于细胞粘附和增殖。
波束 矩阵 matlab
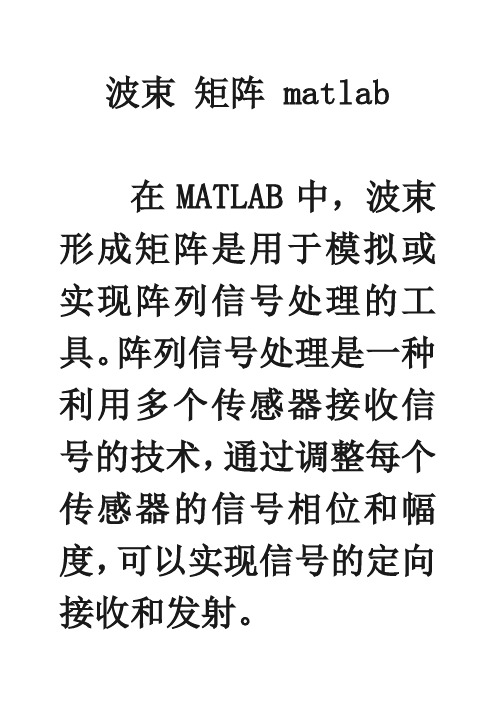
波束矩阵 matlab
在MATLAB中,波束形成矩阵是用于模拟或实现阵列信号处理的工具。
阵列信号处理是一种利用多个传感器接收信号的技术,通过调整每个传感器的信号相位和幅度,可以实现信号的定向接收和发射。
波束形成矩阵通常是一个复数矩阵,其大小取决于阵列的传感器数量和阵列的几何结构。
在MATLAB中,可以使用内置函数来创建波束形成矩阵。
例如,beamform 函数可以根据指定的阵列几何结构和波束形成算法生成波束形成矩阵。
使用波束形成矩阵可以实现多种阵列信号处理任务,如波束形成、信号分离、干扰抑制等。
通过调整波束形成矩阵的元素,可以改变接收或发射信号的方向和形状,从而实现信号的定向控制和优化。
波束形成矩阵是阵列信号处理中不可或缺
的工具,通过使用MATLAB等软件,可以方便地创建和应用波束形成矩阵,从而实现高效的信号处理和通信系统设计。
- 1、下载文档前请自行甄别文档内容的完整性,平台不提供额外的编辑、内容补充、找答案等附加服务。
- 2、"仅部分预览"的文档,不可在线预览部分如存在完整性等问题,可反馈申请退款(可完整预览的文档不适用该条件!)。
- 3、如文档侵犯您的权益,请联系客服反馈,我们会尽快为您处理(人工客服工作时间:9:00-18:30)。
Matrix calculusIn mathematics, matrix calculus is a specialized notation for doing multivariable calculus, especially over spaces of matrices, where it defines the matrix derivative. This notation was to describe systems of differential equations, and taking derivatives of matrix-valued functions with respect to matrix variables. This notation is commonly used in statistics and engineering, while the tensor index notation is preferred in physics.NoticeThis article uses another definition for vector and matrix calculus than the form often encountered within the field of estimation theory and pattern recognition. The resulting equations will therefore appear to be transposed when compared to the equations used in textbooks within these fields.NotationLet M(n,m) denote the space of real n×m matrices with n rows and m columns, such matrices will be denoted using bold capital letters: A, X, Y, etc. An element of M(n,1), that is, a column vector, is denoted with a boldface lowercase letter: a, x, y, etc. An element of M(1,1) is a scalar, denoted with lowercase italic typeface: a, t, x, etc. X T denotes matrix transpose, tr(X) is trace, and det(X) is the determinant. All functions are assumed to be of differentiability class C1 unless otherwise noted. Generally letters from first half of the alphabet (a, b, c, …) will be used to denote constants, and from the second half (t, x, y, …) to denote variables.Vector calculusBecause the space M(n,1) is identified with the Euclidean space R n and M(1,1) is identified with R, the notations developed here can accommodate the usual operations of vector calculus.•The tangent vector to a curve x : R→ R n is•The gradient of a scalar function f : R n→ RThe directional derivative of f in the direction of v is then•The pushforward or differential of a function f : R m→ R n is described by the Jacobian matrix The pushforward along f of a vector v in R m isMatrix calculusFor the purposes of defining derivatives of simple functions, not much changes with matrix spaces; the space of n×m matrices is isomorphic to the vector space R nm. The three derivatives familiar from vector calculus have close analogues here, though beware the complications that arise in the identities below.•The tangent vector of a curve F : R→ M(n,m)•The gradient of a scalar function f : M(n,m) → RNotice that the indexing of the gradient with respect to X is transposed as compared with the indexing of X. The directional derivative of f in the direction of matrix Y is given by•The differential or the matrix derivative of a function F : M(n,m) → M(p,q) is an element of M(p,q) ⊗ M(m,n), a fourth-rank tensor (the reversal of m and n here indicates the dual space of M(n,m)). In short it is an m×n matrix each of whose entries is a p×q matrix.is a p×q matrix defined as above. Note also that this matrix has its indexing and note that each ∂F/∂Xi,jtransposed; m rows and n columns. The pushforward along F of an n×m matrix Y in M(n,m) is thenas formal block matrices.Note that this definition encompasses all of the preceding definitions as special cases.According to Jan R. Magnus and Heinz Neudecker, the following notations are both unsuitable, as the determinants of the resulting matrices would have "no interpretation" and "a useful chain rule does not exist" if these notations are being used:[1]1.2.The Jacobian matrix, according to Magnus and Neudecker,[1] isIdentitiesNote that matrix multiplication is not commutative, so in these identities, the order must not be changed.•Chain rule: If Z is a function of Y which in turn is a function of X, and these are all column vectors, then•Product rule:In all cases where the derivatives do not involve tensor products (for example, Y has more than one row and X has more than one column),ExamplesDerivative of linear functionsThis section lists some commonly used vector derivative formulas for linear equations evaluating to a vector.Derivative of quadratic functionsThis section lists some commonly used vector derivative formulas for quadratic matrix equations evaluating to a scalar.Related to this is the derivative of the Euclidean norm:Derivative of matrix tracesThis section shows examples of matrix differentiation of common trace equations.Derivative of matrix determinantRelation to other derivativesThe matrix derivative is a convenient notation for keeping track of partial derivatives for doing calculations. The Fréchet derivative is the standard way in the setting of functional analysis to take derivatives with respect to vectors. In the case that a matrix function of a matrix is Fréchet differentiable, the two derivatives will agree up to translation of notations. As is the case in general for partial derivatives, some formulae may extend under weaker analytic conditions than the existence of the derivative as approximating linear mapping.UsagesMatrix calculus is used for deriving optimal stochastic estimators, often involving the use of Lagrange multipliers. This includes the derivation of:•Kalman filter•Wiener filter•Expectation-maximization algorithm for Gaussian mixtureAlternativesThe tensor index notation with its Einstein summation convention is very similar to the matrix calculus, except one writes only a single component at a time. It has the advantage that one can easily manipulate arbitrarily high rank tensors, whereas tensors of rank higher than two are quite unwieldy with matrix notation. Note that a matrix can be considered simply a tensor of rank two.Notes[1]Magnus, Jan R.; Neudecker, Heinz (1999 (1988)). Matrix Differential Calculus. Wiley Series in Probability and Statistics (revised ed.).Wiley. pp. 171–173.External links•Matrix Calculus (/engineering/cas/courses.d/IFEM.d/IFEM.AppD.d/IFEM.AppD.pdf) appendix from Introduction to Finite Element Methods book on University of Colorado at Boulder.Uses the Hessian (transpose to Jacobian) definition of vector and matrix derivatives.•Matrix calculus (/hp/staff/dmb/matrix/calculus.html) Matrix Reference Manual , Imperial College London.•The Matrix Cookbook (), with a derivatives chapter. Uses the Hessian definition.Article Sources and Contributors5Article Sources and ContributorsMatrix calculus Source: /w/index.php?oldid=408981406 Contributors: Ahmadabdolkader, Albmont, Altenmann, Arthur Rubin, Ashigabou, AugPi, Blaisorblade,Bloodshedder, CBM, Charles Matthews, Cooli46, Cs32en, Ctacmo, DJ Clayworth, DRHagen, Dattorro, Dimarudoy, Dlohcierekim, Enisbayramoglu, Eroblar, Esoth, Excirial, Fred Bauder,Freddy2222, Gauge, Geometry guy, Giftlite, Giro720, Guohonghao, Hu12, Immunize, Jan mei118, Jitse Niesen, Lethe, Michael Hardy, MrOllie, NawlinWiki, Oli Filth, Orderud, Oussjarrouse, Ozob, Pearle, RJFJR, Rich Farmbrough, SDC, Sanchan89, Stpasha, TStein, The Thing That Should Not Be, Vgmddg, Willking1979, Xiaodi.Hou, Yuzisee, 170 anonymous editsLicenseCreative Commons Attribution-Share Alike 3.0 Unported/licenses/by-sa/3.0/。