Scalable learning in stochastic games
H2O.ai 自动化机器学习蓝图:人类中心化、低风险的 AutoML 框架说明书
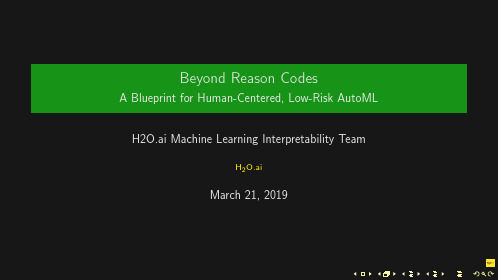
Beyond Reason CodesA Blueprint for Human-Centered,Low-Risk AutoML H2O.ai Machine Learning Interpretability TeamH2O.aiMarch21,2019ContentsBlueprintEDABenchmarkTrainingPost-Hoc AnalysisReviewDeploymentAppealIterateQuestionsBlueprintThis mid-level technical document provides a basic blueprint for combining the best of AutoML,regulation-compliant predictive modeling,and machine learning research in the sub-disciplines of fairness,interpretable models,post-hoc explanations,privacy and security to create a low-risk,human-centered machine learning framework.Look for compliance mode in Driverless AI soon.∗Guidance from leading researchers and practitioners.Blueprint†EDA and Data VisualizationKnow thy data.Automation implemented inDriverless AI as AutoViz.OSS:H2O-3AggregatorReferences:Visualizing Big DataOutliers through DistributedAggregation;The Grammar ofGraphicsEstablish BenchmarksEstablishing a benchmark from which to gauge improvements in accuracy,fairness, interpretability or privacy is crucial for good(“data”)science and for compliance.Manual,Private,Sparse or Straightforward Feature EngineeringAutomation implemented inDriverless AI as high-interpretabilitytransformers.OSS:Pandas Profiler,Feature ToolsReferences:Deep Feature Synthesis:Towards Automating Data ScienceEndeavors;Label,Segment,Featurize:A Cross Domain Framework forPrediction EngineeringPreprocessing for Fairness,Privacy or SecurityOSS:IBM AI360References:Data PreprocessingTechniques for Classification WithoutDiscrimination;Certifying andRemoving Disparate Impact;Optimized Pre-processing forDiscrimination Prevention;Privacy-Preserving Data MiningRoadmap items for H2O.ai MLI.Constrained,Fair,Interpretable,Private or Simple ModelsAutomation implemented inDriverless AI as GLM,RuleFit,Monotonic GBM.References:Locally InterpretableModels and Effects Based onSupervised Partitioning(LIME-SUP);Explainable Neural Networks Based onAdditive Index Models(XNN);Scalable Bayesian Rule Lists(SBRL)LIME-SUP,SBRL,XNN areroadmap items for H2O.ai MLI.Traditional Model Assessment and DiagnosticsResidual analysis,Q-Q plots,AUC andlift curves confirm model is accurateand meets assumption criteria.Implemented as model diagnostics inDriverless AI.Post-hoc ExplanationsLIME,Tree SHAP implemented inDriverless AI.OSS:lime,shapReferences:Why Should I Trust You?:Explaining the Predictions of AnyClassifier;A Unified Approach toInterpreting Model Predictions;PleaseStop Explaining Black Box Models forHigh Stakes Decisions(criticism)Tree SHAP is roadmap for H2O-3;Explanations for unstructured data areroadmap for H2O.ai MLI.Interlude:The Time–Tested Shapley Value1.In the beginning:A Value for N-Person Games,19532.Nobel-worthy contributions:The Shapley Value:Essays in Honor of Lloyd S.Shapley,19883.Shapley regression:Analysis of Regression in Game Theory Approach,20014.First reference in ML?Fair Attribution of Functional Contribution in Artificialand Biological Networks,20045.Into the ML research mainstream,i.e.JMLR:An Efficient Explanation ofIndividual Classifications Using Game Theory,20106.Into the real-world data mining workflow...finally:Consistent IndividualizedFeature Attribution for Tree Ensembles,20177.Unification:A Unified Approach to Interpreting Model Predictions,2017Model Debugging for Accuracy,Privacy or SecurityEliminating errors in model predictions bytesting:adversarial examples,explanation ofresiduals,random attacks and“what-if”analysis.OSS:cleverhans,pdpbox,what-if toolReferences:Modeltracker:RedesigningPerformance Analysis Tools for MachineLearning;A Marauder’s Map of Security andPrivacy in Machine Learning:An overview ofcurrent and future research directions formaking machine learning secure and privateAdversarial examples,explanation ofresiduals,measures of epistemic uncertainty,“what-if”analysis are roadmap items inH2O.ai MLI.Post-hoc Disparate Impact Assessment and RemediationDisparate impact analysis can beperformed manually using Driverless AIor H2O-3.OSS:aequitas,IBM AI360,themisReferences:Equality of Opportunity inSupervised Learning;Certifying andRemoving Disparate ImpactDisparate impact analysis andremediation are roadmap items forH2O.ai MLI.Human Review and DocumentationAutomation implemented as AutoDocin Driverless AI.Various fairness,interpretabilityand model debugging roadmapitems to be added to AutoDoc.Documentation of consideredalternative approaches typicallynecessary for compliance.Deployment,Management and MonitoringMonitor models for accuracy,disparateimpact,privacy violations or securityvulnerabilities in real-time;track modeland data lineage.OSS:mlflow,modeldb,awesome-machine-learning-opsmetalistReference:Model DB:A System forMachine Learning Model ManagementBroader roadmap item for H2O.ai.Human AppealVery important,may require custom implementation for each deployment environment?Iterate:Use Gained Knowledge to Improve Accuracy,Fairness, Interpretability,Privacy or SecurityImprovements,KPIs should not be restricted to accuracy alone.Open Conceptual QuestionsHow much automation is appropriate,100%?How to automate learning by iteration,reinforcement learning?How to implement human appeals,is it productizable?ReferencesThis presentation:https:///navdeep-G/gtc-2019/blob/master/main.pdfDriverless AI API Interpretability Technique Examples:https:///h2oai/driverlessai-tutorials/tree/master/interpretable_ml In-Depth Open Source Interpretability Technique Examples:https:///jphall663/interpretable_machine_learning_with_python https:///navdeep-G/interpretable-ml"Awesome"Machine Learning Interpretability Resource List:https:///jphall663/awesome-machine-learning-interpretabilityAgrawal,Rakesh and Ramakrishnan Srikant(2000).“Privacy-Preserving Data Mining.”In:ACM Sigmod Record.Vol.29.2.URL:/cs/projects/iis/hdb/Publications/papers/sigmod00_privacy.pdf.ACM,pp.439–450.Amershi,Saleema et al.(2015).“Modeltracker:Redesigning Performance Analysis Tools for Machine Learning.”In:Proceedings of the33rd Annual ACM Conference on Human Factors in Computing Systems.URL: https:///en-us/research/wp-content/uploads/2016/02/amershi.CHI2015.ModelTracker.pdf.ACM,pp.337–346.Calmon,Flavio et al.(2017).“Optimized Pre-processing for Discrimination Prevention.”In:Advances in Neural Information Processing Systems.URL:/paper/6988-optimized-pre-processing-for-discrimination-prevention.pdf,pp.3992–4001.Feldman,Michael et al.(2015).“Certifying and Removing Disparate Impact.”In:Proceedings of the21th ACM SIGKDD International Conference on Knowledge Discovery and Data Mining.URL:https:///pdf/1412.3756.pdf.ACM,pp.259–268.Hardt,Moritz,Eric Price,Nati Srebro,et al.(2016).“Equality of Opportunity in Supervised Learning.”In: Advances in neural information processing systems.URL:/paper/6374-equality-of-opportunity-in-supervised-learning.pdf,pp.3315–3323.Hu,Linwei et al.(2018).“Locally Interpretable Models and Effects Based on Supervised Partitioning (LIME-SUP).”In:arXiv preprint arXiv:1806.00663.URL:https:///ftp/arxiv/papers/1806/1806.00663.pdf.Kamiran,Faisal and Toon Calders(2012).“Data Preprocessing Techniques for Classification Without Discrimination.”In:Knowledge and Information Systems33.1.URL:https:///content/pdf/10.1007/s10115-011-0463-8.pdf,pp.1–33.Kanter,James Max,Owen Gillespie,and Kalyan Veeramachaneni(2016).“Label,Segment,Featurize:A Cross Domain Framework for Prediction Engineering.”In:Data Science and Advanced Analytics(DSAA),2016 IEEE International Conference on.URL:/static/papers/DSAA_LSF_2016.pdf.IEEE,pp.430–439.Kanter,James Max and Kalyan Veeramachaneni(2015).“Deep Feature Synthesis:Towards Automating Data Science Endeavors.”In:Data Science and Advanced Analytics(DSAA),2015.366782015.IEEEInternational Conference on.URL:https:///EVO-DesignOpt/groupWebSite/uploads/Site/DSAA_DSM_2015.pdf.IEEE,pp.1–10.Keinan,Alon et al.(2004).“Fair Attribution of Functional Contribution in Artificial and Biological Networks.”In:Neural Computation16.9.URL:https:///profile/Isaac_Meilijson/publication/2474580_Fair_Attribution_of_Functional_Contribution_in_Artificial_and_Biological_Networks/links/09e415146df8289373000000/Fair-Attribution-of-Functional-Contribution-in-Artificial-and-Biological-Networks.pdf,pp.1887–1915.Kononenko,Igor et al.(2010).“An Efficient Explanation of Individual Classifications Using Game Theory.”In: Journal of Machine Learning Research11.Jan.URL:/papers/volume11/strumbelj10a/strumbelj10a.pdf,pp.1–18.Lipovetsky,Stan and Michael Conklin(2001).“Analysis of Regression in Game Theory Approach.”In:Applied Stochastic Models in Business and Industry17.4,pp.319–330.Lundberg,Scott M.,Gabriel G.Erion,and Su-In Lee(2017).“Consistent Individualized Feature Attribution for Tree Ensembles.”In:Proceedings of the2017ICML Workshop on Human Interpretability in Machine Learning(WHI2017).Ed.by Been Kim et al.URL:https:///pdf?id=ByTKSo-m-.ICML WHI2017,pp.15–21.Lundberg,Scott M and Su-In Lee(2017).“A Unified Approach to Interpreting Model Predictions.”In: Advances in Neural Information Processing Systems30.Ed.by I.Guyon et al.URL:/paper/7062-a-unified-approach-to-interpreting-model-predictions.pdf.Curran Associates,Inc.,pp.4765–4774.Papernot,Nicolas(2018).“A Marauder’s Map of Security and Privacy in Machine Learning:An overview of current and future research directions for making machine learning secure and private.”In:Proceedings of the11th ACM Workshop on Artificial Intelligence and Security.URL:https:///pdf/1811.01134.pdf.ACM.Ribeiro,Marco Tulio,Sameer Singh,and Carlos Guestrin(2016).“Why Should I Trust You?:Explaining the Predictions of Any Classifier.”In:Proceedings of the22nd ACM SIGKDD International Conference on Knowledge Discovery and Data Mining.URL:/kdd2016/papers/files/rfp0573-ribeiroA.pdf.ACM,pp.1135–1144.Rudin,Cynthia(2018).“Please Stop Explaining Black Box Models for High Stakes Decisions.”In:arXiv preprint arXiv:1811.10154.URL:https:///pdf/1811.10154.pdf.Shapley,Lloyd S(1953).“A Value for N-Person Games.”In:Contributions to the Theory of Games2.28.URL: http://www.library.fa.ru/files/Roth2.pdf#page=39,pp.307–317.Shapley,Lloyd S,Alvin E Roth,et al.(1988).The Shapley Value:Essays in Honor of Lloyd S.Shapley.URL: http://www.library.fa.ru/files/Roth2.pdf.Cambridge University Press.Vartak,Manasi et al.(2016).“Model DB:A System for Machine Learning Model Management.”In: Proceedings of the Workshop on Human-In-the-Loop Data Analytics.URL:https:///~matei/papers/2016/hilda_modeldb.pdf.ACM,p.14.Vaughan,Joel et al.(2018).“Explainable Neural Networks Based on Additive Index Models.”In:arXiv preprint arXiv:1806.01933.URL:https:///pdf/1806.01933.pdf.Wilkinson,Leland(2006).The Grammar of Graphics.—(2018).“Visualizing Big Data Outliers through Distributed Aggregation.”In:IEEE Transactions on Visualization&Computer Graphics.URL:https:///~wilkinson/Publications/outliers.pdf.Yang,Hongyu,Cynthia Rudin,and Margo Seltzer(2017).“Scalable Bayesian Rule Lists.”In:Proceedings of the34th International Conference on Machine Learning(ICML).URL:https:///pdf/1602.08610.pdf.。
多智能体强化学习的几种BestPractice
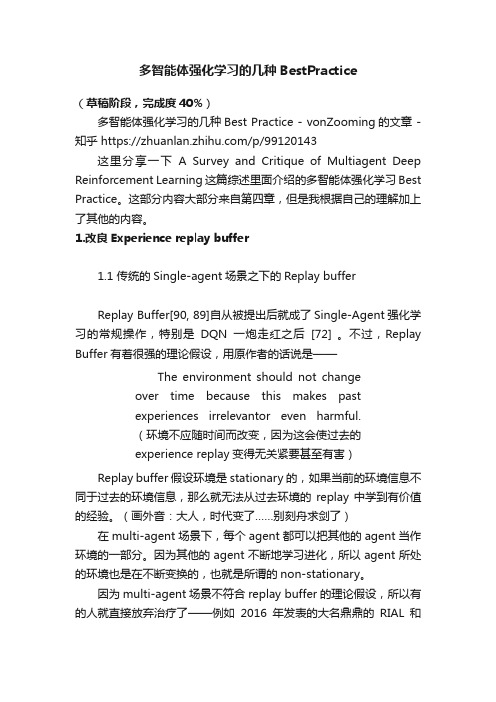
多智能体强化学习的几种BestPractice(草稿阶段,完成度40%)多智能体强化学习的几种Best Practice - vonZooming的文章 - 知乎 https:///p/99120143这里分享一下A Survey and Critique of Multiagent Deep Reinforcement Learning这篇综述里面介绍的多智能体强化学习Best Practice。
这部分内容大部分来自第四章,但是我根据自己的理解加上了其他的内容。
1.改良Experience replay buffer1.1 传统的Single-agent场景之下的Replay bufferReplay Buffer[90, 89]自从被提出后就成了Single-Agent强化学习的常规操作,特别是DQN一炮走红之后[72] 。
不过,Replay Buffer有着很强的理论假设,用原作者的话说是——The environment should not changeover time because this makes pastexperiences irrelevantor even harmful.(环境不应随时间而改变,因为这会使过去的experience replay变得无关紧要甚至有害)Replay buffer假设环境是stationary的,如果当前的环境信息不同于过去的环境信息,那么就无法从过去环境的replay中学到有价值的经验。
(画外音:大人,时代变了……别刻舟求剑了)在multi-agent场景下,每个agent都可以把其他的agent当作环境的一部分。
因为其他的agent不断地学习进化,所以agent所处的环境也是在不断变换的,也就是所谓的non-stationary。
因为multi-agent场景不符合replay buffer的理论假设,所以有的人就直接放弃治疗了——例如2016年发表的大名鼎鼎的RIAL和DIAL中就没有使用replay buffer。
A Comprehensive Survey of Multiagent Reinforcement Learning
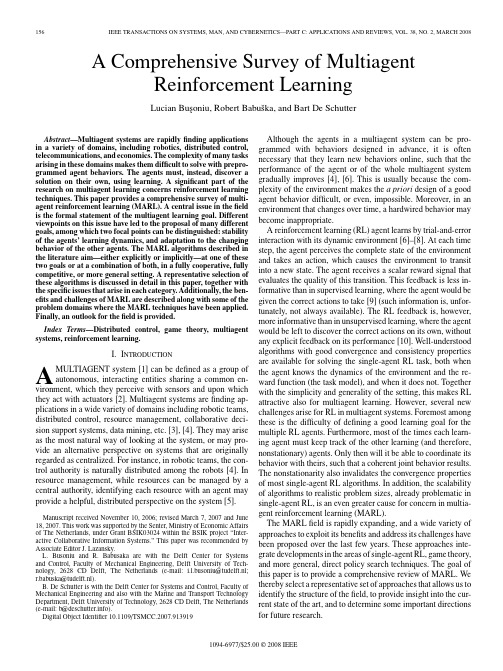
IEEE TRANSACTIONS ON SYSTEMS, MAN, AND CYBERNETICS—PART C: APPLICATIONS AND REVIEWS, VOL. 38, NO. 2, MARCH 2008
A Comprehensive Survey of Multiagent ReinfoN
A
MULTIAGENT system [1] can be defined as a group of autonomous, interacting entities sharing a common environment, which they perceive with sensors and upon which they act with actuators [2]. Multiagent systems are finding applications in a wide variety of domains including robotic teams, distributed control, resource management, collaborative decision support systems, data mining, etc. [3], [4]. They may arise as the most natural way of looking at the system, or may provide an alternative perspective on systems that are originally regarded as centralized. For instance, in robotic teams, the control authority is naturally distributed among the robots [4]. In resource management, while resources can be managed by a central authority, identifying each resource with an agent may provide a helpful, distributed perspective on the system [5].
深度强化学习在游戏人工智能中的应用

深度强化学习在游戏人工智能中的应用IntroductionDeep reinforcement learning is a subset of AI and machine learning, which uses algorithms to train a machine to learn from its own experiences in an environment. In recent years, it has become an integral part of game AI, allowing computers to learn how to play and solve complex tasks. From classic games like chess and Go, deep reinforcement learning has demonstrated its power in building intelligent game-playing agents that can outperform human players.BackgroundGame AI has been a focus of research for several decades, and the use of reinforcement learning in game AI can be traced back to the development of the TD-Gammon algorithm in the late 1990s. This algorithm was able to train a computer to play backgammon at a world-class level, using a combination of neural networks and reinforcement learning techniques.In recent years, the development of deep neural networks has revolutionized the field of reinforcement learning. Deep learning has enabled computers to learn from large and complex datasets, which includes playing games. Deep reinforcement learning has been used to solve various complex games such as Atari games, Dota 2, and Starcraft 2.Applications of Deep Reinforcement Learning in Gaming1) Atari GamesAtari games were an early benchmark for deep reinforcement learning in gaming. In 2013, Google DeepMind created a deep reinforcement learning algorithm, known as the Deep Q-Network (DQN), which was able to achieve human-level performance on several Atari games. The algorithm used a deep neural network to predict the Q-value or the expected reward for each possible action in the game. The DQN algorithm was asignificant milestone in artificial intelligence and helped to increase the interest in deep reinforcement learning.2) Dota 2Dota 2 is a complex strategy game that involves multiple players, each controlling a hero with unique abilities. OpenAI collaborated with Valve to create a bot called OpenAI Five, which used deep reinforcement learning to beat world-class players. The bot was able to learn from its experiences in the game and improve its strategy over time. This demonstration showed the power of deep reinforcement learning in complex multi-agent environments.3) Starcraft 2Starcraft 2 is another complex strategy game that involves multiple players and a large number of units. DeepMind collaborated with Blizzard Entertainment to create AlphaStar, an AI agent that was able to beat professional players on the highest difficulty level. AlphaStar used deep reinforcement learning to learn from its own experiences and improve its gameplay over time.ConclusionDeep reinforcement learning has demonstrated significant potential in gaming. It has shown the ability to solve complex games and has opened up new avenues for research in artificial intelligence. The use of deep reinforcement learning in gaming is not limited to entertainment but can also be used in real-world applications such as robotics and autonomous vehicles. As the field of deep reinforcement learning continues to grow, it is exciting to see the many ways in which it will continue to innovate and transform the world around us.。
外研版七年级英语上册Unit 5 综合测试卷含答案
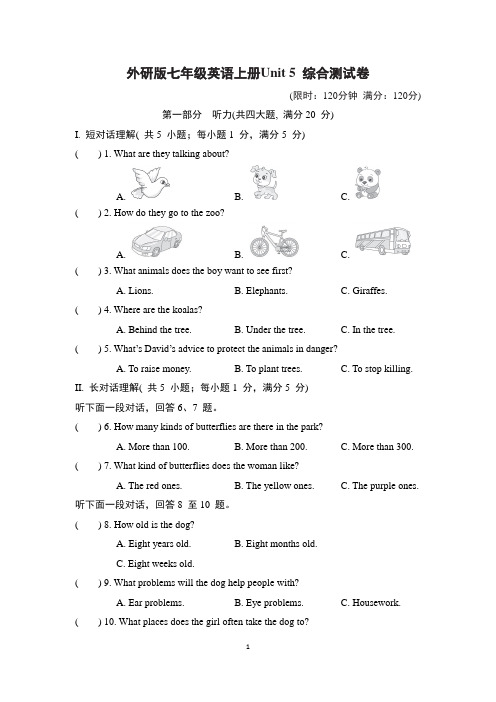
外研版七年级英语上册Unit 5 综合测试卷(限时:120分钟满分:120分)第一部分听力(共四大题, 满分20 分)I. 短对话理解( 共5 小题;每小题1 分,满分5 分)( ) 1. What are they talking about?A. B. C.( ) 2. How do they go to the zoo?A. B. C.( ) 3. What animals does the boy want to see first?A. Lions.B. Elephants.C. Giraffes. ( ) 4. Where are the koalas?A. Behind the tree.B. Under the tree.C. In the tree. ( ) 5. What’s David’s advice to protect the animals in danger?A. To raise money.B. To plant trees.C. To stop killing. II. 长对话理解( 共5 小题;每小题1 分,满分5 分)听下面一段对话,回答6、7 题。
( ) 6. How many kinds of butterflies are there in the park?A. More than 100.B. More than 200.C. More than 300. ( ) 7. What kind of butterflies does the woman like?A. The red ones.B. The yellow ones.C. The purple ones. 听下面一段对话,回答8 至10 题。
( ) 8. How old is the dog?A. Eight years old.B. Eight months old.C. Eight weeks old.( ) 9. What problems will the dog help people with?A. Ear problems.B. Eye problems.C. Housework. ( ) 10. What places does the girl often take the dog to?A. Post offices.B. Bus stops.C. Parks.III. 短文理解(共5 小题; 每小题1 分,满分5 分)( ) 11. How tall is a giraffe at birth?A. About 5 feet.B. About 6 feet.C. About 7 feet. ( ) 12. When can a baby giraffe stand up?A. When it is 20 minutes old.B. When it is 30 minutes old.C. When it is 3 hours old.( ) 13. How long can camels live without water in winter?A. For about a week.B. For about a month.C. For about half a year.( ) 14. How many years can elephants live up to?A. 70.B. 90.C. 100. ( ) 15. How long does an elephant spend eating food every day?A. 8 hours.B. 12 hours.C. 16 hours. IV. 信息转换(共5 小题;每小题1 分,满分5 分)第二部分语言知识运用( 共三大题,满分35 分)V. 单项填空( 共10 小题;每小题1 分,满分10 分)( ) 21. Look! There are four ________ and two lions in the zoo.A. deersB. foxC. wolfD. wolves ( ) 22. I don’t like lions because they are really ________.A. scaryB. interestingC. smartD. friendly( ) 23. Some pigeons can fly several thousand kilometers and don’t ________.A. get upB. get offC. get lostD. get back ( ) 24. He was wearing a pair of sunglasses and I didn’t ________ him at first.A. adviseB. promiseC. recogniseD. hear ( ) 25. —Hello, is Molly there?—Sorry, she ________ her father’s car outside.A. washesB. is washingC. washedD. wash ( ) 26. Now, some new energy vehicles (新能源汽车) can run ________ the speed of over 150 kilometers per hour.A. atB. aboutC. fromD. with ( ) 27. Don’t worry about the boys and girls. I think they can look after ________ well.A. himselfB. ourselvesC. myselfD. themselves ( ) 28. People call beavers “nature’s ________” because they are very creative (有创造力的) in building their houses.A. doctorsB. engineersC. teachersD. farmers ( ) 29. ________, humans should make friends with animals. Then the world will be more beautiful.A. In my opinionB. In surpriseC. As a resultD. In a moment( ) 30. —Look, Mum! ________ fish! It looks like a ball.—It’s a puffer fish. It can puff up to be a ball when f acing danger.A. How unusualB. What unusualC. What a usualD. What an unusualVI. 完形填空( 共20 小题;每小题1 分,满分20 分)AHi, I’m Fu Zai, a 6-month-old corgi (柯基). I’m China’s 31corgi police dog! No corgi was a police dog before. Some people thinkI can’t be a great police dog because of my 32 legs. But I’m here toprove (证明) them 33 ! I was a pet dog. When I was 2 months old, one day while I was playing with my owner in the park, a(n) 34 saw me and thought I had the gift to be a police dog. Then, I started my training. Every day, I 35 in the morning and afternoon. I learn cool things like 36 to listen well, find bombs (炸弹), and use my nose to sniff out (嗅出) 37 things, such as drugs (毒品). My short legs make me stand out! I can sneak (偷偷进入) under cars or get into and search small spaces easily. Bigger dogs can’t do this 38 I can! My only 39 is that I can’t run very fast. When we are outside doing our job, my trainer 40 needs to give me a piggyback (在背上的) ride. It’s one of my favorite parts of the job.( ) 31. A. first B. second C. third D. last( ) 32. A. strong B. long C. short D. weak ( ) 33. A. right B. clever C. wrong D. smart ( ) 34. A. policeman B. actor C. writer D. farmer ( ) 35. A. take a walk B. take classesC. go to workD. take a trip( ) 36. A. how B. what C. when D. where ( ) 37. A. fresh B. delicious C. dangerous D. amazing ( ) 38. A. so B. but C. and D. or( ) 39. A. problem B. interest C. gift D. chance ( ) 40. A. never B. seldom C. always D. hardlyBIt says that bats (蝙蝠) are almost blind (瞎的), but 41 does the bat find the way? In fact, bats find their ways 42 their ears’ help. Bats 43 a sound, but we can’t hear it at all. They cry when they fly and the echoes (回声) of these cries come back to their 44 . In this way they can know where they should go. Bats fly out in the 45 . In the daytime, they just stay together in 46 homes.When the evening comes, they begin to 47 and look for food. The next morning, they come back from work to sleep 48 the evening. Some people 49 that bats are bad animals. In fact, they are very 50 . They catch and eat pests (害虫). This is very good for people.( ) 41. A. what B. how C. when D. where ( ) 42. A. with B. at C. on D. in( ) 43. A. make B. shout C. play D. open ( ) 44. A. eyes B. faces C. ears D. mouths ( ) 45. A. morning B. afternoon C. day D. evening ( ) 46. A. their B. our C. his D. her( ) 47. A. come in B. go outC. try onD. have a rest( ) 48. A. after B. as C. till D. when ( ) 49. A. see B. think C. speak D. have ( ) 50. A. good B. bad C. rude D. careful VII. 补全对话,其中有两项多余( 共5 小题;每小题1 分,满分5 分) Tom: Mom, can you buy me a pet ( 宠物)?Mom: 51. _________Tom: I want a monkey. I like it very much.Mom: 52. _________ It will eat your bananas.Tom: 53. _________ It is cute.Mom: A baby dog? No, you have to feed (喂养) it with your milk by yourself. Tom: Well, Mom... Oh, I can have an elephant. 54. _________Mom: 55. _________You can have a pet, but it can’t be big, and it can’t have your milk or eat your bananas. It can play with you and help you with your study. Tom: Well, I know. Thank you, Mom. But what is it?Mom: Your daddy!G. How about a baby dog?第三部分阅读( 共两节,满分40 分)VIII. 阅读理解(共20 小题;每小题2 分,满分40 分)第一节阅读下列短文,从每小题所给的A、B、C、D 四个选项中选出最佳选项。
有没有兴趣玩游戏英语作文
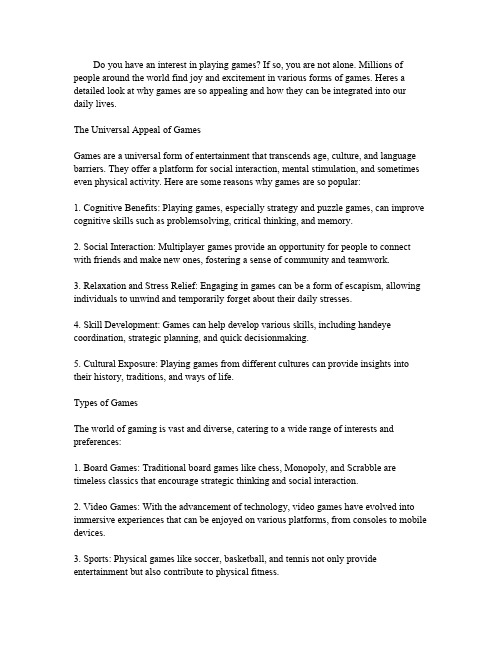
Do you have an interest in playing games?If so,you are not lions of people around the world find joy and excitement in various forms of games.Heres a detailed look at why games are so appealing and how they can be integrated into our daily lives.The Universal Appeal of GamesGames are a universal form of entertainment that transcends age,culture,and language barriers.They offer a platform for social interaction,mental stimulation,and sometimes even physical activity.Here are some reasons why games are so popular:1.Cognitive Benefits:Playing games,especially strategy and puzzle games,can improve cognitive skills such as problemsolving,critical thinking,and memory.2.Social Interaction:Multiplayer games provide an opportunity for people to connect with friends and make new ones,fostering a sense of community and teamwork.3.Relaxation and Stress Relief:Engaging in games can be a form of escapism,allowing individuals to unwind and temporarily forget about their daily stresses.4.Skill Development:Games can help develop various skills,including handeye coordination,strategic planning,and quick decisionmaking.5.Cultural Exposure:Playing games from different cultures can provide insights into their history,traditions,and ways of life.Types of GamesThe world of gaming is vast and diverse,catering to a wide range of interests and preferences:1.Board Games:Traditional board games like chess,Monopoly,and Scrabble are timeless classics that encourage strategic thinking and social interaction.2.Video Games:With the advancement of technology,video games have evolved into immersive experiences that can be enjoyed on various platforms,from consoles to mobile devices.3.Sports:Physical games like soccer,basketball,and tennis not only provide entertainment but also contribute to physical fitness.4.Card Games:From simple games like Go Fish to complex ones like Poker,card games offer a wide range of challenges and strategies.5.RolePlaying Games RPGs:These games allow players to assume the roles of characters in fictional settings,often involving complex narratives and character development.Incorporating Games into Daily LifeIntegrating games into your daily routine can be both enjoyable and beneficial:1.Family Game Nights:Set aside time for family members to play games together, promoting bonding and shared experiences.cational Integration:Use games as a tool for learning in educational settings, making lessons more engaging and interactive.3.Work Breaks:Encourage short gaming sessions during work breaks to help employees relax and recharge.munity Events:Participate in or organize community game events to bring people together and promote social interaction.5.Personal Development:Use games as a means for personal growth,setting goals and tracking progress within the gaming environment.In conclusion,games offer a myriad of benefits and can be a valuable addition to ones lifestyle.Whether you prefer the strategic depth of chess,the adrenaline rush of a firstperson shooter,or the immersive world of an RPG,theres a game out there for everyone.So,the next time youre looking for a way to relax,connect with others,or challenge your mind,consider turning to the world of games.。
DeepWalk论文精读:(2)核心算法

DeepWalk论⽂精读:(2)核⼼算法模块21. 核⼼思想及其形成过程DeepWalk结合了两个不相⼲领域的模型与算法:随机游⾛(Random Walk)及语⾔模型(Language Modeling)。
1.1 随机游⾛由于⽹络嵌⼊需要提取节点的局部结构,所以作者很⾃然地想到可以使⽤随机游⾛算法。
随机游⾛是相似性度量的⼀种⽅法,曾被⽤于内容推荐[11]、社区识别[1, 38]等。
除了捕捉局部结构,随机游⾛还有以下两个好处:1. 容易并⾏,使⽤不同的线程、进程、机器,可以同时在⼀个图的不同部分运算;2. 关注节点的局部结构使得模型能更好地适应变化中的⽹络,在⽹络发⽣微⼩变化时,⽆需在整个图上重新计算。
1.2 语⾔模型语⾔模型(Language Modeling)有⼀次颠覆性创新[26, 27],体现在以下三点:1. 从原来的利⽤上下⽂预测缺失单词,变为利⽤⼀个单词预测上下⽂;2. 不仅考虑了给定单词左侧的单词,还考虑了右侧的单词;3. 将给定单词前后⽂打乱顺序,不再考虑单词的前后顺序因素。
这些特性⾮常适合节点的表⽰学习问题:第⼀点使得较长的序列也可以在允许时间内计算完毕;第⼆、三点的顺序⽆关使得学习到的特征能更好地表征“临近”这⼀概念的对称性。
1.3 如何结合可以结合的原因,是作者观察到:1. 如果不论规模⼤⼩,节点的度服从幂律分布,那么在随机游⾛序列上节点的出现频率也应当服从幂律分布。
2. ⾃然语⾔中词频服从幂律分布,⾃然语⾔模型可以很好地处理这种分布。
所以作者将⾃然语⾔模型中的⽅法迁移到了⽹络社群结构问题上,这也正是DeepWalk的⼀个核⼼贡献。
2. 具体算法算法由两部分组成:第⼀部分是随机游⾛序列⽣成器,第⼆部分是向量更新。
2.1 随机游⾛⽣成器DeepWalk⽤$W_{v_i}$表⽰以$v_i$为根节点的随机游⾛序列,它的⽣成⽅式是:从$v_i$出发,随机访问⼀个邻居,然后再访问邻居的邻居...每⼀步都访问前⼀步所在节点的邻居,直到序列长度达到设定的最⼤值$t$。
玩游戏的好处英语作文

Playing games is a popular pastime that offers a multitude of benefits. Here are some of the advantages of engaging in this activity:1. Enhances Cognitive Skills: Games, especially puzzle and strategy games, can improve memory, concentration, and problemsolving abilities. They challenge the brain to think critically and creatively.2. Stress Relief: Engaging in games can be a great way to unwind and relieve stress aftera long day. They provide a temporary escape from daily worries and can help to clear the mind.3. Social Interaction: Multiplayer games foster social interaction and teamwork. They allow players to connect with others, build relationships, and develop communication skills.4. Improves HandEye Coordination: Fastpaced games, such as action or sports games, can help improve handeye coordination and reflexes, which are essential skills in many areas of life.5. Encourages Learning: Educational games are designed to teach new concepts and skills in a fun and interactive way. They can make learning more engaging, especially for children.6. Boosts Mood: Winning a game or achieving a high score can give a sense of accomplishment and boost selfesteem. It can also release endorphins, which are natural mood elevators.7. Cultivates Patience: Games that require strategy and planning can teach patience and perseverance. Players learn to wait for the right moment to make their moves.8. Promotes Physical Activity: Active or sports games encourage physical movement. Games like Wii Sports or Dance Dance Revolution get players up and moving, contributing to a healthier lifestyle.9. Develops Strategic Thinking: Games that involve planning and foresight can sharpen strategic thinking skills. This can be beneficial in various aspects of life, including work and personal decisionmaking.10. Provides Entertainment: Ultimately, games are a source of entertainment. They provide enjoyment and can be a fun way to spend leisure time.In conclusion, playing games can be a beneficial and enjoyable activity that offers cognitive, emotional, and social advantages. Its important, however, to maintain a balance and not let gaming interfere with other important aspects of life.。
- 1、下载文档前请自行甄别文档内容的完整性,平台不提供额外的编辑、内容补充、找答案等附加服务。
- 2、"仅部分预览"的文档,不可在线预览部分如存在完整性等问题,可反馈申请退款(可完整预览的文档不适用该条件!)。
- 3、如文档侵犯您的权益,请联系客服反馈,我们会尽快为您处理(人工客服工作时间:9:00-18:30)。
Scalable Learning in Stochastic GamesMichael Bowling and Manuela VelosoComputer Science DepartmentCarnegie Mellon UniversityPittsburgh PA,15213-3891AbstractStochastic games are a general model of interaction betweenmultiple agents.They have recently been the focus of a greatdeal of research in reinforcement learning as they are bothdescriptive and have a well-defined Nash equilibrium solu-tion.Most of this recent work,although very general,hasonly been applied to small games with at most hundreds ofstates.On the other hand,there are landmark results of learn-ing being successfully applied to specific large and complexgames such as Checkers and Backgammon.In this paper wedescribe a scalable learning algorithm for stochastic games,that combines three separate ideas from reinforcement learn-ing into a single algorithm.These ideas are tile coding forgeneralization,policy gradient ascent as the basic learningmethod,and our previous work on the WoLF(“Win or LearnFast”)variable learning rate to encourage convergence.Weapply this algorithm to the intractably sized game-theoreticcard game Goofspiel,showing preliminary results of learn-ing in self-play.We demonstrate that policy gradient ascentcan learn even in this highly non-stationary problem with si-multaneous learning.We also show that the WoLF principlecontinues to have a converging effect even in large problemswith approximation and generalization.IntroductionWe are interested in the problem of learning in multiagentenvironments.One of the main challenges with these en-vironments is that other agents in the environment may belearning and adapting as well.These environments are,therefore,no longer stationary.They violate the Markovproperty that traditional single-agent behavior learning re-lies upon.The model of stochastic games captures these problemsvery well through explicit models of the reward functionsof the other agents and their affects on transitions.Theyare also a natural extension of Markov decision processes(MDPs)to multiple agents and so have attracted interestfrom the reinforcement learning community.The prob-lem of simultaneouslyfinding optimal policies for stochasticgames has been well studied in thefield of game theory.Thetraditional solution concept is that of Nash equilibria,a pol-icy for all the players where each is playing optimally withThis looks very similar to the MDP framework except wehave multiple agents selecting actions and the next state and rewards depend on the joint action of the agents.Anotherimportant difference is that each agent has its own separatereward function.The goal for each agent is to select actions in order to maximize its discounted future rewards with dis-count factor.Stochastic games are a very natural extension of MDPsto multiple agents.They are also an extension of matrixgames to multiple states.Two example matrix games are in Figure1.In these games there are two players;one se-lects a row and the other selects a column of the matrix.Theentry of the matrix they jointly select determines the pay-offs.The games in Figure1are zero-sum games,so the rowplayer would receive the payoff in the matrix,and the col-umn player would receive the negative of that payoff.In the general case(general-sum games),each player would havea separate matrix that determines their payoffs.Matching Pennies R-P-SFigure1:Matching Pennies and Rock-Paper-Scissors matrix games.Each state in a stochastic game can be viewed as a matrixgame with the payoffs for each joint action determined bythe matrices.After playing the matrix game and receiving their payoffs the players are transitioned to anotherstate(or matrix game)determined by their joint action.Wecan see that stochastic games then contain both MDPs and matrix games as subsets of the framework.Stochastic Policies.Unlike in single-agent settings,de-terministic policies in multiagent settings can often be ex-ploited by the other agents.Consider the matching pen-nies matrix game as shown in Figure1.If the column player were to play either action deterministically,the row player could win every time.This requires us to consider mixed strategies and stochastic policies.A stochastic pol-icy,,is a function that maps states to mixed strategies,which are probability distributions over the player’s actions.Nash Equilibria.Even with the concept of mixed strate-gies there are still no optimal strategies that are independent of the other players’strategies.We can,though,define a no-tion of best-response.A strategy is a best-response to the other players’strategies if it is optimal given their strategies. The major advancement that has driven much of the devel-opment of matrix games,game theory,and even stochastic games is the notion of a best-response equilibrium,or Nash equilibrium(Nash,Jr.1950).A Nash equilibrium is a collection of strategies for each of the players such that each player’s strategy is a best-response to the other players’strategies.So,no player can do better by changing strategies given that the other players also don’t change strategies.What makes the notion of equilibrium compelling is that all matrix games have such an equilib-rium,possibly having multiple equilibria.Zero-sum,two-player games,where one player’s payoffs are the negative of the other,have a single Nash equilibrium.1In the zero-sum examples in Figure1,both games have an equilibrium con-sisting of each player playing the mixed strategy where all the actions have equal probability.The concept of equilibria also extends to stochastic games.This is a non-trivial result,proven by Shapley(Shap-ley1953)for zero-sum stochastic games and by Fink(Fink 1964)for general-sum stochastic games.Learning in Stochastic Games.Stochastic games have been the focus of recent research in the area of reinforce-ment learning.There are two different approaches be-ing explored.Thefirst is that of algorithms that explic-itly learn equilibria through experience,independent of the other players’policy(Littman1994;Hu&Wellman1998; Greenwald&Hall2002).These algorithms iteratively es-timate value functions,and use them to compute an equi-librium for the game.A second approach is that of best-response learners(Claus&Boutilier1998;Singh,Kearns, &Mansour2000;Bowling&Veloso2002a).These learn-ers explicitly optimize their reward with respect to the other players’(changing)policies.This approach,too,has a strong connection to equilibria.If these algorithms converge when playing each other,then they must do so to an equilib-rium(Bowling&Veloso2001).Neither of these approaches,though,have been scaled beyond games with a few hundred states.Games with a very large number of states,or games with continuous state spaces,make state enumeration intractable.Since previ-ous algorithms in their stated form require the enumera-tion of states either for policies or value functions,this is a major limitation.In this paper we examine learning in a very large stochastic game,using approximation and gener-alization techniques.Specifically,we will build on the idea of best-response learners using gradient techniques(Singh, Kearns,&Mansour2000;Bowling&Veloso2002a).We first describe an interesting game with an intractably large state space.GoofspielGoofspiel(or The Game of Pure Strategy)was invented by Merrill Flood while at Princeton(Flood1985).The game has numerous variations,but here we focus on the simple two-player,-card version.Each player receives a suit of cards numbered through,a third suit of cards is shuf-fled and placed face down as the deck.Each round the next card isflipped over from the deck,and the two players each select a card placing it face down.They are revealed si-multaneously and the player with the highest card wins the card from the deck,which is worth its number in points.Ifthe players choose the same valued card,then neither player gets any points.Regardless of the winner,both players dis-card their chosen card.This is repeated until the deck and players hands are exhausted.The winner is the player with the most points.This game has numerous interesting properties making it a very interesting step between toy problems and more re-alistic problems.First,notice that this game is zero-sum,and as with many zero-sum games any deterministic strat-egy can be soundly defeated.In this game,it’s by simply playing the card one higher than the other player’s deter-ministically chosen card.Second,notice that the number of states and state-action pairs grows exponentially with the number of cards.The standard size of the game is so large that just storing one player’s policy or -table would require approximately 2.5terabytes of space.Just gather-ing data on all the state-action transitions would require welloverplayings of the game.Table 1shows the number of states and state-action pairs as well as the policy size for three different values of .This game obviously requires some form of generalization to make learning possible.An-other interesting property is that randomly selecting actions is a reasonably good policy.The worst-case values of the random policy along with the worst-case values of the best deterministic policy are also shown in Table 1.This game can be described using the stochastic game model.The state is the current cards in the players’hands and deck along with the upturned card.The actions for a player are the cards in the player’s hand.The transitions fol-low the rules as described,with an immediate reward going to the player who won the upturned card.Since the game has a finite end and we are interested in maximizing total reward,we can set the discount factor to be .Although equi-librium learning techniques such as Minimax-Q (Littman 1994)are guaranteed to find the game’s equilibrium,it re-quires maintaining a state-joint-action table of values.Thistable would requireterabytes to store for the card game.We will now describe a best-response learn-ing algorithm using approximation techniques to handle the enormous state space.Three Ideas –One AlgorithmThe algorithm we will use combines three separate ideas from reinforcement learning.The first is the idea of tile coding as a generalization for linear function approximation.The second is the use of a parameterized policy and learning as gradient ascent in the policy’s parameter space.The final component is the use of a WoLF variable learning rate to ad-just the gradient ascent step size.We will briefly overview these three techniques and then describe how they are com-bined into a reinforcement learning algorithm for Goofspiel.Tile Coding.Tile coding (Sutton &Barto 1998),also known as CMACS,is a popular technique for creating a set of boolean features from a set of continuous features.In reinforcement learn-ing,tile coding has been used extensively to create linear approximators of state-action values (e.g.,(Stone &Sutton2001)).Figure 2:An example of tile coding a two dimensional spacewith two overlapping tilings.The basic idea is to lay offset grids or tilings over the mul-tidimensional continuous feature space.A point in the con-tinuous feature space will be in exactly one tile for each of the offset tilings.Each tile has an associated boolean vari-able,so the continuous feature vector gets mapped into a very high-dimensional boolean vector.In addition,nearby points will fall into the same tile for many of the offset grids,and so share many of the same boolean variables in their re-sulting vector.This provides the important feature of gen-eralization.An example of tile coding in a two-dimensional continuous space is shown in Figure 2.This example shows two overlapping tilings,and so any given point falls into two different tiles.Another common trick with tile coding is the use of hash-ing to keep the number of parameters manageable.Each tile is hashed into a table of fixed size.Collisions are sim-ply ignored,meaning that two unrelated tiles may share the same parameter.Hashing reduces the memory requirements with little loss in performance.This is because only a small fraction of the continuous space is actually needed or visited while learning,and so independent parameters for every tile are often not necessary.Hashing provides a means for using only the number of parameters the problem requires while not knowing in advance which state-action pairs need pa-rameters.Policy Gradient AscentPolicy gradient techniques (Sutton et al.2000;Baxter &Bartlett 2000)are a method of reinforcement learning with function approximation.Traditional approaches approxi-mate a state-action value function,and result in a deter-ministic policy that selects the action with the maximum learned value.Alternatively,policy gradient approaches ap-proximate a policy directly,and then use gradient ascent to adjust the parameters to maximize the policy’s value.There are three good reasons for the latter approach.First,there’s a whole body of theoretical work describing conver-gence problems using a variety of value-based learning tech-niques with a variety of function approximation techniques (See (Gordon 2000)for a summary of these results.)Second,value-based approaches learn deterministic policies,and as we mentioned earlier deterministic policies in multiagentV ALUE(det)V ALUE(random)6921515059KB47MB2.5TBTheir main result was a convergence proof for the followingpolicy iteration rule that updates a policy’s parameters,The WoLF principle naturally lends itself to policy gra-dient techniques where there is a well-defined learning rate, .With WoLF we replace the original learning rate withtwo learning rates to be used when winningor losing,respectively.One determination of winning and losing that has been successful is to compare the value ofthe current policy to the value of the average policy over time.With the policy gradient technique above we can define a similar rule that examines the approximatevalue,using,of the current weight vector with the av-erage weight vector over time.Specifically,we are“win-ning”if and only if,(5)When winning in a particular state,we update the parame-ters for that state using,otherwise.Learning in GoofspielWe combine these three techniques in the obvious way.Tile coding provides a large boolean feature vector for any state-action pair.This is used both for the parameterization of the policy and for the approximation of the policy’s value,which is used to compute the policy’s gradient.Gradient updates are then performed on both the policy using equation3and the value estimate using equation4.WoLF is used to vary the learning rate in the policy update according to the rule in inequality5.This composition can be essentially thought of as an actor-critic method(Sutton&Barto1998).Here the Gibbs distribution over the set of parameters is the actor, and the gradient-descent Sarsa(0)is the critic.Tile-coding provides the necessary parameterization of the state.The WoLF principle is adjusting how the actor changes policies based on response from the critic.The main detail yet to be explained and where the algo-rithm is specifically adapted to Goofspiel is in the tile cod-ing.The method of tiling is extremely important to the over-all performance of learning as it is a powerful bias on what policies can and will be learned.The major decision to be made is how to represent the state as a vector of numbers and which of these numbers are tiled together.Thefirst decision determines what states are distinguishable,and the second determines how generalization works across distinguishable states.Despite the importance of the tiling we essentially selected what seemed like a reasonable tiling,and used it throughout our results.We represent a set of cards,either a player’s hand or the deck,byfive numbers,corresponding to the value of the card that is the minimum,lower quartile,median,upper quartile, and maximum.This provides information as to the general shape of the set,which is what is important in Goofspiel. The other values used in the tiling are the value of the card that is being bid on and the card corresponding to the agent’s action.An example of this process in the13-card game is shown in Table2.These values are combined together into three tilings.Thefirst tiles together the quartiles describing the players’hands.The second tiles together the quartiles of the deck with the card available and player’s action.The last tiles together the quartiles of the opponent’s hand with the card available and player’s action.The tilings use tile sizes equal to roughly half the number of cards in the game with the number of tilings greater than the tile sizes to distinguish between any integer state values.Finally,these tiles were all then hashed into a table of size one million in order to keep the parameter space manageable.We don’t suggest that this is a perfect or even good tiling for this domain,but as we will show the results are still interesting.ResultsOne of the difficult and open issues in multiagent reinforce-ment learning is that of evaluation.Before presenting learn-ing results wefirst need to look at how one evaluates learn-ing success.EvaluationOne straightforward evaluation technique is to have two learning algorithms learn against each other and simply ex-amine the expected reward over time.This technique is not useful if one’s interested in learning in self-play,where both players use an identical algorithm.In this case with a sym-metric zero-sum game like Goofspiel,the expected reward of the two agents is necessarily zero,providing no informa-tion.Another common evaluation criterion is that of conver-gence.This is true in single-agent learning as well as mul-tiagent learning.One strong motivation for considering this criterion in multiagent domains is the connection of conver-gence to Nash equilibrium.If algorithms that are guaran-teed to converge to optimal policies in stationary environ-ments,converge in a multiagent learning environment,then the resulting joint policy must be a Nash equilibrium of the stochastic game(Bowling&Veloso2002a).Although,convergence to an equilibrium is an ideal crite-rion for small problems,there are a number of reasons why this is unlikely to be possible for large problems.First,op-timality in large(even stationary)environments is not gen-erally feasible.This is exactly the motivation for exploring function approximation and policy parameterizations.Sec-ond,when we account for the limitations that approximation imposes on a player’s policy then equilibria may cease to ex-ist,making convergence of policies impossible(Bowling& Veloso2002b).Third,policy gradient techniques learn only locally optimal policies.They may converge to policies that are not globally optimal and therefore necessarily not equi-libria.Although convergence to equilibria and therefore conver-gence in general is not a reasonable criterion we would still expect self-play learning agents to learn something.In this paper we use the evaluation technique used by Littman with Minimax-Q(Littman1994).We train an agent in self-play, and then freeze its policy,and train a challenger tofind that policy’s worst-case performance.This challenger is trained using just gradient-descent Sarsa and chooses the action with maximum estimated value with-greedy exploration. Notice that the possible policies playable by the challenger are the deterministic policies(modulo exploration)playable by the learning algorithm being evaluated.Since GoofspielMy Hand1345681113Quartiles***** Deck12359101112-2.2-2.1-2-1.9-1.8-1.7-1.6-1.5-1.4-1.3-1.2-1.1010000200003000040000V a l u e v . W o r s t -C a s e O p p o n e n tNumber of Training GamesWoLF Fast Slow Random -10-9-8-7-6-5-4-3-2010000200003000040000V a l u e v . W o r s t -C a s e O p p o n e n tNumber of Training GamesWoLF Fast Slow Random-26-24-22-20-18-16-14-12010000200003000040000V a l u e v . W o r s t -C a s e O p p o n e n tNumber of Training GamesWoLF Fast Slow RandomFigure 3:Worst-case expected value of the policy learned in self-play.Fast-15-10-5051015010000200003000040000E x p e c t e d V a l u e W h i l e L e a r n i n gNumber of GamesSlow-15-10-5051015010000200003000040000E x p e c t e d V a l u e W h i l e L e a r n i n gNumber of GamesWoLF-15-10-5051015010000200003000040000E x p e c t e d V a l u e W h i l e L e a r n i n gNumber of GamesFigure 4:Expected value of the game while learning.icy gradient approach using an actor-critic model can learn in this domain.In addition,the WoLF principle for encour-aging convergence also seems to hold even when using ap-proximation and generalization techniques.There are a number of directions for future work.Within the game of Goofspiel,it would be interesting to explore alternative ways of tiling the state-action space.This could likely increase the overall performance of the learned policy, but would also examine how generalization might affect the convergence of learning.Might certain generalization tech-niques retain the existence of equilibrium,and is the equilib-rium learnable?Another important direction is to examine these techniques on more domains,with possibly continu-ous state and action spaces.Also,it would be interesting to vary some of the components of the system.Can we use a different approximator than tile-coding?Do we achieve similar results with different policy gradient techniques(e.g. GPOMDP(Baxter&Bartlett2000)).These initial results, though,show promise that gradient ascent and the WoLF principle can scale to large state spaces.ReferencesBaxter,J.,and Bartlett,P.L.2000.Reinforcement learning in POMDP’s via direct gradient ascent.In Proceedings of the Seventeenth International Conference on Machine Learning,41–48.Stanford University:Morgan Kaufman. Bowling,M.,and Veloso,M.2001.Rational and con-vergent learning in stochastic games.In Proceedings of the Seventeenth International Joint Conference on Artifi-cial Intelligence,1021–1026.Bowling,M.,and Veloso,M.2002a.Multiagent learning using a variable learning rate.Artificial Intelligence.In Press.Bowling,M.,and Veloso,M.M.2002b.Existence of multiagent equilibria with limited agents.Technical report CMU-CS-02-104,Computer Science Department, Carnegie Mellon University.Claus,C.,and Boutilier,C.1998.The dynamics of rein-forcement learning in cooperative multiagent systems.In Proceedings of the Fifteenth National Conference on Arti-ficial Intelligence.Menlo Park,CA:AAAI Press.Fink,A.M.1964.Equilibrium in a stochastic-person game.Journal of Science in Hiroshima University,Series A-I28:89–93.Flood,M.1985.Interview by Albert Tucker.The Princeton Mathematics Community in the1930s,Transcript Number 11.Gordon,G.2000.Reinforcement learning with function approximation converges to a region.In Advances in Neu-ral Information Processing Systems12.MIT Press. Greenwald,A.,and Hall,K.2002.Correlated Q-learning. In Proceedings of the AAAI Spring Symposium Workshop on Collaborative Learning Agents.In Press.Hu,J.,and Wellman,M.P.1998.Multiagent reinforce-ment learning:Theoretical framework and an algorithm. In Proceedings of the Fifteenth International Conference on Machine Learning,242–250.San Francisco:Morgan Kaufman.Kuhn,H.W.,ed.1997.Classics in Game Theory.Prince-ton University Press.Littman,M.L.1994.Markov games as a framework for multi-agent reinforcement learning.In Proceedings of the Eleventh International Conference on Machine Learning, 157–163.Morgan Kaufman.Littman,M.2001.Friend-or-foe Q-learning in general-sum games.In Proceedings of the Eighteenth International Conference on Machine Learning,322–328.Williams Col-lege:Morgan Kaufman.Nash,Jr.,J.F.1950.Equilibrium points in-person games. PNAS36:48–49.Reprinted in(Kuhn1997).Osborne,M.J.,and Rubinstein,A.1994.A Course in Game Theory.The MIT Press.Samuel,A.L.1967.Some studies in machine learning using the game of checkers.IBM Journal on Research and Development11:601–617.Shapley,L.S.1953.Stochastic games.PNAS39:1095–1100.Reprinted in(Kuhn1997).Singh,S.;Kearns,M.;and Mansour,Y.2000.Nash con-vergence of gradient dynamics in general-sum games.In Proceedings of the Sixteenth Conference on Uncertainty in Artificial Intelligence,541–548.Morgan Kaufman. Stone,P.,and Sutton,R.2001.Scaling reinforcement learning toward Robocup soccer.In Proceedings of the Eighteenth International Conference on Machine Learn-ing,537–534.Williams College:Morgan Kaufman. Sutton,R.S.,and Barto,A.G.1998.Reinforcement Learn-ing.MIT Press.Sutton,R.S.;McAllester,D.;Singh,S.;and Mansour,Y. 2000.Policy gradient methods for reinforcement learning with function approximation.In Advances in Neural Infor-mation Processing Systems12.MIT Press.Tesauro,G.J.1995.Temporal difference learning and TD–munications of the ACM38:48–68.。