LETTER Communicated by Ad Aertsen On Embedding Synre Chains in a Balanced Network
自然语言处理

自然语言处理2002.11.09中国科学院计算技术研究所1.综述.1.1. 绪论.1.1.1.背景,目标.1.1.1.1. 研究自然语言的动力1.语言是思维的裁体,是人际交流的重要工具。
在人类历史上以语言文字形式记载和流传的知识占到知识总量的80%以上。
就计算机的应用而言,据统计用于数学计算的仅占10%,用于过程控制的不到5%,其余85%左右都是用于语言文字的信息处理。
在这样的社会需求下,自然语言理解作为语言信息处理技术的一个高层次的重要方向,一直是人工智能界所关注的核心课题之一。
2.由于创造和使用自然语言是人类高度智能的表现,因此对自然语言理解的研究也有助于揭开人类智能的奥秘,深化我们对语言能力和思维本质的认识。
.1.1.1.2. 什么是计算语言学计算语言学(Computational Linguistics)指的是这样一门学科,它通过建立形式化的数学模型,来分析、处理自然语言,并在计算机上用程序来实现分析和处理的过程,从而达到以机器来模拟人的部分乃至全部语言能力的目的。
计算语言学(Computational Linguistics)有时也叫计量语言学(Quantitative Linguistics), 数理语言学(Mathematical Linguistics), 自然语言理解(Natural Language Understanding), 自然语言处理(Natural Language Processing), 人类语言技术(Human Language Technology)。
.1.1.1.3. 图灵测验在人工智能界,或者语言信息处理领域中,人们普遍认为可以采用著名的1950年描述的图灵试验(Turing Test )来判断计算机是否“理解”了某种自然语言。
.1.1.1.3.1.Turing模仿游戏(Imitation Game)●场景:男性被试、女性被试、观察者,3者在3个不同的房间,房间号分别为X, Y, O●规则:观察者用电传打字机与被试们通信,男性被试欺骗观察者、女性被试帮助观察者。
数学-科学的王后与仆人
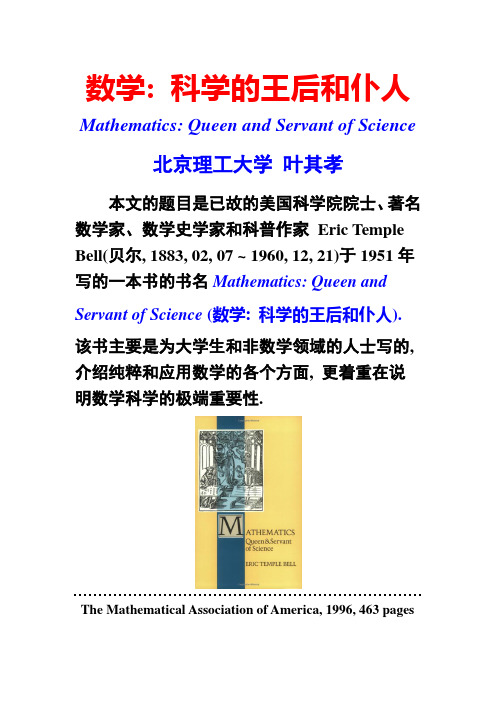
数学: 科学的王后和仆人Mathematics: Queen and Servant of Science北京理工大学叶其孝本文的题目是已故的美国科学院院士、著名数学家、数学史学家和科普作家Eric Temple Bell(贝尔, 1883, 02, 07 ~ 1960, 12, 21)于1951年写的一本书的书名Mathematics: Queen and Servant of Science (数学: 科学的王后和仆人). 该书主要是为大学生和非数学领域的人士写的, 介绍纯粹和应用数学的各个方面, 更着重在说明数学科学的极端重要性.The Mathematical Association of America, 1996, 463 pages实际上这是他1931年写的The Queen of the Sciences (科学的王后)和1937年写的The Handmaiden of the Sciences (科学的女仆)这两本通俗数学论著的合一修订扩大版.Eric Temple Bell Alexander Graham Bell (1847 ~ 1922) 按常识的理解, 女王是优美、高雅、无懈可击、至尊至贵的, 在科学中只有纯粹数学才具有这样的特点, 简洁明了的数学定理一经证明就是永恒的真理, 极其优美而且无懈可击;另一方面, 科学和工程的各个分支都在不同程度上大量应用数学, 这时数学科学就是仆人, 这些仆人是否强有力, 用起来是否得心应手是雇佣这些仆人的主人最为关心的事. 事实上, servant这个字本身就有“供人们利用之物, 有用的服务工具”的意思. 毫无疑问, 我们的目的不是为数学争一个好的名分, 而是想说明数学是怎样通过数学建模来解决各种实际问题的; 数学(数学建模)的极端重要性, 以及探讨正确认识和理解数学科学的作用对于发展我国科学技术、经济以及教育, 从而争取在21世纪把我国真正建设成为屹立于世界民族之林的强国,乃至个人事业发展的至关重要性. 当然, 我们也希望说明王后和仆人集于一身并不矛盾. 历史上, 很多特别受人尊敬的科学家, 不仅仅是由于他们的科学成就, 更因为他们的科学成就能够服务于人类.数学是科学的王后, 算术是数学的王后. 她常常放下架子为天文学和其他科学效劳, 但是在所有情况下, 第一位的是她(数学)应尽的责任. (高斯)Mathematics is the Queen of the Sciences, and Arithmetic the Queen of Mathematics. She often condescends to render service to astronomy and other natural sciences, but under all circumstance the first place is her due.— Carl Friedrich Gauss (卡尔·弗里德里希·高斯, 1777, 4, 30 ~ 1855, 2, 23)From: Bell, Eric T., Mathematics: Queen and Servant of Science, MAA, 1951, p.1;Men of Mathematics, Simon and Schuster, New York, 1937, p. xv.***************************************************自古以来,数学的发展始终与科学技术的发展紧密相连,反之亦然. 首先, 我们来看一下导致我们现在这个飞速发展的信息社会的19、20世纪几乎所有重大科学理论的发展和完善过程中数学(数学建模)所起到的不可勿缺的作用.数学研究的成果往往是重大科学发明的催生素(仅就19、20世纪而言, 流体力学、电磁理论、相对论、量子力学、计算机、信息论、控制论、现代经济学、万维网和互联网搜索引擎、生物学、CT、甚至社会政治学领域等). 但是20世纪上半世纪, 数学虽然也直接为工程技术提供一些工具, 但基本方式是间接的: 先促进其他科学的发展, 再由这些科学提供工程原理和设计的基础. 数学是幕后的无名英雄.现在, 数学无处不在, 数学和工程技术之间,在更广阔的范围内和更深刻的程度上, 直接地相互作用着, 极大地推动了科学和工程科学的发展, 也极大地推动了技术的发展. 数学不仅是幕后的无名英雄, 很多方面开始走向“前台”. 但是对数学的极端重要性迄今尚未有共识, 取得共识对加强一个国家的竞争力来说是至关重要的.硬能力―一位美国朋友谈及对未来中国人的看法: 20年后, 中国年轻人会丢了中国人现在的硬能力, 他们崇拜各种明星, 不愿献身科学, 不再以学术研究为荣, 聪明拔尖的学生都去学金融、法律等赚钱的专业; 而美国人因为认识到其硬能力(例如数学)不行, 进行教育改革, 20年后, 不但保持了其软实力即非专业能力的优势, 而且在硬能力上赶上中国人.‖“正在丢失的硬实力”, 鲁鸣, 《青年文摘》2011年第5期动向:美国很多州新办STEM高中, 一些大学开始开设STEM课程等.STEM = Science + Technology + Engineering + Mathematics2012年2月7日公布的美国总统科技顾问委员会给总统的报告,参与超越:培养额外的100万具有科学、技术、工程和数学学位的大学生(Engage to Excel: Producing One Million Additional College Graduates with Degrees in Science, Technology, Engineering, and Mathematics)The Mathematical Sciences in 2025, the National Academies Press, 2013人们使用的数学科学思想、概念和方法的范围在不断扩大的同时,数学科学的用途也在不断扩展. 21世纪的大部分科学与工程将建立在数学科学的基础上.This major expansion in the uses of the mathematical sciences has been paralleled by a broadening in the range of mathematical science ideas and techniques being used. Much of twenty-first century science and engineering is going to be built on a mathematical science foundation, and that foundation must continue to evolve and expand.数学科学是日常生活的几乎每个方面的组成部分.互联网搜索、医疗成像、电脑动画、数值天气预报和其他计算机模拟、所有类型的数字通信、商业和军事中的优化问题以及金融风险的分析——普通公民都从支撑这些应用功能的数学科学的各种进展中获益,这样的例子不胜枚举.The mathematical sciences are part of almost every aspect of everyday life. Internet search, medical imaging, computer animation, numerical weather predictions and othercomputer simulations, digital communications of all types, optimization in business and the military, analyses of financial risks —average citizens all benefit from the mathematical science advances that underpin these capabilities, and the list goes on and on.调查发现:数学科学研究工作正日益成为生物学、医学、社会科学、商业、先进设计、气候、金融、先进材料等许多研究领域不可或缺的重要组成部分. 这种研究工作涉及最广泛意义下数学、统计学和计算综合,以及这些领域与潜在应用领域的相互作用. 所有这些活动对于经济增长、国家竞争力和国家安全都是至关重要的,而且这种事实应该对作为整体的数学科学的资助性质和资助规模产生影响. 数学科学的教育也应该反映数学科学领域的新的状况.Finding: Mathematical sciences work is becoming an increasingly integral and essential component of a growing array of areas of investigation in biology, medicine, social sciences, business, advanced design, climate, finance, advanced materials, and many more. This work involves the integration of mathematics, statistics, and computation in the broadest sense and the interplay of these areas withareas of potential application. All of these activities are crucial to economic growth, national competitiveness, and national security, and this fact should inform both the nature and scale of funding for the mathematical sciences as a whole. Education in the mathematical sciences should also reflect this new stature of the field.****************************************************************为了以下讲述的方便, 我们先来了解一下什么是数学建模.数学模型(Mathematical Model)是用数学符号对一类实际问题或实际发生的现象的(近似的)描述.数学建模(Mathematical Modeling)则是获得该模型并对之求解、验证并得到结论的全过程.数学建模不仅是了解基本规律, 而且从应用的观点来看更重要的是预测和控制所建模的系统的行为的强有力的工具.数学建模是数学用来解决各种实际问题的桥梁.↑→→→→→→→→↓↑↓↑↓↓↑↓←←←←←通不过↓↓通过)定义:数学建模就是上述框图多次执行的过程数学建模的难点观察、分析实际问题, 作出合理的假设, 明确变量和参数, 形成明确的数学问题. 不仅仅是翻译的问题; 涉及的数学问题可能是复杂、困难的, 求解也许涉及深刻的数学方法. 如何作出正确的判断, 寻找合适、简洁的(解析或近似) 解法; 如何验证模型.简言之:合理假设、模型建立、模型求解、解释验证.记住这16个字, 将会终生受用.数学建模的重要作用:源头创新当然数学建模也有局限性, 不能单独包打天下, 因为实际问题是非常复杂的, 需要多学科协同解决.在图灵(A. M. Turing)的文章: The Chemical Basis of Morphogenesis (形态生成的化学基础), Philosophical Transactions of the Royal Society of London (伦敦皇家学会哲学公报), Series B (Biological Sciences),v.237(1952), 37-72.1. 一个胚胎的模型. 成形素本节将描述一个正在生长的胚胎的数学模型. 该模型是一种简化和理想化, 因此是对原问题的篡改. 希望本文论述中保留的一些特征, 就现今的知识状况而言, 是那些最重要的特征.1. A model of the embryo. MorphogensIn this section a mathematical model of the growing embryo will be described. This model will be asimplification and an idealization, and consequently a falsification. It is to be hoped that the features retained for discussion are those of greatest importance in the present state of knowledge.想单靠数学建模本身来解决重大的生物学问题是不可能的,另一方面,想仅仅依靠实验来获得对生物学的合理、完整的理解也是极不可能的. There is no way mathematical modeling can solve major biological problems on its own. On the other hand, it ishighly unlikely that even a reasonably complete understanding could come solely from experiment.—— J. D. Murray, Why Are There No 3-Headed Monsters? Mathematical Modeling in Biology, Notices of the AMS,v. 59 (2012), no. 6, p.793.自古以来公平、公正的竞赛都是培养、选拔人才的重要手段, 科学和数学也不例外.中学生IMO (国际数学奥林匹克(International Mathematical Olympiad), 1959 ~)北美的大学生Putnbam数学竞赛(1938 ~)全国大学生数学竞赛(2010 ~)Mathematical Contest in Modeling (MCM, 1985 ~)美国大学生数学建模竞赛Interdisciplinary Contest in Modeling (ICM, 1999~)美国大学生跨学科建模竞赛China Undergraduate Mathematical Contest in Modeling (CUMCM, 1992~) 中国大学生数学建模竞赛中国大学生参加美国大学生数学建模竞赛情况中国大学生数学建模竞赛情况在以下讲述中涉及物理方面的具体的数学模型 (问题)的叙述和初步讨论可参考《物理学与偏微分方程》, 李大潜、秦铁虎编著, (上册, 1997; 下册, 2000), 高等教育出版社.Seven equations that rule your world (主宰你生活的七个方程式), by Ian Stewart, NewScientist, 13 February 2012.Fourier transformation 2ˆ()()ix f f x e dx πξξ∞--∞=⎰Wave equation 22222u u c t x ∂∂=∂∂ Ma xwell‘s equation110, , 0, H E E E H H c t c t∂∂∇⋅=∇⨯=-∇⋅=∇⨯=∂∂Schrödinger‘s equation ˆψH ψi t∂=∂Ian Stewart, In Pursuit of the Unknown:17 Equations That Changed the World (追求对未知的认识:改变世界的17个方程), Basic Books, March 13, 2012.目录(Contents)Why Equations? /viii1. The squaw on the hippopotamus ——Pythagoras‘sTheorem/12. Shortening the proceedings —— Logarithms/213. Ghosts of departed quantities —— Calculus/354. The system of the world ——Newton‘s Law ofGravity/535. Portent of the ideal world —— The Square Root ofMinus One/736. Much ado about knotting ——Euler‘s Formula forPolyhedra/837. Patterns of chance —— Normal Distribution/1078. Good vibrations —— Wave Equation/1319. Ripples and blips —— Fourier Transform/14910. The ascent of humanity —— Navier-StokesEquation/16511. Wave in the ether ——Maxwell‘s Equations/17912. Law and disorder —— Second Law ofThermodynamics /19513. One thing is absolute —— Relativity/21714. Quantum weirdness —— Schrödinger Equation/24515. Codes, communications, and computers ——Information Theory/26516. The imbalance of nature —— Chaos Theory/28317. The Midas formula —— Black-Scholes Equation/195Where Next?/317Notes/321Illustration Credits/330Index/331相对论Albert Einstein(1879, 3, 14 ~1955, 4, 18)20世纪最伟大的科学成就莫过于Einstein(爱因斯坦)的狭义和广义相对论了, 但是如果没有Minkowski (闵可夫斯基)几何、Riemann(黎曼)于1854年发明的Riemann几何, 以及Cayley(凯莱), Sylvester(西勒维斯特)和Noether(诺特)等数学家发展的不变量理论, Einstein的广义相对论和引力理论就不可能有如此完善的数学表述. Einstein自己也不止一次地说过.早在1905年, 年仅26岁的爱因斯坦就已提出了狭义相对论. 狭义相对论推倒了牛顿力学的质量守恒、能量守恒、质量能量互不相关、时空永恒不变的基本命题. 这是一场真正的科学革命.为了导出狭义相对论,爱因斯坦作出了两个假设:运动的相对性(所有匀速运动都是相对的)和光速为常数(光的运动例外, 它是绝对的). (1)狭义相对性原理,即在所有惯性系中, 物理学定律具有相同的数学表达形式;(2)光速不变原理,真空中光沿各个方向传播的速率都相等,与光源和观察者的运动状态无关.时空不是绝对独立的.由此可以导出一些推论: 相对论坐标变换式和速度变换式, 同时的相对性, 钟慢尺缩效应和质能关系式等.他的好友物理学家P.Ehrenfest指出实际上还蕴涵着第三个假设, 即这两个假设是不矛盾的. 物体运动的相对性和光速的绝对性, 两者之间的相互制约和作用乃是相对论里一切我们不熟悉的时空特征的根源.(部分参阅李新洲:《寻找自然之律--- 20世纪物理学革命》, 上海科技教育出版社, 2001.)1907 年德国数学家H. Minkowski (1864 ~1909) 提出了―Minkowski 空间‖,即把时间和空间融合在一起的四维空间1,3R. Minkowski 几何为Einstein 狭义相对论提供了合适的数学模型.“没有任何客观合理的方法能够把四维连续统分离成三维空间连续统和一维时间连续统. 因此从逻辑上讲, 在四维时空连续统(space- time continuum)中表述自然定律会更令人满意. 相对论在方法上的巨大进步正是建立在这个基础之上的, 这种进步归功于闵可夫斯基(Minkowski).”—Albert Einstein, The Meaning of Relativity, 1922, Princeton University Press. 中译本, 阿尔伯特·爱因斯坦著, 相对论的意义, (普林斯顿科学文库(Princeton Science Library) 1), 郝建纲、刘道军译, 上海科技教育出版社, 2001, p. 27.有了Minkowski 时空模型后, Einstein 又进一步研究引力场理论以建立广义相对论. 1912 年夏他已经概括出新的引力理论的基本物理原理, 但是为了实现广义相对论的目标, 还必须寻求理论的数学结构, Einstein 为此花了 3 年的时间, 最后, 在数学家M. Grossmann 的介绍下学习掌握了发展相对论引力学说所必需的数学工具—以Riemann几何和Ricci, Levi - Civita的绝对微分学, 也就是Einstein 后来所称的张量分析.“根据前面的讨论, 很显然, 如果要表达广义相对论, 就需要对不变量理论以及张量理论加以推广. 这就产生了一个问题, 即要求方程的形式必须对于任意的点变换都是协变的. 在相对论产生以前很久, 数学家们就已经建立了推广的张量演算理论. 黎曼(Riemann)首先把高斯(Gauss)的思路推广到了任意维连续统, 他很有预见性地看到了……进行这种推广的物理意义. 随后, 这个理论以张量微积分的形式得到了发展, 对此里奇(Ricci)和莱维·齐维塔(Tulio Levi-Civita, 1873~1941)做出了重要贡献. ”—阿尔伯特·爱因斯坦著, 相对论的意义, 郝建纲、刘道军译, 上海科技教育出版社, 2001, p. 57.从数学建模的角度看, 广义相对论讨论的中心问题是引力理论, 其基础是以下两个假设: 1. (等效原理)惯性力场与引力场的动力学效应是局部不可分辨的,(或说引力和非惯性系中的惯性力等效);2. (广义相对性原理) 一切参考系都是平权的,换言之,客观的真实的物理规律应该在任意坐标变换下形式不变——广义协变性(即一切物理定律在所有参考系[无论是惯性的或非惯性的]中都具有相同的形式)。
四面体通讯

四面体通讯。
第35,第46 卷。
第8609 8612,1994EIsevier 科学有限公司打印在中大Britatn Cyclopolyamines:合成的Cyclospermidines 和Cyclospermines,亚精胺和精胺类似物家伙品牌、和平号空间站Wais Iiosseini *和罗曼·鲁珀特Laboratoire de 法国碳化德协调Oqanique,assock au 碳纳米管。
4 研究所Le 比利时、路易·巴斯德贫困生。
rw 帕斯卡尔·布莱克,F 67000 Shasbourg。
法国摘要:合成一系列的循环ataogues natmally occtming多胺多胺,spexmidine和apermine实现。
循环类似物腐命名cycloptttrescine,荷兰组成cycloputtescine在whiih 两家主要atnines相连的亚甲基链可变长度(不适用= 3,4.5。
cycloapermidincs [ 4rl和cyclospaminesi4.111 wexe组成一个cycloptmescine点。
)基团的单或双—功能研发与氨链分别。
在各种生物多胺,PUN- ES &E 。
亚精胺,精胺是无处不在种类繁多生物材虽然,spennine 首次分离出人类的精液列文虎克早1677年,为sperrnine磷酸盐结晶,只是在最近几年很大的关注主要集中于自然发生的聚胺和它们的类似物。
自20世纪70年代初。
用这些化合物处理的文献有大幅增长。
腐胺,亚精胺和精胺被发现在各种各样的动物,细菌,酵母和植物,并与一个巨大的多种生物活性。
例如,它们已被提出了稳定,如E-共同/ 2,分离的线粒体3球状体的膜,以促进转染噬菌体DNA E-大肠杆菌spheroplasts.4的和调节膜功能5 。
他们中也扮演着重要的角色增殖processes.6特别是,它们被证明是参与肿瘤生长的过程,1.6以及,在化学carcinogenesis.7在这方面,一个有趣的观察是在分裂细胞中,在特别是在癌变细胞,多胺的水平如腐胺,亚精胺和精胺显着高于在其他细胞。
知识图谱构建技术综述
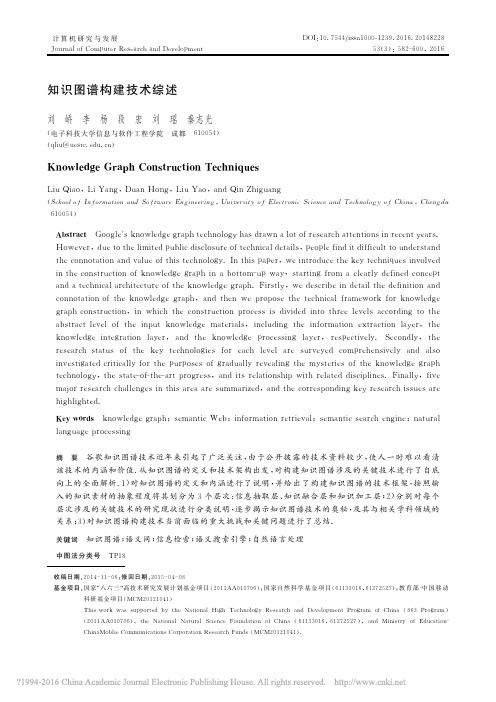
修回日期 : - - - - 2 0 1 4 1 1 0 6; 2 0 1 5 0 4 0 8 收稿日期 : ) ; ; 国家 “ 八六三 ” 高技术研究发展计划基 金 项 目 ( 国家自然科学基金项目( 教 育 部 -中 国 移 动 2 0 1 1 AA 0 1 0 7 0 6 6 1 1 3 3 0 1 6, 6 1 2 7 2 5 2 7) 基金项目 : ) 科研基金项目 ( MCM 2 0 1 2 1 0 4 1 T h i s w o r k w a s s u o r t e d b t h e N a t i o n a l H i h T e c h n o l o R e s e a r c h a n d D e v e l o m e n t P r o r a m o f C h i n a( 8 6 3P r o r a m) p p y g g y p g g ( ) , ,a 2 0 1 1 AA 0 1 0 7 0 6 t h e N a t i o n a l N a t u r a l S c i e n c e F o u n d a t i o n o f C h i n a( 6 1 1 3 3 0 1 6, 6 1 2 7 2 5 2 7) n d M i n i s t r o f E d u c a t i o n - y ) C h i n a M o b l i e C o mm u n i c a t i o n s C o r o r a t i o n R e s e a r c h F u n d s( MCM 2 0 1 2 1 0 4 1 . p
在学术界清华大学建成了第1个大规模中英文跨语言知识图谱xlore中国科学院计算技术研究所基于开放知识网络openkn建立了人立方事立方知立方原型系统中国科学院数学与系统科学研究院陆汝钤院士提出知件knowware的概念上海交通大学构建并发布了中文知识图谱研究平台zhishime复旦大学gdm实验室推出的中文知识图谱项目等2这些项目的特点是知识库规模较大涵盖的知识领域较广泛并且能为用户提供一定的智能搜索及问答服务
系列讲座之十七(Albert S. C. Chan)
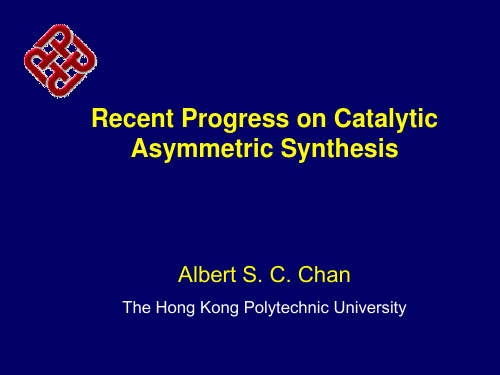
O R1
O OR2
Ru(S-P-Phos)Cl2(DMF)n 50 psi H2
OH O R1 * OR2
P 2
up to 99% ee
R1 = Ph, R2 = Et: 95% ee (BINAP: 85% ee)
(R)-P-Phos
Ru(S-P-Phos)(acac)2, 1000 psi H2 CO2H MeO H3PO4/Sub = 0.6 (M/M) Sub/Cat = 200 (M/M) MeO Me CO2H
(S)-Monophos
(1S,1'S,2R,2'R)-Tangphos
H3CH2C
R P P R R P R O O O P O H3CH2C P CH2CH3 Fe
P CH2CH3
R = Me, (R,R)-Me-Duphos R = Et, (R,R)-Et-Duphos R = i-Pr, (R,R)-iPr-Duphos
[Rh(NBD)2]BF4 (1R,1’R,2S,2’S)-Tangphos
100 1000 20 15 45 (R)
100 1000 24 4 ND
100 1000 48 7 62 (S)
Recent Progress on Catalytic Asymmetric Synthesis
Albert S. C. Chan
The Hong Kong Polytechnic University
Louis Pasteur Chemist 1822 - 1895
“Chance favors only the prepared mind.”
含吡啶基手性膦配体铜配合物催化下芳香酮的不对称硅氢化反应
量子信息概论 by郭光灿
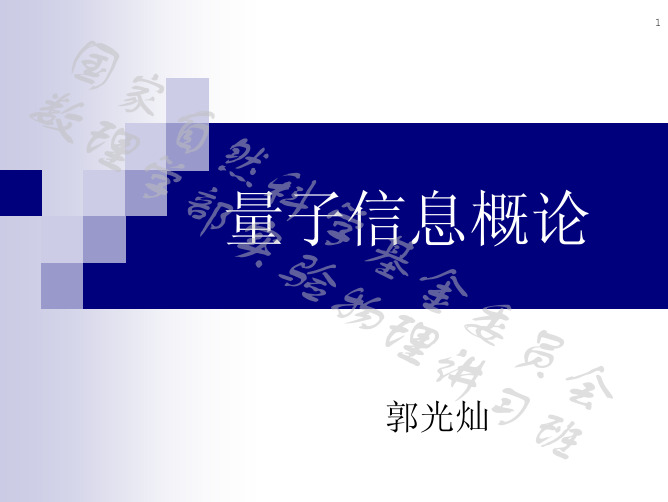
1⎤ − 1⎥⎦
业已证明,任意么正矩阵可做如下分解
物 委 其作用: 理 员 H 0 = 1 ( 0 + 1 )
U
=
eiα
⎡e−iβ /
⎢ ⎣
0
2
0⎤
eiβ
/
2
⎥ ⎦
⎢⎣⎡csions2r2r
−csoisn2r2r ⎥⎦⎤
⎡e−iδ /2
⎢ ⎣
0
0⎤
eiδ
/
2
⎥ ⎦
讲 会 2
式中为实数,注意:第二个矩阵为普
理讲 员 等效表示:ψ = cosθ 0 + eiϕ sin θ 1
会 2
2
习班 式中θ,ϕ为实数,θ和ϕ定义单位三维球面
上的一个点。Bloch球。
3
国
量子比特的物理载体:任意二态的量子体
数 家自 系,如光子、原子、电子、原子核等。 理学 然 一个量子比特表示多少信息? 部实科学 若对 ψ 进行一次测量,只能给出0或1,量子 验 基 比特的测量后的态为 0 或 1 。因此,从一次 物 金委 测量,人们只能获得关于量子比特态的一个 理讲 员 比特的信息。 习班会 X如若不进行测量,一个量子比特代表多少
班 业已证明:任意多量子比特门均可以由CNOT和单量
子比特门构成。
12
国 (3)基于非计算基的测量
家 量子比特 ψ = α 0 + β 1 数 自 采用基矢 0 , 1 进行测量,结果为 0 和 1 的几率分别为α 2 理学 然 和 β 2。
部 科 计算基并非是唯一的测量基,例如,可以选择另组正交基:
习 H 1 = 1 ( 0 − 1 )
通旋转矩阵,第一、三矩阵为绕围Z轴
- 1、下载文档前请自行甄别文档内容的完整性,平台不提供额外的编辑、内容补充、找答案等附加服务。
- 2、"仅部分预览"的文档,不可在线预览部分如存在完整性等问题,可反馈申请退款(可完整预览的文档不适用该条件!)。
- 3、如文档侵犯您的权益,请联系客服反馈,我们会尽快为您处理(人工客服工作时间:9:00-18:30)。
LETTER Communicated by Ad Aertsen On Embedding Syn re Chains in a Balanced NetworkY.Avielaviel@cc.huji.ac.ilInterdisciplinary Center for Neural Computation,Hebrew University, Jerusalem,IsraelC.MehringMehring@biologie.uni-freiburg.deNeurobiology and Biophysics,Institute of Biology III,Albert-Ludwigs-University, Freiburg,GermanyM.Abelesabeles@hbf.huji.ac.ilInterdisciplinaryCenter for Neural Computation,Hebrew University,Jerusalem,Israel D.Hornhorn@post.tau.ac.ilSchool of Physics and Astronomy,Tel Aviv University,Tel Aviv,IsraelWe investigate the formation of syn re waves in a balanced network of integrate-and- re neurons.The synaptic connectivity of this network em-bodies syn re chains within a sparse random connectivity.This network can exhibit global oscillations but can also operate in an asynchronous activity mode.We analyze the correlations of two neurons in a pool as con-venient indicators for the state of the network.We nd,using different models,that these indicators depend on a scaling variable.Beyond a critical point,strong correlations and large network oscilla-tions are obtained.We looked for the conditions under which a syn re wave could be propagated on top of an otherwise asynchronous state of the network.This condition was found to be highly restrictive,requiring a large number of neurons for its implementation in our network.The results are based on analytic derivations and simulations.1IntroductionSyn re chains(SFCs)were introduced by Abeles(1991)as a model for solv-ing a wide array of cognitive and computational tasks.They incorporate rate coding with speci c synchronous activity and have been shown to be can-didates for representing elementary cognitive functions(Bienenstock,1995)Neural Computation15,1321–1340(2003)c°2003Massachusetts Institute of Technology1322Y.Aviel,C.Mehring,M.Abeles,and D.HornFigure1:The connectivity of a syn re chain.The connection between three pools is shown.In the complete chain,each neuron in a pool receives inputs from all w neurons in the pre-pool(w D3in this gure).In addition,each neuron receives inputs from(and projects to)many other neurons in the network.Note that a neuron can participate in many pools.such as binding(Hayun,2002).An SFC dictates a well-de ned connectivity pattern among neurons in the form of feedforward connections between pools of neurons.In a complete chain,all w neurons in a pool(“pre-pool”) connect to all neurons in the successive pool,thus creating a chain of pools. Input connections as well as outputs are allowed,as shown in Figure1.If w,the number of neurons in a pool,is large enough,then a synchronized ring volley of most of the neurons in a pool,a pulse packet,may propagate along the chain(Diesmann,Gewaltig,&Aertsen,1999).To avoid termino-logical confusion,these feedforward connectivity schemes are referred to henceforth as chains and the pulse packet propagating along a chain as a syn re wave(or simply a wave).A wave can propagate in a synchronized manner along a chain,or it can lose its synchrony,dissolving into the back-ground activity.A wave is said to be stable if it reaches the end of the chain as a synchronized pulse packet.We begin by focusing on a chain that is embedded in a large network, whose neurons produce some asynchronous low background activity.Each neuron in the chain receives occasional pulse packets from its pre-pool,asSyn re Chains in a Balanced Network1323 well as some background activity.What are the effects of the background activity on the waves?Can waves travel along chains in a stable manner in the presence of background noise,or will they eventually dissolve into the background activity?Can the background noise cause spontaneous emer-gence of waves along chains?Diesmann et al.(1999)have shown that if the number of neurons in a pool is large enough and if the igniting pulse packet is synchronized and strong enough,the waves are stable in the presence of background noise.Naturally,the next question is whether we can model a network that is capable of low spontaneous asynchronous activity with similar properties of a cortical tissue that is also capable of hosting stable syn re waves.In such a network,the effect of the background activity on the wave is important, but the effect of the chain on the asynchronous state of the network is also crucial.In this letter,we investigate these issues by studying the correlations of two neurons that belong to the same pool.We show that the correlations allow us to distinguish between the different states of the network activity. High correlations occur when the system is in an oscillatory state,one where a syn re wave will be lost in the background of spontaneous activity.The challenge is to nd the appropriate parameters such that stable waves exist in a background of asynchronous global activity and irregular spiking of individual neurons.This problem arises from the existence of two constraints:on one hand, the system is required to maintain a stable asynchronous state,thus en-abling rate coding,and on the other hand,we require the network to allow synchronous propagation of pulse packets—a kind of temporal code.Are these two modes mutually exclusive?As we shall see,it is possible to set up a network with physiological and anatomical realistic parameters that is capable of operating in both modes simultaneously.In the context of the two-neuron problem,we use a simple,analytically amenable model that enables us to write an equation that describes the evo-lution of correlation along a chain.This equation is independent of the input ring rate and leads to the emergence of a new scaling variable.A more re-alistic integrate-and- re neuronal model does show dependence on ring rates.Nevertheless,we nd that the scaling variable still functions as a crit-ical parameter.Moreover,the same holds not only for a pair of neurons,but also in the full network simulation.Our analysis,which is based on correla-tions,suf ces for the discussion of the issues that we confront;hence,we do not delve into the evolution of ring rates(for the latter,see Tetzlaff,Geisel,& Diesmann,2002).Experimentally,neuron activity is typically characterized by steady low (2–5Hz) ring rates,with irregular spiking(Abeles,1991).To accommodate this observation with the known anatomical fact(Abeles,1991)that there can be many inputs(around20,000excitatory and2000inhibitory synapses) to a neuron,it was suggested(Shadlen&Newsome,1994;Gerstein&Man-1324Y.Aviel,C.Mehring,M.Abeles,and D.Horn delbrot,1964)that excitatory and inhibitory inputs cancel each other out, thus reducing the mean input to virtually zero.Thus,the ring of a neuron re ects uctuations of the membrane potential that elicit occasional crossing of the threshold.Another solution(Softky&Koch,1993)has been to argue that temporal correlation of the excitatory postsynaptic potentials(EPSPs) elicit neuronal ring.We consider a system that allows both solutions to co-exist.First,the input is balanced,and second,waves of activity propagate by means of strong temporal correlation.To create large uctuations with a constant mean,we balance the ex-citatory input with an inhibitory input.A network is said to be balanced (van Vreeswijk&Sompolinsky,1998)if each neuron in it receives equal amounts of excitation and inhibition.Its membrane potential will then uc-tuate around some mean value and the ring process is noise driven,and therefore irregular.Balanced networks(BN)have been shown(Brunel,2000) to mimic the in vivo ring statistics of cortical tissue,and it is therefore plau-sible that cortical neurons receive balanced input.BN has also been shown (van Vreeswijk&Sompolinsky,1998)to have a stable asynchronous state,as well as appropriate rate coding properties,such as fast tracking of changes in external input rates.Generally,BN assumes sparse and random connectivity.It is possible to embed SFCs in the excitatory-to-excitatory(E-E)connections of a BN,but this would violate the random connectivity assumption.Would this also disrupt the desired properties of a BN?As we show,there is a wide regime of parameters in which such lack of randomness has little effect.2The ModelFollowing Brunel(2000),we use an integrate-and- re(IF)model,in which the i th neuron’s membrane potential,V i.t/,obeys the equation¿dV i.t/dtD¡V i.t/C RI i.t/;(2.1)where I i.t/is the synaptic current arrivingat the soma and R is the membrane resistance.Spikes are modeled by delta functions;hence,the input is written asRI i.t/DXj Xt fjJ ij±.t¡t f j¡D/;(2.2)where the rst sum is over different neurons,whereas the second sum rep-resents their spikes arriving at times t D t fj¡D¢t f j is the emission time of the f th spike by neuron j,and D is a transmission delay,which we assume here to be the same for any pair of neurons.The sum is over all neurons that project their output to neuron i,both local and external afferents.Syn re Chains in a Balanced Network1325 When V i.t/reaches the ring thresholdµ,an action potential is emitted by neuron i,and after a refractory period¿rp,during which the potential is insensitive to stimulation,the depolarization is reset to V reset.The following parameters were used in all simulations:the transmission delay D D1:5ms,the thresholdµD20mV,the membrane time constant¿D 10ms,the refractory period¿rp D0:5ms,the resetting potential V reset D0 mV,and the membrane resistance R D40MÄ.The inhibitory and excitatory neurons have identical parameters.We used the SYNOD simulation environment(Diesmann,Gewaltig,& Aertsen,1995)for simulations with fewer than10,000neurons and a parallel version of SYNOD for simulation with more neurons.In the simulator,the Lapique(Tuckwell,1988)model was used as an IF model with time steps of0.1ms.Unless otherwise speci ed,we set the synaptic weights J IE D J EE D J;J EI D J II D¡gJ with g D5and J D0:14mV.The constant g is the relative strength of the inhibitory synapses,and J is the EPSP amplitude. Note that the synapses are weak,as J¿µ,and the total input to a neuron is approximately balanced.When simulating a pair of neurons in a pool,the neurons receive local excitatory and inhibitory input from the network as well as external input from external excitatory sources that feed the entire network.For simplic-ity,we assume that the neurons have a single pre-pool.Thus,the neurons receive four types of input,three of which are local:K I inhibitory synapses, w excitatory synapses that come from the previous pool,and K¡w excita-tory synapses from other random connections in the network.The fourth type of input is represented by K excitatory synapses that convey external stimulation.Each of these inputs is modeled as an independent Poisson process with rates v I,v E,and v ext for the local inhibitory,local excitatory, and external excitatory synapses,respectively.In the simple model,which will be de ned later,we re ne the architecture further to include common inputs due to random connections.As a method for inducing correlations between spike trains,we use a“mother process”:a Poisson process with rates v=½in,from which we copy spikes with probability½in.The method is described in detail in Kuhn,Rotter,and Aertsen(in press).In order to mea-sure the output correlation between two spike trains,we rst binned the spike train into1ms bins(each spike is of width0.1ms)and then computed the zero-lag cross-correlation.When simulating the entire network,we simulate all the N E excitatory neurons,as well as the N I D N E=4inhibitory neurons.A Poisson process with rate v ext K simulates the external input.v ext is in units of v thre,where v thre´µJK¿is the minimal rate needed to emit a spike within¿milliseconds (on the average)in a neuron that does not get other inputs.In this article, we setºext D1:5ºthre,which is equivalent to an external rate that ranges between2Hz and21Hz,depending on the value of K.The total input to a neuron is therefore nearly balanced.There is a small bias toward an excess of excitation,which controls the ring rates.1326Y.Aviel,C.Mehring,M.Abeles,and D.HornA sparse connectivity is required to both adhere as closely as possible to biological values and induce a source of randomness.Here we set the sparseness"to be0.1,that is,K D"N E,K I D"N I.In addition to the ex-ternal input(which are K excitatory afferents),each neuron in the network receives K excitatory and K I inhibitory afferents,randomly sampled,from the excitatory and inhibitory population,respectively.Wiring the network is performed as follows.In general,all the connec-tions are chosen at random,except for the E-E connections,which are a superposition of chains.For wiring the E-E connections,each randomly chosen pool of w neurons is wired in an all-to-all fashion to the consecutive pool.We continue this process until the desired number of pools has been reached.We also ensure that a neuron will not receive more than K local connections.If a neuron does not receive K afferents and there are no more pools to be added,we randomly choose additional source neurons for it. At the end of the process,all the excitatory neurons have exactly K affer-ents,and there are chains hardwired into the connectivity matrix of the E-E connections.The resulting connectivity is a mixture of random connectivity and chains. The number of pools is restricted by the number of excitatory synapses and is therefore less than N E Kw2C1.In our simulation,we set the number of pools at1000.If this was not possible due to the above limit,the simulation wasnot performed.A tighter bound on the number of pools is given by the capacity of the system,as discussed in section6.3The ChallengeThe foregoing enabled us to pursue the following scenario.We start with a BN that embodies chains in its connectivity matrix,as described in the previous section.In the absence of external ignition of a wave,we consider the state with global asynchronous activity to be the ground state of the system.External ignition of an SFC will perturb the system from its ground state,leading to wave propagation,masked by the overall activity.Once the ignited wave has reached the end of the chain,the system should return to its ground state.Brunel(2000)has shown that BNs of IF neurons display four major stable states for different regimes of the external input rate,ºext,and the level of balance.The four states are all combinations of the two possible global (population-level)activities and the two possible neuronal ring patterns; the global activity can be either synchronous or asynchronous,whereas the neurons can re in a regular or irregular fashion.Of special interest to us is the state that is characterized by asynchronous population activity and irregular neuronal rings.Following Brunel’s terminology,this is the asynchronous-irregular(AI)state.Syn re Chains in a Balanced Network1327(a)Time [ms]P o p u l a t i o n r a t e(b)Time [ms]P o p u l a t i o n r a t e Figure 2:Population rate of a BN with embedded SFCs indicates a qualitative change in network behavior,as chain width increases.(a)With a chain of width w D 150,the network is in an AI state,characterized by low asynchronous population activity and very mild oscillations with small amplitudes.Average ring rate of the excitatory (inhibitory)neurons in this mode is 12.9Hz (25.7Hz).(b)Increasing the width to w D 250,a synchronous state appears,characterized by sporadic,abrupt synchronized bursts with large amplitudes.The average ring rate of the excitatory (inhibitory)neurons is 36Hz (73Hz).The population rate is the percentage of excitatory neurons that red in 0.2ms.The network parameters are N E D 50,000,K D 5000,number of pools:1000.The network model described in section 2is identical to Brunel’s model except for the connectivity:in Brunel’s model the connectivity is completely random,whereas we embody chains in the network.Another difference is in the dynamic parameters.Brunel varied ºext and g in order to obtain different states;here we vary only the chain width,keeping ºext ,and g constant.As shown in Figure 2a,a BN that embodies chains displays behavior similar to that of Brunel’s AI state (Brunel,2000).This suggests that a small departure from randomness does not refute the results obtained for random networks.Consequently,we use the AI state as the ground state of our system.We search through parameter space to nd this regime in our model.To allow stable propagation of a wave along a chain,we need large pools (Diesmann et al.,1999).However,when w is too large,as is the case in Figure 2b,the AI state loses its stability,with a transition similar to the phase transition that takes place in Brunel’s model when moving from the AI state to the synchronous-irregular (SI)state.In the SI state,as in Figure 2b,the population activity is synchronous,and the neurons re irregularly.(It should be noted,however,that the global oscillations in our case exhibit stronger irregularity than the one of Brunel’s SI state.See Figure 8there.)In the next section,we will see that the transition is due to high correlations between neurons with a relatively large common input.The challenge is to construct a network in which w is large enough to allow stable wave formation yet is not too large to destabilize the asynchronous state.1328Y.Aviel,C.Mehring,M.Abeles,and D.Horn 4A Pair of NeuronsAs hinted above,we suspect that in the case of large w ,the embedded chains stimulate the emergence of correlated spike trains.To see this,consider a pool in a chain.The converging connections from the pre-pool to all the neurons in the pool induce an input that is common to all the neurons in that pool,possibly leading to correlationsamong these neurons’spike trains.This surplus of correlations underlies the instability of the asynchronous state in the full network.We start by studying the effect of common inputs on a pair of neurons that belong to the same pool of an embedded chain.In particular,we are interested in the correlation coef cient between the spike trains of a pair of neurons in a pool,½out .In Figure 3,we describe schematically a pair of neurons and their inputs.Being part of the same pool,they share a common input of at least w neurons from the previous pool (E cc )and some other common input due to random connectivity (E c and I c ).They also receive excitatory (E)and inhibitory (I)inputs that are independent for the two neurons.For simplicity ,we assume that all inputs are uncorrelated,except for the w common input (E cc )that have a pairwise correlation coef cient ½in .We will de ne ½h to be the correlation coef cient between the two mem-brane potentials.The effect of the common input,w ,on the correlation be-tween two neurons,½out ,can be studied in two steps:1.The effect of the commoninput on the correlationof the two membrane potentials:½h .w ;K ;½in /.2.The effect of the correlated membrane potentials on the correlation of the neurons’output:½out .½h /.We start with a simpli ed neuronal model,in which a natural scaling of w =p K emerges,and a transition in the level of correlations is found.We then proceed to an IAF model,where we nd similar qualitative results.4.1A Simple Model.In the appendix,we de ne a semilinear model,the simple model,leading to an analytic expression of ½h .w ;K ;½in /:½h .½in /D .1C g 2/"K C w 2½in .2C g 2/K C w 2½in :(4.1)De ning q ´.2C g 2/,and dividing the nominator and denominator by K yields½h D.q ¡1/"C r 2½in ½;(4.2)where r ´w p K is the scaling variable of this system.Syn re Chains in a Balanced Network1329Figure3:Two neurons of the same pool receive common input;the total input to each neuron is divided into ve uncorrelated sub elds:independent inhibitory and excitatory(E and I),common inhibitory and excitatory(E c and I c),and cor-related common excitatory(E cc)from the previous pool.Solid lines represent excitatory inputs and dashed lines inhibitory inputs.Expressions in parentheses denote the number of synapses that establish each type of input.The total num-ber of excitatory inputs is2K,and the total number of inhibitory inputs that each neuron receives is K.Both neurons receive,on average,common input from u excitatory and u inhibitory neurons.The pairwise correlation assumed among inputs in E cc,½in,affects the correlation between the pair of output neurons,½out.1330Y.Aviel,C.Mehring,M.Abeles,and D.Horn (a)(b)r F i x e d -p o i n t c o r r e l a t i o n r *(r ) Figure 4:Transition in the simple model.(a)½out as a function of ½in for different values of r :1,2,3,and 10.The curves cross the diagonal (dashed line)exactly once,creating a globally stable xed point.(b)The steady-state correlation (solid line,"D 0:1)exhibits a transition from low to high correlation near the critical point,r c (dashed line).In the limit "!0,the transition becomes sharp (dash-dotted line).Parameters:K D 10,000,g D 1.Note that ½h is not a function of the input or output rates in this model.Also note that for r D 0and ">0,½h is always positive.That means that even a pair of neurons with common input that is due to only the random connectivity tends to correlate.In a network where a pattern of connectivity as in Figure 3is abundant,½out of a pair of neurons is likely to be ½in for another pair of neurons.An example of such a network is one with chains hardwired into its connectivity matrix.In such cases,it is relevant to seek a xed point of pairwise correlations,½¤,that obeys ½out D ½in D ½¤.As argued in the appendix,under the constraint of equation A.5,½out D ½h ;thus,it is straightforward to look for such a xed point.In Figure 4a,the ½out .½in /is plotted for several values of r .We note that ½out .½in /crosses the diagonal with slope less than 1exactly once,for all values of r ,resulting in a globally stable xed point:½¤.We calculate ½¤from equation 4.2by letting all ½variables to be equal to ½¤and taking the positive solution:½¤D r 2¡q C p 22C 4.q ¡1/"r 2:(4.3)Two important observations follow from this equation.One is that the result is independent of the ring rate,which we assume is an artifact of the simpli ed model.The other is that the behavior of this equation is a function of the ratio r ,and not of K and w separately.This implies that scaling w by p is the relevant scaling when considering correlation coef cients in this model.Figure 4b displays ½¤as a function of the scaling variable r .The curve displays a transition from low to high correlations near a critical value ofr,r c D p q.Strictly speaking,the transition becomes sharp only in the limit "!0.As can be observed in Figure4b,in this limit,the stable xed point is zero for r smaller than r c,whereas above r c it diverges rapidly.4.2An Integrate-and-Fire Model.When considering transmission ofa highly correlated signal in an asynchronously active population,as we do in this study,two main variables should be examined:neuronal ring rates and correlations between neurons.Clearly,both variables depend on each other.Several studies have dealt with these variables.Tetzlaff,Buscher-mohle,Geisel,and Diesmann(2001)focused on the evolution of output rates as a function of input rates along a chain with noisy input but disregarded the issue of correlation.An integrative study,however,is currently being conducted by Tetzlaff et al.(2002).Salinas and Sejnowski(2000)studied the ring rates and their variability as a function of input correlation.The cor-relation in the input was a result of a common input or common oscillation in the input rates.Analytical results based on a random walk model with drift gave results that were qualitatively similar to a conductance-based IAF model.In addition,Feng and Brown(2000)discussed the impact of tempo-rally correlated input on the output statistics of an IAF neuron.Stroeve and Gielen(2001)considered the correlation between a pair of neurons as a function of a correlated common input.They follow a logic similar to ours and compute½out.½in/for a conductance-based IF model;the resulting½¤was not derived.Here,we study the evolution of the output correlation as a function of the spatially correlated input in an IAF model.The IF model is less amenable to analytic studies than the simple model. Hence,we turn to simulations in order to compute½out.r;½in;ºin/.Note that we added the input ring rateºin as an additional parameter.This is due to the fact that input rates affect correlations of IF neurons(Salinas &Sejnowski,2000;Stroeve&Gielen,2001;Tetzlaff et al.,2001).Figure5a shows½out.½in/for different w and for xed values of K andºin.In contrast to the simple model,½out can cross the diagonal more than once.We look for the xed-point correlations in the dynamic steady state of the system.Following the procedure used for the simple model,we extracted the intersection points of the curve with the diagonal½out.½¤/D½¤.We also calculated the stability of these xed points by measuring the slope at thepoint of intersection.The results are depicted in Figure5b.If j d½¤d½in j<1,the xed point is stable(thick line);otherwise,it is unstable(thin dotted line).Here,we de ne r c to be the point where the saddle node bifurcation ap-pears.Note that the details of the results of Figure5depend on the way correlations were introduced.Following the Poisson mother process,as ex-plained in Kuhn et al.(in press),we introduced correlations well beyond the second order.Had we limited ourselves to currents with second-order correlations only,such as h1and h2of the appendix,the value of r c would change considerably.(a)r in r o u t(b)r r *Figure 5:Transition in IAF neurons.(a)½out as a function of ½in for different values of r (indicated above each curve).The crossings of the sigmoid curves and the diagonal (dashed line)yield the xed-point correlations.(b)Fixed-point correlations as a function of r .Stable xed points are indicated by a thick line and the unstable ones by a thin dotted line.Note that ½¤D 0is stable for any r in the range.Parameters:K D 9000,ºin D 1:5¤ºthre .Unlike the simple model,we note that for small r ½in ,½out »0.This insensitivity of the output correlation re ects the fact that the neurons’input is not completely balanced.Had the neurons’input been perfectly balanced,½out would have been more sensitive to small input correlation.However,we chose the same neurons’parameters as in our simulations of the full network,so as to match the full network simulation better.For r >r c ,the system is bistable.This is different from the simple model in Figure 4b,which had one global stable xed point for any value of r .Note,however,that although this two-neuron system is bistable,this does not imply that a network of IF neurons will be bistable.Even if the network settles rst in the lower (zero correlation)branch,transient spontaneous cor-relations may evolve,pushing the network into the basin of attraction of the upper branch.In fact,our network simulations described in the next section display either a low-correlation AI mode or a high-correlation synchronous mode of activity,depending on the parameters of the network.Moreover,we show in the next section that the critical behavior of the whole network displays scaling in the same variable r as predicted by the simple model.5The Full NetworkThe main lesson from our study of a pair of neurons is the existence of a rapid transition between low correlations to high correlations as function of the scaling variable r D w =p K .This type of behavior is also re ected in the simulations of IF networks.In our simulations,we use K D N =10.Running networks with different values of K (and N ),we search for the transitions,as a function of w ,of the networks from their AI mode to the global oscillatory mode.One possible measure is the coef cient of variance。