The PANTHER database of protein families,
哺乳动物雷帕霉素靶蛋白:神经系统治疗的新靶点
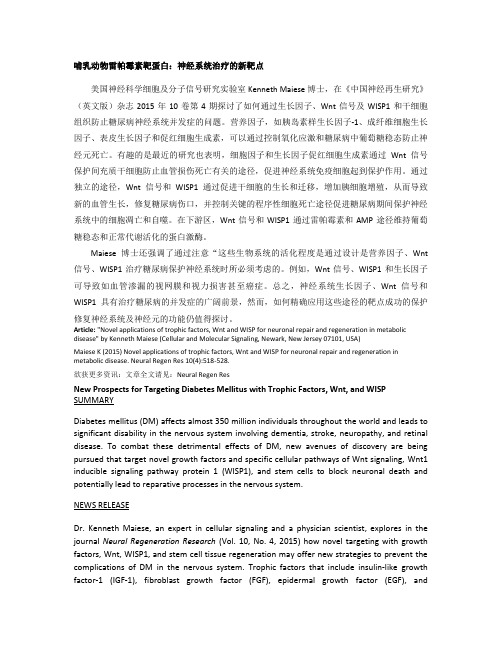
哺乳动物雷帕霉素靶蛋白:神经系统治疗的新靶点美国神经科学细胞及分子信号研究实验室Kenneth Maiese博士,在《中国神经再生研究》(英文版)杂志2015年10卷第4期探讨了如何通过生长因子、Wnt信号及WISP1和干细胞组织防止糖尿病神经系统并发症的问题。
营养因子,如胰岛素样生长因子-1、成纤维细胞生长因子、表皮生长因子和促红细胞生成素,可以通过控制氧化应激和糖尿病中葡萄糖稳态防止神经元死亡。
有趣的是最近的研究也表明,细胞因子和生长因子促红细胞生成素通过Wnt信号保护间充质干细胞防止血管损伤死亡有关的途径,促进神经系统免疫细胞起到保护作用。
通过独立的途径,Wnt信号和WISP1通过促进干细胞的生长和迁移,增加胰细胞增殖,从而导致新的血管生长,修复糖尿病伤口,并控制关键的程序性细胞死亡途径促进糖尿病期间保护神经系统中的细胞凋亡和自噬。
在下游区,Wnt信号和WISP1通过雷帕霉素和AMP途径维持葡萄糖稳态和正常代谢活化的蛋白激酶。
Maiese博士还强调了通过注意“这些生物系统的活化程度是通过设计是营养因子、Wnt信号、WISP1治疗糖尿病保护神经系统时所必须考虑的。
例如,Wnt信号、WISP1和生长因子可导致如血管渗漏的视网膜和视力损害甚至癌症。
总之,神经系统生长因子、Wnt信号和WISP1具有治疗糖尿病的并发症的广阔前景,然而,如何精确应用这些途径的靶点成功的保护修复神经系统及神经元的功能仍值得探讨。
Article: "Novel applications of trophic factors, Wnt and WISP for neuronal repair and regeneration in metabolic disease" by Kenneth Maiese (Cellular and Molecular Signaling, Newark, New Jersey 07101, USA)Maiese K (2015) Novel applications of trophic factors, Wnt and WISP for neuronal repair and regeneration in metabolic disease. Neural Regen Res 10(4):518-528.欲获更多资讯:文章全文请见:Neural Regen ResNew Prospects for Targeting Diabetes Mellitus with Trophic Factors, Wnt, and WISP SUMMARYDiabetes mellitus (DM) affects almost 350 million individuals throughout the world and leads to significant disability in the nervous system involving dementia, stroke, neuropathy, and retinal disease. To combat these detrimental effects of DM, new avenues of discovery are being pursued that target novel growth factors and specific cellular pathways of Wnt signaling, Wnt1 inducible signaling pathway protein 1 (WISP1), and stem cells to block neuronal death and potentially lead to reparative processes in the nervous system.NEWS RELEASEDr. Kenneth Maiese, an expert in cellular signaling and a physician scientist, explores in the journal Neural Regeneration Research(Vol. 10, No. 4, 2015) how novel targeting with growth factors, Wnt, WISP1, and stem cell tissue regeneration may offer new strategies to prevent the complications of DM in the nervous system. Trophic factors that include insulin-like growth factor-1 (IGF-1), fibroblast growth factor (FGF), epidermal growth factor (EGF), anderythropoietin (EPO) can each control glucose homeostasis and prevent neurons from dying during the insults of oxidative stress and DM. Interestingly, recent studies also have revealed that the cytokine and growth factor EPO uses Wnt signaling to the preserve mesenchymal stem cells, protect against vascular injury, block “death-related” pathways, and promote immune cell protection of the nervous system. Through independent pathways, Wnt signaling and WISP1 promote protection in the nervous system during DM by fostering stem cell growth and migration, increasing pancreatic -cell proliferation, leading to new blood vessel growth, repairing diabetic wounds, and controlling critical programmed cell death pathways of apoptosis and autophagy. Further downstream, Wnt and WISP1 assist to maintain glucose homeostasis and normal metabolism through the pathways of the mechanistic target of rapamycin (mTOR) and AMP activated protein kinase (AMPK). Maiese also emphasizes the complexity of trophic factors, Wnt, and WISP1 in designing strategies to protect the nervous system by noting “the degree of activation of these biological systems is an important consideration in developing therapies for DM”. For example, Wnt, WISP1, and growth factors can lead to complications such as vascular leakage in the retina and impair vision as well as lead to cancer is some systems of the body. Pathways such as AMPK also have the potential to lead to the death of pancreatic islet cells under some circumstances. Overall the prospects for developing new therapeutic strategies for the complications of DM in the nervous system with growth factors, Wnt, and WISP1 are met with great enthusiasm but require precise targeting of these pathways to bear clinical success for neuronal protection, repair, and regeneration in the nervous system.Article: "Novel applications of trophic factors, Wnt and WISP for neuronal repair and regeneration in metabolic disease" by Kenneth Maiese (Cellular and Molecular Signaling, Newark, New Jersey 07101, USA)Maiese K (2015) Novel applications of trophic factors, Wnt and WISP for neuronal repair and regeneration in metabolic disease. Neural Regen Res 10(4):518-528.。
蛋白质序列数据库

2 作者
▪ 数据或文章的作者是系统联系相关数据和科学研究的关键 因素;
▪ GenBank数据库的作者的输入全称姓和名的首字母
3 文章
▪ 最常见的生物科学文献是期刊文献,对于生物数据库的引用格式缺省 是期刊文献
▪ 文章也可出现在书、手稿及电子期刊上。 ▪ 期刊名、年份、文章的首页以及文章作者的姓
4 专利权
属性。
5 序列描述:
是在生物和(或)生物文献的上下文中描述一个生 物序列或生物序列集;
生物源(BioSource)-来源生物的信息; 分子信息(MolInfo)--描述器指示分子类型,如基因,
mRNA,EST,肽链信息。
蛋白质数据分析
由于传统的用X光晶体衍射和核磁共振 技术测定蛋白质的三维结构、用生化方法 研究蛋白质功能的效率不高,无法适应由 基因组测序所带来的蛋白质序列数量飞速 增长的需要,近年来,许多科学家致力于 用理论计算的方法预测蛋白质的三维结构 和功能,提高蛋白质功能研究的效率,并 取得了一定的成果。
▪ 2个大写字母(分:基因信息号,核酸序列和蛋白质序列均有gi号; ▪ gi的来源:由源数据库提供;序列仅当其完整地被提交公
共数据库处理后,才最终达到一个序列号和一个gi号; ▪ 位置:在VERSION行中,版本号,gi号 ▪ 修改记录时,新记录与原先记录不同时(哪怕是一个碱基
a. 所有序列条目都经过有经验的分子生物学家和蛋白 质化学家通过计算机工具并查阅有关文献资料仔细核 实。
防御素

• 这些研究案例充分显示了 b- 防御素的功能 多样性, 特别是涉及到精子在附睾的成熟、 保护、 表面修饰等诸多方面 • 由于 b- 防御素既联系免疫又参与生殖, 也 为理解感染、炎症和不育间的复杂关系以 及分子机理提供了新的视角
• Characterization and functions of beta defensins in the epididymis
• Morrison 等观察到鼠的 β-防御素 Defb10 和 Defb11 主要在脑部表达, 而更多的 β-防御素 Defb13、Defb15、Defb35 主要在睾丸和附睾表 达。 • 人类的 β-防御素在各大主要器官都有表达。最近 的文献显示哺乳动物的β-防御素在睾丸和附睾显 著优先表。 • 如大鼠Defb21、Defb24、Defb27、Defb30 和 Defb36, 而且在附睾的头、体、尾等区段呈现不 同的表达模式, 而在其它器官和组织不表达或较少 表达。
• 特别值得注意的是鼠类 β-防御素 13、22 等基因 在 RT-PCR 水平呈现严格附睾特异表达, 这表明 这些 β-防御素可能主要参与了附睾微环境形成和 精子在附睾的成熟与储存, 但具体机制未知。 • 某些人类 β-防御素如 DEFB105、118、119 、 120、121、123、128 在睾丸和附睾也比较特异 地表达。大量 β-防御素在睾丸和附睾特异表达的 生理意义以及具体的作用机制值得深入探讨,或许 可以找到阻断精子成熟的附睾靶标
β-防御素
• 防御素家族包括 3个亚家族, 即 α- 防御素、 β- 防御素和 θ- 防御素。由于近来发现β-防 御素在雄性生殖系统大量表达且与精子成 熟过程相关
• 国际上利用系统的计算搜索策略对基因组 数据库进行搜索, 发现了人类、大猩猩、小 鼠、大鼠和狗的所有 β-防御素, 它们的 β-防 御素基因数目分别为 39 、37 、53 、44 和 43
分子生物学名词解释

分子生物学名词解释大全AAbundance (mRNA 丰度):指每个细胞中mRNA 分子的数目。
Abundant mRNA(高丰度mRNA):由少量不同种类mRNA 组成,每一种在细胞中出现大量拷贝。
Acceptor splicing site (受体剪切位点):内含子右末端和相邻外显子左末端的边界。
Acentric fragment(无着丝粒片段):(由打断产生的)染色体无着丝粒片段缺少中心粒,从而在细胞分化中被丢失。
Active site(活性位点):蛋白质上一个底物结合的有限区域。
Allele(等位基因):在染色体上占据给定位点基因的不同形式。
Allelic exclusion(等位基因排斥):形容在特殊淋巴细胞中只有一个等位基因来表达编码的免疫球蛋白质。
Allosteric control(别构调控):指蛋白质一个位点上的反应能够影响另一个位点活性的能力。
Alu-equivalent family(Alu 相当序列基因):哺乳动物基因组上一组序列,它们与人类Alu家族相关。
Alu family (Alu家族):人类基因组中一系列分散的相关序列,每个约300bp长。
每个成员其两端有Alu 切割位点(名字的由来)。
α-Amanitin(鹅膏覃碱):是来自毒蘑菇Amanita phalloides 二环八肽,能抑制真核RNA聚合酶,特别是聚合酶II 转录。
Amber codon (琥珀密码子):核苷酸三联体UAG,引起蛋白质合成终止的三个MM子之一。
Amber mutation (琥珀突变):指代表蛋白质中氨基酸密码子占据的位点上突变成琥珀MM子的任何DNA 改变。
Amber suppressors (琥珀抑制子):编码tRNA的基因突变使其反MM子被改变,从而能识别UAG MM子和之前的MM 子。
Aminoacyl-tRNA (氨酰-tRNA):是携带氨基酸的转运RNA,共价连接位在氨基酸的NH2基团和tRNA 终止碱基的3¢或者2¢-OH 基团上。
chap11.主要分子生物信息数据库
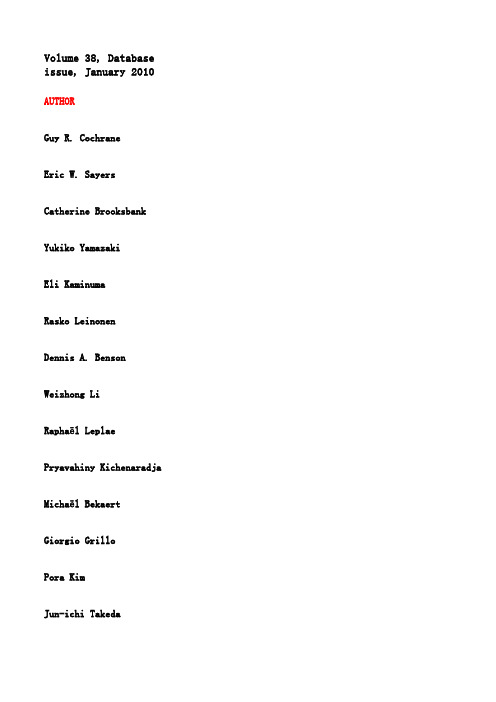
Volume 38, Database issue, January 2010 AUTHORGuy R. CochraneEric W. SayersCatherine BrooksbankYukiko YamazakiEli KaminumaRasko LeinonenDennis A. BensonWeizhong LiRaphaël LeplaePryavahiny KichenaradjaMichaël BekaertGiorgio GrilloPora KimJun-ichi TakedaBruno Contreras-Moreira Riu YamashitaElodie Portales-Casamar Pavel S. NovichkovJuan WangJian-Hua YangMartin Mokrejs Panagiotis AlexiouThe UniProt Consortium Yan ZhangJian RenChristian J. A. Sigrist Cathryn M. Gould Annalisa MarsicoJ. MullerGabriel ÖstlundRobert D. Finn Thomas RatteiNeil D. Rawlings Richard J. Roberts Saskia Preissner Andreas Schlicker Paula de MatosYanli WangGuohui Zheng Christian Koetschan Douglas H. Turner Michail Yu. Lobanov Yan Yuan Tseng Jonathan LeesFrançois EhrenmannSven GriepDonald S. Berkholz Patrick MayThe Gene Ontology ConsortiumJohannes GollTanja Davidsen Konstantinos Liolios Minoru KanehisaIkuo UchiyamaLubos KlucarJ. PelletMitsuteru Nakao Victor M. Markowitz Renzo Kottmann Paramvir S. DehalLuke E. UlrichLauren M. Brinkac Cristina Aurrecoechea Martha B. Arnaud Marek S. Skrzypek Stacia R. EngelHee Shin KimUlrike PfreundtMoritz GilsdorfJun DuanMartin AslettTodd W. HarrisVineet K. SharmaRon CaspiAlex FrolkisJunfeng GaoLewis Y. GeerAndreas RueppJudice L. Y. KohMilana Frenkel-Morgenstern Petras J. Kundrotas Benjamin A. ShoemakerB. Aranda, P. Achuthan Arnaud CeolPawel SmialowskiPeter VanheeMichael KuhnPaul FlicekP. J. KerseyPhil WilkinsonHugh MorganCarol J. BultAndrew BlakeKeiko AkagiJeff B. BowesBrooke RheadKate R. Rosenbloom Chisato YamasakiJon W. HussSamuel HiardSimon A. ForbesHong LiLishan WangAdnan S. SyedBrian A. Kennedy Stefan M. Woerner Misha Kapushesky Nicholas Paul GauthierLorna RichardsonJun ZhaoAedín C. Culhane Ramil N. NurtdinovLi JiJuan Antonio Vizcaíno Catherine Y. Cormier Ron MiloLynn M. SchrimlLiwei LiShaini ThomasEmilia LimFeng ZhuAthanasia Spandidos Agatha Schlüter Zhenhai ZhangWalter Sanseverino Paulino Pérez-Rodríguez Pawel DurekMotohiro MiharaDavid GrantHifzur Rahman Ansari Randi VitaJames RobinsonMartin ShumwayDATABASE NAMEThe 2010 Nucleic Acids Research Database Issue and online Database Collection: a community of data resources Database resources of the National Center for Biotechnology InformationThe European Bioinformatics Institute’s data resources NBRP databases: databases of biological resources in Japan DDBJ launches a new archive database with analytical tools for next-generation sequence dataImprovements to services at the European Nucleotide Archive GenBankNon-redundant patent sequence databases with value-added annotations at two levelsACLAME: A CLAssification of Mobile genetic Elements, update 2010ISbrowser: an extension of ISfinder for visualizing insertion sequences in prokaryotic genomesRecode-2: new design, new search tools, and many more genes UTRdb and UTRsite (RELEASE 2010): a collection of sequences and regulatory motifs of the untranslated regions of eukaryotic mRNAsChimerDB 2.0—a knowledgebase for fusion genes updatedH-DBAS: human-transcriptome database for alternative splicing: update 20103D-footprint: a database for the structural analysis of protein–DNA complexesDBTSS provides a tissue specific dynamic view of Transcription Start SitesJASPAR 2010: the greatly expanded open-access database of transcription factor binding profilesRegPrecise: a database of curated genomic inferences of transcriptional regulatory interactions in prokaryotesTransmiR: a transcription factor–microRNA regulation databasedeepBase: a database for deeply annotating and mining deep sequencing dataIRESite—a tool for the examination of viral and cellular internal ribosome entry sitesmiRGen 2.0: a database of microRNA genomic information and regulationThe Universal Protein Resource (UniProt) in 2010HHMD: the human histone modification databaseMiCroKit 3.0: an integrated database of midbody, centrosome and kinetochorePROSITE, a protein domain database for functional characterization and annotationELM: the status of the 2010 eukaryotic linear motif resource MeMotif: a database of linear motifs in a-helical transmembrane proteinseggNOG v2.0: extending the evolutionary genealogy of genes with enhanced non-supervised orthologous groups, species and functional annotationsInParanoid 7: new algorithms and tools for eukaryotic orthology analysisPANTHER version 7: improved phylogenetic trees, orthologs and collaboration with the Gene Ontology ConsortiumThe Pfam protein families databaseSIMAP—a comprehensive database of pre-calculated protein sequence similarities, domains, annotations and clusters MEROPS: the peptidase databaseREBASE—a database for DNA restriction and modification: enzymes, genes and genomesSuperCYP: a comprehensive database on Cytochrome P450 enzymes including a tool for analysis of CYP-drug interactionsFunSimMat update: new features for exploring functional similarityChemical Entities of Biological Interest: an updateAn overview of the PubChem BioAssay resource3DNALandscapes: a database for exploring the conformational features of DNAThe ITS2 Database III—sequences and structures for phylogenyNNDB: the nearest neighbor parameter database for predicting stability of nucleic acid secondary structureComSin: database of protein structures in bound (complex) and unbound (single) states in relation to their intrinsic disorderf POP: footprinting functional pockets of proteins by comparative spatial patternsGene3D: merging structure and function for a Thousand genomesIMGT/3Dstructure-DB and IMGT/DomainGapAlign: a database and a tool for immunoglobulins or antibodies, T cell receptors, MHC, IgSF and MhcSFPDBe: Protein Data Bank in EuropePDBselect 1992–2009 and PDBfilter-selectProtein Geometry Database: a flexible engine to explore backbone conformations and their relationships to covalent geometryPTGL: a database for secondary structure-based protein topologiesThe Gene Ontology in 2010: extensions and refinementsThe Protein Naming Utility: a rules database for protein nomenclatureThe comprehensive microbial resourceThe Genomes On Line Database (GOLD) in 2009: status of genomic and metagenomic projects and their associated metadataKEGG for representation and analysis of molecular networks involving diseases and drugsMBGD update 2010: toward a comprehensive resource for exploring microbial genome diversityphiSITE: database of gene regulation in bacteriophages ViralORFeome: an integrated database to generate a versatile collection of viral ORFsCyanoBase: the cyanobacteria genome database update 2010The integrated microbial genomes system: an expanding comparative analysis resource: integrated database resource for marine ecological genomicsMicrobesOnline: an integrated portal for comparative and functional genomicsThe MiST2 database: a comprehensive genomics resource on microbial signal transductionPathema: a clade-specific bioinformatics resource center for pathogen researchEuPathDB: a portal to eukaryotic pathogen databasesThe Aspergillus Genome Database, a curated comparative genomics resource for gene, protein and sequence information for the Aspergillus research communityNew tools at the Candida Genome Database: biochemical pathways and full-text literature searchSaccharomyces Genome Database provides mutant phenotypedataBeetleBase in 2010: revisions to provide comprehensive genomic information for Tribolium castaneumFlyTF: improved annotation and enhanced functionality of the Drosophila transcription factor databaseGenomeRNAi: a database for cell-based RNAi phenotypes. 2009 updateSilkDB v2.0: a platform for silkworm (Bombyx mori ) genome biologyTriTrypDB: a functional genomic resource for the TrypanosomatidaeWormBase: a comprehensive resource for nematode research MetaBioME: a database to explore commercially useful enzymes in metagenomic datasetsThe MetaCyc database of metabolic pathways and enzymes and the BioCyc collection of pathway/genome databasesSMPDB: The Small Molecule Pathway DatabaseThe University of Minnesota Biocatalysis/Biodegradation Database: improving public accessThe NCBI BioSystems databaseCORUM: the comprehensive resource of mammalian protein complexes—2009DRYGIN: a database of quantitative genetic interaction networks in yeastDynamic Proteomics: a database for dynamics andlocalizations of endogenous fluorescently-tagged proteins in living human cellsGWIDD: Genome-wide protein docking databaseInferred Biomolecular Interaction Server—a web server to analyze and predict protein interacting partners and binding sitesThe IntAct molecular interaction database in 2010MINT, the molecular interaction database: 2009 updateThe Negatome database: a reference set of non-interacting protein pairsPepX: a structural database of non-redundant protein–peptide complexesSTITCH 2: an interaction network database for small molecules and proteinsEnsembl’s 10th yearEnsembl Genomes: Extending Ensembl across the taxonomic spaceEMMA—mouse mutant resources for the internationalscientific communityEuroPhenome: a repository for high-throughput mouse phenotyping dataThe Mouse Genome Database: enhancements and updatesMouseBook: an integrated portal of mouse resources MouseIndelDB: a database integrating genomic indel polymorphisms that distinguish mouse strainsXenbase: gene expression and improved integrationThe UCSC Genome Browser database: update 2010ENCODE whole-genome data in the UCSC Genome BrowserH-InvDB in 2009: extended database and data mining resources for human genes and transcriptsThe Gene Wiki: community intelligence applied to human gene annotationPatrocles: a database of polymorphic miRNA-mediated gene regulation in vertebratesCOSMIC (the Catalogue of Somatic Mutations in Cancer): a resource to investigate acquired mutations in human cancerdbDEPC: a database of Differentially Expressed Proteins in human CancersHLungDB: an integrated database of human lung cancer researchNetwork of Cancer Genes: a web resource to analyze duplicability, orthology and network properties of cancer genesHRTBLDb: an informative data resource for hormone receptors target binding lociSelTar base, a database of human mononucleotide-microsatellite mutations and their potential impact to tumorigenesis and immunologyGene Expression Atlas at the European BioinformaticsInstitute: version 2.0, an updated comprehensive, multi-species repository of cell cycle experiments and derived analysis resultsEMAGE mouse embryo spatial gene expression database: 2010 updateFlyTED: the Drosophila Testis Gene Expression Database GeneSigDB—a curated database of gene expression signaturesPLANdbAffy: probe-level annotation database for Affymetrix expression microarraysNCBI Peptidome: a new repository for mass spectrometry proteomics dataThe Proteomics Identifications database: 2010 updateProtein Structure Initiative Material Repository: an open shared public resource of structural genomics plasmids for the biological communityBioNumbers—the database of key numbers in molecular andcell biologyGeMInA, Genomic Metadata for Infectious Agents, a geospatial surveillance pathogen databaseBioDrugScreen: a computational drug design resource for ranking molecules docked to the human proteomeCAMP: a useful resource for research on antimicrobial peptidesT3DB: a comprehensively annotated database of common toxins and their targetsUpdate of TTD: Therapeutic Target DatabasePrimerBank: a resource of human and mouse PCR primer pairs for gene expression detection and quantification PeroxisomeDB 2.0: an integrative view of the global peroxisomal metabolomePMRD: plant microRNA databasePRGdb: a bioinformatics platform for plant resistance gene analysisPlnTFDB: updated content and new features of the plant transcription factor databasePhosPhAt: the Arabidopsis thaliana phosphorylation site database. An updateSALAD database: a motif-based database of protein annotations for plant comparative genomicsSoyBase, the USDA-ARS soybean genetics and genomics database AntigenDB: an immunoinformatics database of pathogen antigensThe Immune Epitope Database 2.0IPD—the Immuno Polymorphism DatabaseArchiving next generation sequencing dataWEB ADDRESS/nar/database/a/ http://www.nbrp.jphttp://www.ddbj.nig.ac.jp/ena/patentdata/nr/http://aclame.ulb.ac.behttp://www-genome.biotoul.fr/ISbrowser.php http://recode.ucc.ier.it/http://ercsb.ewha.ac.kr/fusiongenehttp://h-invitational.jp/h-dbas/http://floresta.eead.csic.es/3dfootprint http://dbtss.hgc.jp//transmir/http://www.microrna.gr/mirgen//hhmd/prosite//http://projects.biotec.tu-dresden.de/memotif http://eggnog.embl.dehttp://InParanoid.sbc.su.se/http://mips.gsf.de/simap/http://bioinformatics.charite.de/supercyp http://www.funsimmat.de/chebi/http://its2.bioapps.biozentrum.uniwuerzburg.de /NNDBhttp://antares.protres.ru/comsin//fpop///pdbe/http://bioinfo.tg.fh-giessen.de/pdbselect/ /http://ptgl.zib.de/pn-utilityhttp://www.genome.jp/kegg/http://mbgd.genome.ad.jp//http://genome.kazusa.or.jp/cyanobase//http://metasystems.riken.jp/metabiome/ http://www.smpdb.ca//biosystems/http://mips.helmholtzmuenchen.de/genre/proj/co rum/index.htmlbr.utoronto.ca//Structure/ibis/ibi s.html/intacthttp://mint.bio.uniroma2.it/minthttp://mips.helmholtz-muenchen.de/proj/ppi/negatomehttp://stitch.embl.de////http://www.h-invitational.jp//wiki/Portal:Gene_Wiki //cosmic//index//bio/hlunghttp://bio.ifom-ieo-campus.it/ncg/hrtbldb/gxa/emage//genesigdb http://affymetrix2.bioinf.fbb.msu.ru/peptidome /pride /http://www.bicnirrh.res.in/antimicrobial .sg/group/cjttd/TTD.asp /primerbank/ /PMRDhttp://plntfdb.bio.uni-potsdam.de/v3.0/ phosphat.mpimp-golm.mpg.dehttp://salad.dna.affrc.go.jp/salad/http://www.imtech.res.in/raghava/antigendb /ipd//Traces/sra。
蛇毒素蛋白毕赤酵母表达进展
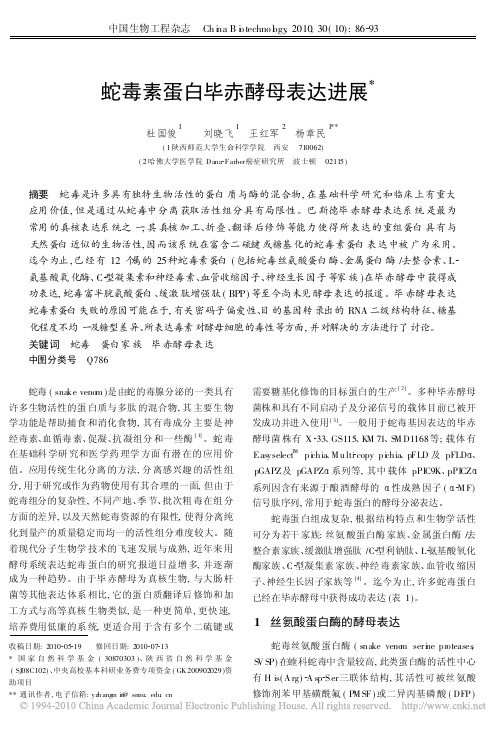
88
中国生物工程杂志 China B io techno logy
Vo.l 30 N o. 10 2010
1 丝氨酸蛋白酶的酵母表达
蛇毒丝氨酸 蛋白酶 ( sn ake venom serine p roteases, SV SP) 在蝰科蛇毒中含量较高, 此类蛋白酶的活性中心 有 H is( A rg) A sp S er三联体 结构, 其 活性可 被丝 氨酸 修饰剂苯 甲基磺酰氟 ( PM SF ) 或二异丙基磷 酸 ( DFP )
类凝血酶
B atroxobin
H arob in A nc rod C a lobin G loshedobin
独特活性 SVSP
型
蛇毒金属蛋白酶
( SVM P)
型
L 氨基酸氧化酶
P roteinC activa tor
A lbo fibrase A lfim eprase F ibrinogenase IV E chistatin Rhodostom in
H a lydin J erdon itin A lbola tin Saxat ilin heterodim e r)
神经毒素 ( NT ) 血管收缩素
p resyn ap tic NT
PLA 2 B ung arotox in
p os ts ynap tic NT
迄今为止, 超过 30 种的蛇毒 类凝血 酶已被发 现, 由于在血栓治疗方面的重要用途, 因而这类 SV SP 是蛇 毒蛋白酵母表达研究最多的。 Yang等 [ 6 7] 用 pP IC9K 载 体在毕赤 酵母 GS115 中分 别表 达了 长白 山白 眉蝮 蛇 ( G loyd ius ussuriensis)类凝血酶 gu ssurobin 基因和蛇岛蝮 ( G loyd ius shedaoensis)的 g loshedobin 基因, 重组 类凝 血 酶蛋白 Gu ssu rob in含有 260个氨基酸和 12个半胱氨酸 残基, 产量 为 3. 5 m g /L, 分 子量 为 28kD a( 理 论 值 为
免疫组学的研究进展
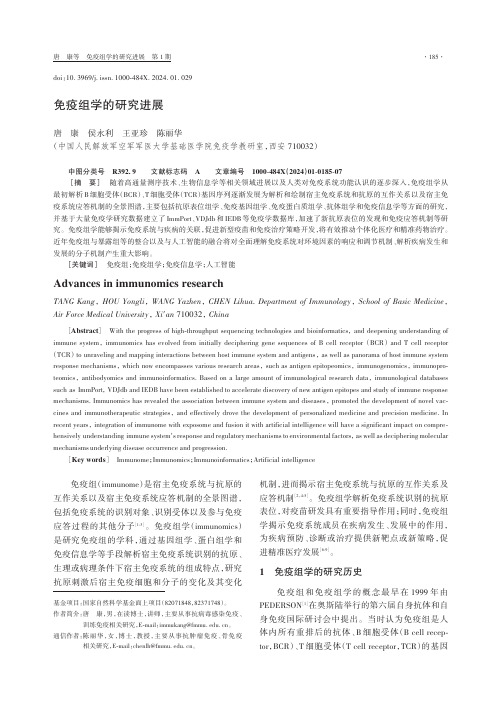
免疫组学的研究进展唐康侯永利王亚珍陈丽华(中国人民解放军空军军医大学基础医学院免疫学教研室,西安 710032)中图分类号R392.9 文献标志码 A 文章编号1000-484X(2024)01-0185-07[摘要]随着高通量测序技术、生物信息学等相关领域进展以及人类对免疫系统功能认识的逐步深入,免疫组学从最初解析B细胞受体(BCR)、T细胞受体(TCR)基因序列逐渐发展为解析和绘制宿主免疫系统和抗原的互作关系以及宿主免疫系统应答机制的全景图谱,主要包括抗原表位组学、免疫基因组学、免疫蛋白质组学、抗体组学和免疫信息学等方面的研究,并基于大量免疫学研究数据建立了ImmPort、VDJdb和IEDB等免疫学数据库,加速了新抗原表位的发现和免疫应答机制等研究。
免疫组学能够揭示免疫系统与疾病的关联,促进新型疫苗和免疫治疗策略开发,将有效推动个体化医疗和精准药物治疗。
近年免疫组与暴露组等的整合以及与人工智能的融合将对全面理解免疫系统对环境因素的响应和调节机制、解析疾病发生和发展的分子机制产生重大影响。
[关键词]免疫组;免疫组学;免疫信息学;人工智能Advances in immunomics researchTANG Kang, HOU Yongli, WANG Yazhen, CHEN Lihua. Department of Immunology, School of Basic Medicine,Air Force Medical University, Xi'an 710032, China[Abstract]With the progress of high-throughput sequencing technologies and bioinformatics, and deepening understanding of immune system,immunomics has evolved from initially deciphering gene sequences of B cell receptor (BCR)and T cell receptor (TCR) to unraveling and mapping interactions between host immune system and antigens, as well as panorama of host immune system response mechanisms, which now encompasses various research areas, such as antigen epitopeomics, immunogenomics, immunopro‐teomics, antibodyomics and immunoinformatics. Based on a large amount of immunological research data, immunological databases such as ImmPort, VDJdb and IEDB have been established to accelerate discovery of new antigen epitopes and study of immune response mechanisms. Immunomics has revealed the association between immune system and diseases, promoted the development of novel vac‐cines and immunotherapeutic strategies, and effectively drove the development of personalized medicine and precision medicine. In recent years, integration of immunome with exposome and fusion it with artificial intelligence will have a significant impact on compre‐hensively understanding immune system's response and regulatory mechanisms to environmental factors, as well as deciphering molecular mechanisms underlying disease occurrence and progression.[Key words]Immunome;Immunomics;Immunoinformatics;Artificial intelligence免疫组(immunome)是宿主免疫系统与抗原的互作关系以及宿主免疫系统应答机制的全景图谱,包括免疫系统的识别对象、识别受体以及参与免疫应答过程的其他分子[1-3]。
蛋白质数据分析
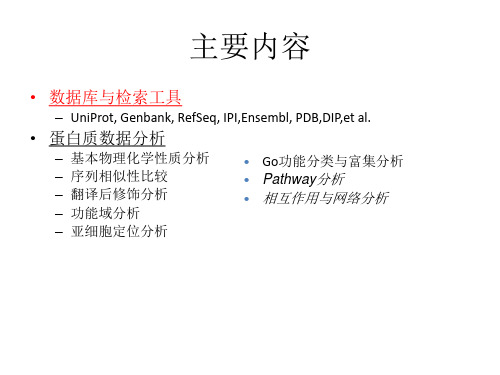
Go功能分类与富集分析
Pathway分析 相互作用与网络分析
亚细胞定位分析
序列相似性比较
• 两序列比较
– 主要工具:BLAST – 常用数据库:NCBI NR,SWISSPROT – 命令示例:
• formatdb -i nr.fasta –o T –p T • blastall –i input.seq –d nr –p blastp –e 1e-3
BLAST
GENEGO HMMER
EMBOSS
Interproscan
BLAST2GO …………………………….
TOOLS
Output
常见数据
GI:120407068 NP_000537.3 XP_001604088.1 AAF36358.1
P53_HUMAN P04637 Q9EX73
IPI00025087.2来自 基本物理化学性质分析 序列相似性比较 翻译后修饰分析 功能域分析
Go功能分类与富集分析
Pathway分析 相互作用与网络分析
亚细胞定位分析
蛋白质功能域分析
一、蛋白质功能域数据资源
数据库名称 PANTHER Pfam
CDD
简短描述
用实验和进化相关数据信息对蛋白质家族进行 分类
• CDD库下载:
/pub/mmdb/cdd/
• 详细信息:
/staff/tao/URLAPI/rpsblast.html
主要内容
• 数据库与检索工具
– UniProt, Genbank, RefSeq, IPI,Ensembl, PDB,DIP,et al.
多序列比较和隐马尔科夫模式分析覆盖蛋白质 功能域和家族
- 1、下载文档前请自行甄别文档内容的完整性,平台不提供额外的编辑、内容补充、找答案等附加服务。
- 2、"仅部分预览"的文档,不可在线预览部分如存在完整性等问题,可反馈申请退款(可完整预览的文档不适用该条件!)。
- 3、如文档侵犯您的权益,请联系客服反馈,我们会尽快为您处理(人工客服工作时间:9:00-18:30)。
The PANTHER database of protein families,subfamilies,functions and pathwaysHuaiyu Mi,Betty Lazareva-Ulitsky,Rozina Loo,Anish Kejariwal,Jody Vandergriff,Steven Rabkin,Nan Guo,Anushya Muruganujan,Olivier Doremieux,Michael J.Campbell,Hiroaki Kitano 1and Paul D.Thomas*Computational Biology,Applied Biosystems,850Lincoln Center Drive,Foster City,CA 94404,USA and 1The Systems Biology Institute and ERATO-SORST Kitano Symbiotic Systems Project/Japan Science and Technology Agency,Suite 6A,M31,6-31-15Jingumae,Shibuya,Tokyo 150-0001,JapanReceived September 15,2004;Revised and Accepted October 8,2004ABSTRACTPANTHER is a large collection of protein families that have been subdivided into functionally related sub-families,using human expertise.These subfamilies model the divergence of specific functions within protein families,allowing more accurate asso-ciation with function (ontology terms and pathways),as well as inference of amino acids impor-tant for functional specificity.Hidden Markov models (HMMs)are built for each family and subfamily for classifying additional protein sequences.The latest version,5.0,contains 6683protein families,divided into 31705subfamilies,covering $90%of mamma-lian protein-coding genes.PANTHER 5.0includes a number of significant improvements over previous versions,most notably (i)representation of path-ways (primarily signaling pathways)and asso-ciation with subfamilies and individual protein sequences;(ii)an improved methodology for defin-ing the PANTHER families and subfamilies,and for building the HMMs;(iii)resources for scoring sequences against PANTHER HMMs both over the web and locally;and (iv)a number of new web resources to facilitate analysis of large gene lists,including data generated from high-throughput expression experiments.Efforts are underway to add PANTHER to the InterPro suite of databases,and to make PANTHER consistent with the PIRSF database.PANTHER is now publicly available without restriction at http://panther.appliedbio .INTRODUCTIONThe philosophy,as well as the basic methodology,behind the PANTHER database has been described previously (1,2);therefore,we focus here on the recent improvements to the database and to the functionality available on the website.In brief,there are two main parts to PANTHER:PANTHER/LIB,a library of protein families and subfamilies;and PANTHER/X,a set of ontology terms describing protein function.The data-base’s main advantage is in the curator-defined grouping of protein sequences into functional subfamilies,allowing more detailed and accurate association with the ontology terms,and now biological pathways.Each family and subfamily is repre-sented by a phylogenetic tree of ‘training sequences’,and a hidden Markov model (HMM)that represents these sequences as a statistical model.The HMM library can be searched to classify new sequences,or to provide a score to predict the likely functional consequence of a mutation (1).PANTHER is quite comprehensive for the annotation of protein sequences encoded by metazoan genomes:$90%of mammalian protein-coding genes,and nearly two-thirds of Drosophila genes,are hit by a PANTHER HMM.The PANTHER database has recently been expanded to include associations between protein sequences and the biological pathways they participate in.Like the molecular function and biological process ontology terms,these path-ways are associated with individual protein sequences,and when possible with PANTHER subfamily HMMs,by expert curators.We have also improved the methodology used to define protein families and subfamilies.These improvements are mainly in two areas:global clustering of protein sequence space to allow definition of family boundaries,and new algo-rithms that make use of ontology terms to provide a guide for curators to define both families and subfamilies.*To whom correspondence should be addressed.Tel:+16505542723;Fax:+16505542344;Email:paul.thomas@The online version of this article has been published under an open access ers are entitled to use,reproduce,disseminate,or display the open access version of this article for non-commercial purposes provided that:the original authorship is properly and fully attributed;the Journal and Oxford University Press are attributed as the original place of publication with the correct citation details given;if an article is subsequently reproduced or disseminated not in its entirety but only in part or as a derivative work this must be clearly indicated.For commercial re-use permissions,please contact journals.permissions@.ª2005,the authorsNucleic Acids Research,Vol.33,Database issue ªOxford University Press 2005;all rights reservedD284–D288Nucleic Acids Research,2005,Vol.33,Database issue doi:10.1093/nar/gki078There are also a number of significant improvements to the website.Perhaps most importantly for users,the site is now free of the previous restrictions on its use(3).In addition, HMMs can be downloaded,and/or searched interactively using a protein sequence as a query.Pathways can be inter-actively browsed and queried.Gene lists(e.g.from mRNA expression data)can be uploaded to the site and analyzed relative to molecular functions,biological processes and pathways.STATISTICS FOR PANTHER5.0PANTHER/LIB(library of protein family and subfamily HMMs),version 5.0contains256413training sequences, grouped into6683families.These families were then divided further into31705subfamilies.PANTHER HMMs have been used to annotate the protein-coding genes annotated in the human,mouse,rat and Drosophila melanogaster genomes.The fractions of these genes that were given a functional annotation by PANTHER 5.0are shown in Table1.PANTHER WEBSITE FUNCTIONALITYSeveral resources are now available at the PANTHER website. Interactive(i)Ontology term browser.The PANTHER Prowler(1)isdesigned for browsing ontology terms to retrieve asso-ciated families,subfamilies or individual proteins. (ii)Updated:tree and multiple sequence alignment(MSA) viewer.The PANTHER Tree-Attribute Viewer facilitates exploration of each protein family tree.It has been mod-ified recently to allow a user to view either the sequence annotations as described previously(1),or the family MSA.The MSA view includes a number of features such as highlighting subfamily-specific amino acid conservation.(iii)New:sequence search against PANTHER HMMs.The website now provides interactive scoring of user-submitted sequences against the PANTHER library. (iv)Classification of whole ers can browse or query stored PANTHER HMM hits for all protein sequences annotated in the whole genomes of human, mouse,rat[from the LocusLink database(4)]and Drosophila melanogaster[from FlyBase(5)].(v)New:ers can browse or query pathways associated with PANTHER families,subfamilies and training sequences(Figure1).Pathway diagrams were drawn by expert curators using the CellDesigner software program(6),which supports Systems Biology Markup Language(SBML)standards(7)and uses the process notation of Kitano(8)to represent pathways as a series of reactions.The same curators associated proteins in the diagrams with PANTHER families,subfamilies and train-ing sequences.There are over60pathways,primarily signaling pathways,available as of January2005. (vi)New:gene expression analysis ers can upload gene lists(e.g.from mRNA expression experiments)and view them in the context of the pathways described above.In addition,users can analyze gene lists to look for sta-tistically significant trends with respect to different groupings of genes:families,molecular functions,biolo-gical processes and pathways.In addition to the binomial test described previously(9),the Mann–Whitney U-test as described in(10)can be performed on uploaded data to look for statistically significant differences in distributions of uploaded values(Figure2).(vii)New:pie and bar charts of functions.Graphical repre-sentations of the functions of genes or proteins across an entire list can be generated in a single click from any list on the site,either generated at the website or uploaded bya user(Figure3).Downloads(i)PANTHER HMMs are available in both SAM(11)andHMMER(12)format.(ii)Modified InterProScan(13)software can be down-loaded for scoring sequences locally against PANTHER HMMs.INTEGRATION WITH OTHER WEB RESOURCES PANTHER has been mapped to existing InterPro(14) entries,and thisfile is available from http://panther. /downloads/.PANTHER will be incorporated into the InterPro suite of databases incrementally. PANTHER HMMs have also been mapped to existing PIRSF(15)entries,and a collaboration is currently underway to make PANTHER and PIRSF consistent and cross-referenced.NEW METHODS FOR PANTHER5.0For version5.0,we implemented a number of improvements to the PANTHER library building procedure as described previously(1).At the end of this process,we evaluatedTable1.Number of genes from each organism classified using PANTHER HMMsGenome No.ofgenes No.of geneswith PANTHERHMM hitNo.of geneswith MFassociationNo.of geneswith BPassociationLocusLink human1623214533(89.5%)10453(64.4%)10410(64.1%)LocusLink mouse1502013147(87.5%)10012(66.7%)9933(66.1%)LocusLink rat45164391(97.2%)3967(87.8%)3969(87.9%)FlyBaseD.melanogaster 136549325(68.3%)6253(45.8%)5719(41.9%)These classifications can be searched on the PANTHER website.For Locus-Link,only genes associated with at least one reviewed RefSeq(accession no.beginning with‘NP’)were considered.Genes encoding proteinsthat hit a PANTHER HMM can be classified to a family or subfamily,and mostbut not all of these are associated with meaningful molecular function(MF)or biological process(BP)classifications.Nucleic Acids Research,2005,Vol.33,Database issue D285the HMM classifications of a test set of over 10000sequences from SWISS-PROT to make sure that the new process did not lower the accuracy of the classifications reported (16).We found that the classification accuracy was nearly identical,and the coverage was slightly improved in 5.0,probably due to the new HMM building process outlined below.Global UPGMA clustering to define family boundariesPANTHER version 3.0(1,2)used seed-based clustering to define protein families.The advantage of this approach was its modularity:new families could be easily added in areas that were inadequately covered in previous versions.However,the seed-based clustering resulted in significant redundancy for anumber of large protein families,such as protein kinases and G-protein-coupled receptors,which were covered by a number of families that overlapped to varying degrees.The current version,PANTHER version 5.0,addresses this issue by implementing a global clustering of proteins.Proteins from PANTHER version 4.0were clustered using a similarity metric derived from the pairwise BLASTP scores:S a ,b ðÞ=max S a ,a ðÞ,S b ,b ðÞ½1where S (a ,b )is the BLASTP raw score for the alignment of sequences a and b using the BLOSUM62matrix and masked for low-complexity segments.The denominator is the largest self-alignment score,and therefore,the similarity is the frac-tion of the maximum score possible for an alignment of sequences a and b .In cases where there weremultipleFigure 1.CellDesigner (6)diagram of the insulin/IGF receptor signaling pathway.Proteins (blue and brown boxes)are mapped onto PANTHER HMMs.Active forms (dashed-line boxes)and phosphorylated forms (small circles around the letter ‘P’)of proteins are clearly indicated in the diagram.Over 60pathways (mostly signaling pathways)are currently available.D286Nucleic Acids Research,2005,Vol.33,Database issuehigh-scoring pairs (HSPs;i.e.partial alignments),S (a ,b )was set equal to the sum of the scores for the maximal set of non-overlapping HSPs.This pairwise similarity was used to define single-linkage clusters (maximal clusters in which each protein is connected to at least one other protein in the cluster by a non-zero similarity score).A dendrogram was built for each single-linkage cluster using the UPGMA algorithm (17).The family labels from the PANTHER version 4.0library were then used to define the optimal cut of each UPGMA dendrogram into family clusters,to maximize the correspondence to previous versions of PANTHER.In the great majority of cases,the PANTHER version 5.0family was almost identical to the corresponding family in the previous version of the library.Only about 40subtrees in the UPGMA dendrograms,primarily those that were represented by overlapping clusters in the previous version,had to be broken further into functionally homogeneous clusters using manual curation.Overall,the family clusters identified from the UPGMA dendrograms covered over 96%of the version 4.0training sequences.The rest of the sequences were either singletons according to Equation 1(often due to low-complexity masking),or lay outside the family boundaries defined by PANTHER ver-sion 4.0family labels on the UPGMA dendrograms.Each of these ‘leftover’sequences (unmasked)was scored against SAM HMMs built for the family clusters,and was brought into the family of the best scoring HMM if the NLL-NULL score was less than À50.Those leftovers not meeting this criterion were added as singleton families if they were from a primate or rodent species;otherwise they were removed from the library.Simplified HMM building processThe UPGMA-derived family clusters allow us to simplify the HMM-building process detailed previously (1).Rather than building ‘initial’and ‘extended’HMMs,for PANTHER 5.0,we built the family HMM directly from the UPGMA family cluster in a single step.Because the HMM training sequences are of varying lengths,we pre-set the SAM buildmodel –model length option to be 1.1times the maximum sequence length in the cluster,and also added the option –sw2,to create a local HMM.Similar to previous versions of the library,this temporary HMM was used to create an alignment (using the SAM align2model procedure with the Àsw2option)that could be used to estimate the weights of the sequences in the initial HMM.A weighted model was then constructed followed by a weighted alignment.In PANTHER 5.0,we used a faster version of TIPS (version 2.0,available from the Downloads section of the PANTHER website)to create the phylogenetic trees (18).As in previous versions,the MSA was used as input to the new TIPS2algorithm,along with the following parameters.-prior ,-score_matrix BLOSUM 62,-cut_using_dis-tance 0.5,-pair_type 1and -use_are_as_branch_length 0.Subfamily division guided by ontology termsBecause the subfamily labels and associated ontology terms were expanded and reviewed by curators for both versions 3.0and 4.0,and shown to have a high rate of accuracy (16),we developed an algorithm for optimally dividing a treeintoFigure 2.Statistical analysis of gene expression experiment results for a liver cancer versus normal cell ers can upload a list of genes/transcripts,along with an associated value (e.g.fold change,but can be any continuous variable).The list is divided automatically into groups sharing the same function (molecular function,biological process or pathway),and the distribution of values for each group is compared statistically with the overall distribution using the Mann–Whitney U -test to look for coordinated changes across each group(10).Figure 3.Pie chart of molecular functions represented in a list of ers can upload protein IDs to the PANTHER website,and pie charts can be drawn from any list.Nucleic Acids Research,2005,Vol.33,Database issue D287subfamilies given subfamily labels on each sequence(18). These divisions were then reviewed once again by expert curators,and adjusted if necessary.This methodology will allow regular updates to PANTHER training sequences with minimal curation effort.Another significant advantage of this approach is that any arbitrary grouping of sequences can be superimposed on our phylogenetic trees to define subfamilies(and associated HMMs).This approach will allow straightforward incorpora-tion of external annotations such as those produced by single protein family databases,or from large ontology association projects such as GOA(19,20).REFERENCES1.Thomas,P.D.,Campbell,M.C.,Kejariwal,A.,Mi,H.,Karlak,B.,Daveran,R.,Diemer,K.,Muruganujan,A.and Narechania,A.(2003)PANTHER:a library of protein families and subfamilies indexed byfunction.Genome Res.,13,2129–2141.2.Thomas,P.D.,Kejariwal,A.,Campbell,M.C.,Mi,H.,Diemer,K.,Guo,N.,Ladunga,I.,Ulitsky-Lazareva,B.,Muruganujan,A.and Rabkin,S.(2003) PANTHER:a browsable database of gene products organized bybiological function,using curated protein family and subfamilyclassification.Nucleic Acids Res.,31,334–341.3.Thomas,P.D.,Campbell,M.C.,Kejariwal,A.,Mi,H.,Karlak,B.,Daveran,R.,Diemer,K.,Muruganujan,A.and Narechania,A.(2003)Corrigendum for PANTHER:a library of protein families and subfamilies indexed by function.Nucleic Acids Res.,31,2024.4.Pruitt,K.D.and Maglott,D.R.(2001)RefSeq and LocusLink:NCBI gene-centered resources.Nucleic Acids Res.,29,137–140.5.FlyBase Consortium(2002)The FlyBase database of the Drosophilagenome projects and community literature.Nucleic Acids Res.,30,106–108.6.Funahashi,A.,Morohashi,M.and Kitano,H.(2003)CellDesigner:aprocess diagram editor for gene-regulatory and biochemical networks.Biosilico,1,159–162.7.Hucka,M.,Finney,A.,Sauro,H.M.,Bolouri,H.,Doyle,J.C.,Kitano,H.,Arkin,A.P.,Bornstein,B.J.,Bray,D.,Cornish-Bowden,A.et al.(2003)The Systems Biology Markup Language(SBML):a medium forrepresentation and exchange of biochemical network models.Bioinformatics,19,524–531.8.Kitano,H.(2003)A graphical notation for biochemical networks.Biosilico,1,169–176.9.Cho,R.J.and Campbell,M.J.(2000)Transcription,genomes,function.Trends Genet.,16,409–415.10.Clark,A.G.,Glanowski,S.,Nielsen,R.,Thomas,P.D.,Kejariwal,A.,Todd,M.J.,Tanenbaum,D.M.,Civello,D.,Lu,F.,Murphy,B.et al.(2003) Inferring nonneutral evolution from human–chimp–mouse orthologous trios.Science,302,1960–1963.11.Karplus,K.,Barrett,C.and Hughey,R.(1998)Hidden Markov modelsfor detecting remote protein homologies.Bioinformatics,14,846–856.12.Eddy,S.R.(1996)Hidden Markov models.Curr.Opin.Struct.Biol.,6,361–365.13.Zdobnov,E.M.and Apweiler,R.(2001)InterProScan—an integrationplatform for the signature-recognition methods in InterPro.Bioinformatics,17,847–848.14.Mulder,N.J.,Apweiler,R.,Attwood,T.K.,Bairoch,A.,Barrell,D.,Bateman,A.,Binns,D.,Biswas,M.,Bradley,P.,Bork,P.et al.(2003)The InterPro Database,2003brings increased coverage and newfeatures.Nucleic Acids Res.,31,315–318.15.Wu,C.H.,Nikolskaya,A.,Huang,H.,Yeh,L.S.,Natale,D.A.,Vinayaka,C.R.,Hu,Z.Z.,Mazumder,R.,Kumar,S.,Kourtesis,P.et al.(2004)PIRSF:family classification system at the Protein Information Resource.Nucleic Acids Res.,32,112–114.16.Mi,H.,Vandergriff,J.,Campbell,M.,Narechania,A.,Majoros,W.,Lewis,S.,Thomas,P.D.and Ashburner,M.(2003)Assessment ofgenome-wide protein function classification for Drosophilamelanogaster.Genome Res.,13,2118–2128.17.Sokal,R.R.and Michener,C.D.(1958)A statistical method for evaluationsystematic relationships.Univ.Kansas Sci.Bull.,28,1409–1438. zareva-Ulitsky,B.and Thomas,P.D.(2005).On the quality of tree-based protein classification.Bioinformatics,in press.19.Gene Ontology Consortium(2000)Gene Ontology:tool for theunification of biology.Nature Genet.,25,25–29.20.Camon,E.,Magrane,M.,Barrell,D.,Binns,D.,Fleischmann,W.,Kersey,P.,Mulder,N.,Oinn,T.,Maslen,J.,Cox,A.et al.(2003)TheGene Ontology Annotation(GOA)project:implementation ofGO in SWISS-PROT,TrEMBL,and InterPro.Genome Res.,13,662–672.D288Nucleic Acids Research,2005,Vol.33,Database issue。