Applications of Ontologies in Software Engineering
微软无线光学桌面4000系列说明书
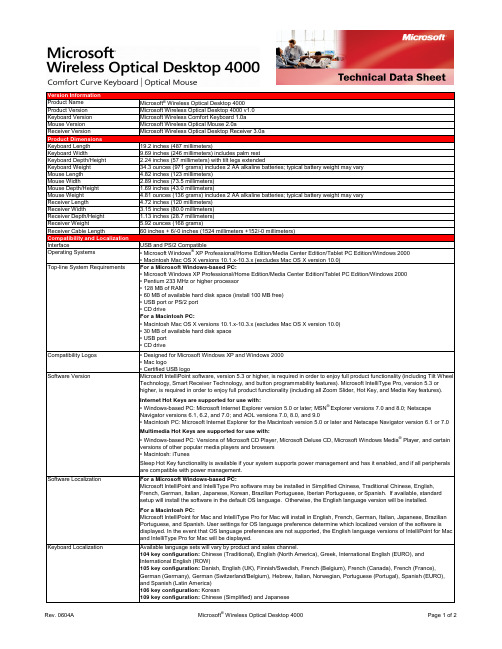
Version InformationProduct Name Microsoft® Wireless Optical Desktop 4000Product Version Microsoft Wireless Optical Desktop 4000 v1.0Keyboard Version Microsoft Wireless Comfort Keyboard 1.0aMouse Version Microsoft Wireless Optical Mouse 2.0aReceiver Version Microsoft Wireless Optical Desktop Receiver 3.0aProduct DimensionsKeyboard Length19.2 inches (487 millimeters)Keyboard Width9.69 inches (246 millimeters) includes palm restKeyboard Depth/Height 2.24 inches (57 millimeters) with tilt legs extendedKeyboard Weight34.3 ounces (971 grams) includes 2 AA alkaline batteries; typical battery weight may varyMouse Length 4.82 inches (123 millimeters)Mouse Width 2.89 inches (73.5 millimeters)Mouse Depth/Height 1.69 inches (43.0 millimeters)Mouse Weight 4.81 ounces (136 grams) includes 2 AA alkaline batteries; typical battery weight may varyReceiver Length 4.72 inches (120 millimeters)Receiver Width 3.15 inches (80.0 millimeters)Receiver Depth/Height 1.13 inches (28.7 millimeters)Receiver Weight 5.92 ounces (168 grams)Receiver Cable Length60 inches + 6/-0 inches (1524 millimeters +152/-0 millimeters)Compatibility and LocalizationInterface USB and PS/2 CompatibleOperating Systems• Microsoft Windows® XP Professional/Home Edition/Media Center Edition/Tablet PC Edition/Windows 2000• Macintosh Mac OS X versions 10.1.x-10.3.x (excludes Mac OS X version 10.0)Top-line System Requirements For a Microsoft Windows-based PC:• Microsoft Windows XP Professional/Home Edition/Media Center Edition/Tablet PC Edition/Windows 2000• Pentium 233 MHz or higher processor• 128 MB of RAM• 60 MB of available hard disk space (install 100 MB free)• USB port or PS/2 port• CD driveFor a Macintosh PC:• Macintosh Mac OS X versions 10.1.x-10.3.x (excludes Mac OS X version 10.0)• 30 MB of available hard disk space• USB port• CD driveCompatibility Logos• Designed for Microsoft Windows XP and Windows 2000• Mac logo• Certified USB logoSoftware Version Microsoft IntelliPoint software, version 5.3 or higher, is required in order to enjoy full product functionality (including Tilt Wheel Technology, Smart Receiver Technology, and button programmability features). Microsoft IntelliType Pro, version 5.3 orhigher, is required in order to enjoy full product functionality (including all Zoom Slider, Hot Key, and Media Key features).Internet Hot Keys are supported for use with:• Windows-based PC: Microsoft Internet Explorer version 5.0 or later; MSN® Explorer versions 7.0 and 8.0; NetscapeNavigator versions 6.1, 6.2, and 7.0; and AOL versions 7.0, 8.0, and 9.0• Macintosh PC: Microsoft Internet Explorer for the Macintosh version 5.0 or later and Netscape Navigator version 6.1 or 7.0Multimedia Hot Keys are supported for use with:• Windows-based PC: Versions of Microsoft CD Player, Microsoft Deluxe CD, Microsoft Windows Media® Player, and certainversions of other popular media players and browsers• Macintosh: iTunesSleep Hot Key functionality is available if your system supports power management and has it enabled, and if all peripheralsare compatible with power management.Software Localization For a Microsoft Windows-based PC:Microsoft IntelliPoint and IntelliType Pro software may be installed in Simplified Chinese, Traditional Chinese, English,French, German, Italian, Japanese, Korean, Brazilian Portuguese, Iberian Portuguese, or Spanish. If available, standardsetup will install the software in the default OS language. Otherwise, the English language version will be installed.For a Macintosh PC:Microsoft IntelliPoint for Mac and IntelliType Pro for Mac will install in English, French, German, Italian, Japanese, BrazilianPortuguese, and Spanish. User settings for OS language preference determine which localized version of the software isdisplayed. In the event that OS language preferences are not supported, the English language versions of IntelliPoint for Macand IntelliType Pro for Mac will be displayed.Keyboard Localization Available language sets will vary by product and sales channel.104 key configuration: Chinese (Traditional), English (North America), Greek, International English (EURO), andInternational English (ROW)105 key configuration: Danish, English (UK), Finnish/Swedish, French (Belgium), French (Canada), French (France),German (Germany), German (Switzerland/Belgium), Hebrew, Italian, Norwegian, Portuguese (Portugal), Spanish (EURO),and Spanish (Latin America)106 key configuration: Korean109 key configuration: Chinese (Simplified)and JapaneseTracking TechnologyMouse Tracking System Microsoft-proprietary optical technologyImaging Rate Dynamically adaptable to 6000 frames per secondX-Y Resolution400 points per inch (15.75 points per millimeter)Tracking Speed Up to 36 inches (914 millimeters) per secondWireless TechnologyWireless Platform27 MHz Radio Frequency (RF)Wireless Channels• Keyboard: 27.095 MHz channel 0, 27.195 MHz channel 1• Mouse: 27.145 MHz Mouse channel 1Wireless IDs• Keyboard: Over 65,000 random identification codes• Mouse: Over 65,000 random identification codesWireless Range 6 feet (1.83 meters) typical. Note: RF range is affected by many factors, such as nearby metallic objects and relativepositioning of the keyboard, mouse, and receiver.Product Feature PerformanceQWERTY Key Life1,000,000 actuations per keyZoom Slider Life250,000 actuations in either directionHot Key Features Web/Home, Calendar, Mail, Messenger, My Documents, Show My Favorites, My Favorites 1, My Favorites 2, My Favorites 3, My Favorites 4, My Favorites 5, Calculator, Log Off, and SleepHot Key Life500,000 actuations per keyMedia Key Features Mute, Volume -, Volume +, Play/Pause, Stop (Media), Previous Track, and Next TrackMedia Key Life500,000 actuations per keyEnhanced Function Key Features Help, Undo, Redo, New, Open, Close, Reply, Forward, Send, Spell, Save, and PrintTyping Speed1000 characters per minuteMouse Button Features 3 buttons including scroll wheel buttonRight & Left Button Life1,000,000 actuations at no more than 4 actuations per secondWheel Button Life150,000 actuations at no more than 4 actuations per secondMouse Scrolling Features Tilt wheel enables vertical and horizontal scrollingWheel Vertical Scrolling Life• 100,000 revolutions (away from user)• 100,000 revolutions (towards user)Wheel Horizontal Scrolling Life350,000 actuations per side at no more than 4 actuations per secondStorage Temperature & Humidity-40 °F (-40 °C) to 140 °F (60 °C) at < 5% to 65% relative humidity (non-condensing)Operating Temperature & Humidity14 °F (-10 °C) to 104 °F (40 °C) at <5% to 80% relative humidity (non-condensing)Power RequirementsBattery Type and Quantity• Keyboard: 2 AA alkaline batteries (included)• Mouse: 2 AA alkaline batteries (included)Battery Life• Keyboard: 6 months typical• Mouse: 6 months typicalCertification InformationCountry of Manufacture Keyboard and Receiver: Thailand and Mouse: People's Republic of China (PRC)ISO 9002 Qualified Manufacturer YesFCC ID This device complies with part 15 of the FCC Rules and Industry Canada RSS-210. Operation is subject to the following two conditions: (1) This device may not cause harmful interference, and (2) this device must accept any interference received,including interference that may cause undesired operation. Tested to comply with FCC standards. For home and office use.Model numbers: 1027, Wireless Optical Comfort Keyboard 1.0; 1008, Wireless Optical Mouse 2.0; and 1029, WirelessOptical Desktop Receiver 3.0. FCC IDs: C3K1027 and C3K1008.Agency and Regulatory Approvals• FCC Declaration of Conformity (USA)• UL and cUL Listed Accessory (USA and Canada)• RSS-210 and ICES-003 data on file (Canada)• TUV-T Certificate (European Union)• R&TTE Declaration of Conformity, Safety and EMC (European Union)• GOST Certificate (Russia)• VCCI Certificate (Japan)• ACA/MED Declaration of Conformity (Australia and New Zealand)• BSMI and DGT Certificates (Taiwan)• MIC Certificate (Korea)• CMII Certificate (China)• NOM Certificates (Mexico)• SABS Certificate (South Africa)• CB Scheme Certificate (International)• WHQL (International) ID: 890572Results stated herein are based on internal Microsoft testing. Individual results and performance may vary. Any device images shown are not actual size. This document is provided for informational purposes only and is subject to change without notice. Microsoft makes no warranty, express or implied, with this document or the information contained herein. Review any public use or publications of any data herein with your local legal counsel.©2006 Microsoft Corporation. All rights reserved. Microsoft, the IntelliEye logo, IntelliMouse, MSN, the Laser Technology logo, the Optical Technology logo, Natural, Windows, and Windows NT are either registered trademarks or trademarks of Microsoft Corporation in the U.S. and/or other countries. Mac and the Mac logo are trademarks of Apple Computer, Inc., registered in the U.S. and/or other countries. The names of actual companies and products mentioned herein may be trademarks of their respective owners.。
技术栈(technologystack)
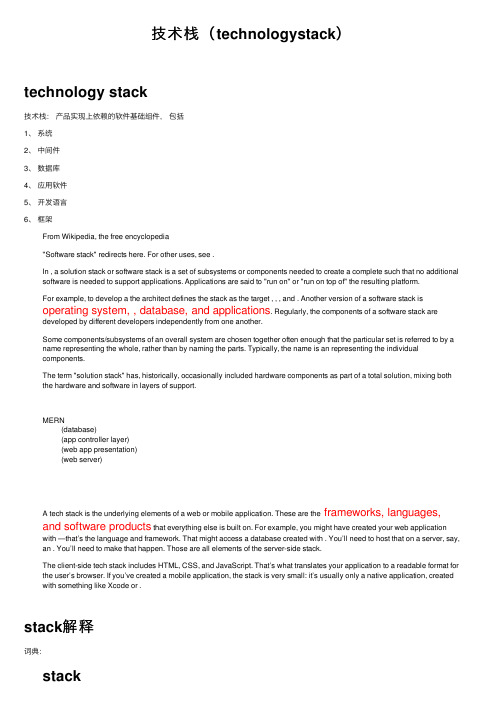
技术栈(technologystack)technology stack技术栈:产品实现上依赖的软件基础组件,包括1、系统2、中间件3、数据库4、应⽤软件5、开发语⾔6、框架From Wikipedia, the free encyclopedia"Software stack" redirects here. For other uses, see .In , a solution stack or software stack is a set of subsystems or components needed to create a complete such that no additional software is needed to support applications. Applications are said to "run on" or "run on top of" the resulting platform.For example, to develop a the architect defines the stack as the target , , , and . Another version of a software stack isoperating system, , database, and applications. Regularly, the components of a software stack are developed by different developers independently from one another.Some components/subsystems of an overall system are chosen together often enough that the particular set is referred to by a name representing the whole, rather than by naming the parts. Typically, the name is an representing the individualcomponents.The term "solution stack" has, historically, occasionally included hardware components as part of a total solution, mixing both the hardware and software in layers of support.MERN(database)(app controller layer)(web app presentation)(web server)A tech stack is the underlying elements of a web or mobile application. These are the frameworks, languages,and software products that everything else is built on. For example, you might have created your web application with —that’s the language and framework. That might access a database created with . You’ll need to host that on a server, say, an . You’ll need to make that happen. Those are all elements of the server-side stack.The client-side tech stack includes HTML, CSS, and JavaScript. That’s what translates your application to a readable format for the user’s browser. If you’ve created a mobile application, the stack is very small: it’s usually only a native application, created with something like Xcode or .stack解释词典:stack美 [stæk]英 [stæk]n.堆栈;⼀摞;⼤量;许多v.(使)放成整齐的⼀叠(或⼀摞、⼀堆)⽹络堆叠;栈区;烟囱1.积材,层积,堆积;〔英国〕⼀堆〔⽊材等的计量单位,=108⽴⽅英尺〕2.(麦杆等的)堆,垛;⼲草堆3.(赌博时的)⼀堆筹码4.烟囱;⼀排[⼀群]烟囱,车船的烟突5.(图书馆的)许多书架,书库6.枪架7.〔英国〕(突出海⾯的)浪蚀岩柱,海中孤峰8.〔⼝语〕许多,⼤量9.【机械⼯程】管组10.【⽆线电】选式存储器栈:堆叠软件技术为什么会有栈的概念,与⼈类认识世界的⼀般概念对应,总是从具体到抽象,从底层到⾼层,⼀个⼤的系统会分为若⼲功能层,例如OSI的七层架构:物理层、链路层、⽹络层、传输层、会话层、表⽰层JAVAEE的三层结构:模型层、逻辑层、表⽰层微软技术栈,底层为基础,上层为应⽤。
ik分词器 添加分词规则
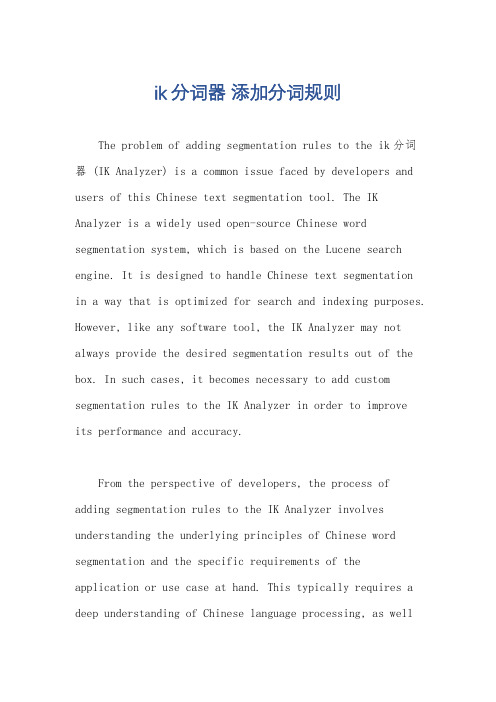
ik分词器添加分词规则The problem of adding segmentation rules to the ik分词器 (IK Analyzer) is a common issue faced by developers and users of this Chinese text segmentation tool. The IK Analyzer is a widely used open-source Chinese word segmentation system, which is based on the Lucene search engine. It is designed to handle Chinese text segmentationin a way that is optimized for search and indexing purposes. However, like any software tool, the IK Analyzer may not always provide the desired segmentation results out of the box. In such cases, it becomes necessary to add custom segmentation rules to the IK Analyzer in order to improveits performance and accuracy.From the perspective of developers, the process of adding segmentation rules to the IK Analyzer involves understanding the underlying principles of Chinese word segmentation and the specific requirements of theapplication or use case at hand. This typically requires a deep understanding of Chinese language processing, as wellas familiarity with the IK Analyzer's architecture andrule-based segmentation approach. Developers may need to analyze the specific text data that the IK Analyzer will be processing and identify patterns or nuances that are not adequately handled by the default segmentation rules. They may also need to consider the impact of any custom rules on the overall performance and efficiency of the segmentation process.From the perspective of users, the need to add custom segmentation rules to the IK Analyzer may arise from encountering specific challenges or limitations in the default segmentation behavior. For example, users may find that the IK Analyzer fails to properly segment certain types of compound words, technical terms, or domain-specific jargon that are relevant to their particular use case. In such situations, users may seek to add custom rules to the IK Analyzer in order to improve the accuracy and relevance of the segmentation results. This could be particularly important in applications such as information retrieval, natural language processing, or text mining, where the quality of segmentation directly impacts theperformance of the overall system.In addressing the problem of adding segmentation rules to the IK Analyzer, it is important to consider the trade-offs and implications of introducing custom rules. While custom rules can potentially improve the accuracy and relevance of segmentation results, they also introduce complexity and the risk of overfitting to specific use cases or data sets. This means that developers and users need to approach the task of adding custom rules with a balanced perspective, taking into account the broader goals and requirements of the application or system in which the IK Analyzer is being used.One approach to adding custom segmentation rules to the IK Analyzer is to leverage the extensibility and customization features that are built into the software. The IK Analyzer provides a range of options for defining custom dictionaries, synonyms, and stop words, which can be used to influence the segmentation behavior. By carefully curating and managing these custom resources, developers and users can effectively add new segmentation rules andimprove the coverage and accuracy of the IK Analyzer's segmentation process. This approach requires a deep understanding of the IK Analyzer's configuration and customization options, as well as a thorough analysis of the specific segmentation challenges that need to be addressed.Another approach to adding custom segmentation rules to the IK Analyzer is to leverage external libraries, tools, or resources that are specifically designed for Chinese word segmentation. For example, developers and users may consider integrating domain-specific dictionaries, ontologies, or knowledge graphs that can provide additional context and guidance for the segmentation process. By combining the strengths of the IK Analyzer with external resources, it is possible to enhance the segmentation capabilities and address specific challenges that may be difficult to handle with the default rules alone. This approach requires a good understanding of the available resources and how they can be effectively integrated with the IK Analyzer, as well as the ability to evaluate and adapt external resources to the specific requirements ofthe segmentation task at hand.In conclusion, the problem of adding segmentation rules to the IK Analyzer is a complex and multifaceted challenge that requires a deep understanding of Chinese language processing, the IK Analyzer's architecture, and thespecific requirements of the application or use case at hand. Both developers and users need to carefully analyze the segmentation challenges they are facing, consider the implications of adding custom rules, and explore different approaches for enhancing the IK Analyzer's segmentation capabilities. By taking a balanced and informed approach to adding custom rules, it is possible to improve the accuracy and relevance of the IK Analyzer's segmentation results and better meet the needs of the applications and systems in which it is used.。
最新建立数位游戏本体导向知识库应用於认知复健PPT课件
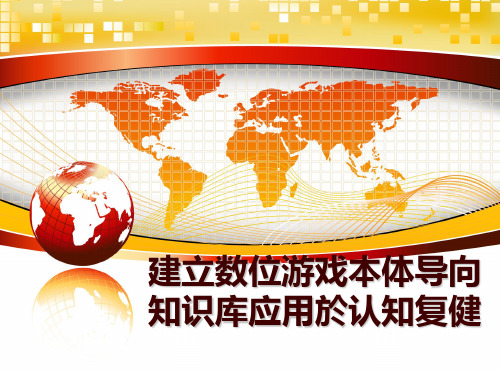
高三英语信息技术单选题50题
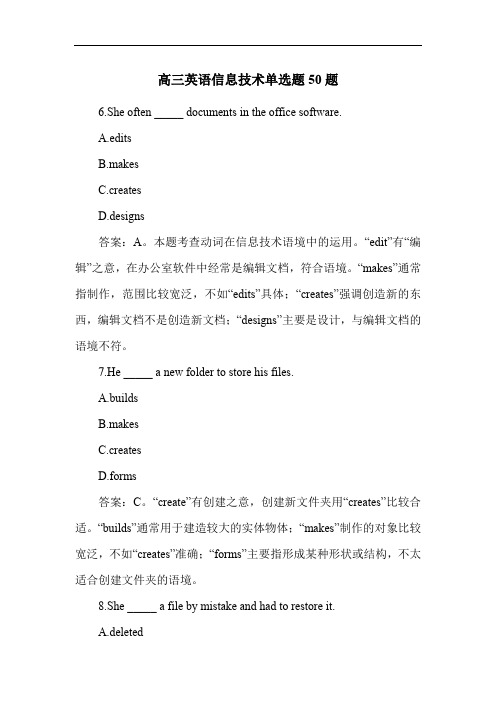
高三英语信息技术单选题50题6.She often _____ documents in the office software.A.editsB.makesC.createsD.designs答案:A。
本题考查动词在信息技术语境中的运用。
“edit”有“编辑”之意,在办公室软件中经常是编辑文档,符合语境。
“makes”通常指制作,范围比较宽泛,不如“edits”具体;“creates”强调创造新的东西,编辑文档不是创造新文档;“designs”主要是设计,与编辑文档的语境不符。
7.He _____ a new folder to store his files.A.buildsB.makesC.createsD.forms答案:C。
“create”有创建之意,创建新文件夹用“creates”比较合适。
“builds”通常用于建造较大的实体物体;“makes”制作的对象比较宽泛,不如“creates”准确;“forms”主要指形成某种形状或结构,不太适合创建文件夹的语境。
8.She _____ a file by mistake and had to restore it.A.deletedB.removedC.lostD.discarded答案:A。
“delete”表示删除,不小心删除了文件符合语境。
“removed”通常指移除某个物体,不一定是删除文件;“lost”是丢失,不一定是主动删除导致的;“discarded”侧重于丢弃不要的东西,不如“deleted”准确。
9.He _____ the file to another location.A.movedB.shiftedC.transferredD.carried答案:C。
“transfer”有转移、传送之意,把文件转移到另一个位置用“transferred”比较恰当。
“moved”和“shifted”比较笼统,没有“transfer”在信息技术语境中那么准确;“carried”通常指携带,不太适合文件转移的语境。
软件出问题的英语作文
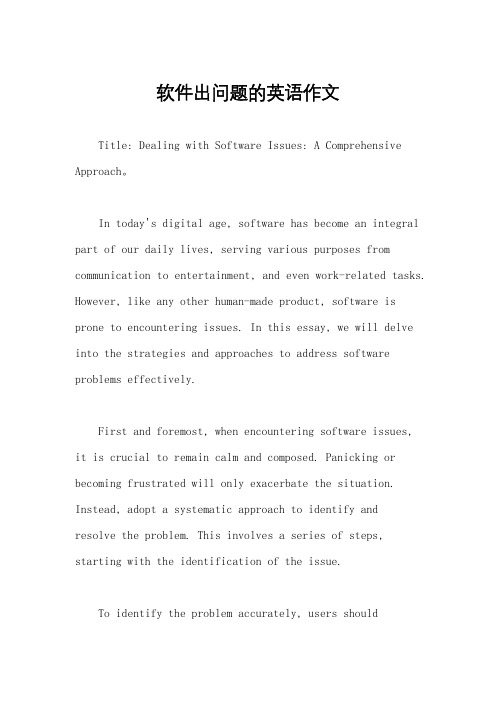
软件出问题的英语作文Title: Dealing with Software Issues: A Comprehensive Approach。
In today's digital age, software has become an integral part of our daily lives, serving various purposes from communication to entertainment, and even work-related tasks. However, like any other human-made product, software is prone to encountering issues. In this essay, we will delve into the strategies and approaches to address software problems effectively.First and foremost, when encountering software issues,it is crucial to remain calm and composed. Panicking or becoming frustrated will only exacerbate the situation. Instead, adopt a systematic approach to identify andresolve the problem. This involves a series of steps, starting with the identification of the issue.To identify the problem accurately, users shouldcarefully observe any error messages or abnormal behaviors exhibited by the software. Additionally, noting the circumstances under which the issue occurred, such as specific actions taken or recent software updates, can provide valuable clues for troubleshooting.Once the problem is identified, the next step is to conduct a thorough analysis of its root cause. This may involve examining the software's code, configuration settings, or compatibility with the operating system and other applications. In some cases, consulting online forums or seeking assistance from technical support professionals may be necessary to pinpoint the underlying issue.After identifying the root cause of the problem, the next phase involves implementing a solution. Depending on the nature of the issue, this may range from applying software patches or updates provided by the developer to modifying configuration settings or reinstalling the software entirely. It is essential to follow recommended procedures and precautions during the implementation process to avoid causing further complications.In situations where resolving the issue requires technical expertise beyond the user's capabilities, seeking assistance from IT professionals or software developers is advisable. These experts possess the knowledge and experience to diagnose and address complex software issues effectively. Moreover, they can provide guidance on preventive measures to minimize the likelihood of similar problems occurring in the future.In addition to reactive measures, proactive steps can also be taken to mitigate the impact of software issues. Regularly updating software to the latest versions, maintaining system backups, and implementing robust cybersecurity measures can help prevent and minimize the impact of software-related disruptions.Furthermore, fostering a culture of continuous learning and adaptation is essential in navigating the ever-evolving landscape of software technologies. This includes staying informed about emerging trends, best practices, and innovative solutions for addressing software issueseffectively.In conclusion, encountering software issues is an inevitable aspect of using technology in our daily lives. However, by adopting a systematic and proactive approach to identify, analyze, and resolve problems, users can minimize disruptions and optimize their software experience. Additionally, seeking assistance from knowledgeable professionals and staying informed about software trends can empower individuals to navigate and overcome software challenges effectively.。
ieee1

A Web Search Contextual Crawler Using OntologyRelation MiningWu ChenshengBeijing Municipal Institute of Science and TechnologyInformationBeijing, ChinaHou Wei, Shi Yanqin, Liu TongSchool of Information EngineeringUniversity of Science and Technology Beijing Beijing Municipal Institute of Science and TechnologyInformationBeijing, ChinaAbstract—In order to increase the correctness and the recall of vertical web search system, a novel web crawler ORC is proposed in this paper. By introducing ontologies, the semantic concepts are defined. The relations between ontologies are exploited to evaluate the importance of web documents’ context. These ontologies are organized by a network structure that is updated periodically. Thanking to the ontology relation mining, the crawling space is expanded and focused more. A primitive vertical search system is built based on ORC at the end of this paper.Keywords-vertical search; ontology; data mining; web crawlerI.I NTRODUCTIONNowadays, Web Search Engine (alternatively, Information Extraction System) has already been one of indispensable applications. Several power search engines have been built, such as google, baidu and so on. However, do they really return your favorite want? Sometimes, it would be failed when you focus on something professional or uncommon concepts. This problem is relieved by vertical search engines[1], which concentrates on only certain concepts or regions. However, to the best of our knowledge, this problem is not solved properly yet up to now. How to increase the correctness and the recall is one of the most urgent issues in web search society.One ideal scenario is semantic web[2], which aims to bridge the concepts between humans and machines. Although many efforts had been made, and several methods had been proposed to establish semantic webs, the ratio of standard semantic webs is very little in the world. In other words, semantic web is not the main current at present.If the structure of web document is organized regular, such as XML, the situation would be better. But the condition is opposite. For that reason, another technique had been proposed, that is ontology. In philosophy, ontology is the study of the nature of being, existence or reality in general, as well as of the basic categories of being and their relations. Traditionally listed as a part of the major branch of philosophy known as metaphysics, ontology deals with questions concerning what entities exist or can be said to exist, and how such entities can be grouped, related within a hierarchy, and subdivided according to similarities and differences. In computer science and information science, an ontology is a formal representation of a set of concepts within a domain and the relationships between those concepts. It is used to reason about the properties of that domain, and may be used to define the domain.In theory, an ontology is a "formal, explicit specification of a shared conceptualization"[3]. An ontology provides a shared vocabulary, which can be used to model a domain — that is, the type of objects and/or concepts that exist, and their properties and relations.In some of web search engines, ontology is utilized to describe and define concepts. The crawlers (alternatively spiders), a kind of agent software, explore the World Wide Web and establish the indices of the concepts. Generally speaking, a common web search engine is composed of crawler algorithms, concept index databases, and search algorithms. Among them, crawler algorithm decides the recall and correctness at a big extent.When the concepts related closely, similar concepts should be likely the wants of users either, besides the one user submitted. Data mining is the process of extracting hidden patterns from large amounts of data. As more data is gathered, with the amount of data doubling every three years[4], data mining is becoming an increasingly important tool to transform this data into information. It is commonly used in a wide range of profiling practices, such as marketing, surveillance, fraud detection and scientific discovery.Constructing the relation networks of ontologies by data mining method, is a novel strategy to organize the concept index database and explore heuristically. That is the main topic of this paper.This paper is organized as follows: The state-of-the-art web search engine and data mining technology are introduced in section II; in section III, ontology concept description technology is discussed; a novel web crawler algorithm ORC, which is based on ontology relation mining, is proposed in section IV; in section V, a primitive search website using ORC is presented.II.B ACKGROUNDA web search engine is a tool designed to search for information on the World Wide Web. Its research could be derived from 1990s. One of the first "full text" crawler-based search engines was WebCrawler, which came out in 1994. Unlike its predecessors, it let users search for any word in any978-1-4244-4507-3/09/$25.00 ©2009 IEEEwebpage, which has become the standard for all major search engines since. It was also the first one to be widely known by the public. Also in 1994 Lycos (which started at Carnegie Mellon University) was launched, and became a major commercial endeavor.Search engines were also known as some of the brightest stars in the Internet investing frenzy that occurred in the late 1990s[5]. Several companies entered the market spectacularly, receiving record gains during their initial public offerings. Some have taken down their public search engine, and are marketing enterprise-only editions, such as Northern Light. Many search engine companies were caught up in the dot-com bubble, a speculation-driven market boom that peaked in 1999 and ended in 2001. Nowadays, a series of search engines are booming out, such as Yahoo, Google, Live search and so on.Web search engines work by storing information about many web pages, which they retrieve from the WWW itself. These pages are retrieved by a Web crawler — an automated Web browser which follows every link it sees. Exclusions can be made by the use of robots. The contents of each page are then analyzed to determine how it should be indexed (for example, words are extracted from the titles, headings, or special fields called meta tags). Data about web pages are stored in an index database for use in later queries. Some search engines, such as Google, store all or part of the source page as well as information about the web pages, whereas others, such as AltaVista, store every word of every page they find. This cached page always holds the actual search text since it is the one that was actually indexed, so it can be very useful when the content of the current page has been updated and the search terms are no longer in it. This problem might be considered to be a mild form of linkrot, and Google's handling of it increases usability by satisfying user expectations that the search terms will be on the returned webpage. This satisfies the principle of least astonishment since the user normally expects the search terms to be on the returned pages. Increased search relevance makes these cached pages very useful, even beyond the fact that they may contain data that may no longer be available elsewhere.While data mining can be used to uncover hidden patterns in data samples that have been "mined", it is important to be aware that the use of a sample of the data may produce results that are not indicative of the domain. Data mining will not uncover patterns that are present in the domain, but not in the sample. Humans have been "manually" extracting information from data for centuries, but the increasing volume of data in modern times has called for more automatic approaches. As data sets and the information extracted from them has grown in size and complexity, direct hands-on data analysis has increasingly been supplemented and augmented with indirect, automatic data processing using more complex and sophisticated tools, methods and models. The proliferation, ubiquity and increasing power of computer technology has aided data collection, processing, management and storage. However, the captured data needs to be converted into information and knowledge to become useful. Data mining is the process of using computing power to apply methodologies, including new techniques for knowledge discovery, to data[6].Data mining identifies trends within data that go beyond simple data analysis. Through the use of sophisticated algorithms, non-statistician users have the opportunity to identify key attributes of processes and target opportunities. However, abdicating control and understanding of processes from statisticians to poorly informed or uninformed users can result in false-positives, no useful results, and worst of all, results that are misleading and/or misinterpreted.III.O NTOLOGYHistorically, ontologies arise out of the branch of philosophy known as metaphysics, which deals with the nature of reality – of what exists. This fundamental branch is concerned with analyzing various types or modes of existence, often with special attention to the relations between particulars and universals, between intrinsic and extrinsic properties, and between essence and existence. The traditional goal of ontological inquiry in particular is to divide the world "at its joints", to discover those fundamental categories, or kinds, into which the world’s objects naturally fall.During the second half of the 20th century, philosophers extensively debated the possible methods or approaches to building ontologies, without actually building any very elaborate ontologies themselves. By contrast, computer scientists were building some large and robust ontologies with comparatively little debate over how they were built.Since the mid-1970s, researchers in the field of artificial intelligence have recognized that capturing knowledge is the key to building large and powerful AI systems. AI researchers argued that they could create new ontologies as computational models that enable certain kinds of automated reasoning. In the 1980s, the AI community began to use the term ontology to refer to both a theory of a modeled world and a component of knowledge systems. Some researchers, drawing inspiration from philosophical ontologies, viewed computational ontology as a kind of applied philosophy[7].In the early 1990s, the widely cited Web page and paper "Toward Principles for the Design of Ontologies Used for Knowledge Sharing" by Tom Gruber[8] is credited with a deliberate definition of ontology as a technical term in computer science. Gruber introduced the term to mean a specification of a conceptualization. That is, an ontology is a description, like a formal specification of a program, of the concepts and relationships that can exist for an agent or a community of agents. This definition is consistent with the usage of ontology as set of concept definitions, but more general. And it is a different sense of the word than its use in philosophy.Ontologies are often equated with taxonomic hierarchies of classes, class definitions, and the subsumption relation, but ontologies need not be limited to these forms. Ontologies are also not limited to conservative definitions – that is, definitions in the traditional logic sense that only introduce terminology and do not add any knowledge about the world. To specify aconceptualization, one needs to state axioms that do constrain the possible interpretations for the defined terms[9].IV.ORCVertical search, or domain-specific search, part of a larger sub-grouping known as "specialized" search, is a relatively new tier in the Internet search, industry consisting of search engines that focus on specific slices of content. The type of content in special focus may be based on topicality or information type. For example, an intelligent medical search engine would clearly be specialized in terms of its topical focus, whereas a video search engine would seek out results within content that is in a video format. So vertical search may focus on all manner of differentiating criteria, such as particular locations, multimedia object types and so on.Broad-based search engines such as Google or Yahoo fetch very large numbers of documents using a Web crawler. Another program called an indexer then reads these documents and creates a search index based on words contained in each document. Each search engine uses a proprietary algorithm to create its indexes so that, ideally, only meaningful results are returned for each query.Vertical search engines, on the other hand, send their spiders out to a highly refined database, and their indexes contain information about a specific topic. As a result, they are of most value to people interested in a particular area; what’s more, the companies that advertise on these search engines reach a much focused audience. For example, there are search engines for veterinarians, doctors, patients, job seekers, house hunters, recruiters, travelers and corporate purchasers, to name a few.Pursuant, for a certain key word, the crawlers of vertical search usually explore broader documents than the one of general search engines. In this section, Ontology Relation Crawler, shortly ORC, is discussed. Its distinguish feature is the contextual sensitivity, which is based on ontology co-existent relation mining.A.Ontology OganizationIn ORC, the concepts described by ontologies are organized by network, as shown in Figure 1. An example of a set of ontologies in ORC about science is depicted. Every ontology is denoted by a frame, and connected with each other by curves. These curves imply the relations between the ontologies. Each curve is correspondent with a coefficient, support called. It decides the co-existent relation between the two ontologies connected by the curve.Figure 1. Example of ontologies in ORCThe supports of the ontology co-existent relations could be compute by frequent pattern mining, a kind of data mining method. The support is evident to be understood. If there are N documents the crawler explored, and s ones from them contain both of ontology A and B, then the support of the pattern A B∧is s N. All of the supports would be updated by the ORC periodically by one frequent pattern mining method modified from fp growth.B.ORC AlgorithmFigure 2. ORC AlgorithmORC algorithm is one iterative process as shown in Figure 2. Every web document online is identified by a Uniform Resource Locator (Url). The domain ontology set O is preset by expertise. In step 7, current document is indexed into the ontologies found.A template database D is maintained by ORC. This database records the information of co-existent relations of ontologies. The supports are updated, in step 11 and 12, by incremental frequent pattern mining from D. The CreateChild function, in step 14,defines the next explore directions by two ways: 1) The links ofcurrent document; 2) The indexed documents which contained co-existent ontologies.C.Rank AlgorithmFigure 3. Rank AlgorithmThe contextual sensitivity of ORC becomes from its ontology network based on co-existent relations. It is realized by its rank algorithm as shown in Figure 3. In step 5, a set of key word relative ontologies would be found for certain document. Only relative ontologies are considered here. Then the certain document’s rank is assessed on its relation degree with the key word by supports in the network in step 8. At last, the rank list would be adjusted for each document in step 9.V.A PRIMITIVE WEB SEARCH ENGINEORC algorithm is utilized in the system, which is a vertical search engine about popular science. This system is implemented by Visual Studio 2008, and the main technology taken is C#. The aim of the system is to popularize science to citizens conveniently. The configuration of the host of the system is, CPU 2.4GHz, RAM 4G.TEXTTOONTO[11] is adopted firstly to establish automatically the ontology database used here, and then the ontology database is purified by the expertise in popular science. As shown in Figure 4, the ontology database is the foundation of the deep search engine of popular science. The initial Html database is comprised of 10000 Html documents about popular science. After 138 hours’ computing, a Url Index Database, whose size was 15 million with 0.1 million ontologies, was acquired by ORC. The user interface component call the function of search operation in the Url index database, in order to feedback the wants of users. From general queries, the system unusually obtains more contextually deeper and broader results than Google and Yahoo, especially in Chinese.Figure 4. The deep search engine of popular scienceVI.CONCLUSIONTo attack the problem of correctness and recall of vertical web search system, a novel web crawler ORC is proposed in this paper. It introduces ontologies, on which the semantic concepts are defined. The relations between ontologies are exploited to evaluate the importance of web documents’ context. These ontologies are organized by a network structure that is updated periodically. Due to the ontology relation mining, the crawling space is expanded and focused more. At the end of this paper, a primitive vertical search system is built based on ORC.A CKNOWLEDGMENTThis work was supported in part by Systems Engineering Study Center Beijing.R EFERENCES[1]M. Chau and H. Chen, "Comparison of three vertical search spiders,"Computer, 2003, vol. 36, pp. 56-62.[2]S.A. McIlraith, T.C. Son and H. Zeng, "Semantic Web services,"Intelligent Systems, 2001, vol. 16, pp.46-53.[3]T. Gruber, "A translation approach to portable ontology specifications,"Knowledge Acquisition, 1993, vol. 5, pp. 199-199.[4]L., Peter and H.R. Varian, "How Much Information,"/how-much-info-2003, 2003.[5]G. Neil, "The dynamics of competition in the internet search enginemarket," International Journal of Industrial Organization, 2001, vol. 19 , pp.1103–1117, doi:10.1016/S0167-7187(01)00065-0.[6]K. Mehmed, “Data Mining: Concepts, Models, Methods, and Algorithms,”John Wiley & Sons, 2003.[7]T. Gruber, "Ontology," To appear in the Encyclopedia of DatabaseSystems, Ling Liu and M. Tamer Özsu (Eds.), Springer-Verlag, 2008.[8]T. R. Gruber, "Toward Principles for the Design of Ontologies Used forKnowledge Sharing," Proc. International Journal Human-Computer Studies, 1995, vol. 43, pp. 907-928.[9]T. R. Gruber, "A translation approach to portable ontologies," Proc.Knowledge Acquisition, 1993, vol. 5, pp. 199-220.[10]J. Han, "Data Mining and Knowledge Discovery," Springer Netherlands,2004.[11]S. Bloehdorn, A. Hotho and S. Staab, “An Ontology-based framework fortext mining,” Journal for computational linguistics and language technology, 2005, Vol.20, pp. 1-20.。
网络信息系统
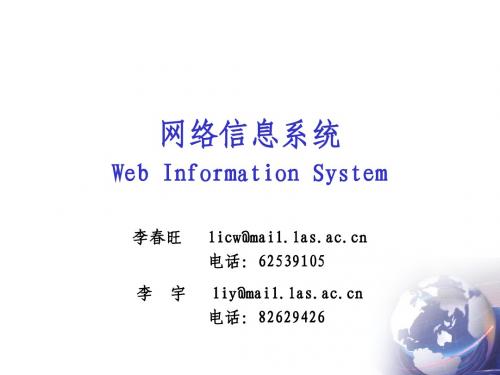
Web Information System
李春旺 李 宇 licw@ 电话:62539105 liy@ 电话:82629426
课程安排
课程内容 考核形式 第一章 WIS概论(1) 平时成绩 : 20% 第二章 XML(2) 最后大开卷: 80% 第三章 Web Services(2) 第四章 Semantics Web(1) 参考教材 第五章 Web Mining(1) 《Web 信 息 系 统 导 论 》 第六章 Web Search (1) 李广建编著,高等教育出 第七章 Web Integration(1) 版社,2008 第八章 Web Mashup(1) 专题讨论(2) 考试
Work typically with well defined and closed data repository
WIS
Work typically with heterogeneous, dynamic and distributed data
一般与限定好的并且是封闭的数据 库一起工作
Serve to well known and specific audience
Web2.0时代WIS 特点
开放性
开放标准:Open standard 开放数据:Linked open data (链接1 链接2) 开放源码: Open sources 开放服务: Open services 信息交互从一对多转向多对多,边际同核心一样重要。 控制和权力结构从中央集中式转向分散式、去中心化。 需求驱动,用户参与。 因为系统互联以及服务集成与嵌入,造成Web系统内容、 功能之间的边界正在加速溶解。
(free) No cookies, no scripts, no frames, no web bugs 目前出500多卷
- 1、下载文档前请自行甄别文档内容的完整性,平台不提供额外的编辑、内容补充、找答案等附加服务。
- 2、"仅部分预览"的文档,不可在线预览部分如存在完整性等问题,可反馈申请退款(可完整预览的文档不适用该条件!)。
- 3、如文档侵犯您的权益,请联系客服反馈,我们会尽快为您处理(人工客服工作时间:9:00-18:30)。
Applications of Ontologies in Software EngineeringHans-Jörg Happel*) and Stefan Seedorf†)*) FZI Forschungszentrum InformatikForschungsgruppe Information Process Engineering (IPE)Haid-und-Neu-Str. 10-14, 76137 Karlsruhe, Germanyhappel@fzi.de†) Universität MannheimLehrstuhl für Wirtschaftinformatik III, Schloss, 68131 Mannheim, Germanyseedorf@uni-mannheim.deAbstract. The emerging field of semantic web technologies promises newstimulus for Software Engineering research. However, since the underlyingconcepts of the semantic web have a long tradition in the knowledgeengineering field, it is sometimes hard for software engineers to overlook thevariety of ontology-enabled approaches to Software Engineering. In this paperwe therefore present some examples of ontology applications throughout theSoftware Engineering lifecycle. We discuss the advantages of ontologies ineach case and provide a framework for classifying the usage of ontologies inSoftware Engineering.1 IntroductionThe communities of Software Engineering and Knowledge Engineering share a number of common topics [1]. While Software Engineering research has been continuously striving towards a higher degree of abstraction and emphasizing software modeling during the last decade, the Knowledge Engineering community has been eager to promote several modeling approaches in order to realize the vision of the semantic web [2].With the advent of web-based software and especially web services, the overlap becomes even more evident. However, both communities mostly live in their own worlds. The number of forums for discussing synergies is still relatively small (e.g. SWESE1, SEKE2 and W3C3) although growing steadily.The discussion on integrating Software and Knowledge Engineering approaches tends to be academic, focusing on aspects like meta-modeling, thereby neglecting important aspects such as applicability and providing little guidance for software engineers. Further, both are cultivating their own understanding of central concepts, making it difficult for members of each community to grasp the concepts of the other one. To overcome this gap, we review potential benefits the Software Engineering1 /msid/conferences/SWESE/2 /seke/3 /2001/sw/BestPractices/2 Hans-Jörg Happel and Stefan Seedorfcommunity can achieve by applying ontologies in various stages of the development lifecycle in this paper.The intended contribution of this paper is threefold. We first provide a concise description of various ontology-based approaches in Software Engineering, ordered by their position in the Software Engineering lifecycle (chapter 2). Second, we propose a framework which allows us to classify the different approaches (chapter 3). Finally, we try to derive some generic advantages of ontologies in the context of Software Engineering.1.1 Software EngineeringSoftware engineering is the “application of a systematic, disciplined, quantifiable approach to the development, operation, and maintenance of software” [3]. Although the claim of software development being an engineering discipline is subject to ongoing discussions, there is no doubt that it has undergone fundamental changes during the last three decades. This assertion holds true both for emergence of new technology and sophistication of methodology.In order to cope with the complexity inherent to software, there has been a constant drive to raise the level of abstraction through modeling and higher-level programming languages. For example, the paradigm of model-driven development proposes that the modeling artifacts are “executable”, i.e. through automated validation and code generation as being addressed by the OMG Model Driven Architecture (MDA) [4]. However, many problems have only partially been solved including component reuse, composition, validation, information and application integration, software testing and quality. Such fundamental issues are the motivation for new approaches affecting every single aspect in Software Engineering. Within this paper, we will thus restrain the scope of interesting applications and techniques to ontologies.1.2 Knowledge EngineeringThe engineering of knowledge-based systems is a discipline which is closely related with Software Engineering. The term Knowledge Engineering is often associated with the development of expert-systems, involving methodologies as well as knowledge representation techniques. Since its early days the notion of “ontology” in computer science has emerged from that discipline, giving rise to Ontology Engineering [5], which we focus on in this paper.Despite sharing the same roots, ontologies emphasize aspects such as inter-agent communication and interoperability [6]. In computer science, the concept “ontology” is interpreted in many different ways and concrete ontologies can vary in several dimensions, such as degree of formality, authoritativeness or quality. As proposed by Oberle [7], different kinds of ontologies can be classified according to purpose, specificity and expressiveness. The first dimension ranges from application ontologies to reference ontologies that are primarily used to reduce terminological ambiguity among members of a community. In the specificity dimension, Oberle distinguishes generic (upper level), core and domain ontologies. Domain ontologies are specific to a universe of discourse, whereas generic and core ontologies meet a higher level ofApplications of Ontologies in Software Engineering 3 generality. According to the expressiveness of the formalism used, one can further distinguish lightweight and heavyweight ontologies.Due to the emergence of the “semantic web” vision ontologies have been attracting much attention recently. Along with this vision, new technologies and tools have been developed for ontology representation, machine-processing, and ontology sharing. This makes their adoption in real-world applications much easier. While ontologies are about to enter mainstream Software Engineering practices, their applications in Software Engineering are manifold, which increases terminological confusion. We therefore try to alleviate some of the confusion by providing a framework for categorizing potential uses of ontologies in Software Engineering.2 Ontologies in the Software Engineering LifecycleIn this chapter, we will present concrete approaches for using ontologies in the context of Software Engineering. The presentation will be in the order of appearance in the Software Engineering lifecycle. Each approach will be described concerning the general problem it tries to solve. It is followed by a short description of the approach and the assumed advantages of ontologies.2.1 Analysis and DesignWithin software analysis and design, two main areas of application are identified: First, requirements engineering can benefit from ontologies in terms of knowledge representation and process support. Second, component reuse is chosen as a potential application area during design.2.1.1 Requirements engineeringProblem addressed: The phase of requirements engineering deals with gathering the desired system functionality from the customers. Since the involved software engineers are often no domain experts, they must learn about the problem domain from the customers. A different understanding of the concepts involved may lead to an ambiguous, incomplete specification and major rework after system implementation. Therefore it is important to assure that all participants in the requirements engineering phase have a shared understanding of the problem domain. Moreover, change of requirements needs to be considered because of changing customer’s objectives.Description of approach: An ontology can be used for both, to describe requirements specification documents [8, 9] and formally represent requirements knowledge [10, 11]. In most cases, natural language is used to describe requirements, e.g. in the form of use cases. However, it is possible to use normative language or formal specification languages which are generally more precise and pave the way towards the formal system specification. Because the degree of expressiveness can be adapted to the actual needs, ontologies can cover semi-formal and structured as well as formal representation [11].4 Hans-Jörg Happel and Stefan SeedorfFurther, the “domain model”4 represents the understanding of the domain under consideration, i.e. in the form of concepts, their relations and business rules. In its simplest form, a glossary may serve as a basis for a domain model. However, it can be formalized using a conceptual modelling language such as the UML. Moreover, the problem domain can be described using an ontology language, with varying degrees formalization and expressiveness.Advantages of ontologies: In contrast to traditional knowledge-based approaches, e.g. formal specification languages, ontologies seem to be well suited for an evolutionary approach to the specification of requirements and domain knowledge [11]. Moreover, ontologies can be used to support requirements management and traceability [8, 10]. Automated validation and consistency checking are considered as a potential benefit compared to semi-formal or informal approaches providing no logical formalism or model theory. Finally, formal specification may be a prerequisite to realize model-driven approaches in the design and implementation phase.2.1.2 Component reuseProblem addressed: Modern Software Engineering practices advise developers to look for components that already exist when implementing functionality, since reuse can avoid rework, save money and improve the overall system quality. Usually, this search for reusable components takes place after the analysis phase, when the functional requirements are settled [12]. Since most reuse repositories are limited to a plain syntactical key-word based search, they are suffering from low precision (due to homonyms) and low recall (due to synonyms) [13].Description of approach: Ontologies can help here to describe the functionality of components using a knowledge representation formalism that allows more convenient and powerful querying [14]. One approach implementing this is the KOntoR system, that allows to store semantic descriptions of components in a knowledge base and run semantic queries on it (using the SPARQL language).Advantages of ontologies: Compared to traditional approaches, ontologies provide two advantages in this scenario. First, they help to join information that normally resides isolated in several separate component descriptions. Second, it provides background knowledge (e.g. about the properties of a certain software license) that allows non-experts to query from their point of view (ask for a license that allows to modify source code).2.2 ImplementationA critical step in the development process is moving from analysis and design to implementation. To this end, the way in which the problem domain is mapped to code has always been playing a pivotal role. The question arises how ontologies can be leveraged to narrow the gap between design and implementation. Two areas of interest are the overlaps of software modelling with ontology languages and the run-4 In Software Engineering, the terms domain model, ontology and CIM (Computation Independent Model) are sometimes used to describe the same thing [cf. 20].Applications of Ontologies in Software Engineering 5 time usage of ontologies in applications. We also look at techniques where ontologies support coding and code documentation.2.2.1 Integration with Software Modelling LanguagesProblems addressed: The current MDA-based infrastructure provides an architecture for creating models and metamodels, define transformations between those models, and managing metadata. Though the semantics of a model is structurally defined by its metamodel, the mechanisms to describe the semantics of the domain are rather limited compared to knowledge representation languages [cf. 15]. MDA–based languages do not have a knowledge-based foundation to enable reasoning. Other possible shortcomings include validation and automated consistency checking. However, this is addressed by the Object Constraint Language (OCL).Description of approach: There are several alternatives for integrating MDA-based information representation languages and ontology languages, which are exemplified in [16]. Whereas some regard the UML as ontology representation language by defining direct mappings between language constructs [17], others employ the UML as modelling syntax for ontology development [18]. In most cases, MDA-compliant languages and RDF/OWL are regarded as two distinct technological spaces sharing a “semantic overlap” where synergies can be realized by defining bridges between them [19]. The Ontology Definition Metamodel (ODM) [20] is an effort to standardize the mappings between knowledge representation and conceptual modelling languages. It specifies a set of MOF metamodels for RDF Schema and OWL among others, informative mappings between those languages, and profiles for a UML-based notation.Advantages of ontologies: Software modelling languages and methodologies can benefit from the integration with ontology languages such as RDF and OWL in various ways, e.g. by reducing language ambiguity, enabling validation and automated consistency checking [cf. 15]. Ontology languages provide better support for logical inference, integration and interoperability than MOF-based languages. UML-based tools can be extended more easily to support the creation of domain vocabularies and ontologies. Since ontologies promote the notion of identity, ODM and related approaches simplify the sharing and mediation of domain models.2.2.2 Ontology as Domain Object ModelProblems addressed: Since a domain model is initially unknown and changes over time, a single abstraction and separation of concerns is considered feasible if not necessary [cf. 21]. Therefore a single representation of the domain model should be shared by all participants throughout the lifecycle to increase quality and reduce costs [22]. The mapping of a domain model to code should therefore be automatized to enable the dynamic use by other components and applications.Description of approach: The programmatic access of ontologies and manipulation of knowledge bases using ontology APIs requires special knowledge by the developers. Therefore an intuitive approach for object-oriented developers is desirable [cf. 23]. This can be achieved by ontology tools that generate an API from the ontology, e.g. by mapping concepts of the ontology to classes in an object-6 Hans-Jörg Happel and Stefan Seedorforiented language. The generated domain object model can then be used managing models, inferencing, and querying. Tools supporting those features are already available today, e.g. [23] and [24].Advantages of ontologies: The end-to-end use of ontologies in analysis and design as well as implementation is highly suitable for rapid application development [22]. Not only is this an intuitive way for object-oriented developers for managing ontologies and knowledge models, interoperability with other components or applications is improved as well. The use of a web-based knowledge representation format enables developers to discover sharable domain models and knowledge bases from internal and external repositories.2.2.3 Coding SupportProblem addressed: In object-oriented software development, the concept of encapsulation demands the decoupling of the interface specification from its implementation in order to make requesting applications independent from internal modifications [25]. Nowadays, developers face a large number of frameworks and libraries they have to access through application programming interfaces (APIs). Thus, the documentation of APIs has become an important issue. Some IDEs like Eclipse use this information to enhance developer productivity by providing auto-completion of method calls. However, many operations (such as the connection to a database) require several calls to an API. While developers could benefit from formalized knowledge about the interrelations of method calls in the API in a similar way to auto-completion, there is currently no support for this.Description of approach: The SmartAPI approach [26] suggests enriching APIs with semantic information. Since the semantics of string parameters like "username" or "password" is only clear for users, but not for machines, they must be annotated with the concept "database user name". The authors propose to store those annotations via a public web service to enable a collaborative knowledge acquisition effort. Besides the easier location of API interfaces and methods, the authors present how a suitable sequence of method calls can be automatically generated, given a desired goal state (like getting a database result set).Advantages of ontologies: In the SmartAPI scenario, the main advantage of ontologies is that they provide a globally unique identifier for concepts. While at the programming level it is convenient to have a limited set of data "types" like strings, that can be used for multiple purposes, an ontology enables developers to annotate API elements with an unambiguous concept. A potential drawback is the extra-effort for modelling the semantic layer. In the case of APIs, this is partially eased since an initial modelling effort scales well with the estimated reuse. However, the question of incentives for someone to semantically describe an API still remains.2.2.4 Code DocumentationProblem addressed: The maintenance of software systems is one of the most dominant activities in Software Engineering. However, programming languages as the default representation of knowledge in Software Engineering are badly suited forApplications of Ontologies in Software Engineering 7 maintenance tasks. They describe knowledge in a procedural way and are rather geared towards the execution of code than towards the querying of knowledge [27].Description of approach: So called "Software Information Systems" (SIS) [28, 29] were among the first approaches that applied description logics to Software Engineering problems. Their main goal was to improve the maintainability of large software systems by providing powerful query mechnisms. The LaSSIE system [28] for example consists of programming-language independent descriptions of software structures and an ontology that describes the problem domain of the software. Both can be manually connected to allow e.g. querying for all functions dealing with a certain domain object.Advantages of ontologies: Here, ontologies provide a unified representation for both problem domain and source code, thus enabling easier cross-references among both information spheres. Moreover, it is easy to create arbitrary views on the source code (e.g. concerning a variable). Reasoning is applied to create those views, e.g. to find all places where a variable is accessed either directly or indirectly.2.3 Deployment and Run-time2.3.1 Semantic MiddlewareProblem addressed: In modern three-tier architectures, the middleware layer lies in the focus of attention. Sophisticated middleware infrastructure like application servers shield a lot of complexity from the application developer, but creates challenging tasks for the administrator. Issues like interdependencies between modules or legal constraints make the management of middleware systems a cumbersome task. Description of approach: In the context of his work in the area of semantic management of middleware [7], Oberle developed a number of ontologies for the formal description of concepts from component-based- and service-oriented development5. His goal is to support system administrators in managing server applications, e.g. by making knowledge about library dependencies explicit. The conceptualization of the ontology was driven by two objectives: to provide a precise, formal definition of some ambiguous terms from Software Engineering (like "component" or "service") as well as structures supporting the formalization of middleware knowledge (i.e. by modelling the dependencies of libraries, licenses etc.). Advantages of ontologies: In this case, ontologies provide a mechanism to capture knowledge about the problem domain. So the semantic tools in this approach create an information space where knowledge, e.g. about library dependencies, can be stored. Reasoning can then be applied to reuse this knowledge for various purposes. Oberle provides a detailed qualitative analysis on the modelling effort for a number of use-cases [7].2.3.2 Business RulesProblem addressed: In most software systems, the "business logic" - i.e. the mechanisms implemented in software systems to comply with the business policies of 5 8 Hans-Jörg Happel and Stefan Seedorfa company - are hard-coded in programming languages. Thus, changes to the business logic of a software system require modifications to the source code, triggering the normal compilation and deployment cycle. Since many companies are facing flexible, frequently changing business environments nowadays, technologies are sought, that support a quick propagation of new business rules into the core software systems [30]. Description of approach: (Business) rule engines are a possible solution approach for this problem. The core idea is to untangle business logic and processing logic. The business logic is modelled declaratively with logical statements and processed by a rule engine. Similar to a reasoner, it applies inference algorithms to derive new facts on a knowledge base. Most rule engines forward-chain rules in the knowledge base to infer actions the system should take [31]. While business rule engines are available for quite some time, they can be regarded as "ontology-based" approaches towards Software Engineering since they run declarative knowledge on a special middleware. Also there are standardization efforts in place to enable interoperability between rule formalisms used by the industrial vendors and those proposed in the semantic web community6. A better integration of rules with available description logics formalisms [32] could also help to establish a "knowledge layer" in system architectures which is served by a special kind of middleware (i.e. inference engines).Advantages of ontologies: The main advantage in this approach is the declarative specification of knowledge which tends to change frequently. Business rules that would be hard-coded in most current systems, can be changed more easily, because they are not buried implicitly in some source code, but explicitly stated in a formal language that can be presented in a user friendly way for editing.2.3.3 Semantic Web ServicesProblem addressed: Offering data and services via well-defined interface descriptions in the web is the core idea of "web services" [33]. While web services enable developers to combine information from different sources to new services in the first place7, the actual composition process remains troublesome. First, it is rather difficult to find appropriate services, since most industry standards (e.g. WSDL) are purely syntactical. Thus, also the wiring of services has to be done manually, since an algorithm can not find out, whether a string output "credcardNo" of some service is appropriate as a string input value for "ccNumber" for another service.Description of approach: The basic idea of the semantic web services effort is to add a semantic layer on top of the existing web service infrastructure [34]. Input parameters, functionality and return values are annotated semantically, such that - at least in theory - automatic discovery, matching and composition of service-based workflows. Several standards and frameworks like OWL-S [35] or WSMX [36] are currently under development.Advantages of ontologies: In the case of semantic web services, ontologies provide the flexibility that is sought in dynamic scenarios. They can ensure discovery and interoperability in cases that were not anticipated by the initial developer, since semantic descriptions can be extended in the course of time. Even mediation among6 /2005/rules/7 e.g. …mash-ups“ - /Applications of Ontologies in Software Engineering 9services that have been developed independently and annotated with different ontologies could interoperate by defining mappings that are supported by most ontology languages.2.4 Maintenance2.4.1 Project SupportProblem addressed: In software maintenance workflows, several kinds of related information exists without an explicit connection. This is problematic, since a unified view could avoid redundant work and speed up problem solving. A bug resolution process for example usually involves the discovery and reporting of a bug (often into a bug tracking system), subsequent discussion inside a developer group, and finally changes in the code that hopefully resolve the bug. While the discussion on the mailing list and the code changes are clearly triggered by the bug report, their relation is not necessarily explicit and often kept separately. Since it is difficult to manage larger amounts of bugs without all existing context information, the lack of tool support may lead to delays in bug fixing and duplicate work or discussions.Description of approach: Dhruv [37, 38] is a semantic-web enabled prototype to support problem-solving processes in web communities. The application scenario is how open source communities deal with bugs in their software under development. Ontologies help to connect the electronic communication (via forums and mailing lists) of the developers with bug-reports and the affected areas in the source code. Central concepts are the community (e.g. developers), their interactions and content (e.g. emails). The knowledge is codified in three kinds of ontologies: two “content” ontologies describe the structure of artefacts, i.e. a software ontology based on Welty’s work and a taxonomy of software bugs. Second, an ontology of interactions describes the communication flow among the developers. Third, a community ontology defines several roles that are involved in the problem solving process.Advantages of ontologies: In the Dhruv system, ontologies primarily provide a layer to integrate data from different source into a unified semantic model. The combined data can then be used to derive additional information that was not stated explicitly in one of the single sources before. The author gives the example of classifying people into roles like “bug-fixer” or “core developer” [37, p. 173].2.4.2 UpdatingProblem addressed: Agile development practices like rapid prototyping have led to an acceleration of release cycles for software products. So, keeping one's application zoo up-to-date is a time consuming tasks that involves checking for new versions, downloading and installing them. Although lots of modern software programs come with auto-update functions, there is no general mechanism to cope with such problems in a platform independent way.Description of approach: Dameron describes a framework for automatically updating the Protege ontology editor and its plug-ins [39]. Therefore he uses an10 Hans-Jörg Happel and Stefan Seedorfextension of the DOAP ontology8 and a python script that retrieves the most recent version number and a download URL by calling a web service that does reasoning.Advantages of ontologies: The basic advantage of an RDF-based solution in contrast to e.g. describing the download information in XML is extensibility. Using an XML schema, all plug-in providers must provide their data in the specified format. In order to stay compatible to the update script, changes would have to be done centrally and distributed to all plug-in providers. Using an RDF ontology, every provider is free to add or subclass concepts from the initial version without being at risk to become incompatible.2.4.3 TestingProblem addressed: Software tests are an important part of quality assurance [3]. However, the writing of test cases is an expensive endeavour that does no directly yield business value. It is also not a trivial task, since the derivation of suitable test cases demands a certain amount of domain knowledge.Description of idea: Ontologies could help to generate basic test cases since they encode domain knowledge in a machine processable format. A simple example for this would be regarding cardinality constraints. Since those constraints define restrictions on the association of certain classes, they can be used to derive equivalency classes for testing (see also [23]).Advantages of ontologies: Ontologies may not be the first candidate for such a scenario, since there are formalisms like OCL that are specialized for such tasks. However, once domain knowledge is available in an ontology format anyway (e.g. due to one of the various other scenarios described in this paper), it might be feasible to reuse that knowledge.3 Categorizing Ontologies in Software EngineeringThe preceding section has presented a number of different approaches for using ontologies in the context of Software Engineering. In this chapter, we propose a simple classification scheme that allows a better differentiation among the various ideas. While the ordering according to their position in the Software Engineering lifecycle was suitable to provide a first roundtrip in the world of ontologies and Software Engineering, we think there should be more meaningful distinctions regarding their application. Common categorizations of ontologies rank them by their level of abstraction and their expressivity (see sec. 1). However, when trying to understand how ontologies can be applied in Software Engineering and what the benefits are in each case, this distinction does not help much.The Ontology Driven Architecture (ODA) note at W3C merely served as a starting point to elaborate a systematic categorization of the approaches and to derive more clearly defined acronyms [cf. 15]. Rethinking the approaches described in section 2, and bearing in mind the basic properties of Software Engineering, we propose two dimensions of comparison to achieve a more precise classification. First, we distinguish the role of ontologies in the context of Software Engineering between 8 /doap/。