Web Usage Mining for a Better Web-Based Learning Environment
Web使用挖掘技术的研究
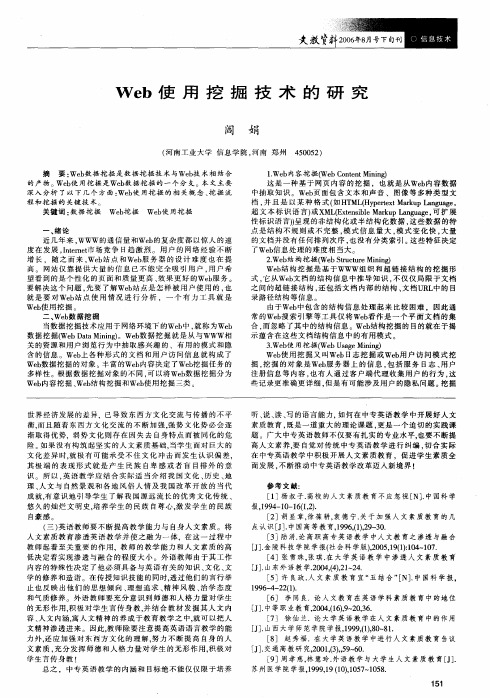
3.Web使用挖掘(Web Usage Mining)
Web使用挖掘又叫Web 日志挖掘或Web用户访问模式挖 掘, 挖掘的对象是Web服务器上的信息, 包括服务 日志、 用户 注册信息等内容, 也有人通过客户端代理收集用户的行为, 这 些记录更准确更详细, 但是有可东西方文化交流与传播的不平 衡, 而且随着东西方文化交流的不断加强, 强势文化势必会逐 渐取得优势, 弱势文化则存在因失去 自 身特点而被同化的危 险。 如果没有构筑起坚实的人文素质基础, 当学生面对巨大的 文化差异时, 就极有可能承受不住文化冲击而发生认识偏差, 其极端的表现形式就是产生民族 自卑感或者盲 目排外 的意 识。所以, 英语教学应结合实际适当介绍我国文化 、 历史 、 地 理、 人文与 自然景观和各地风俗人情及我国改革开放的当代 成就, 有意识地引导学生了解我国源远流长的优秀文化传统、 悠久的灿烂文明史, 培养学生的民族 自尊心, 激发学生的民族
档, 并且是以某种格式(如HTM L(Hypertext M arkup Language, 超文本标识语言)或XM L(Extensible M arkup Language, 可扩展 性标识语言 ))呈现的 非结构化或半结构化数据, 这些数据的 特 点是结构不规则或不完整, 模式信息量大, 模式变化快, 大量
自豪感 。
听、 读、 说、 写的语言能力, 如何在中专英语教学中开展好人文
素质教育 , 既是一道重大的理论课题 , 更是一个迫切的实践课
题。广大中专英语教师不仅要有扎实的专业水平, 也要不断提 高人文素养, 觉对传统中专英语教学进行纠编, 要自 切合实际 在中专英语教学中积极开展人文素质教育, 促进学生素质全
的文档并没有任何排列次序, 也没有分类索引。 这些特征决定 了Web信息处理的难度相当大。
保护树让我们的生活更美好英语作文
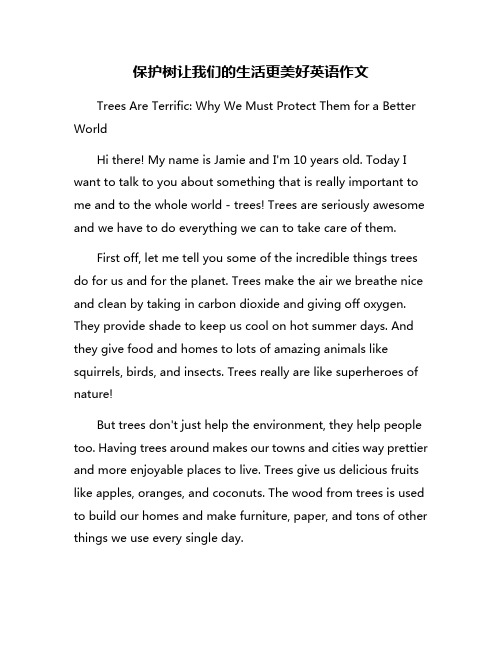
保护树让我们的生活更美好英语作文Trees Are Terrific: Why We Must Protect Them for a Better WorldHi there! My name is Jamie and I'm 10 years old. Today I want to talk to you about something that is really important to me and to the whole world - trees! Trees are seriously awesome and we have to do everything we can to take care of them.First off, let me tell you some of the incredible things trees do for us and for the planet. Trees make the air we breathe nice and clean by taking in carbon dioxide and giving off oxygen. They provide shade to keep us cool on hot summer days. And they give food and homes to lots of amazing animals like squirrels, birds, and insects. Trees really are like superheroes of nature!But trees don't just help the environment, they help people too. Having trees around makes our towns and cities way prettier and more enjoyable places to live. Trees give us delicious fruits like apples, oranges, and coconuts. The wood from trees is used to build our homes and make furniture, paper, and tons of other things we use every single day.With all the wonderful things trees do for our world, you'd think we would go out of our way to protect them. But sadly, the opposite is happening. Forests are being cut down and destroyed faster than ever before. It's estimated that around 15 billion trees are cut down each year! That's a crazy huge number. Just picture 15 billion teddy bears - there would barely be any room to move with that many!The main reasons forests are disappearing are for things like logging to get wood, clearing land for farms and buildings, and making space for mining. Cutting down too many trees causes lots of problems. It damages the homes of forest animals, leading many species toward extinction. It increases greenhouse gas emissions that cause climate change and global warming. And it means we lose out on all the environmental, health, and economic benefits that trees provide.So what can we do to protect trees and keep our planet green and healthy? Well, there are actually lots of things that kids like you and me can do to help!First, we can spread awareness about how important trees are and why we need to save them. We can give presentations at school, write blog posts online, or even make fun videos abouttrees to share with our friends. The more people that understand the problem, the more can be done to solve it.We can also take part in or organise tree planting events in our local parks, schoolyards, or neighborhoods. Getting our hands dirty by actually planting new trees is a great way to make a direct, positive impact. And we'll get to watch those little baby trees grow up big and strong over the years. How cool is that?Another way to help is by reducing our usage of products made from trees, like paper and wood. We can reuse and recycle as much as possible and try to buy items made from recycled or sustainable materials instead. Every little bit of conservation counts.We can pick up litter too, especially around parks and forested areas. Keeping nature clean helps trees and other plants thrive. And why not volunteer for a park or forest clean-up day when one is happening in your community? It's a fun way to get outdoors and do good.Finally, we can speak up and get involved in campaigns that fight against deforestation and irresponsible logging practices. Kids may not be able to vote yet, but we have a powerful voice that shouldn't be ignored. We could write letters to our political leaders, sign petitions, or participate in protests to demandbetter policies that restrict harmful deforestation. After all, the trees can't speak up for themselves, so we need to be their voice!I hope you can see now just how vital trees are to the health of our entire planet. And I hope you'll join me in taking action, no matter how small, to protect and preserve these amazing lifeforms. The future of the world we know and love depends on it. After all, Earth is the only home we've got - so let's take care of it by taking care of trees!Trees are terrific. Let's keep it that way together.。
基于Web挖掘的个性化服务系统的研究与实现
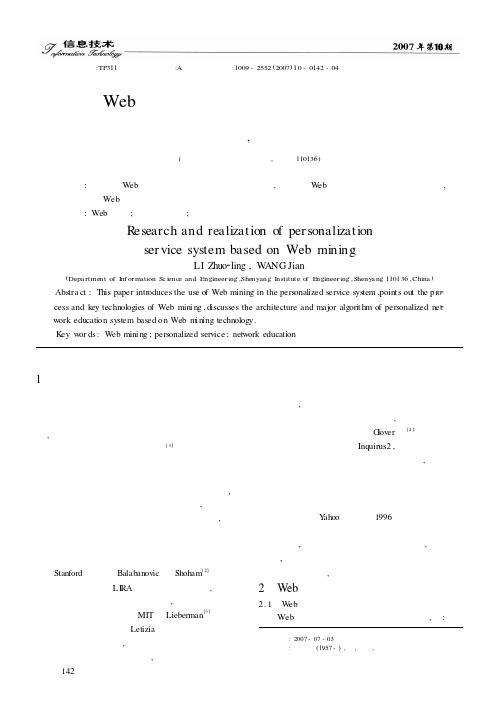
中图分类号:TP311 文献标识码:A 文章编号:1009-2552(2007)10-0142-04基于Web挖掘的个性化服务系统的研究与实现李卓玲,王 健(沈阳工程学院信息工程系,沈阳110136)摘 要:介绍了W eb挖掘在个性化服务系统中的作用,指出了W eb挖掘的基本过程和关键技术,论述了应用Web挖掘技术实现的个性化网络教学系统的体系结构及其主要算法。
关键词:Web挖掘;个性化服务;网络教学R esearch and realization of per sonalizationser vice system based on Web miningLI Zhuo2ling,W ANG Jian(Depa rtment of Inf or mation Sc i ence and Engineer ing,Shenyang Institute o f E ngineer ing,Sheny a ng110136,China) Abstra ct:This paper intr oduces the use of Web mining in the personalized service system,point s out the pr o2 cess and key technologies of Web mini ng,discusses the architecture and major algorit hm of personalized net2 w ork education system based on Web m i ning technology.K ey w or ds:W eb mini ng;personalized service;netw ork education1 个性化服务系统个性化服务是根据用户的使用行为、习惯、偏好和特点来向用户提供满足其个性化需求的一种服务。
浅谈Web数据挖掘技术

浅谈Web数据挖掘技术作者:李晓玮来源:《电脑知识与技术》2013年第22期摘要:随着网络的快速发展与普及,大量有用的网络信息给人们生活、工作和学习带来了便利。
与此同时网络中还存在着许多无用的信息,如何从浩如烟海的数据海洋中,快速准确的查找数据,成为了当今社会不可忽视的问题。
Web数据挖掘技术,正是解决这一问题的关键。
该文从Web数据挖掘技术的角度,阐述Web数据挖掘的概念、分类、过程及常见的Web数据挖掘算法。
关键词: Web数据挖掘;PageRank算法;网络数据中图分类号:TP311.12 文献标识码:A 文章编号:1009-3044(2013)22-4992-021 概述当前,人们随时随地都在利用网络获取信息,不断利用网络进行着上传和下载的操作,这些信息数据在网络上传播和储存着。
因此,网络就形成了一个庞大的数据存储集散地。
如何从海量的网络数据中快速有效地对数据进行分析和检索,并在其中发觉潜在有用的信息,是当今社会需要解决的问题。
Web数据挖掘技术正是很好的解决了这个问题,以下将探讨一下Web 数据挖掘技术。
2 Web数据挖掘概念2.1数据挖掘Web数据挖掘是数据挖掘的一个分支,首先需要了解什么是数据挖掘。
数据挖掘(Data Mining, DM),是指从大量数据中提取有效的、新颖的、潜在有用的、最终可被理解的知识的过程。
在数据库系统中称其为知识发现(Knowledge Discovery in Database, KDD)。
Web 数据挖掘技术融合了数据库系统、统计学、信息科学、人工智能、机器学习等,是一个新兴的多学科交叉应用领域。
2.2 Web数据挖掘Web数据挖掘是在数据挖掘技术的基础上,针对网络数据主要是Web文档和服务日志文件进行的数据分析、归纳和汇总并在其中发现和提取潜在有用的信息及知识的技术。
3 Web数据挖掘的分类根据 Web 数据挖掘的对象,可将 Web 数据挖掘划分为三种类型。
英语读书笔记

英语读书笔记yesterday i read a book the name of the book is《dr bethune》.dr bethune was a famous doctor from canada. in 1938 he came to china. at that time china was at war with japan. he worked as a doctor in the chinese army and saved many soldiers’ lives. he worked very hard and became sick. dr bethune died in 1939. he was only 49 years old. he was a good man and we remember him today.i think the book is very very good!written by wu qingxiangmar. 31XXhow to do research-------reading after a science paperthese days i am busy preparing my dissertation which is about web usage mining . i read some english papers and learnt much from them . and now i want to say something about a paper titled “web usage mining :discovery and applications of usage patterns from web data”.this is the first english paper i read about dissertation and gave me great help .this paper is a review about web usage mining. it introduced web usage mining in detail . although it is a little old for it was published in XX its contents are very useful today . it is organized according to the sequence of web usage mining and the six main parts are introduction which tells me what is web usage mining the sources and abstraction of web data the three steps of web usage mining taxonomy and project survey websift overview privacy issues . the third and fourth parts are most important . it had a list of existing project about web usage mining which i saw many times in other papers but this paper is the one creating this list . besides it has been referred for more then twenty times . as we all know that the higher the referred number is the more important the paper is so i consider this paper to be an important and successful one in this region.in my opinion the success of this paper dues to three reasons . the first reason is the profound computer knowledge owned by the authors . web usage mining relates to many subjects such as artificial intelligence ontology semantic analysis but the most basic knowledge is computer science . the four authors are all professors of department of computer science and engineering in university of minnesota . for myself i am not major in computer science and i am not very good at computers so i feel a little difficult to understand technologies used in this region.second they had read a large number of papers before they wrote this paper . there are fifty nine references listed after main contents . “stand on the shoulders of giants” this sentence tell us a truth : one can never successful all by oneself . and what’s more learn from others can save a lot of time and energy especially for us new learner . how to learn from others is a skill all of us should master but learning doesn’t mean copy or plagiary . other people’s knowledge and production is just our foundation upon this foundation we must have our own thoughts and creation. there are many remarks in this paper where referred other’s production.third the authors had the experience of developing a web usage mining project . they don’t just engage in idle theorizing so their comprehension on this issue is greatly profound. they know what we may meet in a real project development and they know how to resolve them . after reading this paper i also read some other papers written in chinese . some of them are not based on real project and can not give useful resolution . websift is the name of system they developed which can be used to data mining and analysis . 共212i wrote this article not only because it gave me much help in preparing my dissertation but also because it tells me how to study andhow to do research . honesty and preciseness are two essential making aresearcher must have . hard-working is the necessary condition leadingto success . this is just the beginning of my dissertation i should learnfrom these four authors both their knowledge and their attitude to study.共212党员年终工作总结范文回顾一年来的经历,有收获也有不足。
社会性软件支持的WebCL中人际交互的研究的开题报告
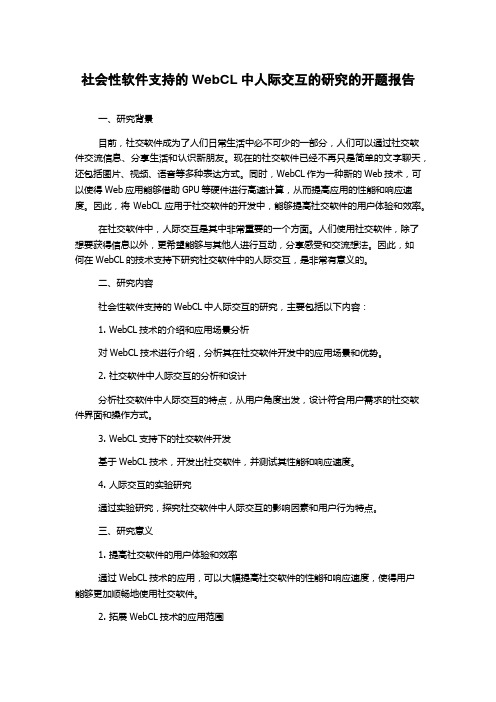
社会性软件支持的WebCL中人际交互的研究的开题报告一、研究背景目前,社交软件成为了人们日常生活中必不可少的一部分,人们可以通过社交软件交流信息、分享生活和认识新朋友。
现在的社交软件已经不再只是简单的文字聊天,还包括图片、视频、语音等多种表达方式。
同时,WebCL作为一种新的Web技术,可以使得Web应用能够借助GPU等硬件进行高速计算,从而提高应用的性能和响应速度。
因此,将WebCL应用于社交软件的开发中,能够提高社交软件的用户体验和效率。
在社交软件中,人际交互是其中非常重要的一个方面。
人们使用社交软件,除了想要获得信息以外,更希望能够与其他人进行互动,分享感受和交流想法。
因此,如何在WebCL的技术支持下研究社交软件中的人际交互,是非常有意义的。
二、研究内容社会性软件支持的WebCL中人际交互的研究,主要包括以下内容:1. WebCL技术的介绍和应用场景分析对WebCL技术进行介绍,分析其在社交软件开发中的应用场景和优势。
2. 社交软件中人际交互的分析和设计分析社交软件中人际交互的特点,从用户角度出发,设计符合用户需求的社交软件界面和操作方式。
3. WebCL支持下的社交软件开发基于WebCL技术,开发出社交软件,并测试其性能和响应速度。
4. 人际交互的实验研究通过实验研究,探究社交软件中人际交互的影响因素和用户行为特点。
三、研究意义1. 提高社交软件的用户体验和效率通过WebCL技术的应用,可以大幅提高社交软件的性能和响应速度,使得用户能够更加顺畅地使用社交软件。
2. 拓展WebCL技术的应用范围将WebCL技术应用于社交软件的开发中,可以拓展其应用范围,并提高其在Web开发中的实用性。
3. 探究人际交互行为的特点和影响因素通过实验研究,可以深入探究社交软件中人际交互行为的特点和影响因素,为社交软件开发和设计提供参考。
四、研究方法本研究主要应用实验研究方法,通过设计实验,分析社交软件中人际交互的影响因素和用户行为特点。
web日志分析常用方法及应用

Web日志挖掘分析的方法日志文件的格式及其包含的信息①2006-10-17 00:00:00②202.200.44.43 ③218.77.130.24 80 ④GET⑤/favicon.ico⑥Mozilla/5.0+(Windows;+U;+Windows+NT+5.1;+zh-CN;+rv:1.8.0.3)+Gecko/20060426+Firefox/1.5.0.3。
①访问时间;②用户IP地址;③访问的URL,端口;④请求方法(“GET”、“POST”等);⑤访问模式;⑥agent,即用户使用的操作系统类型和浏览器软件。
一、日志的简单分析1、注意那些被频繁访问的资源2、注意那些你网站上不存在资源的请求。
常见的扫描式攻击还包括传递恶意参数等:3、观察搜索引擎蜘蛛的来访情况4、观察访客行为应敌之策:1、封杀某个IP2、封杀某个浏览器类型(Agent)3、封杀某个来源(Referer)4、防盗链5、文件重命名作用:1.对访问时间进行统计,可以得到服务器在某些时间段的访问情况。
2.对IP进行统计,可以得到用户的分布情况。
3.对请求URL的统计,可以得到网站页面关注情况。
4.对错误请求的统计,可以更正有问题的页面。
二、Web挖掘根据所挖掘的Web 数据的类型,可以将Web 数据挖掘分为以下三类:Web 内容挖掘(Web Content Mining)、Web 结构挖掘(Web Structure Mining)、Web 使用挖掘(Web Usage Mining)(也称为Web日志挖掘)。
①Web内容挖掘。
Web内容挖掘是指从文档的内容中提取知识。
Web内容挖掘又分为文本挖掘和多媒体挖掘。
目前多媒体数据的挖掘研究还处于探索阶段,Web文本挖掘已经有了比较实用的功能。
Web文本挖掘可以对Web上大量文档集合的内容进行总结、分类、聚类、关联分析,以及利用Web文档进行趋势预测等。
Web文档中的标记,例如<Title>和<Heading>等蕴含了额外的信息,可以利用这些信息来加强Web文本挖掘的作用。
关于爬虫的外文文献
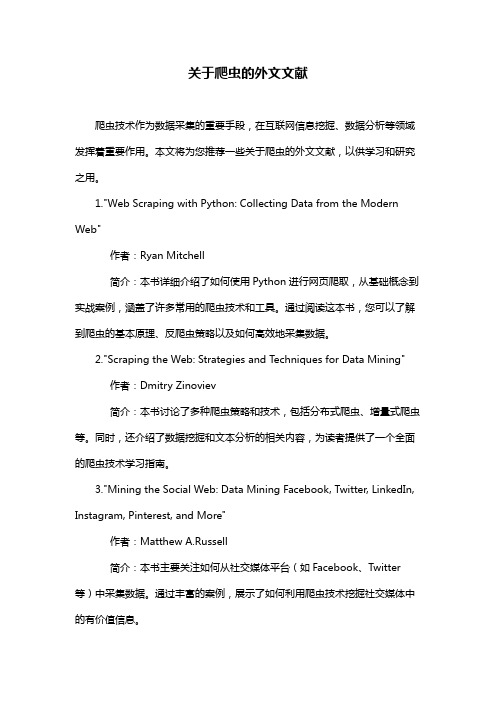
关于爬虫的外文文献爬虫技术作为数据采集的重要手段,在互联网信息挖掘、数据分析等领域发挥着重要作用。
本文将为您推荐一些关于爬虫的外文文献,以供学习和研究之用。
1."Web Scraping with Python: Collecting Data from the Modern Web"作者:Ryan Mitchell简介:本书详细介绍了如何使用Python进行网页爬取,从基础概念到实战案例,涵盖了许多常用的爬虫技术和工具。
通过阅读这本书,您可以了解到爬虫的基本原理、反爬虫策略以及如何高效地采集数据。
2."Scraping the Web: Strategies and Techniques for Data Mining"作者:Dmitry Zinoviev简介:本书讨论了多种爬虫策略和技术,包括分布式爬虫、增量式爬虫等。
同时,还介绍了数据挖掘和文本分析的相关内容,为读者提供了一个全面的爬虫技术学习指南。
3."Mining the Social Web: Data Mining Facebook, Twitter, LinkedIn, Instagram, Pinterest, and More"作者:Matthew A.Russell简介:本书主要关注如何从社交媒体平台(如Facebook、Twitter 等)中采集数据。
通过丰富的案例,展示了如何利用爬虫技术挖掘社交媒体中的有价值信息。
4."Crawling the Web: An Introduction to Web Scraping and Data Mining"作者:Michael H.Goldwasser, David Letscher简介:这本书为初学者提供了一个关于爬虫技术和数据挖掘的入门指南。
内容包括:爬虫的基本概念、HTTP协议、正则表达式、数据存储和数据分析等。
- 1、下载文档前请自行甄别文档内容的完整性,平台不提供额外的编辑、内容补充、找答案等附加服务。
- 2、"仅部分预览"的文档,不可在线预览部分如存在完整性等问题,可反馈申请退款(可完整预览的文档不适用该条件!)。
- 3、如文档侵犯您的权益,请联系客服反馈,我们会尽快为您处理(人工客服工作时间:9:00-18:30)。
Web Usage Mining for a Better Web-Based Learning EnvironmentOsmar R.Za¨ıaneDepartment of Computing ScienceUniversity of AlbertaEdmonton,Alberta,Canadaemail:zaianecs.ualberta.caABSTRACTWeb-based technology is often the technology of choice for distance education given the ease of use of the tools to browse the resources on the Web,the relative afford-ability of accessing the ubiquitous Web,and the simplic-ity of deploying and maintaining resources on the World-Wide Web.Many sophisticated web-based learning envi-ronments have been developed and are in use around the world.The same technology is being used for electronic commerce and has become extremely popular.However, while there are clever tools developed to understand on-line customer’s behaviours in order to increase sales and profit,there is very little done to automatically discover access patterns to understand learners’behaviour on web-based distance cators,using on-line learning environments and tools,have very little support to evalu-ate learners’activities and discriminate between different learners’on-line behaviours.In this paper,we discuss some data mining and machine learning techniques that could be used to enhance web-based learning environments for the educator to better evaluate the leaning process,as well as for the learners to help them in their learning endeavour. KEY WORDSData Mining,e-learning,Web Usage Mining,Learning Ac-tivity Evaluation,Adaptive Web Sites1.Introduction and backgroundWith the rapid development of the World-Wide Web (WWW),the increased popularity and ease of use of its tools,the World-Wide Web is becoming the most important media for collecting,sharing and distributing information. Many organizations and corporations provide information and services on the Web such as automated customer sup-port,on-line shopping,and a myriad of resources and ap-plications.Web-based applications and environments for electronic commerce,distance education,on-line collabo-ration,news broadcasts,etc.,are becoming common prac-tice and widespread.The WWW is becoming ubiquitous and an ordinary tool for everyday activities of common people,from a child sharing musicfiles with friends to a senior receiving photographs and messages from grand-children across the world.It is typical to see web pages for courses in allfields taught at universities and colleges providing course notes and related resources even if these courses are delivered in traditional classrooms.It is not surprising that the Web is the means of choice to archi-tect modern advanced distance education systems.Dis-tance education is afield where web-based technology was very quickly adopted and used for course delivery and knowledge sharing.Typical web-based learning en-vironments such as Virtual-U[5]and Web-CT[13]in-clude course content delivery tools,synchronous and asyn-chronous conferencing systems,polling and quiz modules, virtual workspaces for sharing resources,white boards, grade reporting systems,logbooks,assignment submission components,etc.In a virtual classroom,educators provide resources such as text,multimedia and simulations,and moderate and animate discussions.Remote learners are en-couraged to peruse the resources and participate in activi-ties.However,it is very difficult and time consuming for educators to thoroughly track and assess all the activities performed by all learners on all these tools.Moreover,it is hard to evaluate the structure of the course content and its effectiveness on the learning process.Resource providers do their best to structure the content assuming its efficacy. Educators,using Web-based learning environments,are in desperate need for non-intrusive and automatic ways to get objective feedback from learners in order to better fol-low the learning process and appraise the on-line course structure effectiveness.On the learner’s side,it would be very useful if the system could automatically guide the learner’s activities and intelligently recommend on-line ac-tivities or resources that would favour and improve the learning.These tools do not exist yet and to the best of our knowledge there is no distance leaning system to date that provides such automated facilities either on the learner’s side or educator’s side.In thefield of electronic com-merce,however,given the lucrative prospects,a significant research effort has been made to devise elaborate methods to take advantage of customers’accesses and purchase be-haviours in order to enhance the purchasing experience and customer satisfaction by user profiling and smart recom-mendations,and thus increase profit.For example,sys-tems for recommendation such as that sug-gests books to purchase related to a current purchase based on preference information and similar user purchases,or recommendation of movies with moviefi,use col-laborativefiltering which predicts a person’s preferencesas a linear weighted combination of other people’s pref-erences.Recently,researchers have used web access his-tory to make web sites more adaptive and personalized and hence more attractive to visitors,which is critical to keep customers loyal.WUM[8]is a special web sequence anal-yser for improving web pages layout and structure based on the history of access sequences.Entire conferences and workshops have been dedicated to web usage analysis for the benefit of e-commerce[10,11,12].While the analogy with e-commerce seems straight forward,it is certainly not as simple as it appears.It is true that in e-commerce the goal is to increase sales and profit and it is achieved by understanding customer access behaviour[2],and in e-learning the goal might be to improve the learning and it could be also achieved by understanding learners’access patterns.However,many concepts involved are fundamen-tally different.For instance a purchase transaction,hence a session,which is a fundamental building block for most web usage mining algorithms,is somehow defined starting from a initial access to the web site to a purchasing or order operation,usually in a very short time frame(i.e.the same access session).In e-learning,a learning session can span many access sessions.To learn a concept or attain an exact result in a quiz,many access sessions,spread over many days and even weeks may be needed.Moreover,while the goal in e-commerce sites may be clear,for example en-couraging the customers to buy more products and keeping them loyal,the goals in e-learning are vague,difficult to qualify/quantify and subjective.Web-based course delivery systems,like any web site or web-based application,rely on web servers to provide access to resources and applications.Every single request that a Web server receives is recorded in an access log mainly registering the origin of the request,a time stamp and the resource requested,whether the request is for a web page containing an article from a course chapter,the an-swer to an on-line exam question,or a participation in an on-line conference discussion.The web log provides a raw trace of the learners’navigation and activities on the site.In order to process these log entries and extract valuable pat-terns that could be used to enhance the learning system or help in the learning evaluation,a significant cleaning and transformation phase needs to take place so as to prepare the information for data mining algorithms.The follow-ing section presents the issues related to web log cleaning and transformation.Section3enumerates some important data mining tasks that can be adopted in web usage mining. Section4illustrates with examples how web usage mining can be useful to enhance web-based learning environments. Finally,Section5presents some concluding remarks. 2.Web Log CleansingThere is an assortment of web log analysis tools available [2].Most of them,like NetTracker,webtrends,analog and SurfAid,etc.,provide limited statistical analysis of web log data[16].For example,a typical report has entries of the form:“during this time period t,there were n clicks oc-curring for this particular web page p”.However,the re-sults provided by these tools are limited in their abilities to help understand the implicit usage information and hidden trends.New products use more sophisticated and complex analytic means but are generic,require important manual intervention and often resort to sampling due to the huge size of web logs[2].The most commonly used method to evaluate access to web resources or user interest in re-sources is by counting page accesses or“hits”.However, this is not sufficient and often not correct.Web server log files of current common web servers contain insufficient data upon which to base thorough analysis.However,they contain useful data from which a well-designed data min-ing system can discover beneficial information.Web server logfiles customarily contain:the domain name(or IP ad-dress)of the request;the user name of the user who gener-ated the request(if applicable);the date and time of the re-quest;the method of the request(GET or POST);the name of thefile requested;the result of the request(success,fail-ure,error,etc.);the size of the data sent back;the URL of the referring page;the identification of the client agent;and a cookie,a sting of data generated by an application and exchanged between the client and the server.A log entry is automatically added each time a request for a resource reaches the web server.While this may reflect the actual use of the resources on a site,it does not record reader be-haviours like frequent backtracking or frequent reloading of the same resource when the resource is cached by the browser or a proxy.It is important to note that the entries of all users are mixed in the log,simply ordered chrono-logically even though one single page request from a user may generate multiple entries in the server log.One major problem in web log mining is to identify unique users and associate users with their access log entries.In e-learning applications,however,the problem is simplified since users are not anonymous but need to login to the system as reg-istered learners.However,identifying sessions is a non-trivial task.The goal is to identify sequences of activities from the collection of mixed log entries as described above, and model them as sessions of learning activities to be pre-sented to the educators for evaluation and interpretation,or forwarded to advanced data mining tools to further discover intrinsic useful patterns.The major steps for web log data transformation can be summarized as follows:Remove irrelevant entriesIdentify access sessionsMap access log entries to learning activitiesComplete traversal pathsGroup access sessions by learner to identify learning sessionsIntegrate with other data about learners and groups of learnersRemoving irrelevant entries is the simple task of weeding out requests for images,for example,or non-userrequests such as web crawler requests etc.Identifying ses-sions is a demanding task.The aim is to recognize se-quences of events such as A B C B D...whereA,B,C,D,etc.are page or script requests.The chal-lenge is to recognize the beginning and the end of sessions.The problem comes from the fact that HTTP,the protocol used for information exchange between web servers and browsers,is stateless and does not keep track of seman-tic sessions.In e-commerce applications,the end of ses-sions are usually the purchase of a product or the check-out of an e-cart,and idle times between requests that ex-ceed25to30minutes are use to identify cuts between ses-sions.This heuristic is not necessarily true in the on-linelearning context since learners can wander in other sites gathering relevant information while their access session at the e-learning site is still on hold.Moreover a learningsession can span over days with different accesses.Many pages in e-learning applications are dynamically generated by script requests such as quiz pages,conference messages,etc.Mapping access log entries with actual learning activ-ities consists of replacing script calls with their assignedparameter values with concrete activities.This is an ardu-ous task that assumes thorough knowledge of the applica-tion scripts and their respective parameters and requires amapping table provided by the application designers.The result is a sequence of learners’relevant on-line activities of the form:Login ExerciseList SubmissionQuiz1 ExerciseList plet-ing the traversal paths consists of inferring cache hits andproxy meddling based on the structure of the web site and how pages and activities are effectively linked together.Fi-nally,integrating the cleaned click streams with existingdata about learners can be very valuable and beneficial. Such data could be the profiles of the learners,their quan-titative and qualitative evaluations,etc.For instance com-bining the grades associated with completed activities with the sequences of events leading to these activities can help discover appropriate patterns that can help discriminate be-tween sequence of activities that yield good results and se-quence of events that are not as effective.The web log cleaning and transformation phase often consumes80%to95%of the effort and resources needed for web usage mining[2].The result of the pre-processing is a database of sets of pertinent activity sequences grouped by learner.This is usually modeled with sequences of to-kens associated with user identification stored inflatfiles that current data mining algorithms can act upon.The in-formation can also be stored in a data warehouse like in [16]allowing ad-hoc online analytical processing.The other two phases after data pre-processing are pattern dis-covery using intricate data mining algorithms,and pattern evaluation[9]eful Data Mining TasksWhat is needed are summarization trends and patterns that can be interpreted by educators delivering their courses on-line.Due to the importance of e-commerce and the lucra-tive opportunities behind understanding on-line customer purchasing behaviours,there is tremendous research effort in developing data mining algorithms and systems tailored for e-business related web usage data mining[4].In ad-dition to descriptive statistical analysis provided by most web access log analysis tools such as calculating hit fre-quency,average,median,etc.,length and duration of ses-sions and other limited low-level statistical measures,there have been some data mining approaches adapted specifi-cally for web usage mining.The most used methods are association rules mining,clustering,classification,sequen-tial pattern analysis and dependency modeling[9],as well as prediction.These techniques are primarily used for per-sonalization,system improvement such as web caching and network traffic improvements,site modification,and mar-keting intelligence[9].None of these applications,how-ever,was tailored to distance learning,but the methods are general enough that e-learning systems could benefit from them.Association rules generation is the discovery of rela-tionships between items in transactions.It is typically used for market basket analysis to discover rules of the form“x% of customers who buy item A and B also buy item C.”Clus-tering is an unsupervised grouping of objects,while classi-fication is a supervised grouping.In web mining,the ob-jects could be users,events,sessions,pages,etc.Sequential pattern analysis is similar to association rules but takes into account the sequences of events.In other words,the fact that a page A is requested before another page B is captured in the patterns discovered.All these techniques were de-signed for knowledge discovery from very large databases of numerical data[6]and were adapted for web mining and applied in on-line business with relative success.4.Enhancing Web-Based Learning Environ-mentsWebSIFT[1]is a set of comprehensive web usage tools that is able to perform many data mining tasks and dis-cover a variety of patterns from web logs.A versatile sys-tem,WebLogMiner[16],uses data warehousing technol-ogy for pattern discovery and trend summarization from web logs.However these wide-ranging tools are not inte-grated in e-learning systems and it is cumbersome for an educator who doesn’t have extensive knowledge in data mining to use these tools to improve the effectiveness of web-based learning environments.A new web usage min-ing system dedicated for e-learning is being developed to allow educators to assess on-line learning activities[15]. For an educator using a web-based course delivery environ-ment,it could be beneficial to track the activities happening in the course web site and extract patterns and behaviours prompting needs to change,improve,or adapt the coursecontents.For example,one could identify the paths fre-quently and regularly visited,the paths never visited,the clusters of learners based on the paths they follow,etc.For a learner using a web-based course delivery environment, it could be beneficial to receive hints from the system on what subsequent activity to perform based on similar be-haviour by other”successful”learners.For example,the system could suggest shortcuts to frequently visited pages based on previous user activities,or suggest activities that made similar learners more”successful”.It could also be beneficial if the system adapts the course content logical structure to the learner’s learning pace,interest,or previ-ous behaviour.Web-based course content is not always presented and structured in an intuitive way.By analyzing common traversal paths of the course content web pages or frequent changes in individual traversal paths,the lay-out of the course can be reorganized or adapted to better fit the needs of a group or an individual.We see two types of data mining in the context of e-learning:off-line web usage mining and integrated web usage mining.Off-line web usage mining is the discovery of patterns with a stan-dalone application.This pattern discovery process would allow educators to assess the access behaviours,validate the learning models used,evaluate the learning activities, compare learners and their access patterns,etc.We have designed and implemented a prototype of such an applica-tion as a tool for educators to apply association rules to dis-cover relationships between learning activities that learn-ers perform,sequential analysis to discover interesting pat-terns in the sequences of on-line activities,and clustering to group similar access behaviours[15].While most data mining algorithms need specific parameters and threshold values to tune the discovery process,the users of web usage mining applications in the context of e-learning,namely educators and e-learning site designers,are not necessar-ily savvy in the intricate complexities of data mining al-gorithms.For this purpose we have tried to design new algorithms that need minimum input from the user and au-tomatically adjust to the web log data at hand.In[3]we propose a totally non-parametric approach for clustering web sessions.Off-line web usage mining helps educators put in question and validate the learning models they use as well as the structure of the web site as it is perused by the learners.In contrast,integrated web usage mining is a process of discovering patterns that is incorporated with the e-learning application.This encompasses adaptive web sites,personalization of activities,and automatic recom-menders that suggest activities to learners based on their preferences as well as their history of activities and the ac-cess patterns discovered from the communal accesses.We are currently designing a recommender based association rule mining similar to the text categorization we developed in[14].The idea consists of discovering relevant associa-tions between learning activities and generating association rules that are applied in real time when in a current session the activities of the antecedent of a rule are verified then the activities in the consequent of the rule are suggested to the learner as the recommended next step in the learning session.The algorithm for text categorization presented in [14]can also be used to automatically categorize learners’messages sent on an asynchronous conferencing system in order to help the educators better assess the information ex-change in a course related forum.5.Conclusions and Future WorkThe Web is an excellent tool to deliver on-line courses in the context of distance education.However,counting only on web traffic statistical analysis does not take ad-vantage in the potential of hidden patterns inside the web logs.Web usage mining is a non-trivial process of extract-ing useful implicit and previously unknown patterns from the usage of the Web.Significant research is invested to discover these useful patterns to increase profitability of e-commerce sites.However,the goals of these applica-tions and methods,“turning visitors into purchasers”,are different from the goals in e-learning:“turning learners into effective better learners.”We have seen some exam-ples where data mining techniques can enhance on-line ed-ucation for the educators as well as the learners.While some tools using data mining techniques to help educators and learners are being developed,the re-search is still in its infancy.In addition,with the awareness of the potential advantages of integrated web usage mining and the insufficient data recorded by web servers,there is a need for more specialized logs from the application side to enrich the information already logged by the web server.This added value by specific event recording on the e-learning side will give clicksteams and the patterns discovered a better meaning and interpretation.REFERENCES[1]R.Cooley,B.Mobasher,J.Srivastava,Web Mining: Information and Pattern Discovery on the World Wide Web,Proceedings of the ninth IEEE international confer-ence on Tools with AI,1997.[2]H. A.Edelstein,Pan for Gold in the Clickstream,Informationweek,March2001, /828/mining.htm[3]A.Foss,W.Wang,O.R.Za¨ıane,A Non-Parametric Approach to Web Log Analysis,Proc.Web Mining Workshop,in conjunction with the SIAM International Conference on Data Mining,Chicago,IL,USA,April7, 2001[4]M.N.Garofalakis,R.Rastogi,S.Seshadri,K.Shim, Data Mining and the Web:Past,Present and Future, Proceedings of WIDM99,Kansas City,U.S.A.,1999. [5]C.Groeneboer,D.Stockley,T.Calvert,Virtual-U:A collaborative model for online learning environments,Pro-ceedings Second International Conference on Computer Support for Collaborative Learning,Toronto,Ontario,December,1997.[6]J.Han and M.Kamber,Data Mining Concepts and Techniques,Morgan Kaufmann Publisher,2001[7]J.Han,J.Pei,B.Mortazavi-Asl,Q.Chen,U.Dayal, M.-C.Hsu,“FreeSpan:Frequent Pattern-Projected Sequential Pattern Mining”,Proc.2000Int.Conf. on Knowledge Discovery and Data Mining(KDD’00), Boston,MA,August2000[8]M.Spiliopoulou,L.C.Faulstich,K.Winkler,A Data Miner analyzing the Navigational Behaviour of Web Users,Proceedings of workshop on Machine Learning in User Modeling of the ACAI’99,Creta,Greece,July,1999.[9]J.Srivastava,R.Cooley,M.Deshpande,P.Tan,Web Usage Mining:Discovery and Applications of Usage Patterns form Web Data,SIGKDD Explorations,V ol.1, No.2,Jan.2000.[10]The International Workshop on Web Knowledge Discovery and Data Mining,Kyoto,Japan,April18,2000, .sg/home/awkng/wkddm2000.htm [11]Third International Workshop on Advanced Is-sues of E-Commerce and Web-based Information Systems San Jose,CA,USA,June21-22,2001 /wecwis2001.html[12]Third WEBKDD workshop on data mining for web applications:Mining Log Data Across All Customer TouchPoints,San Francisco,CA,USA, August26,2001,/ron-nyk/WEBKDD2001/index.html[13]WebCT:/[14]O.R.Za¨ıane and Maria-Luiza Antonie,Automatic Text Categorization using Association Rule Mining,sub-mitted to the Journal of Intelligent Information Systems, Special Issue on Automated Text Categorization,2001 [15]O.R.Za¨ıane,J.Luo,Towards Evaluating Learners’Behaviour in a Web-Based Distance Learning Environ-ment,Proc.IEEE International Conference on Advanced Learning Technologies(ICALT2001),Madison,WI, USA,6-8August2001[16]O.R.Za¨ıane,M.Xin,J.Han,Discovering Web Access Patterns and Trends by Applying OLAP and Data Mining Technology on Web Logs,Proceedings from the ADL’98-Advances in Digital Libraries,Santa Barbara, 1998.。