A distributed underlay channel assignment for cognitive cooperative ad hoc networks based on interfe
LTE_3GPP_36.213-860(中文版)
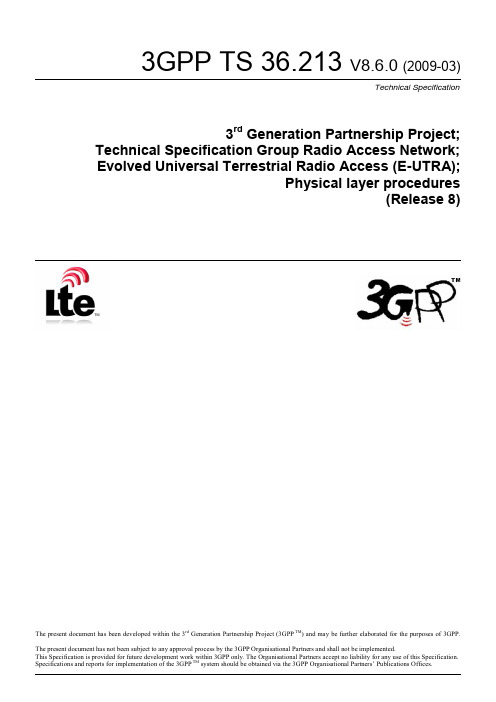
3GPP
Release 8
3
3GPP TS 36.213 V8.6.0 (2009-03)
Contents
Foreword ...................................................................................................................................................... 5 1 2 3
Internet
Copyright Notification No part may be reproduced except as authorized by written permission. The copyright and the foregoing restriction extend to reproduction in all media.
© 2009, 3GPP Organizational Partners (ARIB, ATIS, CCSA, ETSI, TTA, TTC). All rights reserved. UMTS™ is a Trade Mark of ETSI registered for the benefit of its members 3GPP™ is a Trade Mark of ETSI registered for the benefit of its Members and of the 3GPP Organizational Partners LTE™ is a Trade Mark of ETSI currently being registered for the benefit of i ts Members and of the 3GPP Organizational Partners GSM® and the GSM logo are registered and owned by the GSM Association
音频系统专业名词解释和英汉对照
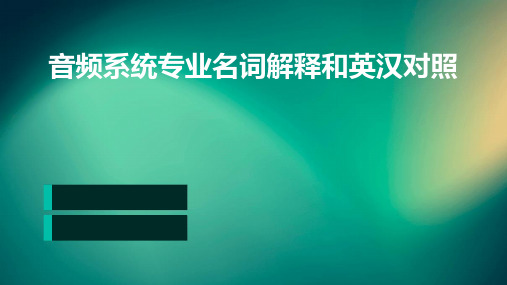
24位:24位
02 音频处理技术
均衡器
总结词
均衡器是一种音频处理设备,用于调整音频信号的频率特性,以改善音质或满足特定听觉需求。
详细描述
均衡器通过调整不同频段的增益来改变音频信号的频谱分布,从而实现对音色的调整和优化。在录音 、混音和母带处理等过程中,均衡器被广泛应用于调整音频信号的平衡和和谐度,以达到更好的听觉 效果。
总结词
混响是一种模拟声音在空间中传播的自然现 象的效果,通常用于创造空间感。
详细描述
混响是模拟声音在封闭空间中传播的自然现 象的效果,例如在房间、大厅或教堂中。通 过模拟不同环境下的声音反射和散射,混响 效果可以创造出空间感和环境感,使声音更 加自然和逼真。混响效果广泛应用于录音、
混音和扩声等领域。
指游戏中的音频处理软件,用于实时处理游戏中的声音效果和 语音聊天等。
05 音频系统专业词汇英汉对 照
Audio (音频)
总结词
音频通常是指模拟或数字信号的声波,用于记录、传输和重放声音。
详细描述
音频是声音的电信号表示,可以模拟或数字形式存在。模拟音频信号是连续变化的电压或电流,而数字音频则是 离散的样本值。音频广泛应用于录音、广播、电视、电影、音乐制作等领域。
05
04
音效设计
指根据电影情节和场景,设计出相应 的声音效果,以增强电影的表现力。
游戏音效
游戏音效 游戏音效设计
环绕声 声音引擎
指在游戏制作过程中,使用音频设备和技术,为游戏制作逼真 的声音效果,增强游戏的沉浸感和体验感。
指根据游戏情节和场景,设计出相应的声音效果,以增强游戏 的沉浸感。
指使用多个扬声器,模拟出三维空间的声音效果,使玩家能够 感受到声音的来源方向和距离。
Autodesk Nastran 2023 参考手册说明书
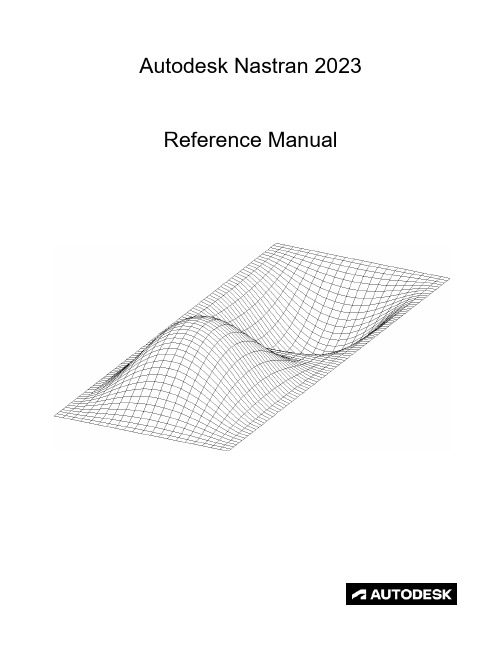
FILESPEC ............................................................................................................................................................ 13
DISPFILE ............................................................................................................................................................. 11
File Management Directives – Output File Specifications: .............................................................................. 5
BULKDATAFILE .................................................................................................................................................... 7
分组语音流的马尔可夫调制泊松模型
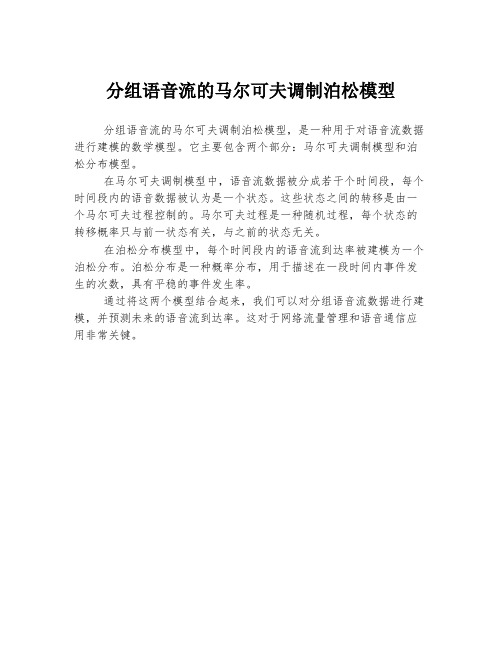
分组语音流的马尔可夫调制泊松模型
分组语音流的马尔可夫调制泊松模型,是一种用于对语音流数据进行建模的数学模型。
它主要包含两个部分:马尔可夫调制模型和泊松分布模型。
在马尔可夫调制模型中,语音流数据被分成若干个时间段,每个时间段内的语音数据被认为是一个状态。
这些状态之间的转移是由一个马尔可夫过程控制的。
马尔可夫过程是一种随机过程,每个状态的转移概率只与前一状态有关,与之前的状态无关。
在泊松分布模型中,每个时间段内的语音流到达率被建模为一个泊松分布。
泊松分布是一种概率分布,用于描述在一段时间内事件发生的次数,具有平稳的事件发生率。
通过将这两个模型结合起来,我们可以对分组语音流数据进行建模,并预测未来的语音流到达率。
这对于网络流量管理和语音通信应用非常关键。
北京理工大学5G移动通信技术期末试题
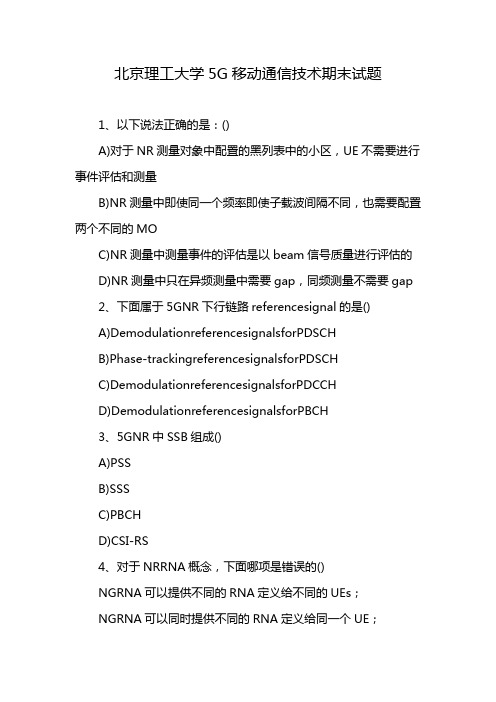
北京理工大学5G移动通信技术期末试题1、以下说法正确的是:()A)对于NR测量对象中配置的黑列表中的小区,UE不需要进行事件评估和测量B)NR测量中即使同一个频率即使子载波间隔不同,也需要配置两个不同的MOC)NR测量中测量事件的评估是以beam信号质量进行评估的D)NR测量中只在异频测量中需要gap,同频测量不需要gap2、下面属于5GNR下行链路referencesignal的是()A)DemodulationreferencesignalsforPDSCHB)Phase-trackingreferencesignalsforPDSCHC)DemodulationreferencesignalsforPDCCHD)DemodulationreferencesignalsforPBCH3、5GNR中SSB组成()A)PSSB)SSSC)PBCHD)CSI-RS4、对于NRRNA概念,下面哪项是错误的()NGRNA可以提供不同的RNA定义给不同的UEs;NGRNA可以同时提供不同的RNA定义给同一个UE;UE需要支持listofcells和listofRANareas2种RNA配置;UE不需要2种RNA配置listofcells和listofRANareas都支持;5、下面哪些RB可以配置不同的PDCPVersion()A)SRB1B)SRB2C)MNterminatedMCGBearerD)SNterminatedMCGBearer6、下面关于BWP的描述正确的是()A)BWP可以支持更低的UE带宽能力B)BWP可以支持不同的numerologyC)BWP的灵活调度可以大大降低功耗D)在某一个时刻,最多只能有一个激活的DLBWP和ULBWP7、BWP切换有哪些方式()A)TCI B)DCI C)Timer D)RMSI8、MCGfailure的触发原因有哪几种()A)uponT310expiryB)uponT312expiryC)uponrandomaccessproblemindicationfromMCGMACwh ileneither,T304norT311isrunningD)uponindicationfromMCGRLCthatthemaximumnumbero fretransmissionshasbeenreachedforanSRBorDRB9、5GNR中有哪几种Raster()A)GlobalRasterB)ChannelRasterC)SSRaster10、NR测量根据RS接收信号的类型可以分为:()A)基于SSB的测量B)基于CSI的测量C)基于CSl-RS的测量D)基于PSS的测量11、5GNRSCS可以是0A)15kHz,30kHz,60kHzB)120kHz,240kHzC)480kHzD)960kHz12、关于dualConnectivityPHR以下说法正确的是()A)LTEDC下两个CG都会配置B)LTEDC下只配置MCG的相关参数C)EN-DC下两个CG都会配置PHR相关参数D)EN-DC下只配置MCG的相关参数。
llm大语言模型量化方法
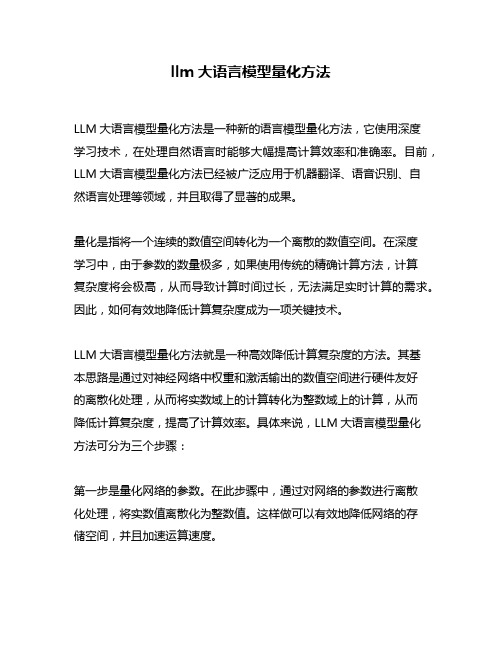
llm大语言模型量化方法LLM大语言模型量化方法是一种新的语言模型量化方法,它使用深度学习技术,在处理自然语言时能够大幅提高计算效率和准确率。
目前,LLM大语言模型量化方法已经被广泛应用于机器翻译、语音识别、自然语言处理等领域,并且取得了显著的成果。
量化是指将一个连续的数值空间转化为一个离散的数值空间。
在深度学习中,由于参数的数量极多,如果使用传统的精确计算方法,计算复杂度将会极高,从而导致计算时间过长,无法满足实时计算的需求。
因此,如何有效地降低计算复杂度成为一项关键技术。
LLM大语言模型量化方法就是一种高效降低计算复杂度的方法。
其基本思路是通过对神经网络中权重和激活输出的数值空间进行硬件友好的离散化处理,从而将实数域上的计算转化为整数域上的计算,从而降低计算复杂度,提高了计算效率。
具体来说,LLM大语言模型量化方法可分为三个步骤:第一步是量化网络的参数。
在此步骤中,通过对网络的参数进行离散化处理,将实数值离散化为整数值。
这样做可以有效地降低网络的存储空间,并且加速运算速度。
第二步是量化网络的输出。
在此步骤中,对网络的输出进行离散化处理,将实数域的输出转化为整数域的输出。
这样做可以大幅降低计算复杂度,提高运算速度,并且保持了原始网络的输出准确度。
第三步是训练量化网络。
在此步骤中,通过对离散化嵌入层的训练,可以提高网络的分辨率和准确性,使其更好地适应任务的要求。
同时,网络中的量化参数也可以被训练,以提高网络的分类精度。
总之,LLM大语言模型量化方法是一种高效的深度学习方法,其优点在于可以大幅降低计算复杂度,提高计算速度,并且保持了原始网络的输出准确度。
所以,LLM大语言模型量化方法在深度学习领域中有着广泛的应用前景,将为我们的生活带来更多的便利和创新。
多重信号分类music算法

多重信号分类music算法多重信号分类music算法__________________________________多重信号分类MUSIC算法是一种常见的无参数估计技术,它可以在无需任何先验信息的情况下估计信号的参数,并以最优的方式将信号分类为相应的模型。
MUSIC算法是一种多重信号分类技术,它可以根据接收信号的信息特征来判断信号的模型,并以此来分类信号。
MUSIC算法的优势在于它不需要设计复杂的估计器,而且可以有效地处理维度灾难。
MUSIC算法是一种基于统计学的方法,它通过采用相关函数和谱估计来估计信号的参数。
MUSIC 算法是一种多信号分类技术,它主要用于分类复杂信号,如高斯噪声、信息信号、脉冲噪声等。
MUSIC算法可以有效地估计信号的特征参数,并以此来进行分类。
MUSIC算法的基本思想是通过估计接收端所收到的信号的相关函数,并将其分解为各个信号成分,然后使用正交函数将各个信号成分进行正交化。
之后,使用相关函数的特征值来估计各个信号成分的功率,并使用此功率来对信号进行分类。
MUSIC算法的基本流程是首先采样接收端所收到的信号,然后使用相关函数来估计接收端所收到的信号,并将其分解为各个信号成分,然后使用正交函数将各个信号成分进行正交化,再使用相关函数的特征值来估计各个信号成分的功率,最后使用此功率来对信号进行分类。
MUSIC算法在多重信号分类方面有很大的优势,它能够有效地估计信号的特征参数,并以此来进行分类。
它还能够有效地处理高斯噪声、脉冲噪声、随机信息信号等复杂信号。
此外,MUSIC算法不需要任何先验信息,也不需要设计复杂的估计器,这使得它能够快速地进行多重信号分类。
MUSIC算法在实际应用中也有很大的价值,它能够有效地帮助用户进行多重信号分类,从而使用户能够快速地实施不同的应用。
例如,在无人驾驶中,MUSIC算法可以帮助用户快速地识别不同的物体;在医学图像诊断中,MUSIC算法也能够有效地帮助医生诊断不同的疾病。
cd模型的工作原理

cd模型的工作原理
CD模型,即Contrastive Divergence模型,是一种基于马尔可
夫链Monte Carlo Markov Chains(MCMC)的无监督学习算法,通常用于训练受限玻尔兹曼机(Restricted Boltzmann Machine,RBM)或深度信念网络(Deep Belief Networks,DBN)。
CD模型的工作原理如下:
1. 初始化模型参数:首先,初始化RBM的可见层和隐层的权
重矩阵,以及偏置项。
偏置项用于定义每个神经元被激活的基础概率。
2. 正向传播:通过RBM的可见层和隐层之间的权重矩阵,将
输入样本的特征映射到隐层,计算出隐层的激活值。
3. 反向传播:将隐层的激活值再次传递回可见层,通过可见层和隐层之间的权重矩阵,计算重构的可见层。
4. 通过Gibbs采样计算对比分歧:在每一轮迭代中,利用当前
的可见层和隐层状态,通过互相传递信息的方式来更新模型的参数。
5. 权重更新:通过对比重构的可见层和原始的可见层的误差,计算出梯度,然后使用梯度下降算法或其他优化算法来更新模型的权重参数和偏置项。
6. 重复迭代:重复执行2-5步骤,迭代更新模型的参数,直到
达到预定的迭代次数或收敛条件。
通过这种方式,CD模型通过学习数据的分布特征,能够对输入数据进行无监督的特征学习和表示学习,进而可以用于生成样本和进行概率推断等任务。
- 1、下载文档前请自行甄别文档内容的完整性,平台不提供额外的编辑、内容补充、找答案等附加服务。
- 2、"仅部分预览"的文档,不可在线预览部分如存在完整性等问题,可反馈申请退款(可完整预览的文档不适用该条件!)。
- 3、如文档侵犯您的权益,请联系客服反馈,我们会尽快为您处理(人工客服工作时间:9:00-18:30)。
Shadi Ebrahimi Asl
Petropars Ltd. School of Electrical Engineering, Iran University of Science and Technology Tehran, Iran
where IT(fC,BC) is the IT in a channel with central frequency fC and bandwidth BC, P(fC,BC) is the average power in watts on the channel, S(fC,BC) is the power spectral density of P(fC,BC) and k is Boltzmann's constant ( 1.38 × 10 −23 Joules per Kelvin). Another problem for channel assignment in cognitive underlay transmission is achieving a good secondary capacity when high power primary signal is available in the host channel. Since cognitive users are generally low power radios in comparison with PUs, they receive the primary signal as high power interference. However, in this condition no modification is permitted to be exerted on the primary system. Thus, cognitive users must suppress the high power interference from the primary base station (PBS) to improve their achieved capacities. In [6][14], using adaptive array by secondary user has been proposed to reduce the interference between primary and secondary networks. Ref. [7] and [8] study performance of cognitive links with the assumption that a cognitive radio respectively knows or estimates the primary signal to subtract it from the total received signal. In this paper, we develop a single user and a multi user version of a Distributed Underlay Channel Assignment (DUCHA) algorithm in the downlink channel of a primary cell for a cognitive cooperative ad hoc network overlaid with a primary cell. We assume there is a mobile PU in the primary cell that transmits signal to the PBS on an uplink channel. In our proposed channel assignment scenario all cognitive channel applicants (CCHAs)
1 P ( f C , BC ) BC = IT ( f C , BC ) = kBC
∫ S( f
BC
C
, BC )df
kBC
,
(1)
Categories and Subject Descriptors
C.2.1 [COMPUTER-COMMUNICATION Wireless communication. NETWORKS]:
General Terms
Algorithms.
Keywords
Cognitive radio, Underlay transmission, Direct sequence spread spectrum, Jammer suppression.
1. INTRODUCTION
Cognitive radio has been introduced as a secondary user in a licensed host channel such that it produces no harmful interference to the primary user (PU) of that channel [1]. Accordingly, if a group of cognitive radios aim to use a common host channel they must use a channel assignment algorithm
Permission to make digital or hard copies of all or part of this work for personal or classroom use is granted without fee provided that copies are not made or distributed for profit or commercial advantage and that copies bear this notice and the full citation on the first page. To copy otherwise, or republish, to post on servers or to redistribute to lists, requires prior specific permission and/or a fee. MobiWac’09, October 26–27, 2009, Tenerife, Canary Islands, Spain. Copyright 2009 ACM 978-1-60558-617-5/09/10...$10.00.
Bahman AbolhasFra bibliotekaniSchool of Electrical Engineering, Iran University of Science and Technology, Tehran, Iran
shadi_ebrahimiasl@ee.iust.ac.ir ABSTRACT
In this paper, we develop a single user and a multi user version of a Distributed Underlay Channel Assignment (DUCHA) algorithm for spectrum access to a downlink channel of a primary cell for a cognitive cooperative ad hoc network overlaid with the cell. We use direct sequence spread spectrum as a medium interface for cognitive links. We investigate performance of DUCHA algorithm in three spreading scenarios with regard to the bandwidth of the channel. Simulation results show that when there are 100 cognitive channel applicants and spreading factor is 100 the multi user DUCHA algorithm assigns the channel to an average 51.8 percent of channel applicants and in this case it achieves 22.57 bps/Hz spectral efficiency under high power interference from the primary base station of the cell. We show that in this condition the increased in-band and out of band interference temperatures are non-harmful and have values of respectively 22.48 dB and -0.99 dB.
99
that are interested in using the downlink channel first share their measured data in a common control channel. Then, each CCHA independently executes the proposed DUCHA algorithm to find the best set of qualified CCHAs for secondary transmissions. Two main criteria in DUCHA algorithm for selecting a CCHA for secondary transmission are: locating out of no-talk zone1 of the PU and producing an in-band IT which does not increase the total IT at the PU above a determined harmful level. In order to overcome the high power interference from the PBS to cognitive links we consider the signal from the PBS as a destructive noise jammer. We use direct sequence spread spectrum (DSSS) as a medium interface in cognitive links such that cognitive receivers are able to suppress this jamming signal by using suitable spreading factors. We investigate performance of the proposed DUCHA algorithm in three spreading scenarios. We show that in our proposed transmission scenario the proposed multi user DUCHA algorithm assigns the downlink channel of the primary system to 51.8 percent of CCHAs when there are 100 CCHAs in the cognitive network and spreading factor is 100 and also in this condition it achieves 22.57 bps/Hz spectral efficiency under high power interference from the PBS of the host channel. We show that in this condition the increased in-band and out of band IT are non-harmful and have values of respectively 22.48 dB and -0.99 dB. The rest of this paper is organized as follows. In Section II, we introduced our proposed Distributed Underlay Channel Assignment (DUCHA) algorithm. In Section III, we investigate performance of the proposed DUCHA algorithm in three spreading scenarios through computer simulations. Conclusions of this work are presented in Section IV. We use term secondary transmission and cognitive transmission interchangeably throughout this paper.