统计学习[The Elements of Statistical Learning]第四章习题
统计学习[The Elements of Statistical Learning]第七章习题
![统计学习[The Elements of Statistical Learning]第七章习题](https://img.taocdn.com/s3/m/1e5b030002020740be1e9b7f.png)
m∈L,m>l
α10−l < 2π
10m−l 10−l +
2
2
m∈L
10m−l 1
10m−l 10−l
= 2π
++
+
22
2
2
m∈L,m>l
m∈L,m<l
10m−l 1 ∞ 10−i
< 2π
++
(7)
22
2
m∈L,m>l
i=1
10m−l 1 1
1
< 2π
+ + = 2π(k + )
(8)
2 22
2
m∈L,m>l
≥0
⇒ 0 ≤ Sii < 1
yi
−
fˆ−i(xi)
=
yi − fˆ(xi) 1 − Sii
≥ yi − fˆ(xi)
// from (1)(4)
3. If the recipe for producing ˆf from y does not depend on y itself and S depends only on the xi and λ, we can just replace yi with fˆ−i(xi) in y (y → y ) without S being changed in the
// from (3)
=
xTi A−1
+
SiixTi A−1 1 − Sii
(XT y − xiyi)
(4)
=
xTi A−1XT y
−
xTi A−1xiyi
+
统计学习StatisticalLearning
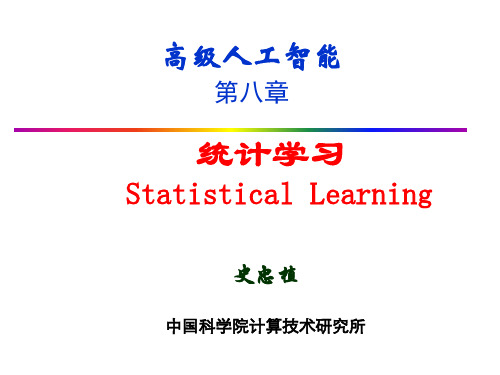
samples (large l/h)
Large l/h induces a small which
decreases the the upper bound on risk
Small samples? Small empirical risk doesn’t guarantee anything! …we need to minimise both terms of the RHS of the risk bounds
统计方法是从事物的外在数量上的表现去推断该事物可 能的规律性。科学规律性的东西一般总是隐藏得比较深, 最初总是从其数量表现上通过统计分析看出一些线索, 然后提出一定的假说或学说,作进一步深入的理论研究。 当理论研究 提出一定的结论时,往往还需要在实践中加 以验证。就是说,观测一些自然现象或专门安排的实验 所得资料,是否与理论相符、在多大的程度上相符、偏 离可能是朝哪个方向等等问题,都需要用统计分析的方 法处理。
For given z1,…,zl and an admissible structure S1S2…Sn… S, SRM chooses function Q(z,lk) minimising Remp in Sk for which the
Problem: how rich class of classifications q(x;θ) to use.
underfitting
good fit
overfitting
Problem of generalization: a small emprical risk Remp does not
Vapnik V N. 1995. The Nature of Statistical Learning Theory. Springer-Verlag, New York
统计学习方法PDF
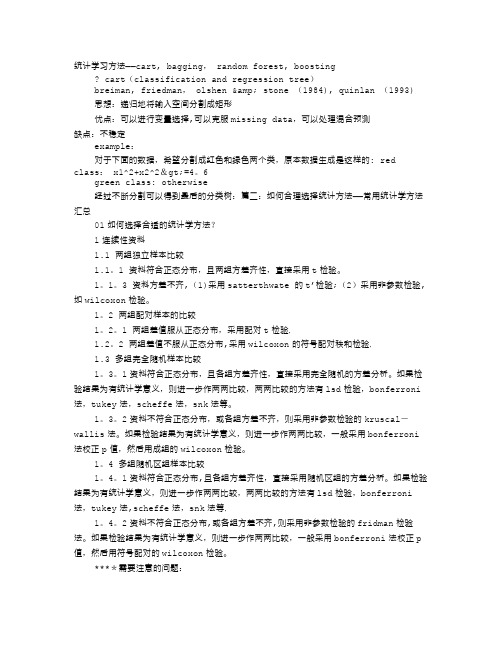
统计学习方法——cart, bagging, random forest, boosting? cart(classification and regression tree)breiman, friedman, olshen & stone (1984), quinlan (1993) 思想:递归地将输入空间分割成矩形优点:可以进行变量选择,可以克服missing data,可以处理混合预测缺点:不稳定example:对于下面的数据,希望分割成红色和绿色两个类,原本数据生成是这样的: red class: x1^2+x2^2&gt;=4。
6green class: otherwise经过不断分割可以得到最后的分类树:篇二:如何合理选择统计方法——常用统计学方法汇总01如何选择合适的统计学方法?1连续性资料1.1 两组独立样本比较1.1。
1 资料符合正态分布,且两组方差齐性,直接采用t检验。
1。
1。
3 资料方差不齐,(1)采用satterthwate 的t’检验;(2)采用非参数检验,如wilcoxon检验。
1。
2 两组配对样本的比较1。
2。
1 两组差值服从正态分布,采用配对t检验.1.2。
2 两组差值不服从正态分布,采用wilcoxon的符号配对秩和检验.1.3 多组完全随机样本比较1。
3。
1资料符合正态分布,且各组方差齐性,直接采用完全随机的方差分析。
如果检验结果为有统计学意义,则进一步作两两比较,两两比较的方法有lsd检验,bonferroni 法,tukey法,scheffe法,snk法等。
1。
3。
2资料不符合正态分布,或各组方差不齐,则采用非参数检验的kruscal-wallis法。
如果检验结果为有统计学意义,则进一步作两两比较,一般采用bonferroni 法校正p值,然后用成组的wilcoxon检验。
1。
4 多组随机区组样本比较1。
4。
1资料符合正态分布,且各组方差齐性,直接采用随机区组的方差分析。
统计学习[The Elements of Statistical Learning]第五章习题
![统计学习[The Elements of Statistical Learning]第五章习题](https://img.taocdn.com/s3/m/4e4b6369011ca300a6c3907f.png)
4.
N
N
L(yi, g˜(xi)) + λJ(g˜) ≥ L(yi, g(xi)) + λJ(g)
i=1
i=1
with equality iff ρ(x) = 0.
1
Proof 1.
2.
3.
K(·, xi), f
HK
= ∞ ci i=1 γi
K(·, xi), φi(·)
=
∞ i=1
ci γi
[γi
(5)
β
i=1
N
= min
(yi
−
βT
1
VDγ2
φ(xi))2
+
λβT
β
β
i=1
N
= min
(yi
−
cT
φ(xi))2
+
λ(VD−γ
1 2
c)T
VDγ−
1 2
c
c
i=1
//
Let
c
=
1
Dγ2
VT β
N
= min
c
(yi − cT φ(xi))2 + λcT cD−γ 1
i=1
N
= min
{cj }∞ 1 i=1
m=1
Let gkm = hm(x)φk(x)dx and calculate ·, φ (y) , then
M
gkmhm(y) = γkφk(y)
(4)
m=1
M
gkm( hm(y)φ (y)dy) = γk φk(y)φ (y)dy
m=1
M
gkmg m = γkδk,
m=1
3
where
概率论与数理统计专业硕士研究生培养方案070103
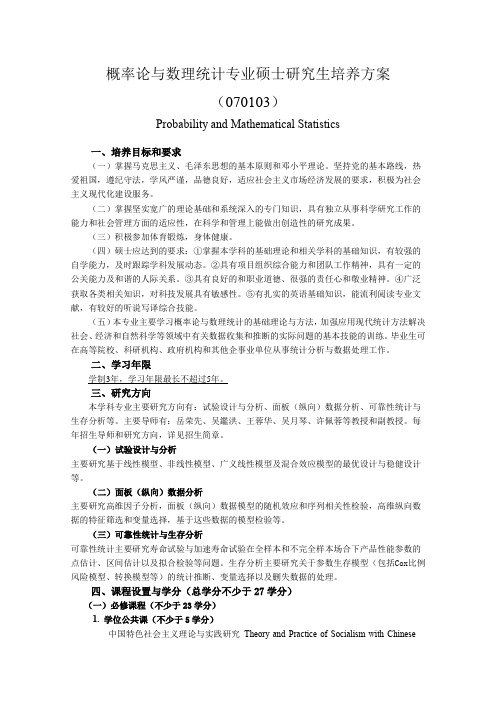
概率论与数理统计专业硕士研究生培养方案(070103)Probability and Mathematical Statistics一、培养目标和要求(一)掌握马克思主义、毛泽东思想的基本原则和邓小平理论。
坚持党的基本路线,热爱祖国,遵纪守法,学风严谨,品德良好,适应社会主义市场经济发展的要求,积极为社会主义现代化建设服务。
(二)掌握坚实宽广的理论基础和系统深入的专门知识,具有独立从事科学研究工作的能力和社会管理方面的适应性,在科学和管理上能做出创造性的研究成果。
(三)积极参加体育锻炼,身体健康。
(四)硕士应达到的要求:①掌握本学科的基础理论和相关学科的基础知识,有较强的自学能力,及时跟踪学科发展动态。
②具有项目组织综合能力和团队工作精神,具有一定的公关能力及和谐的人际关系。
③具有良好的和职业道德、很强的责任心和敬业精神。
④广泛获取各类相关知识,对科技发展具有敏感性。
⑤有扎实的英语基础知识,能流利阅读专业文献,有较好的听说写译综合技能。
(五)本专业主要学习概率论与数理统计的基础理论与方法,加强应用现代统计方法解决社会、经济和自然科学等领域中有关数据收集和推断的实际问题的基本技能的训练。
毕业生可在高等院校、科研机构、政府机构和其他企事业单位从事统计分析与数据处理工作。
二、学习年限学制3年,学习年限最长不超过5年。
三、研究方向本学科专业主要研究方向有:试验设计与分析、面板(纵向)数据分析、可靠性统计与生存分析等。
主要导师有:岳荣先、吴鑑洪、王蓉华、吴月琴、许佩蓉等教授和副教授。
每年招生导师和研究方向,详见招生简章。
(一)试验设计与分析主要研究基于线性模型、非线性模型、广义线性模型及混合效应模型的最优设计与稳健设计等。
(二)面板(纵向)数据分析主要研究高维因子分析,面板(纵向)数据模型的随机效应和序列相关性检验,高维纵向数据的特征筛选和变量选择,基于这些数据的模型检验等。
(三)可靠性统计与生存分析可靠性统计主要研究寿命试验与加速寿命试验在全样本和不完全样本场合下产品性能参数的点估计、区间估计以及拟合检验等问题。
统计学习理论
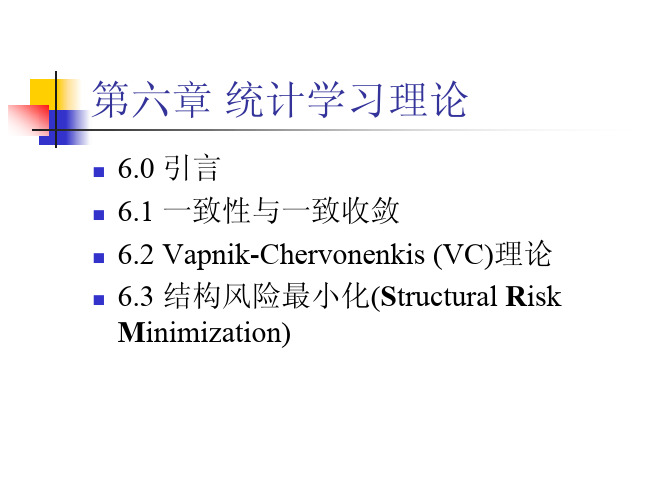
是很特殊的,通常只需要有某一合理数量的观测就可以解 决。
6.0 引言
平方误差损失函数: L( y, f ( x,θ )) = ( y − f ( x,θ ))2
ε 不敏感损失函数:
L( y, f ( x,θ )) =| y − f ( x,θ ) |ε ={ 0 | y − f ( x,θ ) |≤ ε | y − f ( x,θ ) | 其他
Θ = ℜ d × ℜ.
损失函数:
L( y, f ( x,θ )) = ( y − f ( x,θ )) = ( y − w x − b) .
2 T 2
6.0 引言
学习:从学习函数集中挑一个“最优”的。 什么是“最优”?
统计推断:期望风险最小化( RM ) 期望风险
∆
R(θ ) = R( f (•,θ )) = ∫ L( y, f ( x,θ ))dF ( x, y )
经验风险和期望风险
期望风险
经验风险 n
6.1 一致性与一致收敛
经验风险和期望风险都是学习函数集的 函数(泛函)。 学习的目的:通过求使经验风险最小化 的学习函数来逼近使期望风险最小化的 函数。 注意: ERM原则一致性的充分必要条件 取决于学习函数集中最差函数的性能。
6.1 一致性与一致收敛
期望风险和经验风险都是 θ 的函数,概率论中的大数定理只 说明了当样本趋于无穷多时经验风险将在概率意义上趋近 于期望风险,并没有保证两个风险的 θ 是同一点,更不能保 证经验风险能够趋近于期望风险。 即使有办法使这些条件在样本数无穷大时得到保证, 也无 法认定在这些前提下得到的经验风险最小化方法在样本数 有限时仍能得到好的结果。
统计学习[The Elements of Statistical Learning]第二章习题
![统计学习[The Elements of Statistical Learning]第二章习题](https://img.taocdn.com/s3/m/7958f544b307e87101f6967f.png)
Proof “If there are multiple observation pairs xi, yi , = 1, . . . , Ni at each value of xi, the risk
is limited.”(Page 32) We should estimate the parameters θ in fθ by minimizing the residual sum-of-
squares, i.e. calculate argminθ
i
Ni =1
(fθ
(xi
)
−
yi
)2,
while
Ni
Ni
argmin
(yi − fθ(xi))2 = argmin
(yi2 − 2yi fθ(xi) + fθ(xi)2)
θ
i =1
θ
i =1
= argmin
θ
i
Ni
yi2 − 2Ni
=1
Ni =1
1
P (X = x|(mk)i, Gk) = 10 f (x; (mk)i, I/5)
(5)
10 1
P (X = x|mk, Gk) = 10 f (x; (mk)i, I/5)
(6)
i=1
The values of mk is unknown, so we should marginalize them out.
(3)
1
where
f (x; µ, σ2) = √ 1 e−(x−µ)2/(2σ2)
(4)
2πσ2
Then for each class 100 observations are generated as follows: for each observation, an (mk)i at random with probability 1/10 is picked, and then generated a N ((mk)i; I/5), thus leading to a mixture of Gaussian clusters for each class. So we have
统计学习理论导论(清华大学张学工讲义)-1
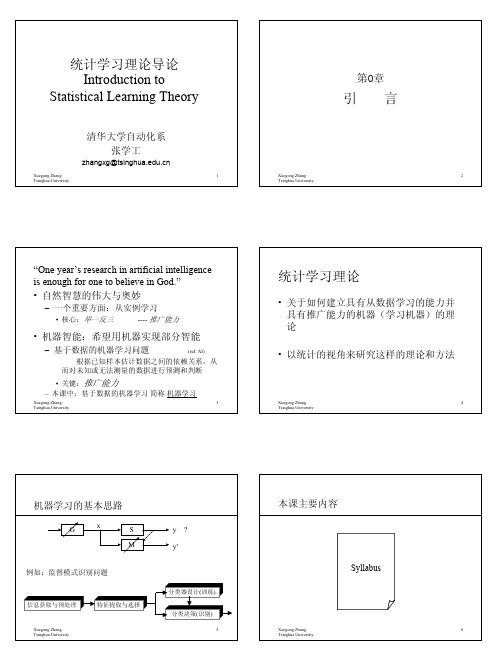
• How to decide the structure of the MLP?
(How many hidden layers and nodes?)
– Ask God, or guess then pray
• How to choose the neuron function?
– Usually Sigmoid (S-shaped) function
– the effort to approach mathematic models for natural nervous systems
– the effort to implement man-made intelligence
• Three types of NN:
– Feedforward NN – Feedback NN – Competitive Learning (Self-organizing) NN
Xuegong Zhang
27
Tsinghua University
学习过程的应用分析与理论分析学派
• 关于感知器学习能力的若干结论: – 关于收敛性的结论 – 关于收敛以后的测试错误率(推广能力)的结论
[Novikoff, 1962] [Aizerman, Braverman, and Rozonoer, 1964]
• 学习过程的应用分析学派:
– 最小化训练错误数是不言而喻的归纳原则,学习的主要问题在于 寻找同时构造所有神经元的系数的方法,使所形成的分类面能达
到最小的训练错误率,(这样即可得到好的推广性)
• 学习过程的理论分析学派:
Xuegong Zhang
14
Tsinghua University
- 1、下载文档前请自行甄别文档内容的完整性,平台不提供额外的编辑、内容补充、找答案等附加服务。
- 2、"仅部分预览"的文档,不可在线预览部分如存在完整性等问题,可反馈申请退款(可完整预览的文档不适用该条件!)。
- 3、如文档侵犯您的权益,请联系客服反馈,我们会尽快为您处理(人工客服工作时间:9:00-18:30)。
ˆ = (N − 2)Σ
k=1 gi =k 2
(xi − µ ˆk )(xi − µ ˆk )T (xi xT ˆT ˆk µ ˆT i − 2 xi µ k +µ k)
k=1 gi =k 2
(9)
=
// xT ˆ k = xi µ ˆT i µ k
(10)
=
k=1 N gi =k
xi xT ˆk µ ˆT i − Nk µ k
(5)
=
k=1
Nk µ ˆk
N yk − (N1 y1 + N2 y2 ) N
x i = Nk µ ˆk
(6) (7) (8)
N1 N2 (y2 − y1 )(ˆ µ2 − µ ˆ1 ) N = N (ˆ µ2 − µ ˆ1 ) // y1 = N/N1 , y2 = N/N2 =
2
We also have
//
gi =k
x i = Nk µ ˆk
(11)
=
i=1 N
xi xT ˆ1 µ ˆT ˆ2 µ ˆT i − (N1 µ 1 + N2 µ 2)
2
(12)
xi x ¯T =
i=1 k=1 gi =k
xk
2 k=1
gi =k
xk
T
N
(13) (14)
= Meanwhile
N N
1 (N1 µ ˆ 1 + N2 µ ˆ2 )(N1 µ ˆ 1 + N2 µ ˆ2 )T N
1
ˆ0 , and hence the predicted values f ˆ= β ˆ0 + β ˆT x. Consider the following 5. Find the solution β rule: classify to class 2 if y ˆi > 0 and class 1 otherwise. Show this is not the same as the LDA rule unless the classes have equal numbers of observations. (Fisher, 1936; Ripley, 1996) Proof 1. Consider the log-ratio of each class density (equation 4.9 in text book) log π2 1 Pr(G = 2|X = x) = log − (µ2 + µ1 )T Σ−1 (µ2 − µ1 ) + xT Σ−1 (µ2 + µ1 ) Pr(G = 1|X = x) π1 2
N1 N2 N (y2
(17) (18)
(19) (20) (21)
4. By replacing N with ˆ= β
− y1 ) (from (7) and (8)) and from (20), we still have
N1 N2 ˆ −1 (ˆ ˆ −1 (ˆ (y2 − y1 ) − (ˆ µ2 − µ ˆ1 )T β Σ µ2 − µ ˆ1 ) ∝ Σ µ2 − µ ˆ1 ) N (N − 2)
When it > 0, the LDA rule will classify x to class 2, meanwhile, we need to estimate the parameters of the Gaussian distributions using our training data 1 ˆ −1 (ˆ ˆ −1 (ˆ µ2 + µ ˆ 1 )T Σ µ2 − µ ˆ1 ) + log(π1 ) − log(π2 ) xT Σ µ2 − µ ˆ1 ) > (ˆ 2 1 T ˆ −1 1 T ˆ −1 N1 N2 = µ ˆ Σ µ ˆ2 − µ ˆ Σ µ ˆ1 + log − log 2 2 2 1 N N 2. Let β = (β, β0 )T and compute the partial deviation of the RSS(β ), then we have ∂ RSS(β ) = −2 (yi − β0 − β T xi ) = 0 ∂β0 i=1 ∂ RSS(β ) = −2 xi (yi − β0 − β T xi ) = 0 ∂β i=1 We can also derive that β0 =
1
a = W− 2 b,
1
aT = bT W− 2
1
Hence the generalized eigenvalue problem max(aT Ba) = max(bT W− 2 BW− 2 b)
a b
1 1
subject to
aT Wa = bT W− 2 WW− 2 b = 1 So the problem is transformed to a standard eigenvalue problem. Ex. 4.2 Suppose we have features x ∈ Rp , a two-class response, with class sizes N1 , N2 , and the target coded as −N/N1 , N/N2 . 1. Show that the LDA rule classies to class 2 if 1 T ˆ −1 N1 1 T ˆ −1 N2 ˆ −1 (ˆ ˆ2 Σ µ ˆ2 − µ ˆ1 Σ µ ˆ1 + log xT Σ µ2 − µ ˆ1 ) > µ − log , 2 2 N N and class 1 otherwise. 2. Consider minimization of the least squares criterion
N
N
xi [β (xi − x ¯)] =
i=1 i=1
T
xi [(xi − x ¯) β ] =
i=1
T
xi xT i
−
i=1
xi x ¯T β
(15)
1 ˆ + (N1 µ = (N − 2)Σ ˆ1 µ ˆT ˆ2 µ ˆT (N1 µ ˆ 1 + N2 µ ˆ2 )(N1 µ ˆ 1 + N2 µ ˆ2 )T β // from (12)(14) 1 + N2 µ 2)− N (16) ˆ + N1 N2 (ˆ µ2 µ ˆT ˆ1 µ ˆT ˆ2 µ ˆT ˆ1 µ ˆT = (N − 2)Σ 2 −µ 2 −µ 1 +µ 1) β N ˆ B β = N (ˆ ˆ + N1 N2 Σ µ2 − µ ˆ1 ) // from (8) = (N − 2)Σ N 3. ˆ B β = (ˆ Σ µ2 − µ ˆ1 )(ˆ µ2 − µ ˆ 1 )T β ˆ B β is in the direction (ˆ (ˆ µ2 − µ ˆ1 )T β is a scalar, therefore Σ µ2 − µ ˆ1 ), and ˆ= β N1 N2 ˆ 1 ˆ −1 Σ N (ˆ µ2 − µ ˆ1 ) − ΣB β // from (18) N −2 N 1 N1 N2 ˆ −1 (ˆ = N− (ˆ µ2 − µ ˆ 1 )T β Σ µ2 − µ ˆ1 ) N −2 N ˆ −1 (ˆ ∝Σ µ2 − µ ˆ1 )
The Element of Statistical Learning – Chapter 4
oxstar@SJTU January 6, 2011
Ex. 4.1 Show how to solve the generalized eigenvalue problem max aT Ba subject to aT Wa = 1 by transforming to a standard eigenvalue problem. Answer W is the common covariance matrix, and it’s positive-semidefinite, so we can define b = W 2 a,
ˆ µ ˆy k = ˆ µ ˆy = gi =k
y ˆi y ˆi
Nk
gi =
= =
gi =k
ˆ T xi B
gi =
Ni ˆ T xi B
ˆTµ =B ˆx k ˆTµ =B ˆx
N N N(1)(2) Nhomakorabea1 N
N
N
(yi − β T xi )
i=1
// from (1)
(3)
xi [β T (xi − x ¯)] =
i=1 i=1 2
xi yi −
1 N
N
yj
j =1
// from (2)(3)
(4)
=
k=1 gi =k 2
xi yk −
N1 y1 + N2 y2 N //
gi =k
Compare (24) with (25), we can find that they are the same rule. But when N1 = N2 , these rules are obviously different. ˆ via linear regression. In detail, let Ex. 4.3 Suppose we transform the original predictors X to Y T −1 T ˆ ˆ Y = X(X X) X Y = XB, where Y is the indicator response matrix. Similarly for any input ˆ T x ∈ RK . Show that LDA using Y ˆ is identical to LDA x ∈ Rp , we get a transformed vector y ˆ=B in the original space. ˆ T x, so we have Proof Transformed vector y ˆ=B