Modeling multi-country mortality dependence and its application in pricing survivor index swap
多因子量化模型简介
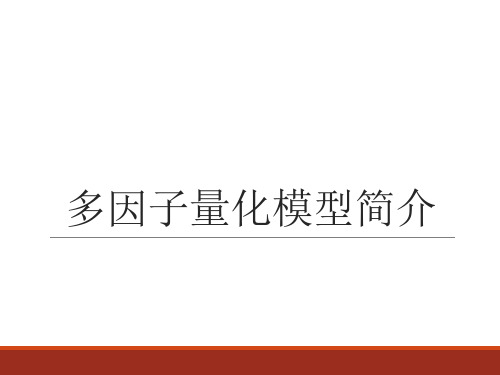
而这一效应不能用CAPM模型解释
o 1981年,大卫·布斯和雷克斯·桑奎菲尔德成立
了维度投资顾问公司(Dimensional Fund
Advisors),买入小市值、估值低的股票,获
得了高额回报
超额收益
组合波动性高于市场
beta<1:
组合波动性低于市场
11
CAPM模型的应用:
被动型基金
o 统计分析发现,股票扣除交易费用后净回报的平均
alpha为接近0的负数,说明股票市场对风险的补偿非
常有效
o 迈克尔·詹森、约翰·博格发现,股票型共同基金的
alpha接近0,四分之三的股票型基金回报不及市场指
=0
= −
∙
2
久期与大类资产
Discount rate change and Duration for selected assets from 2009-10 to year-end 2015
1.0%
MSCI
Emerging
0.5%
Change in Discount Rate
M
有效组合的超额收益来自其风险Leabharlann rF0P9
证券市场线
o 单个证券i的期望收益率与其对市场方差的贡献率 i 之间存在线性关系,
而不像有效组合那样与标准差(总风险)有线性关系
o 这一线性关系在以 E[ri ] 为纵坐标、 i 为横坐标的坐标系中代表一条直线,
这条直线被称为证券市场线(security market line, SML)
多因子量化模型简介
量化 vs 非量化 (有非量化吗?)
修正持久期是衡量价格对收益率变化的敏感度的指标。在市场利率水平发生一
基于集成学习的糖尿病分析预测

应用技术0 引言糖尿病是是一组以高血糖为特征的代表性疾病,目前广泛在中老年群体发病,患病者会导致血糖升高,激素紊乱,破坏人体正常的免疫系统。
因此,通过测量人体的一些参数指标,来预测人体是否患有糖尿病,以便于及时采取治疗手段就显得十分有必要。
在本文中,将根据皮马印第安人糖尿病数据集,通过一些人体的特征,构建相应的分类模型,来预测其是否患有糖尿病。
本研究采用开放的皮马印第安人糖尿病数据集,数据集包含了768个皮马印第安人糖尿病信息样本(样本均为女性),每个样本包含该女性年龄,怀孕次数,血糖指数,血压指数等8个特征,目标值Y表示该女性样本是否患有糖尿病。
我们总共有768名皮马印第安人女性样本,我们将数据划分为500个样本作为测试集,用于训练我们的模型,剩下的268名女性样本作为测试集,用来检验我们训练出的模型的好坏。
1 多分类模型构建■1.1 kNN模型1.1.1 kNN模型原理kNN(k -Nearest Neighbor)算法,又称之为k临近算法,是数据挖掘与机器学习中最简单的分类方法之一。
K 临近指的是待分类样本点最近的k个邻居。
kNN 模型最初由 Cover 和 Hart 于 1968 年被提出, 是一个在理论上比较成熟的方法[1]。
kNN模型的主要思想是,将训练集绘制在特征空间中,然后将待分类样本,通过特定的距离计算公式,得到该样本在该特征空间最近的k个邻居,然后采取投票原则,将k 个邻居中得票最多的类别作为待分类样本的类别。
在我们要解决的问题中,我们的训练集样本包括500名皮马印第安人女性以及相关的8个特征,将它们绘制在特征空间里。
在测试集的268个样本中,我们计算每一个样本与训练集中500个样本的距离远近,挑选出最近距离的k个样本,然后采取投票原则,k个样本中所属类别最多的类别就是测试样本的类别。
从而判断该样本是否患有糖尿病。
1.1.2 结果分析我们采用了sklearn机器学习库中kNN模型算法,对我们的数据集进行了训练。
计量经济学(重要名词解释)

——名词解释将因变量与一组解释变量和未观测到的扰动联系起来的方程,方程中未知的总体参数决定了各解释变量在其他条件不变下的效应。
与经济分析不同,在进行计量经济分析之前,要明确变量之间的函数形式。
经验分析(Empirical Analysis):在规范的计量分析中,用数据检验理论、估计关系式或评价政策有效性的研究。
确定遗漏变量、测量误差、联立性或其他某种模型误设所导致的可能偏误的过程线性概率模型(LPM)(Linear Probability Model, LPM):响应概率对参数为线性的二值响应模型。
没有一个模型可以通过对参数施加限制条件而被表示成另一个模型的特例的两个(或更多)模型。
有限分布滞后(FDL)模型(Finite Distributed Lag (FDL) Model):允许一个或多个解释变量对因变量有滞后效应的动态模型。
布罗施-戈弗雷检验(Breusch-Godfrey Test):渐近正确的AR(p)序列相关检验,以AR(1)最为流行;该检验考虑到滞后因变量和其他不是严格外生的回归元。
布罗施-帕甘检验(Breusch-Pagan Test)/(BP Test):将OLS 残差的平方对模型中的解释变量做回归的异方差性检验。
若一个模型正确,则另一个非嵌套模型得到的拟合值在该模型是不显著的。
因此,这是相对于非嵌套对立假设而对一个模型的检验。
在模型中包含对立模型的拟合值,并使用对拟合值的t 检验来实现。
回归误差设定检验(RESET)(Regression Specification Error Test, RESET):在多元回归模型中,检验函数形式的一般性方法。
它是对原OLS 估计拟合值的平方、三次方以及可能更高次幂的联合显著性的F 检验。
怀特检验(White Test):异方差的一种检验方法,涉及到做OLS 残差的平方对OLS 拟合值和拟合值的平方的回归。
这种检验方法的最一般的形式是,将OLS 残差的平方对解释变量、解释变量的平方和解释变量之间所有非多余的交互项进行回归。
美国经济金融化测度研究

美国经济金融化测度研究作者:裴祥宇来源:《商业研究》2017年第01期内容提要:本文从部门视角构建测度经济金融化的指标体系,采用基于模糊层次分析法的主观静态赋权法和基于熵值法的客观动态赋权法对美国经济金融化进行综合评价。
结果显示,样本期内的美国经济金融化趋势不断增强,金融部门扩张强劲,非金融企业部门金融化呈现周期性上升态势;联邦基金利率调节在一定范围内对非金融企业部门金融化产生波动性影响,但无法左右其金融化程度不断加深的进程。
关键词:经济金融化;模糊层次分析法;熵值法;综合评价中图分类号:F11文献标识码:A文章编号:1001-148X(2017)01-0091-09一、引言“金融化”一般用以描述20世纪70、80年代后,发达资本主义国家的经济活动和资本积累由实体经济向金融部门转移的过程。
关于金融化现象本身的测度和演进过程的研究,Krippner (2005)、Epstein(2005)指出,应当选取合适指标测度经济金融化的起点和趋势,对经济金融化进行较为严格的经验验证。
但不同学者赋予经济金融化不同角度的含义,用于测度经济金融化的指标也各有侧重。
从宏观经济视角来看,相关文献大多采用金融相关比率FIR、金融资产占GDP比重、信贷规模、金融从业人员占比等指标证明研究样本存在金融化趋势或是金融化趋势加强①。
从部门角度的研究主要考察金融部门扩张以及非金融部门利润来源渠道的变化(Krippner,2005;Foster,2010)。
总之,国外学者对经济金融化的描述缺乏一个度量金融化程度较为全面的指标体系。
国内学者在建立经济金融化指标体系方面做过初步尝试。
蔡则祥等(2004)根据经济金融化发展的阶段性特征,分别从经济货币化、经济信用化、经济证券化和经济虚拟化四个方面建立衡量经济金融化指标体系。
赵峰(2010)从宏观和部门结构、金融和非金融部门内部结构、收入分配和居民消费结构等三个方面选取10个指标,考察美国经济向金融化转型的过程。
多模态模型中英文训练

多模态模型中英文训练In the realm of artificial intelligence, multimodalmodels have emerged as a critical tool for understanding and processing data from various sources. These models aretrained to interpret and integrate information from text, images, audio, and more, enhancing their ability to comprehend complex data sets.Training such models in English involves a nuanced understanding of the language's intricacies, including idiomatic expressions and contextual meanings. The process requires vast datasets and a deep learning architecture that can adeptly handle the linguistic subtleties of English.Conversely, training in Chinese presents its own set of challenges, such as the tonal nature of the language and the complexity of its characters. The model must learn to distinguish between homophones and understand the context in which characters are used to convey different meanings.The integration of both English and Chinese training is essential for models that aim to operate in multilingual environments. It demands a sophisticated approach to learning, where the model can switch between languages seamlessly, recognizing the unique features of each while maintaining a unified understanding.To achieve this, the training process must bemeticulously designed, with a focus on both the syntactic and semantic aspects of each language. This includes the use of parallel corpora, where equivalent sentences in both languages are used to align the models' understanding.Moreover, the model's performance is significantly influenced by the quality and diversity of the training data. It is crucial to include a wide range of examples that cover different domains and styles to ensure the model's robustness and adaptability.Finally, the success of multimodal models in a bilingual training context hinges on continuous evaluation and refinement. Regular assessments against real-world scenarios help identify areas for improvement and guide the model towards becoming more effective in handling multilingual and multimodal data.。
多因素模型英语
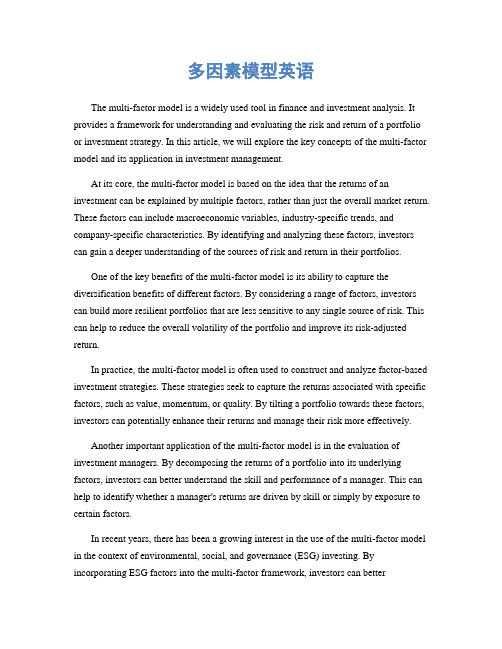
多因素模型英语The multi-factor model is a widely used tool in finance and investment analysis. It provides a framework for understanding and evaluating the risk and return of a portfolio or investment strategy. In this article, we will explore the key concepts of the multi-factor model and its application in investment management.At its core, the multi-factor model is based on the idea that the returns of an investment can be explained by multiple factors, rather than just the overall market return. These factors can include macroeconomic variables, industry-specific trends, and company-specific characteristics. By identifying and analyzing these factors, investors can gain a deeper understanding of the sources of risk and return in their portfolios.One of the key benefits of the multi-factor model is its ability to capture the diversification benefits of different factors. By considering a range of factors, investors can build more resilient portfolios that are less sensitive to any single source of risk. This can help to reduce the overall volatility of the portfolio and improve its risk-adjusted return.In practice, the multi-factor model is often used to construct and analyze factor-based investment strategies. These strategies seek to capture the returns associated with specific factors, such as value, momentum, or quality. By tilting a portfolio towards these factors, investors can potentially enhance their returns and manage their risk more effectively.Another important application of the multi-factor model is in the evaluation of investment managers. By decomposing the returns of a portfolio into its underlying factors, investors can better understand the skill and performance of a manager. This can help to identify whether a manager's returns are driven by skill or simply by exposure to certain factors.In recent years, there has been a growing interest in the use of the multi-factor model in the context of environmental, social, and governance (ESG) investing. By incorporating ESG factors into the multi-factor framework, investors can betterunderstand the impact of sustainability considerations on the risk and return of their portfolios. This can help to align investment decisions with broader social and environmental goals.It is important to note that the multi-factor model is not without its limitations. One challenge is the identification and selection of relevant factors, as well as the estimation of their risk and return premia. Additionally, the model assumes that the relationship between factors and returns is stable over time, which may not always be the case in practice.In conclusion, the multi-factor model is a powerful tool for understanding and managing the risk and return of investment portfolios. By considering a range of factors, investors can build more resilient portfolios, enhance their returns, and better evaluate the performance of investment managers. As the use of the multi-factor model continues to evolve, it is likely to play an increasingly important role in the field of investment management.。
文献引用联合国全球多维贫困指数报告格式
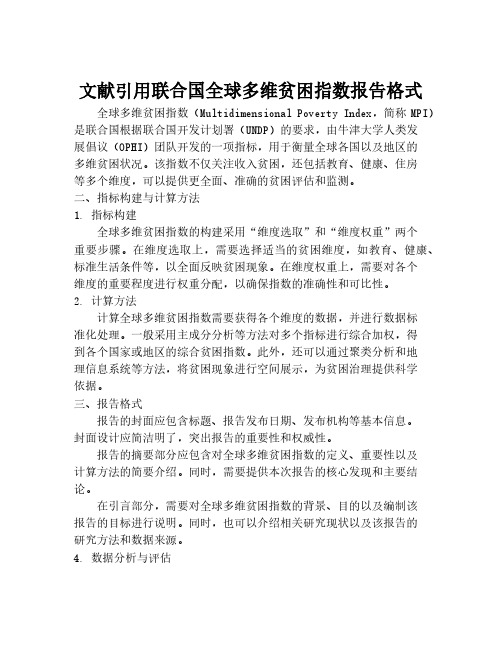
文献引用联合国全球多维贫困指数报告格式全球多维贫困指数(Multidimensional Poverty Index,简称MPI)是联合国根据联合国开发计划署(UNDP)的要求,由牛津大学人类发展倡议(OPHI)团队开发的一项指标,用于衡量全球各国以及地区的多维贫困状况。
该指数不仅关注收入贫困,还包括教育、健康、住房等多个维度,可以提供更全面、准确的贫困评估和监测。
二、指标构建与计算方法1. 指标构建全球多维贫困指数的构建采用“维度选取”和“维度权重”两个重要步骤。
在维度选取上,需要选择适当的贫困维度,如教育、健康、标准生活条件等,以全面反映贫困现象。
在维度权重上,需要对各个维度的重要程度进行权重分配,以确保指数的准确性和可比性。
2. 计算方法计算全球多维贫困指数需要获得各个维度的数据,并进行数据标准化处理。
一般采用主成分分析等方法对多个指标进行综合加权,得到各个国家或地区的综合贫困指数。
此外,还可以通过聚类分析和地理信息系统等方法,将贫困现象进行空间展示,为贫困治理提供科学依据。
三、报告格式报告的封面应包含标题、报告发布日期、发布机构等基本信息。
封面设计应简洁明了,突出报告的重要性和权威性。
报告的摘要部分应包含对全球多维贫困指数的定义、重要性以及计算方法的简要介绍。
同时,需要提供本次报告的核心发现和主要结论。
在引言部分,需要对全球多维贫困指数的背景、目的以及编制该报告的目标进行说明。
同时,也可以介绍相关研究现状以及该报告的研究方法和数据来源。
4. 数据分析与评估该部分应详细分析各个国家和地区的全球多维贫困指数,提供相关数据表格和图表进行支撑和解释。
需要重点关注贫困维度的构成、各维度权重分配以及不同国家或地区之间的差异及变化趋势。
5. 结果与讨论在结果部分,应对数据分析进行总结和概括,回答研究提出的问题和目标。
同时,也可以对研究结果进行讨论,探讨影响贫困指数的因素以及可能的政策建议。
在结论部分,需要对报告的主要发现进行总结,并强调报告的重要性和对全球贫困治理的意义。
基于多层次模糊系统的贫困等级认定模型英文
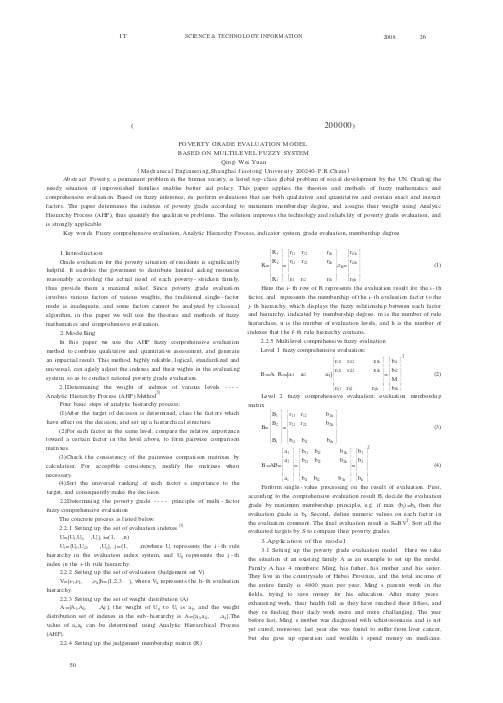
科技信息
○I T 技术论坛○
SCIE NCE & TE CHNO LO GY INFORM ATION
2008 年 第 26 期
基于多层次模糊系统的贫困等机 械与 动力 工程 学院 中 国 上海 200000)
PO VE RTY GRADE EVALUATION M ODEL BASED ON MULTILE VE L FUZZY SYSTEM
Qin g- Wei Yuan ( Mech anical Engi neeri ng,Sh anghai J iaot ong Un iversit y 200240 , P.R.Ch ina ) 【Ab str act 】Povert y, a permanent problem in the human societ y, is list ed top- class global probl em of soci al development by the UN. Gradi ng the needy situation of i mpoveri shed fami lies enables better aid policy. This paper applies the theori es and methods of fuzzy mathematics and comprehensive eval uati on. Based on fuzzy inference, its perform evaluations that are bot h qual itative and quanti tat ive and contai n exact and inexact factors. The paper determines the i ndexes of poverty grade according to maximum membership degree, and assigns thei r weight using Anal yti c Hierarchy Process (AHP), thus quantify the qualit ati ve probl ems. The solution improves t he technology and reliabi lity of povert y grade evaluation, and is strongly applicable 【Key wor ds 】Fuzzy comprehensive eval uation, Analytic Hierarchy Process, indi cator system, grade evaluation, membership degree
- 1、下载文档前请自行甄别文档内容的完整性,平台不提供额外的编辑、内容补充、找答案等附加服务。
- 2、"仅部分预览"的文档,不可在线预览部分如存在完整性等问题,可反馈申请退款(可完整预览的文档不适用该条件!)。
- 3、如文档侵犯您的权益,请联系客服反馈,我们会尽快为您处理(人工客服工作时间:9:00-18:30)。
Corresponding author. E-mail addresses: chouwenwang@ (C.-W. Wang), syang@.tw (S.S. Yang), jerry2@.tw (H.-C. Huang). /10.1016/j.insmatheco.2015.03.019 0167-6687/© 2015 Published by Elsevier B.V.
∗
mortality-linked securities (Blake et al., 2006; Dowd et al., 2006; Biffis and Blake, 2009; Blake et al., 2010; Biffis et al., 2011; Huang et al., 2011; Wang et al., 2011; Wang and Yang, 2013). As a potential means to increase hedge effectiveness, basis risk has been a primary concern in recent market developments. Basis risk results when the mortality experience of the index in longevity securities differs from the longevity risk exposure of the pension plan or annuity portfolio, when hedging longevity risk with the securities based on longevity indices. Dowd et al. (2006) point out that a hedge is only as good as the reference index based on the insurer’s own mortality experience. Coughlan et al. (2011) also caution that basis risk can result in an imperfect longevity hedge, leaving some residual amount of risk. It is thus essential to evaluate the extent of this risk and weight the degree of risk reduction against the cost of the hedge. As Blake et al. (2012) explain, one of the reasons the EIB longevity bond never launched was because the basis risk in the bond appeared too great. It might have provided a reasonable hedge for male pension plan members in their 60s, but pension plans also include male members in their 70s and 80s, as well as women. The Swiss Re Mortality Bond, issued in 2003, reflects a combined mortality index instead, including France, England, the United States, Italy, and Switzerland. The
Modeling multi-country mortality dependence and its application in pricing survivor index swaps—A dynamic copula approach
Chou-Wen Wang a,d , Sharon S. Yang b,d , Hong-Chiห้องสมุดไป่ตู้ Huang c,d,∗
Article history: Available online 23 April 2015 Keywords: Dynamic copula Non-Gaussian distributions Mortality dependence Survivor swaps Stochastic mortality model
a b c d
Department of Risk Management & Insurance, National Kaohsiung First University of Science and Technology, Taiwan Department of Finance, National Central University, Taiwan Department of Risk Management and Insurance, National Chengchi University, Taipei, Taiwan Risk and Insurance Research Center, College of Commerce, National Chengchi University, Taipei, Taiwan
article
info
abstract
This paper introduces mortality dependence in multi-country mortality modeling using a dynamic copula approach. Specifically, we use time-varying copula models to capture the mortality dependence structure across countries, examining both symmetric and asymmetric dependence structures. In addition, to capture the phenomenon of a heavy tail for the multi-country mortality index, we consider not only the setting of Gaussian innovations but also non-Gaussian innovations under the Lee–Carter framework model. As tests of the goodness of fit of different dynamic copula models, the pattern of mortality dependence, and the distribution of the innovations, we used empirical mortality data from Finland, France, the Netherlands, and Sweden. To understand the effect of mortality dependence on longevity derivatives, we also built a valuation framework for pricing a survivor index swap, then investigated the fair swap rates of a survivor swap numerically. We demonstrate that failing to consider the dynamic copula mortality model and non-Gaussian innovations would lead to serious underestimations of the swap rates and loss reserves. © 2015 Published by Elsevier B.V.
C.-W. Wang et al. / Insurance: Mathematics and Economics 63 (2015) 30–39
31
mortality bond issued by Nathan Ltd. in 2008 similarly depends on a combined mortality index, for four countries: the United States, the United Kingdom, Canada, and Germany. Therefore, finding ways to deal with the basis risk through a longevity hedge is a critical question; it is unacceptable to ignore the basis risk when designing mortality-linked securities. The mortality index underlying a mortality-linked security is particularly important for dealing with basis risk and increasing hedge effectiveness. We introduce a survivor index swap that can transfer the longevity risk pool across different insurers in different countries. Survivor swaps have been explored widely in prior literature (Dawson, 2002; B