Zero-curvature condition in two dimensions. Relativistic particle models and finite W-trans
Collagen-structure, function, and biosynthesis

Collagens—structure,function,and biosynthesisK.Gelse a ,E.Po ¨schl b ,T.Aigner a,*aCartilage Research,Department of Pathology,University of Erlangen-Nu ¨rnberg,Krankenhausstr.8-10,D-91054Erlangen,GermanybDepartment of Experimental Medicine I,University of Erlangen-Nu ¨rnberg,91054Erlangen,GermanyReceived 20January 2003;accepted 26August 2003AbstractTherepresents a complex alloy of variable members of diverse protein families defining structuralintegrity and various physiological functions.The most abundant family is the collagens with more than 20different collagen types identified so far.Collagens are centrally involved in the formation of fibrillar and microfibrillar networks of the extracellular matrix,basement membranes as well as other structures of the extracellular matrix.This review focuses on the distribution and function of various collagen types in different tissues.It introduces their basic structural subunits and points out major steps in the biosynthesis and supramolecular processing of fibrillar collagens as prototypical members of this protein family.A final outlook indicates the importance of different collagen types not only for the understanding of collagen-related diseases,but also as a basis for the therapeutical use of members of this protein family discussed in other chapters of this issue.D 2003Elsevier B.V .All rights reserved.Keywords:Collagen;Extracellular matrix;Fibrillogenesis;Connective tissueContents1.Collagens—general introduction .............................................15322.Collagens—the basic structural module..........................................15323.Distribution,structure,and function of different collagen types...............................15353.1.Collagen types I,II,III,V and XI—the fibril-forming collagens ...........................15353.2.Collagen types IX,XII,and XIV—The FACIT collagens...............................15373.3.Collagen type VI—a microfibrillar collagen.....................................15383.4.Collagen types X and VIII—short chain collagens..................................15383.5.Collagen type IV—the collagen of basement membranes...............................15384.Biosynthesis of collagens ................................................15404.1.Transcription and translation ............................................15404.2.Posttranslational modifications of collagen .....................................15404.3.Secretion of collagens...............................................15414.4.Extracellular processing and modification ......................................15410169-409X/$-see front matter D 2003Elsevier B.V .All rights reserved.doi:10.1016/j.addr.2003.08.002*Corresponding author.Tel.:+49-9131-8522857;fax:+49-9131-8524745.E-mail address:thomas.aigner@patho.imed.uni-erlangen.de (T.Aigner)/locate/addrAdvanced Drug Delivery Reviews 55(2003)1531–15465.Functions of collagens beyond biomechanics ......................................15426.Perspectives .....................................................1542Acknowledgements....................................................1543References........................................................1543gens were once considered to be a group of proteinswith a characteristic molecular structure with theirfibrillar structures contributing to the extracellularscaffolding.Thus,collagens are the major structuralelement of all connective tissues and are also foundin the interstitial tissue of virtually all parenchymal 2.Collagens—the basic structural module The name ‘‘collagen’’is used as a generic term for proteins forming a characteristic triple helix of three polypeptide chains and all members of the collagen family form these the K.Gelse et al./Advanced Drug Delivery Reviews 55(2003)1531–15461532Table 1Table showing the various collagen types as they belong to the major collagen families TypeMolecular compositionGenes (genomic localization)Tissue distribution Fibril-forming collagens I [a 1(I)]2a 2(I)COL1A1(17q21.31–q22)bone,COL1A2(7q22.1)II[a 1(II)]3COL2A1(12q13.11–q13.2)III [a 1(III)]3COL3A1(2q31)Va 1(V),a 2(V),a 3(V)COL5A1(9q34.2–q34.3)COL5A2(2q31)COL5A3(19p13.2)XI a 1(XI)a 2(XI)a 3(XI)COL11A1(1p21)COL11A2(6p21.3)COL11A3=COL2A1Basement membrane collagens IV [a 1(IV)]2a 2(IV);a 1–a 6COL4A1(13q34)basement membranesCOL4A2(13q34)COL4A3(2q36–q37)COL4A4(2q36–q37)COL4A5(Xq22.3)COL4A6(Xp22.3)Microfibrillar collagen VI a 1(VI),a 2(VI),a 3(VI)COL6A1(21q22.3)widespread:dermis,cartilage,placenta,lungs,vessel wall,COL6A2(21q22.3)intervertebral discCOL6A3(2q37)Anchoring fibrils VII [a 1(VII)]3COL7A1(3p21.3)skin,dermal–epidermal junctions;oral mucosa,cervix,Hexagonal network-forming collagens VIII [a 1(VIII)]2a 2(VIII)COL8A1(3q12–q13.1)endothelial cells,Descemet’s membrane COL8A2(1p34.3–p32.3)X [a 3(X)]3COL10A1(6q21–q22.3)hypertrophic cartilageF ACIT collagens IX a 1(IX)a 2(IX)a 3(IX)COL9A1(6q13)cartilage,vitreous humor,corneaCOL9A2(1p33–p32.2)XII [a 1(XII)]3COL12A1(6q12–q13)perichondrium,ligaments,tendonXIV [a 1(XIV)]3COL9A1(8q23)dermis,tendon,vessel wall,placenta,lungs,liver XIX [a 1(XIX)]3COL19A1(6q12–q14)human rhabdomyosarcomaXX [a 1(XX)]3corneal epithelium,embryonic skin,sternal cartilage,tendon XXI[a 1(XXI)]3COL21A1(6p12.3–11.2)blood vessel wallTransmembrane collagens XIII [a 1(XIII)]3COL13A1(10q22)epidermis,hair follicle,endomysium,intestine,chondrocytes,lungs,liver XVII [a 1(XVII)]3COL17A1(10q24.3)dermal–epidermal junctionsMultiplexins XV [a 1(XV)]3COL15A1(9q21–q22)fibroblasts,smooth muscle cells,kidney,pancreas,XVI [a 1(XVI)]3COL16A1(1p34)fibroblasts,amnion,keratinocytes XVIII [a 1(XVIII)]3COL18A1(21q22.3)lungs,liverGiven are the molecular composition,the genomic localization of the different chains as well as the basic tissue distribution.K.Gelse et al./Advanced Drug Delivery Reviews 55(2003)1531–15461533extracellular matrix although their size,function and tissue distribution vary considerably.So far,26ge-netically distinct collagen types have been described [4,7–11].Based on their structure and supramolecular orga-nization,they can be grouped into fibril-forming collagens,fibril-associated collagens (FACIT),net-work-forming collagens,anchoring fibrils,transmem-brane collagens,basement membrane collagens and others with unique functions (see Table 1).The different collagen types are characterized by considerable complexity and diversity in their struc-ture,their splice variants,the presence of additional,non-helical domains,their assembly and their func-tion.The most abundant and widespread family of collagens with about 90%of the total collagen is represented by the fibril-forming collagens.Types Itissues [4,12,13].Type IV collagens with a more flexible triple helix assemble into meshworks restrict-ed to basement membranes.The microfibrillar type VI collagen is highly disulfide cross-linked and contrib-utes to a network of beaded filaments interwoven with other collagen fibrils [14].F ibril-a ssociated c ollagens with i nterrupted t riplehelices (FACIT)such as types IX,XII,and XIV collagens associate as single mol-ecules with large collagen fibrils and presumably play a role in regulating the diameter of collagen fibrils [9].Types VIII and X collagens form hexagonal networks while others (XIII and XVII)even span cell membranes [15].Despite the rather high structural diversity among the different collagen types,all members of the collagen family have one characteristic feature:a right-handed triple helix composed of three a -chains (Fig.1)[7,16].These might be formed by three identical chains (homotrimers)as in collagens II,III,VII,VIII,X,and others or by two or more different chains (heterotrimers)as in collagen types I,IV ,V ,VI,IX,and XI.Each of the three a -chains within the molecule forms an extended left-handed helix with a pitch of 18amino acids per turn [17].The three chains,staggered by one residue relative to each other,are supercoiled around a central axis in a right-handed manner to form the triple helix [18].A structural prerequisite for the assembly into a triple helix is a glycine residue,the smallest amino acid,in every third position of the polypeptide chains resulting in a (Gly-X-Y)n repeat structure which characterizes the ‘‘col-lagenous’’domains of all collagens.The a -chains assemble around a central axis in a way that all glycine residues are positioned in the center of the triple helix,while the more bulky side chains of the other amino acids occupy the outer positions.This allows a close packaging along the central axis of the molecule.The X and Y position is often occupied by proline and hydroxyproline.Depending on the colla-gen type,specific proline and lysine residues areFig.1.Molecular structure of fibrillar collagens with the various subdomains as well as the cleavage sites for N-and C-procollagenases (shown is the type I collagen molecule).Whereas they are arranged in tendon in a parallel manner they show a rather network-like supramolecular arrangement in articular cartilage.K.Gelse et al./Advanced Drug Delivery Reviews 55(2003)1531–15461534modified by post-translational enzymatic hydroxyl-ation.The content of4-hydroxyproline is essential for the formation of intramolecular hydrogen bonds and contributes to the stability of the triple helical conformation.Some of the hydroxylysines are further modified by glycosylation.The length of the triple helical part varies considerably between different collagen types.The helix-forming(Gly-X-Y)repeat is the predominating motif in fibril-forming collagens (I,II,III)resulting in triple helical domains of300nm in length which corresponds to about1000amino acids[3,4].In other collagen types,these collagenous domains are much shorter or contain non-triple helical interruptions.Thus,collagen VI or X contains triple helices with about200or460amino acids,respec-tively[4].Although the triple helix is a key feature of all collagens and represents the major part in fibril-forming collagens,non-collagenous domains flanking the central helical part are also important structural components(Fig.1).Thus,the C-propeptide is thought to play a fundamental role in the initiation of triple helix formation,whereas the N-propeptide is thought to be involved in the regulation of primary fibril diameters[3].The short non-helical telopeptides of the processed collagen monomers(see Fig.1)are involved in the covalent cross-linking of the collagen molecules as well as linking to other molecular structures of the surrounding matrix[38].FACIT collagens are characterized by several non-collagenous domains interrupting the triple he-lices,which may function as hinge regions[19].In other collagens like collagens IV,VI,VII,VIII or X,non-collagenous domains are involved in net-work formation and aggregation.In contrast to the highly conserved structure of the triple helix,non-collagenous domains are characterized by a more structural and functional diversity among different collagen families and types.Interruptions of the triple helical structure may cause intramolecular flexibility and allow specific proteolytic cleavage. Native triple helices are characterized by their resistance to proteases such as pepsin,trypsin or chymotrypsin[20]and can only be degraded by different types of specific collagenases.Collagenase A(MMP-1)[21],the interstitial collagenase,is expressed by a large variety of cells and is thought to be centrally involved in tissue remodeling, e.g. during wound healing.MMP-8(collagenase B)is largely specific for neutrophil granulocytes[22]and, thus,thought to be mainly involved in tissue destruction during acute inflammatory processes. MMP-13(collagenase C)[23]is expressed by hypertrophic chondrocytes as well as osteoblasts and osteoclasts[24]and therefore most likely plays an important role in cartilage and bone remodeling. Many other matrix metalloproteinases are able to cleave the denatured collagen(‘‘gelatin’’).The de-tailed analysis of the interplay of MMPs as well as specific inhibitors will describe the reactivities in vivo as well as potential pharmaceutical options for intervention[25–27].3.Distribution,structure,and function of different collagen types3.1.Collagen types I,II,III,V and XI—the fibril-forming collagensThe classical fibril-forming collagens include col-lagen types I,II,III,V,and XI.These collagens are characterized by their ability to assemble into highly orientated supramolecular aggregates with a charac-teristic suprastructure,the typical quarter-staggered fibril-array with diameters between25and400nm (Fig.2).In the electron microscope,the fibrils are defined by a characteristic banding pattern with a periodicity of about70nm(called the D-period)based on a staggered arrangement of individual collagen monomers[28].Type I collagen is the most abundant and best studied collagen.It forms more than90%of the organic mass of bone and is the major collagen of tendons,skin,ligaments,cornea,and many intersti-tial connective tissues with the exception of very few tissues such as hyaline cartilage,brain,and vitreous body.The collagen type I triple helix is usually formed as a heterotrimer by two identical a1(I)-chains and one a2(I)-chain.The triple helical fibres are,in vivo,mostly incorporated into composite containing either type III collagen(in skin and reticular fibres)[29]or type V collagen(in bone, tendon,cornea)[30].In most organs and notably in tendons and fascia,type I collagen provides tensile stiffness and in bone,it defines considerable biome-chanical properties concerning load bearing,tensileK.Gelse et al./Advanced Drug Delivery Reviews55(2003)1531–15461535strength,and torsional stiffness in particular after calcification.The fibril-forming type II collagen is the charac-teristic and predominant component of hyaline carti-lage.It is,however,not specifically restricted to cartilage where it accounts for about80%of the total collagen content since it is also found in the vitreous body,the corneal epithelium,the notochord, the nucleus pulposus of intervertebral discs,and embryonic epithelial–mesenchymal transitions[4]. The triple helix of type II collagen is composed of three a1(II)-chains forming a homotrimeric molecule similar in size and biomechanical properties to that of type I collagen[31].Collagen fibrils in cartilage represent heterofibrils containing in addition to the dominant collagen II,also types XI and IX collagens which are supposed to limit the fibril diameter to about15–50nm[32]as well as other non-collage-nous pared to type I collagen,type II collagen chains show a higher content of hydroxy-lysine as well as glucosyl and galactosyl residuesmatrix of hyaline cartilageof the type II collagen pre-mRNA results in twoforms of the a1(II)-chains.In the splice variant IIB, Fig.2.(A)Schematic representation of the supramolecular assembly of the collagen fibrils in the characteristic quarter-staggered form.The monomers are300-nm long and40-nm gaps separate consecutive monomers causing the characteristic appearance of the collagen type I fibrils on the ultrastructural level.(B+C)Collagen type I(B)and II(C)fibrils as they are arranged in normal tendon(B)and articular cartilage(C). Whereas they are arranged in tendon in a parallel manner,they show a rather network-like supramolecular arrangement in articular cartilage.K.Gelse et al./Advanced Drug Delivery Reviews55(2003)1531–15461536theexonN-terminal propeptide is excluded,whereas it is retained in the IIA variant,the embryonic form found in prechondrogenic mesenchyme [33,34],osteo-phytes [35,36],perichondrium,vertebrae [33]and chondrogenic tumors [37].The switch from IIA to IIB suggests a role during developmental processes chains and is widely distributed in collagen I contain-ing tissues with the exception of bone [38].It is an important component of reticular fibres in the inter-stitial tissue of the lungs,liver,dermis,spleen,and vessels.This homotrimeric molecule also often con-tributes to mixed fibrils with type I collagen and is also abundant in elastic tissues [39].Types V and XI collagens are formed as hetero-trimers of three different a -chains (a 1,a 2,a 3).It is remarkable that the a 3-chain of type XI collagen is encoded by the same gene as the a 1-chain of type II collagen and only the extent of glycosylation and hydroxylation differs from a 1(II)[4].Although it is finally not sorted out,a combination between differ-ent types V and XI chains appears to exist in various tissues [40–43].Thus,types V and XI collagens form a subfamily within fibril-forming collagens,though they share similar biochemical properties and func-tions with other members of this family.As men-tioned before,type V collagen typically forms heterofibrils with types I and III collagens and contributes to the organic bone matrix,corneal stro-ma and the interstitial matrix of muscles,liver,lungs,and placenta [12].Type XI collagen codistributes largely in articular cartilage with type II collagen [4,13].The large amino-terminal non-collagenous domains of types V and XI collagens are processed only partially after secretion and their incorporation into the heterofibrils is thought to control their assembly,growth,and diameter [44].Since their triple helical domains are immunologically masked in tissues,they are thought to be located central in the fibrils rather than on their surface [12,45].Thus,type V collagen may function as a core structure of the fibrils with types I and III collagens polymerizing around this central axis.Analogous to this model,type XI collagen is supposed to form the core ofII heterofibrils [3].A high content of in the N-terminal domains of a 1(V)-and a 2(V)-chains,with 40%of the residues being O-sulfated,supports a strong interaction with the more basic triple helical part and is likely to stabilize the fibrillar complex [46].3.2.Collagen types IX,XII,and XIV—The F ACIT collagensThe collagen types IX,XII,XIV,XVI,XIX,and XX belong to the so-called F ibril-A ssociated C olla-gens with I nterrupted T riple helices (FACIT colla-gens).The structures of these collagens are characterized by ‘‘collagenous domains’’interrupted by short non-helical domains and the trimeric mole-cules are associated with the surfaces of various fibrils.Collagen type IX codistributes with type II colla-gen in cartilage and the vitreous body [4].The heterotrimeric molecule consists of three different a -chains (a 1(IX),a 2(IX),and a 3(IX))forming three triple helical segments flanked by four globular domains (NC1–NC4)[47].Type IX collagen mole-cules are located periodically along the surface of type II collagen fibrils in antiparallel direction [48].This interaction is stabilized by covalent lysine-derived cross-links to the N-telopeptide of type II collagen.A hinge region in the NC3domain provides flexibility in the molecule and allows the large and highly cationic globular N-terminal domain to reach out from the fibril where it presumably interacts with proteo-glycans or other matrix components [13,49].A chon-droitin-sulfate side chain is covalently linked to a serine residue of the a 2(IX)-chain in the NC3domain and the size may vary between tissues [50].It might be involved in the linkage of various collagen fibres as well as their interaction with molecules of the extracellular matrix.Additionally,collagen type XVI is found in hyaline cartilage and skin [51]and is associated with a subset of the collagen ‘‘type II fibers’’(Graessel,personal communication).Types XII and type XIV collagens are similar in structure and share sequence homologies to type IX collagen.Both molecules associate or colocalize with type I collagen in skin,perichondrium,periosteum,tendons,lung,liver,placenta,and vessel walls [4].The function of these collagens,as well as of collagen55(2003)1531–15461537types XIX [52]and XX [53],within the tissue is still poorly understood.3.3.Collagen type VI—a microfibrillar collagen Type VI collagen is an heterotrimer of three differ-ent a -chains (a 1,a 2,a 3)with short triple helical domains and rather extended globular termini [54,55].This is in particular true for the a 3-chain which is nearly as twice as long as the other chains due to a large N-and C-terminal globular domains.However,these extended domains are subject not only to alternative splicing,but also to extensive posttrans-lational processing,both within and outside the cell [56,57].The primary fibrils assemble already inside the cell to antiparallel,overlapping dimers,which then align in a parallel manner to form tetramers.Following secretion into the extracellular matrix,type VI collagen tetramers aggregate to filaments and form an indepen-dent microfibrillar network in virtually all connective tissues,except bone [14,57,58].Type VI collagen fibrils appear on the ultrastructural level as fine fila-ments,microfibrils or segments with faint crossband-ing of 110-nm periodicity [58–63],although not all fine filaments represent type VI collagen [64–68].3.4.Collagen types X and VIII—short chain collagens Types X and VIII collagens are structurally related short-chain collagens.Type X collagen is a charac-teristic component of hypertrophic cartilage in the fetal and juvenile growth plate,in ribs and vertebrae [7].It is a homotrimeric collagen with a large C-terminal and a short N-terminal domain and experi-ments in vitro are indicative for its assembly to hexagonal networks [69].The function of type X collagen is not completely resolved.A role in endo-chondral ossification and matrix calcification is dis-cussed.Thus,type X collagen is thought to be involved in the calcification process in the lower hypertrophic zone [69–72],a possibility supported by the restricted expression of type X collagen in thecalcified zone of adult articular cartilage [73,74]and its prevalence in the calcified chick egg shell [75].In fetal cartilage,type X collagen has been localized in fine filaments as well as associated with type II fibrils.[76].Mutations of the COL10A1gene are causative for the disease Schmid type metaphyseal chondrodysplasia (SMCD)impeding endochondral ossification in the metaphyseal growth plate.This leads to growth deficiency and skeletal deformities with short limbs [77].Type VIII collagen is very homologous to type X collagen in structure but shows a distinct distribution and may therefore have different functions [78].This network-forming collagen is produced by endothelial cells and assembles in hexagonal lattices,e.g.in the Descemet’s membrane in the cornea [79].3.5.Collagen type IV—the collagen of basement membranesType IV collagen is the most important structural component of basement membranes integrating lam-inins,nidogens and other components into the visible two-dimensional stable supramolecular ag-gregate.The structure of type IV collagen is characterized by three domains:the N-terminal 7S domain,a C-terminal globular domain (NC1),and the central triple helical part with short interruptions of the Gly-X-Y repeats resulting in a flexible triple helix.Six subunit chains have been identified yet,a 1(IV)–a 6(IV),associating into three distinct heterotrimeric molecules.The predominant form is represented by a 1(IV)2a 2(IV)heterotrimers forming the essential network in most embryonic and adult basement membranes.Specific dimeric interactions of the C-terminal NC1domains,cross-linking of four 7S domains as well as interactions of the triple helical domains,are fundamental for the stable network of collagen IV [80].The isoforms a 3(IV)–a 6(IV)show restricted,tissue-specific ex-pression patterns and are forming either an inde-pendent homotypic network of a 3(IV)a 4(IV)a 6(IV)Fig.3.Schematic representation of collagen synthesis starting form the nuclear transcription of the collagen genes,mRNA processing,ribosomal protein synthesis (translation)and post-translational modifications,secretion and the final steps of fibril formation.(SP:signal peptidase;GT:hydroxylysyl galactosyltransferase and galactosylhydroxylysyl glucosyltransferase;LH:lysyl hydroxylase;PH:prolyl hydroxylase;OTC:oligosaccharyl transferase complex;PDI:protein disulphide isomerase;PPI:peptidyl-prolyl cis -trans -isomerase;NP:procollagen N -proteinase;CP:procollagen C-proteinase;LO:lysyl oxidase;HSP47:heat shock protein 47,colligin1).K.Gelse et al./Advanced Drug Delivery Reviews 55(2003)1531–15461538K.Gelse et al./Advanced Drug Delivery Reviews55(2003)1531–15461539heterotrimers(kidney,lung)or a composite network of a5(IV)2a6(IV)/a1(IV)2a2(IV)molecules[81]. Mutations of the major isoform a1(IV)2a2(IV)are assumed to be embryonic lethal,but defects of the a5(IV),as well as a3(IV)or a4(IV)-chains are causative for various forms of Alport syndrome due to the importance of the a3a4a6heterotrimer for stability and function of glomerular and alveolar basement membranes[3].4.Biosynthesis of collagensThe biosynthesis of collagens starting with gene transcription of the genes within the nucleus to the aggregation of collagen heterotrimers into large fibrils is a complex multistep process(Fig.3).Since most of our knowledge of these mechanisms is based on fibril-forming collagens,this discussion will mostly focus on type I collagen.It is likely that the basic mecha-nisms of triple helix formation and processing will also apply for other collagen types.4.1.Transcription and translationThe regulation of the transcriptional activities of collagens depends largely on the cell type,but may also be controlled by numerous growth factors and cytokines(for review,see Ref.[38]).Thus,bone formation is stimulated,at least in the adult,by members of the TGF-h-superfamily as well as the insulin-like-growth factors.In other tissues,fibro-blast-growth-factors and many other agents are even more important.To discuss this in more detail is beyond the scope of this review and needs to be evaluated for all collagens and tissues separately.Most collagen genes revealed a complex exon–intron pattern,ranging from3to117exons,with the mRNAs of fibrillar collagens encoded by more than 50exons.Therefore,in many cases,different mRNA species could be detected,caused by multiple tran-scription initiation sites,alternative splicing of exons or combination of both.For example,in the cornea and the vitreous body,a shorter form of type IX collagen mRNA is generated by an additional start site between exons6and7[4].Alternative splicing has been reported for many collagen types and was first described for type II collagen.A longer form of type II collagen(COL2A)is expressed by chondro-progenitor cells and varies from a shorter form (COL2B)where exon2is excluded[33]and which is the main gene product of mature articular chon-drocytes.More recently,more than17different tran-scripts have been reported for type XIII collagen[82] and alternative splicing also generates heterogeneous transcription products for collagens VI,XI,XII[82–85].In addition to splicing,the pre-mRNA undergoes capping at the5V end and polyadenylation at the3V end and the mature mRNA is transported to the cytoplasm and translated at the rough endoplasmatic reticulum.Ribosome-bound mRNA is translated into prepro-collagen molecules which protrude into the lumen of the rough endoplasmatic reticulum with the help of a signal recognition domain recognized by the cor-responding receptors.4.2.Posttranslational modifications of collagenAfter removal of the signal peptide by a signal peptidase(Fig.3),the procollagen molecules undergo multiple steps of post-translational modifications.Hy-droxylation of proline and lysine residues catalyzed by prolyl3-hydroxylase,prolyl4-hydroxylase,and lysyl hydroxylase,respectively.All three enzymes require ferrous ions,2-oxoglutarate,molecular oxy-gen,and ascorbate as cofactors.In fibril-forming collagens,approximately50%of the proline residues contain a hydroxylgroup at position4and the extent of prolyl-hydroxylation is species-dependent.The organisms living at lower environmental temperatures show a lower extent of hydroxylation[86].The presence of4-hydroxyproline is essential for intramo-lecular hydrogen bonds and thus contributes to the thermal stability of the triple helical domain,and therefore also to the integrity of the monomer and collagen fibril.The function of3-hydroxyproline is not known[3].The extent of lysine hydroxylation also varies between tissues and collagen types[87]. Hydroxylysine residues are able to form stable inter-molecular cross-linking of collagen molecules in fibrils and additionally represent sites for the attach-ment of carbohydrates.Glucosyl-and galactosyl-residues are transferred to the hydroxyl groups of hydroxylysine;this is catalyzed by the enzymes hydroxylysyl galactosyltransferase and galactosylhy-droxylysyl-glucosyltransferase,respectively(Fig.3).K.Gelse et al./Advanced Drug Delivery Reviews55(2003)1531–1546 1540。
two-stage least squares analysis
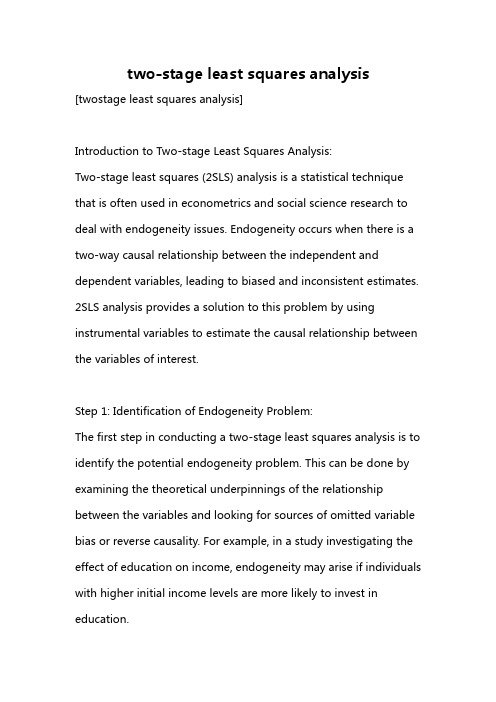
two-stage least squares analysis [twostage least squares analysis]Introduction to Two-stage Least Squares Analysis:Two-stage least squares (2SLS) analysis is a statistical technique that is often used in econometrics and social science research to deal with endogeneity issues. Endogeneity occurs when there is a two-way causal relationship between the independent and dependent variables, leading to biased and inconsistent estimates. 2SLS analysis provides a solution to this problem by using instrumental variables to estimate the causal relationship between the variables of interest.Step 1: Identification of Endogeneity Problem:The first step in conducting a two-stage least squares analysis is to identify the potential endogeneity problem. This can be done by examining the theoretical underpinnings of the relationship between the variables and looking for sources of omitted variable bias or reverse causality. For example, in a study investigating the effect of education on income, endogeneity may arise if individuals with higher initial income levels are more likely to invest in education.Step 2: Selection of Instrumental Variables:Once the endogeneity problem is identified, the next step is to select appropriate instrumental variables. Instrumental variables are variables that are correlated with the endogenous variable but are not directly related to the dependent variable. The instrumental variables should satisfy the conditions of relevance and exogeneity. Relevance means that the instrumental variables have a significant impact on the endogenous variable, while exogeneity implies that the instrumental variables are not affected by the outcome variable.Step 3: Estimation of First Stage Regressions:In the first stage of two-stage least squares analysis, the instrumental variables are used to estimate the relationship between the endogenous variable and the instrumental variables. This estimation is done through a regression analysis, where the endogenous variable is regressed on the instrumental variables. The estimated coefficients from this regression represent the predicted values of the endogenous variable, which are then used in the second stage of the analysis.Step 4: Check for Strong Instruments:After estimating the first stage regressions, it is important to evaluate the strength of the instrumental variables. Weak instruments can lead to biased estimates and reduced precision. Several statistical tests, such as the F-statistic and the Kleibergen-Paap Wald rk F statistic, can be used to assess the strength of the instruments. If the instruments are weak, alternative identification strategies or different instrumental variables should be considered.Step 5: Estimation of Second Stage Regressions:In the second stage of two-stage least squares analysis, the predicted values of the endogenous variable obtained from the first stage regression are used as an instrumental variable in the regression of the dependent variable on the endogenous variable and other control variables. This two-stage regression effectively eliminates the endogeneity problem and provides consistent and unbiased estimates of the causal relationship of interest.Step 6: Interpretation of Results:After conducting the two-stage least squares analysis, the estimated coefficients from the second stage regression can beinterpreted as the causal effects of the independent variables on the dependent variable, accounting for the endogeneity problem. These estimates should be evaluated in conjunction with their statistical significance and the goodness of fit of the regression model.Conclusion:Two-stage least squares analysis is a valuable technique for addressing endogeneity issues in econometric and social science research. By using instrumental variables and conductingtwo-stage regressions, this method provides unbiased and consistent estimates of causal relationships. However, it is important to carefully select appropriate instruments and assess their strength to ensure the validity of the analysis.。
俱乐部趋同
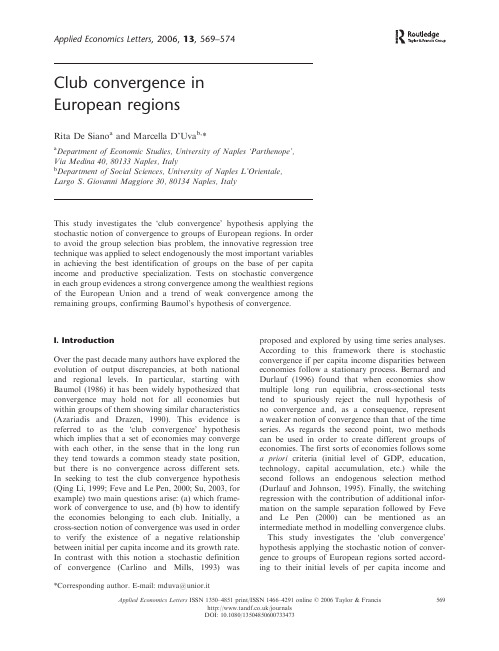
Applied Economics Letters,2006,13,569–574Club convergence inEuropean regionsRita De Siano a and Marcella D’Uva b,*a Department of Economic Studies,University of Naples‘Parthenope’,Via Medina40,80133Naples,Italyb Department of Social Sciences,University of Naples L’Orientale,Largo S.Giovanni Maggiore30,80134Naples,ItalyThis study investigates the‘club convergence’hypothesis applying the stochastic notion of convergence to groups of European regions.In order to avoid the group selection bias problem,the innovative regression tree technique was applied to select endogenously the most important variables in achieving the best identification of groups on the base of per capita income and productive specialization.Tests on stochastic convergence in each group evidences a strong convergence among the wealthiest regions of the European Union and a trend of weak convergence among the remaining groups,confirming Baumol’s hypothesis of convergence.I.IntroductionOver the past decade many authors have explored the evolution of output discrepancies,at both national and regional levels.In particular,starting with Baumol(1986)it has been widely hypothesized that convergence may hold not for all economies but within groups of them showing similar characteristics (Azariadis and Drazen,1990).This evidence is referred to as the‘club convergence’hypothesis which implies that a set of economies may converge with each other,in the sense that in the long run they tend towards a common steady state position, but there is no convergence across different sets. In seeking to test the club convergence hypothesis (Qing Li,1999;Feve and Le Pen,2000;Su,2003,for example)two main questions arise:(a)which frame-work of convergence to use,and(b)how to identify the economies belonging to each club.Initially,a cross-section notion of convergence was used in order to verify the existence of a negative relationship between initial per capita income and its growth rate. In contrast with this notion a stochastic definition of convergence(Carlino and Mills,1993)was proposed and explored by using time series analyses. According to this framework there is stochastic convergence if per capita income disparities between economies follow a stationary process.Bernard and Durlauf(1996)found that when economies show multiple long run equilibria,cross-sectional tests tend to spuriously reject the null hypothesis of no convergence and,as a consequence,represent a weaker notion of convergence than that of the time series.As regards the second point,two methods can be used in order to create different groups of economies.The first sorts of economies follows some a priori criteria(initial level of GDP,education, technology,capital accumulation,etc.)while the second follows an endogenous selection method (Durlauf and Johnson,1995).Finally,the switching regression with the contribution of additional infor-mation on the sample separation followed by Feve and Le Pen(2000)can be mentioned as an intermediate method in modelling convergence clubs. This study investigates the‘club convergence’hypothesis applying the stochastic notion of conver-gence to groups of European regions sorted accord-ing to their initial levels of per capita income and*Corresponding author.E-mail:mduva@unior.itApplied Economics Letters ISSN1350–4851print/ISSN1466–4291onlineß2006Taylor&Francis569/journalsDOI:10.1080/13504850600733473productive specialization(De Siano and D’Uva, 2004,2005)through the application of an innovative methodology known as Classification and Regression Tree Analysis(CART).Unlike other partitioning methods,CART allows a regression to be performed together with a classification analysis on the same ‘learning’dataset,without requiring particular speci-fication of the functional form for the predictor variables which are selected endogenously.The importance of similarities in the initial productive specialization has been highlighted by several theore-tical contributions(Jacobs,1969;Marshall,1980; Romer,1986;Lucas,1988;Helg et al.,1995;Bru lhart, 1998;Ottaviano and Puga,1998)which found that it can be crucial in determining both the nature and size of responses to external shocks.The paper is organized as follows:Section II introduces the methodology of the empirical analysis, Section III displays the dataset,Section IV shows the results of econometric analysis and Section V concludes.II.MethodologyThe empirical analysis is carried out in two parts:first regions are grouped through the classification and regression tree analyses(CART),then convergence is tested within‘clubs’using the time series analysis. CART methodology(Breiman et al.,1984)provides binary recursive partitioning using non-parametric approaches in order to construct homogeneous groups of regions using splitting variables which minimize the intra-group‘impurity’as predictors. The final outcome is a tree with branches and ‘terminal nodes’,as homogeneous as possible,where the average value of the node represents the predicted value of the dependent variable.In this analysis the regression is carried out through the least squares method using the regional GDP growth rate as dependent variable and initial GDP and specializa-tion indexes as explicative variables.In the second part of the study Carlino and Mills(1993)notion of stochastic convergence is applied in each group identified by CART methodology.It follows that if the logarithm of a region’s per capita income relative to the group’s average does not contain a unit root,the region converges.The model(Ben-David, 1994;Qing Li,1999)is the following:y j i,t ¼ iþ i tþ’y i,tÀ1þ"i,tð1Þwhere y j i,t is the log of region i per capita income inyear t,j is the region’s group and"is white noise errorwith0mean.Summing Equation1over j for eachgroup and dividing the outcome by the number ofregions within the group,the following equation isobtained:"y t¼" þ" tþ’"y tÀ1þ"tð2Þwhere"y t is the group’s average per capita incomein year t(the group superscript is suppressed).Subtracting Equation2from Equation1one has:RI i,t¼AþBtþ’RI i,tÀ1þ"tð3Þwhere RI i,t is the logarithm of region i per capitaincome relative to the group’s average at time t(y j i,tÀ"y t).For each region of the sample we apply theAugmented Dickey–Fuller(ADF)test(Dickey andFuller,1979)using the ADF regression ofEquation3:ÁRI t¼ þ tþ RI tÀ1þX kj¼1c jÁRI tÀjþ"tð4ÞAt this point,considering the low power of the ADFtest in the case of short time series,we run alsothe Kwiatkowski et al.(1992)test(KPSS)for trendstationarity.The null hypothesis of the KPSS test isthe trend stationarity against the unit root alter-native.If the KPSS statistic is larger than the criticalvalues the null hypothesis is rejected.The combinedanalysis of KPSS and ADF tests results leads on thefollowing possibilities(Qing Li,1999):.rejection by ADF tests and failure to reject byKPSS!strong convergence;.failure to reject by both ADF and KPSS!weakconvergence;.rejection by KPSS test and failure to rejectADF!no convergence;.rejection by both ADF and KPSS tests invitesto perform further analyses.III.Data DescriptionThis section presents the dataset used both to groupthe sample regions and to run the econometricanalysis.Data for GDP and employment are fromthe Eurostat New Cronos Regio database at NUTS2level.1Annual values for GDP per inhabitant in termsof Purchasing Power Parity(PPP)and the number of1According to EC Regulation No.1059/2003.570R.De Siano and M.D’Uvaemployees in the NACE92productive branches from1981to 2000are used.The sample consists of 123regions belonging to nine countries:11Belgian,8Dutch,29German,222French,20Italian,18Spanish,5Portuguese,2Greek,38British.4For each region (i )the following initial productivespecialization indexes (SP)were built for all theconsidered branches 5(j ):SP ij ¼E ij P n j ¼1ij P m i ¼1E ij P n j ¼1P mi ¼1ijð5Þwhere E indicates the number of employees.IV.Empirical ResultsThe main purpose of the study is to test the ‘clubconvergence’hypothesis across the European regions.In particular,the study aims to investigate whethera region’s per capita income converges to the averageof the group to which it belongs.In order to avoidthe group selection bias problem,the regressiontree technique was applied to select endogenouslythe most important variables in achieving thebest identification of groups (De Siano and D’Uva,2005).If the majority of regions in a groupconverges,the group may be considered a conver-gence ‘club’.The CART method allowed a tree to be built withfour terminal nodes including regions showing a morehomogeneous behaviour of per capita GDP growthrate and productive specialization.Results of CARTanalysis together with the stochastic convergence tests for each group are presented in what follows.The first group consists of 11regions (from Spain,Greece and Portugal)characterized by:the highest estimated mean value of GDP growth rate (126.08%)despite the lowest initial income level (average equal to 4144.3);strong specialization in the agriculture sector (the highest and equal to 3.75),construction branch (2.09)and food and beverages compartment (1.93);the minimum specialization in chemical,energy,and machinery branches and the highest in food-beverages-tobacco,mineral and construction.More than 80%of these regions display ‘weak’convergence while remaining regions show ‘strong’convergence (Table 1).The second group includes 23regions (mainly from Belgium,Spain,Italy and the United Kingdom)characterized by:an average GDP growth rate equal to 111.36%and the second highest initial income level (5788.78);strong specialization in agriculture (2.68)sector,food and beverage (1.26),construction (1.52)and energy (1.20)compartments;the highest specialization in chemical products (0.98);the second highest level of specialization in agricul-ture construction and energy.Almost all these regions present ‘weak’convergence (Table 2).The third group is formed by 21regions from Belgium,France,Germany,the Netherlands,Spain,the UK and Italy (only Abruzzo)characterized by:an estimate for the GDP growth rate of 106%and an average initial level of income equal to 6920.6;main specializations in manufacturing (1.03),mineral products (1.13),construction (1.22),food and beverage (1.45)and energy (1.21);the highest 2The analysis starts from 1984due to the lack of data in the respective regional labour statistics.3During the period 1983–1987there has been a different aggregation of Greek regions at NUTS2level.Kriti and Thessalia are the only regions which presents data for the period 1984–2000.4The geographic units for UK are at NUTS1level of Eurostat classification because of the lack of data for NUTS2units.5Agricultural-forestry and fishery,manufacturing,fuel and power products,non-metallic minerals and minerals,food-beverages-tobacco,textiles-clothing-leather and footwear,chemical products,metal products,machinery-equipment and electrical goods,various industries,building and construction,transport and communication,credit and insurance services.Table 1.Convergence test results of group 1Regions group 1ADF statistics KPSS statistics l ¼4Regions group 1ADF statistics KPSS statistics l ¼4Castilla-la ManchaÀ2.9780.099gr 43Kriti À4.05ÃÃ0.080ExtremaduraÀ3.320.097Pt11Norte À4.03ÃÃ0.126AndaluciaÀ2.630.094Pt12Centro (P)À2.290.123Ceuta y MelillaÀ1.770.123Pt14Alentejo À2.770.104CanariasÀ1.940.121Pt15Algarve À2.010.086ThessaliaÀ1.760.137Notes :ÃÃdenote statistical significance using unit root critical values at the 5%(À3.645).Club convergence in European regions571specialization in energy and manufacturing branches.Except for Abruzzo and Noord Brabant,which donot converge,all the other regions ‘weakly’convergeto the group’s average (Table 3).The fourth group contains 68regions (almost allGerman,French and Italian (North-Centre)andsome Belgian and Dutch)characterized by thelowest estimation of the GDP growth rate (97.8%),despite their highest initial GDP level (8893.9);thehighest specialization in the branches of the servicessector (1.16and 1.07,respectively)and in machinery(1.01);the lowest specialization in agriculture,foodand beverages,textile and construction activities.These regions present the highest percentage of‘strong’convergence to the group’s average (morethan 60%,Table 4).Table 5presents the summary of convergence testsresults (percentage are in parentheses).The main outcome of this study is the evidence of strong convergence among the wealthiest regions of the European Union.Besides,it appears that there is a trend of weak convergence also among the remaining groups (percentages are considerably over 80%).Therefore,Baumol’s hypothesis of conver-gence within clubs showing similar characteristics is confirmed.V.Conclusion This study tests the ‘club convergence’hypothesis applying the stochastic notion of convergence to groups of European regions.In order to avoid the group selection bias problem,the innovative regression tree technique was applied to selectTable 3.Convergence test results of group 3Regions group 3ADF statistics KPSS statistics l ¼4Regions group 3ADF statistics KPSS statistics l ¼4LimburgÀ1.680.116Abruzzo 2.600.153ÃÃHainautÀ0.800.091Friesland À3.620.142NamurÀ1.840.094Noord-Brabant À2.590.148ÃÃNiederbayernÀ1.270.104Limburg (NL)À2.980.128OberpfalzÀ1.400.097Yorkshire and The Humber À1.610.085TrierÀ1.430.119East Midlands À2.190.091Comunidad Foral de NavarraÀ2.750.071West Midlands À1.920.080La RiojaÀ1.770.119East Anglia À2.150.134BalearesÀ2.960.108South West À1.950.091LimousinÀ2.410.083Scotland 2.220.093Languedoc-RoussillonÀ3.390.105Notes :ÃÃdenote statistical significance using KPSS stationary critical values at the 5%level (0.146).Table 2.Convergence test results of group 2Regions group 2ADF statistics KPSS statistics l ¼4Regions group 2ADF statistics KPSS statistics l ¼4Vlaams BrabantÀ1.220.100Murcia À1.530.124Brabant WallonÀ1.600.111Molise À2.170.078Luxembourg1.190.122Campania À3.220.078Lu neburgÀ0.280.114Puglia À2.820.115GaliciaÀ1.690.140Basilicata À2.100.140Principado de AsturiasÀ1.550.146ÃÃCalabria À5.07ÃÃÃ0.106CantabriaÀ1.080.133Sicilia À2.980.142Aragon À1.580.142Sardegna À2.210.141Comunidad de MadridÀ1.380.091Lisboa e Vale do Tejo À2.620.141Castilla y Leon À2.580.138Wales À2.120.098Cataluna À1.550.097Northern Ireland À1.790.120Comunidad Valenciana À1.420.105Notes :ÃÃand ÃÃÃdenote statistical significance using KPSS stationary critical values at the 5%level (0.146)and 1%level (0.216)respectively,using unit root critical values at the 5%(À3.645)and 1%(À4.469).572R.De Siano and M.D’Uvaendogenously the most important variables inachieving the best identification of groups.Testson stochastic convergence in each group identifiedby CART evidence strong convergence among thewealthiest regions of the European Union and atrend of weak convergence among the remaininggroups.References Azariadis,C.and Drazen,A.(1990)Threshold externalities in economic development,Quarterly Journal of Economics ,105,501–26.Baumol,W.J.(1986)Productivity growth,convergence and welfare:what the long run data show,AmericanEconomic Review ,76,1072–85.Table 5.Convergence test resultsGroupsNo.of regions Strong convergence Weak convergence No convergence 1112(18,19)9(81,81)2231(4.35)21(91.3)1(4.35)32119(90.48)2(9.52)46843(63.23)20(29.41)4(5.88)Table 4.Convergence test results of group 4Regions group 4ADF statistics KPSS statistics l ¼4Regions group 4ADF statistics KPSS statistics l ¼4RegionBruxelles capitale À2.650.112Haute-Normandie À4.11ÃÃ0.102AntwerpenÀ2.770.102Centre (FR)À5.13ÃÃÃ0.099Oost-VlaanderenÀ3.150.078Basse-Normandie À3.86ÃÃ0.101West-VlaanderenÀ3.030.097Bourgogne À5.03ÃÃÃ0.113Licge À3.060.089Nord-Pas-de-Calais À4.37ÃÃ0.130StuttgartÀ4.22ÃÃ0.123Lorraine À4.41ÃÃ0.139KarlsruheÀ4.51ÃÃÃ0.088Alsace À4.13ÃÃ0.094FreiburgÀ5.11ÃÃÃ0.092Franche-Comte À5.20ÃÃÃ0.145Tu bingenÀ4.94ÃÃÃ0.104Pays de la Loire À4.34ÃÃ0.116OberbayernÀ4.17ÃÃ0.094Bretagne À4.41ÃÃ0.124MittelfrankenÀ3.79ÃÃ0.089Poitou-Charentes À4.74ÃÃÃ0.102UnterfrankenÀ0.420.140Aquitaine À3.290.104SchwabenÀ4.11ÃÃ0.084Midi-Pyre ne es À5.48ÃÃÃ0.103BremenÀ3.76ÃÃ0.121Rho ne-Alpes À4.93ÃÃÃ0.104HamburgÀ3.350.097Auvergne À4.43ÃÃ0.135DarmstadtÀ3.150.125Provence-Alpes-Co te d’Azur À5.10ÃÃÃ0.109GießenÀ3.020.088Corse À2.560.166ÃÃKasselÀ3.0120.094Piemonte À3.460.112BraunschweigÀ3.82ÃÃ0.116Valle d’Aosta À4.36ÃÃ0.080HannoverÀ3.96ÃÃ0.083Liguria À4.26ÃÃ0.117Weser-EmsÀ3.400.084Lombardia À4.04ÃÃ0.101Du sseldorfÀ3.94ÃÃ0.097Trentino-Alto Adige À3.84ÃÃ0.109Ko lnÀ3.96ÃÃ0.084Veneto À3.68ÃÃ0.106Mu nsterÀ4.04ÃÃ0.087Friuli-Venezia Giulia À4.20ÃÃ0.116DetmoldÀ4.06ÃÃ0.099Emilia-Romagna À3.120.136ArnsbergÀ3.98ÃÃ0.096Toscana À3.190.121KoblenzÀ3.88ÃÃ0.113Umbria À3.560.146ÃÃRheinhessen-PfalzÀ4.18ÃÃ0.107Marche À3.250.136SaarlandÀ4.35ÃÃ0.090Lazio À3.96ÃÃ0.098Schleswig-HolsteinÀ3.360.089Drenthe À1.850.134Pais VascoÀ3.630.159ÃÃUtrecht À2.400.155ÃÃI le de FranceÀ4.61ÃÃÃ0.110Noord-Holland À1.990.137Champagne ArdenneÀ3.79ÃÃ0.157ÃÃZuid-Holland À2.200.138Picardie À4.44ÃÃ0.142Zeeland À3.78ÃÃ0.093Notes :ÃÃand ÃÃÃdenote statistical significance using KPSS stationary critical values at the 5%level (0.146)and 1%level (0.216)respectively,using unit root critical values at the 5%(À3.645)and 1%(‘4.469).Club convergence in European regions573Ben-David, D.(1994)Convergence clubs and diverging economies,unpublished manuscript,University of Houston,Ben-Gurion University and CEPR. Bernard, A. B.and Durlauf,S.N.(1996)Interpreting tests of the convergence hypothesis,Journal of Econometrics,71,161–73.Breiman,L.,Friedman,J.L.,Olshen,R.A.and Stone,C.J.,(1984)Classification and Regression Trees,Wadsworth,Belmont,CA.Bru lhart,M.(1998)Economic geography,industrial location and trade:the evidence,World Economy,21, 775–801.Carlino,G.A.and Mills,L.O.(1993)Are US regional incomes converging?A time series analysis,Journal of Monetary Economics,32,335–46.De Siano,R.and D’Uva,M.(2004)Specializzazione e crescita:un’applicazione alle regioni dell’Unione Monetaria Europea,Rivista Internazionale di Scienze Sociali,4,381–98.De Siano,R.and D’Uva,M.(2005)Regional growth in Europe:an analysis through CART methodology, Studi Economici,87,115–28.Dickey,D.A.and Fuller,W.A.(1979)Distribution of the estimators for autoregressive time series with a unit root,Journal of The American Statistical Association, 74,427–31.Durlauf,S.N.and Johnson,P.A.(1995)Multiple regimes and cross-country growth behaviour,Journal of Applied Econometrics,10,365–84.Feve,P.and Le Pen,Y.(2000)On modelling convergence clubs,Applied Economic Letters,7,311–14.Helg,R.,Manasse,P.,Monacelli,T.and Rovelli,R.(1995) How much(a)symmetry in Europe?Evidence from industrial sectors,European Economic Review,39, 1017–41.Jacobs,J.(1969)The Economy of Cities,Jonathen Cape, London.Kwiatkowski, D.,Phillips,P. C. B.,Schmidt,P.and Shin,Y.(1992)Testing the null hypothesis of stationarity against the alternative of a unit root:how sure are we that economic time series have a unit root?,Journal of Econometrics,54, 159–78.Lucas,R. E.(1988)On the mechanics of economic development,Journal of Monetary Economics,22, 3–42.Marshall,A.(1980)Principles of Economics,Macmillan, London.Ottaviano,I.and Puga,D.(1998)Agglomeration in the global economy:a survey of the‘new economic geography’,World Economy,21,707–31.Qing,L.(1999)Convergence clubs:some further evidence, Review of International Economics,7,59–67. Romer,P.M.(1986)Increasing returns and long run growth,Journal of Political Economy,94, 1002–37.Su,J.J.(2003)Convergence clubs among15OECD countries,Applied Economic Letters,10,113–18.574R.De Siano and M.D’Uva。
斜率之和为0二级结论
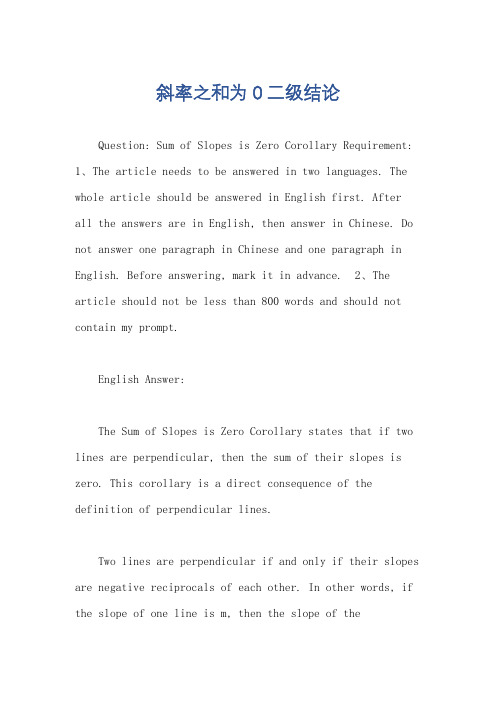
斜率之和为0二级结论Question: Sum of Slopes is Zero Corollary Requirement: 1、The article needs to be answered in two languages. The whole article should be answered in English first. Afterall the answers are in English, then answer in Chinese. Do not answer one paragraph in Chinese and one paragraph in English. Before answering, mark it in advance. 2、The article should not be less than 800 words and should not contain my prompt.English Answer:The Sum of Slopes is Zero Corollary states that if two lines are perpendicular, then the sum of their slopes is zero. This corollary is a direct consequence of the definition of perpendicular lines.Two lines are perpendicular if and only if their slopes are negative reciprocals of each other. In other words, if the slope of one line is m, then the slope of theperpendicular line is -1/m.The Sum of Slopes is Zero Corollary can be used to solve a variety of problems involving perpendicular lines. For example, it can be used to find the equation of a line that is perpendicular to a given line, or to find the distance between two parallel lines.中文回答。
T.W. ANDERSON (1971). The Statistical Analysis of Time Series. Series in Probability and Ma

425 BibliographyH.A KAIKE(1974).Markovian representation of stochastic processes and its application to the analysis of autoregressive moving average processes.Annals Institute Statistical Mathematics,vol.26,pp.363-387. B.D.O.A NDERSON and J.B.M OORE(1979).Optimal rmation and System Sciences Series, Prentice Hall,Englewood Cliffs,NJ.T.W.A NDERSON(1971).The Statistical Analysis of Time Series.Series in Probability and Mathematical Statistics,Wiley,New York.R.A NDRE-O BRECHT(1988).A new statistical approach for the automatic segmentation of continuous speech signals.IEEE Trans.Acoustics,Speech,Signal Processing,vol.ASSP-36,no1,pp.29-40.R.A NDRE-O BRECHT(1990).Reconnaissance automatique de parole`a partir de segments acoustiques et de mod`e les de Markov cach´e s.Proc.Journ´e es Etude de la Parole,Montr´e al,May1990(in French).R.A NDRE-O BRECHT and H.Y.S U(1988).Three acoustic labellings for phoneme based continuous speech recognition.Proc.Speech’88,Edinburgh,UK,pp.943-950.U.A PPEL and A.VON B RANDT(1983).Adaptive sequential segmentation of piecewise stationary time rmation Sciences,vol.29,no1,pp.27-56.L.A.A ROIAN and H.L EVENE(1950).The effectiveness of quality control procedures.Jal American Statis-tical Association,vol.45,pp.520-529.K.J.A STR¨OM and B.W ITTENMARK(1984).Computer Controlled Systems:Theory and rma-tion and System Sciences Series,Prentice Hall,Englewood Cliffs,NJ.M.B AGSHAW and R.A.J OHNSON(1975a).The effect of serial correlation on the performance of CUSUM tests-Part II.Technometrics,vol.17,no1,pp.73-80.M.B AGSHAW and R.A.J OHNSON(1975b).The influence of reference values and estimated variance on the ARL of CUSUM tests.Jal Royal Statistical Society,vol.37(B),no3,pp.413-420.M.B AGSHAW and R.A.J OHNSON(1977).Sequential procedures for detecting parameter changes in a time-series model.Jal American Statistical Association,vol.72,no359,pp.593-597.R.K.B ANSAL and P.P APANTONI-K AZAKOS(1986).An algorithm for detecting a change in a stochastic process.IEEE rmation Theory,vol.IT-32,no2,pp.227-235.G.A.B ARNARD(1959).Control charts and stochastic processes.Jal Royal Statistical Society,vol.B.21, pp.239-271.A.E.B ASHARINOV andB.S.F LEISHMAN(1962).Methods of the statistical sequential analysis and their radiotechnical applications.Sovetskoe Radio,Moscow(in Russian).M.B ASSEVILLE(1978).D´e viations par rapport au maximum:formules d’arrˆe t et martingales associ´e es. Compte-rendus du S´e minaire de Probabilit´e s,Universit´e de Rennes I.M.B ASSEVILLE(1981).Edge detection using sequential methods for change in level-Part II:Sequential detection of change in mean.IEEE Trans.Acoustics,Speech,Signal Processing,vol.ASSP-29,no1,pp.32-50.426B IBLIOGRAPHY M.B ASSEVILLE(1982).A survey of statistical failure detection techniques.In Contribution`a la D´e tectionS´e quentielle de Ruptures de Mod`e les Statistiques,Th`e se d’Etat,Universit´e de Rennes I,France(in English). M.B ASSEVILLE(1986).The two-models approach for the on-line detection of changes in AR processes. In Detection of Abrupt Changes in Signals and Dynamical Systems(M.Basseville,A.Benveniste,eds.). Lecture Notes in Control and Information Sciences,LNCIS77,Springer,New York,pp.169-215.M.B ASSEVILLE(1988).Detecting changes in signals and systems-A survey.Automatica,vol.24,pp.309-326.M.B ASSEVILLE(1989).Distance measures for signal processing and pattern recognition.Signal Process-ing,vol.18,pp.349-369.M.B ASSEVILLE and A.B ENVENISTE(1983a).Design and comparative study of some sequential jump detection algorithms for digital signals.IEEE Trans.Acoustics,Speech,Signal Processing,vol.ASSP-31, no3,pp.521-535.M.B ASSEVILLE and A.B ENVENISTE(1983b).Sequential detection of abrupt changes in spectral charac-teristics of digital signals.IEEE rmation Theory,vol.IT-29,no5,pp.709-724.M.B ASSEVILLE and A.B ENVENISTE,eds.(1986).Detection of Abrupt Changes in Signals and Dynamical Systems.Lecture Notes in Control and Information Sciences,LNCIS77,Springer,New York.M.B ASSEVILLE and I.N IKIFOROV(1991).A unified framework for statistical change detection.Proc.30th IEEE Conference on Decision and Control,Brighton,UK.M.B ASSEVILLE,B.E SPIAU and J.G ASNIER(1981).Edge detection using sequential methods for change in level-Part I:A sequential edge detection algorithm.IEEE Trans.Acoustics,Speech,Signal Processing, vol.ASSP-29,no1,pp.24-31.M.B ASSEVILLE, A.B ENVENISTE and G.M OUSTAKIDES(1986).Detection and diagnosis of abrupt changes in modal characteristics of nonstationary digital signals.IEEE rmation Theory,vol.IT-32,no3,pp.412-417.M.B ASSEVILLE,A.B ENVENISTE,G.M OUSTAKIDES and A.R OUG´E E(1987a).Detection and diagnosis of changes in the eigenstructure of nonstationary multivariable systems.Automatica,vol.23,no3,pp.479-489. M.B ASSEVILLE,A.B ENVENISTE,G.M OUSTAKIDES and A.R OUG´E E(1987b).Optimal sensor location for detecting changes in dynamical behavior.IEEE Trans.Automatic Control,vol.AC-32,no12,pp.1067-1075.M.B ASSEVILLE,A.B ENVENISTE,B.G ACH-D EVAUCHELLE,M.G OURSAT,D.B ONNECASE,P.D OREY, M.P REVOSTO and M.O LAGNON(1993).Damage monitoring in vibration mechanics:issues in diagnos-tics and predictive maintenance.Mechanical Systems and Signal Processing,vol.7,no5,pp.401-423.R.V.B EARD(1971).Failure Accommodation in Linear Systems through Self-reorganization.Ph.D.Thesis, Dept.Aeronautics and Astronautics,MIT,Cambridge,MA.A.B ENVENISTE and J.J.F UCHS(1985).Single sample modal identification of a nonstationary stochastic process.IEEE Trans.Automatic Control,vol.AC-30,no1,pp.66-74.A.B ENVENISTE,M.B ASSEVILLE and G.M OUSTAKIDES(1987).The asymptotic local approach to change detection and model validation.IEEE Trans.Automatic Control,vol.AC-32,no7,pp.583-592.A.B ENVENISTE,M.M ETIVIER and P.P RIOURET(1990).Adaptive Algorithms and Stochastic Approxima-tions.Series on Applications of Mathematics,(A.V.Balakrishnan,I.Karatzas,M.Yor,eds.).Springer,New York.A.B ENVENISTE,M.B ASSEVILLE,L.E L G HAOUI,R.N IKOUKHAH and A.S.W ILLSKY(1992).An optimum robust approach to statistical failure detection and identification.IFAC World Conference,Sydney, July1993.B IBLIOGRAPHY427 R.H.B ERK(1973).Some asymptotic aspects of sequential analysis.Annals Statistics,vol.1,no6,pp.1126-1138.R.H.B ERK(1975).Locally most powerful sequential test.Annals Statistics,vol.3,no2,pp.373-381.P.B ILLINGSLEY(1968).Convergence of Probability Measures.Wiley,New York.A.F.B ISSELL(1969).Cusum techniques for quality control.Applied Statistics,vol.18,pp.1-30.M.E.B IVAIKOV(1991).Control of the sample size for recursive estimation of parameters subject to abrupt changes.Automation and Remote Control,no9,pp.96-103.R.E.B LAHUT(1987).Principles and Practice of Information Theory.Addison-Wesley,Reading,MA.I.F.B LAKE and W.C.L INDSEY(1973).Level-crossing problems for random processes.IEEE r-mation Theory,vol.IT-19,no3,pp.295-315.G.B ODENSTEIN and H.M.P RAETORIUS(1977).Feature extraction from the encephalogram by adaptive segmentation.Proc.IEEE,vol.65,pp.642-652.T.B OHLIN(1977).Analysis of EEG signals with changing spectra using a short word Kalman estimator. Mathematical Biosciences,vol.35,pp.221-259.W.B¨OHM and P.H ACKL(1990).Improved bounds for the average run length of control charts based on finite weighted sums.Annals Statistics,vol.18,no4,pp.1895-1899.T.B OJDECKI and J.H OSZA(1984).On a generalized disorder problem.Stochastic Processes and their Applications,vol.18,pp.349-359.L.I.B ORODKIN and V.V.M OTTL’(1976).Algorithm forfinding the jump times of random process equation parameters.Automation and Remote Control,vol.37,no6,Part1,pp.23-32.A.A.B OROVKOV(1984).Theory of Mathematical Statistics-Estimation and Hypotheses Testing,Naouka, Moscow(in Russian).Translated in French under the title Statistique Math´e matique-Estimation et Tests d’Hypoth`e ses,Mir,Paris,1987.G.E.P.B OX and G.M.J ENKINS(1970).Time Series Analysis,Forecasting and Control.Series in Time Series Analysis,Holden-Day,San Francisco.A.VON B RANDT(1983).Detecting and estimating parameters jumps using ladder algorithms and likelihood ratio test.Proc.ICASSP,Boston,MA,pp.1017-1020.A.VON B RANDT(1984).Modellierung von Signalen mit Sprunghaft Ver¨a nderlichem Leistungsspektrum durch Adaptive Segmentierung.Doctor-Engineer Dissertation,M¨u nchen,RFA(in German).S.B RAUN,ed.(1986).Mechanical Signature Analysis-Theory and Applications.Academic Press,London. L.B REIMAN(1968).Probability.Series in Statistics,Addison-Wesley,Reading,MA.G.S.B RITOV and L.A.M IRONOVSKI(1972).Diagnostics of linear systems of automatic regulation.Tekh. Kibernetics,vol.1,pp.76-83.B.E.B RODSKIY and B.S.D ARKHOVSKIY(1992).Nonparametric Methods in Change-point Problems. Kluwer Academic,Boston.L.D.B ROEMELING(1982).Jal Econometrics,vol.19,Special issue on structural change in Econometrics. L.D.B ROEMELING and H.T SURUMI(1987).Econometrics and Structural Change.Dekker,New York. D.B ROOK and D.A.E VANS(1972).An approach to the probability distribution of Cusum run length. Biometrika,vol.59,pp.539-550.J.B RUNET,D.J AUME,M.L ABARR`E RE,A.R AULT and M.V ERG´E(1990).D´e tection et Diagnostic de Pannes.Trait´e des Nouvelles Technologies,S´e rie Diagnostic et Maintenance,Herm`e s,Paris(in French).428B IBLIOGRAPHY S.P.B RUZZONE and M.K AVEH(1984).Information tradeoffs in using the sample autocorrelation function in ARMA parameter estimation.IEEE Trans.Acoustics,Speech,Signal Processing,vol.ASSP-32,no4, pp.701-715.A.K.C AGLAYAN(1980).Necessary and sufficient conditions for detectability of jumps in linear systems. IEEE Trans.Automatic Control,vol.AC-25,no4,pp.833-834.A.K.C AGLAYAN and R.E.L ANCRAFT(1983).Reinitialization issues in fault tolerant systems.Proc.Amer-ican Control Conf.,pp.952-955.A.K.C AGLAYAN,S.M.A LLEN and K.W EHMULLER(1988).Evaluation of a second generation reconfigu-ration strategy for aircraftflight control systems subjected to actuator failure/surface damage.Proc.National Aerospace and Electronic Conference,Dayton,OH.P.E.C AINES(1988).Linear Stochastic Systems.Series in Probability and Mathematical Statistics,Wiley, New York.M.J.C HEN and J.P.N ORTON(1987).Estimation techniques for tracking rapid parameter changes.Intern. Jal Control,vol.45,no4,pp.1387-1398.W.K.C HIU(1974).The economic design of cusum charts for controlling normal mean.Applied Statistics, vol.23,no3,pp.420-433.E.Y.C HOW(1980).A Failure Detection System Design Methodology.Ph.D.Thesis,M.I.T.,L.I.D.S.,Cam-bridge,MA.E.Y.C HOW and A.S.W ILLSKY(1984).Analytical redundancy and the design of robust failure detection systems.IEEE Trans.Automatic Control,vol.AC-29,no3,pp.689-691.Y.S.C HOW,H.R OBBINS and D.S IEGMUND(1971).Great Expectations:The Theory of Optimal Stop-ping.Houghton-Mifflin,Boston.R.N.C LARK,D.C.F OSTH and V.M.W ALTON(1975).Detection of instrument malfunctions in control systems.IEEE Trans.Aerospace Electronic Systems,vol.AES-11,pp.465-473.A.C OHEN(1987).Biomedical Signal Processing-vol.1:Time and Frequency Domain Analysis;vol.2: Compression and Automatic Recognition.CRC Press,Boca Raton,FL.J.C ORGE and F.P UECH(1986).Analyse du rythme cardiaque foetal par des m´e thodes de d´e tection de ruptures.Proc.7th INRIA Int.Conf.Analysis and optimization of Systems.Antibes,FR(in French).D.R.C OX and D.V.H INKLEY(1986).Theoretical Statistics.Chapman and Hall,New York.D.R.C OX and H.D.M ILLER(1965).The Theory of Stochastic Processes.Wiley,New York.S.V.C ROWDER(1987).A simple method for studying run-length distributions of exponentially weighted moving average charts.Technometrics,vol.29,no4,pp.401-407.H.C S¨ORG¨O and L.H ORV´ATH(1988).Nonparametric methods for change point problems.In Handbook of Statistics(P.R.Krishnaiah,C.R.Rao,eds.),vol.7,Elsevier,New York,pp.403-425.R.B.D AVIES(1973).Asymptotic inference in stationary gaussian time series.Advances Applied Probability, vol.5,no3,pp.469-497.J.C.D ECKERT,M.N.D ESAI,J.J.D EYST and A.S.W ILLSKY(1977).F-8DFBW sensor failure identification using analytical redundancy.IEEE Trans.Automatic Control,vol.AC-22,no5,pp.795-803.M.H.D E G ROOT(1970).Optimal Statistical Decisions.Series in Probability and Statistics,McGraw-Hill, New York.J.D ESHAYES and D.P ICARD(1979).Tests de ruptures dans un mod`e pte-Rendus de l’Acad´e mie des Sciences,vol.288,Ser.A,pp.563-566(in French).B IBLIOGRAPHY429 J.D ESHAYES and D.P ICARD(1983).Ruptures de Mod`e les en Statistique.Th`e ses d’Etat,Universit´e deParis-Sud,Orsay,France(in French).J.D ESHAYES and D.P ICARD(1986).Off-line statistical analysis of change-point models using non para-metric and likelihood methods.In Detection of Abrupt Changes in Signals and Dynamical Systems(M. Basseville,A.Benveniste,eds.).Lecture Notes in Control and Information Sciences,LNCIS77,Springer, New York,pp.103-168.B.D EVAUCHELLE-G ACH(1991).Diagnostic M´e canique des Fatigues sur les Structures Soumises`a des Vibrations en Ambiance de Travail.Th`e se de l’Universit´e Paris IX Dauphine(in French).B.D EVAUCHELLE-G ACH,M.B ASSEVILLE and A.B ENVENISTE(1991).Diagnosing mechanical changes in vibrating systems.Proc.SAFEPROCESS’91,Baden-Baden,FRG,pp.85-89.R.D I F RANCESCO(1990).Real-time speech segmentation using pitch and convexity jump models:applica-tion to variable rate speech coding.IEEE Trans.Acoustics,Speech,Signal Processing,vol.ASSP-38,no5, pp.741-748.X.D ING and P.M.F RANK(1990).Fault detection via factorization approach.Systems and Control Letters, vol.14,pp.431-436.J.L.D OOB(1953).Stochastic Processes.Wiley,New York.V.D RAGALIN(1988).Asymptotic solutions in detecting a change in distribution under an unknown param-eter.Statistical Problems of Control,Issue83,Vilnius,pp.45-52.B.D UBUISSON(1990).Diagnostic et Reconnaissance des Formes.Trait´e des Nouvelles Technologies,S´e rie Diagnostic et Maintenance,Herm`e s,Paris(in French).A.J.D UNCAN(1986).Quality Control and Industrial Statistics,5th edition.Richard D.Irwin,Inc.,Home-wood,IL.J.D URBIN(1971).Boundary-crossing probabilities for the Brownian motion and Poisson processes and techniques for computing the power of the Kolmogorov-Smirnov test.Jal Applied Probability,vol.8,pp.431-453.J.D URBIN(1985).Thefirst passage density of the crossing of a continuous Gaussian process to a general boundary.Jal Applied Probability,vol.22,no1,pp.99-122.A.E MAMI-N AEINI,M.M.A KHTER and S.M.R OCK(1988).Effect of model uncertainty on failure detec-tion:the threshold selector.IEEE Trans.Automatic Control,vol.AC-33,no12,pp.1106-1115.J.D.E SARY,F.P ROSCHAN and D.W.W ALKUP(1967).Association of random variables with applications. Annals Mathematical Statistics,vol.38,pp.1466-1474.W.D.E WAN and K.W.K EMP(1960).Sampling inspection of continuous processes with no autocorrelation between successive results.Biometrika,vol.47,pp.263-280.G.F AVIER and A.S MOLDERS(1984).Adaptive smoother-predictors for tracking maneuvering targets.Proc. 23rd Conf.Decision and Control,Las Vegas,NV,pp.831-836.W.F ELLER(1966).An Introduction to Probability Theory and Its Applications,vol.2.Series in Probability and Mathematical Statistics,Wiley,New York.R.A.F ISHER(1925).Theory of statistical estimation.Proc.Cambridge Philosophical Society,vol.22, pp.700-725.M.F ISHMAN(1988).Optimization of the algorithm for the detection of a disorder,based on the statistic of exponential smoothing.In Statistical Problems of Control,Issue83,Vilnius,pp.146-151.R.F LETCHER(1980).Practical Methods of Optimization,2volumes.Wiley,New York.P.M.F RANK(1990).Fault diagnosis in dynamic systems using analytical and knowledge based redundancy -A survey and new results.Automatica,vol.26,pp.459-474.430B IBLIOGRAPHY P.M.F RANK(1991).Enhancement of robustness in observer-based fault detection.Proc.SAFEPRO-CESS’91,Baden-Baden,FRG,pp.275-287.P.M.F RANK and J.W¨UNNENBERG(1989).Robust fault diagnosis using unknown input observer schemes. In Fault Diagnosis in Dynamic Systems-Theory and Application(R.Patton,P.Frank,R.Clark,eds.). International Series in Systems and Control Engineering,Prentice Hall International,London,UK,pp.47-98.K.F UKUNAGA(1990).Introduction to Statistical Pattern Recognition,2d ed.Academic Press,New York. S.I.G ASS(1958).Linear Programming:Methods and Applications.McGraw Hill,New York.W.G E and C.Z.F ANG(1989).Extended robust observation approach for failure isolation.Int.Jal Control, vol.49,no5,pp.1537-1553.W.G ERSCH(1986).Two applications of parametric time series modeling methods.In Mechanical Signature Analysis-Theory and Applications(S.Braun,ed.),chap.10.Academic Press,London.J.J.G ERTLER(1988).Survey of model-based failure detection and isolation in complex plants.IEEE Control Systems Magazine,vol.8,no6,pp.3-11.J.J.G ERTLER(1991).Analytical redundancy methods in fault detection and isolation.Proc.SAFEPRO-CESS’91,Baden-Baden,FRG,pp.9-22.B.K.G HOSH(1970).Sequential Tests of Statistical Hypotheses.Addison-Wesley,Cambridge,MA.I.N.G IBRA(1975).Recent developments in control charts techniques.Jal Quality Technology,vol.7, pp.183-192.J.P.G ILMORE and R.A.M C K ERN(1972).A redundant strapdown inertial reference unit(SIRU).Jal Space-craft,vol.9,pp.39-47.M.A.G IRSHICK and H.R UBIN(1952).A Bayes approach to a quality control model.Annals Mathematical Statistics,vol.23,pp.114-125.A.L.G OEL and S.M.W U(1971).Determination of the ARL and a contour nomogram for CUSUM charts to control normal mean.Technometrics,vol.13,no2,pp.221-230.P.L.G OLDSMITH and H.W HITFIELD(1961).Average run lengths in cumulative chart quality control schemes.Technometrics,vol.3,pp.11-20.G.C.G OODWIN and K.S.S IN(1984).Adaptive Filtering,Prediction and rmation and System Sciences Series,Prentice Hall,Englewood Cliffs,NJ.R.M.G RAY and L.D.D AVISSON(1986).Random Processes:a Mathematical Approach for Engineers. Information and System Sciences Series,Prentice Hall,Englewood Cliffs,NJ.C.G UEGUEN and L.L.S CHARF(1980).Exact maximum likelihood identification for ARMA models:a signal processing perspective.Proc.1st EUSIPCO,Lausanne.D.E.G USTAFSON, A.S.W ILLSKY,J.Y.W ANG,M.C.L ANCASTER and J.H.T RIEBWASSER(1978). ECG/VCG rhythm diagnosis using statistical signal analysis.Part I:Identification of persistent rhythms. Part II:Identification of transient rhythms.IEEE Trans.Biomedical Engineering,vol.BME-25,pp.344-353 and353-361.F.G USTAFSSON(1991).Optimal segmentation of linear regression parameters.Proc.IFAC/IFORS Symp. Identification and System Parameter Estimation,Budapest,pp.225-229.T.H¨AGGLUND(1983).New Estimation Techniques for Adaptive Control.Ph.D.Thesis,Lund Institute of Technology,Lund,Sweden.T.H¨AGGLUND(1984).Adaptive control of systems subject to large parameter changes.Proc.IFAC9th World Congress,Budapest.B IBLIOGRAPHY431 P.H ALL and C.C.H EYDE(1980).Martingale Limit Theory and its Application.Probability and Mathemat-ical Statistics,a Series of Monographs and Textbooks,Academic Press,New York.W.J.H ALL,R.A.W IJSMAN and J.K.G HOSH(1965).The relationship between sufficiency and invariance with applications in sequential analysis.Ann.Math.Statist.,vol.36,pp.576-614.E.J.H ANNAN and M.D EISTLER(1988).The Statistical Theory of Linear Systems.Series in Probability and Mathematical Statistics,Wiley,New York.J.D.H EALY(1987).A note on multivariate CuSum procedures.Technometrics,vol.29,pp.402-412.D.M.H IMMELBLAU(1970).Process Analysis by Statistical Methods.Wiley,New York.D.M.H IMMELBLAU(1978).Fault Detection and Diagnosis in Chemical and Petrochemical Processes. Chemical Engineering Monographs,vol.8,Elsevier,Amsterdam.W.G.S.H INES(1976a).A simple monitor of a system with sudden parameter changes.IEEE r-mation Theory,vol.IT-22,no2,pp.210-216.W.G.S.H INES(1976b).Improving a simple monitor of a system with sudden parameter changes.IEEE rmation Theory,vol.IT-22,no4,pp.496-499.D.V.H INKLEY(1969).Inference about the intersection in two-phase regression.Biometrika,vol.56,no3, pp.495-504.D.V.H INKLEY(1970).Inference about the change point in a sequence of random variables.Biometrika, vol.57,no1,pp.1-17.D.V.H INKLEY(1971).Inference about the change point from cumulative sum-tests.Biometrika,vol.58, no3,pp.509-523.D.V.H INKLEY(1971).Inference in two-phase regression.Jal American Statistical Association,vol.66, no336,pp.736-743.J.R.H UDDLE(1983).Inertial navigation system error-model considerations in Kalmanfiltering applica-tions.In Control and Dynamic Systems(C.T.Leondes,ed.),Academic Press,New York,pp.293-339.J.S.H UNTER(1986).The exponentially weighted moving average.Jal Quality Technology,vol.18,pp.203-210.I.A.I BRAGIMOV and R.Z.K HASMINSKII(1981).Statistical Estimation-Asymptotic Theory.Applications of Mathematics Series,vol.16.Springer,New York.R.I SERMANN(1984).Process fault detection based on modeling and estimation methods-A survey.Auto-matica,vol.20,pp.387-404.N.I SHII,A.I WATA and N.S UZUMURA(1979).Segmentation of nonstationary time series.Int.Jal Systems Sciences,vol.10,pp.883-894.J.E.J ACKSON and R.A.B RADLEY(1961).Sequential and tests.Annals Mathematical Statistics, vol.32,pp.1063-1077.B.J AMES,K.L.J AMES and D.S IEGMUND(1988).Conditional boundary crossing probabilities with appli-cations to change-point problems.Annals Probability,vol.16,pp.825-839.M.K.J EERAGE(1990).Reliability analysis of fault-tolerant IMU architectures with redundant inertial sen-sors.IEEE Trans.Aerospace and Electronic Systems,vol.AES-5,no.7,pp.23-27.N.L.J OHNSON(1961).A simple theoretical approach to cumulative sum control charts.Jal American Sta-tistical Association,vol.56,pp.835-840.N.L.J OHNSON and F.C.L EONE(1962).Cumulative sum control charts:mathematical principles applied to their construction and use.Parts I,II,III.Industrial Quality Control,vol.18,pp.15-21;vol.19,pp.29-36; vol.20,pp.22-28.432B IBLIOGRAPHY R.A.J OHNSON and M.B AGSHAW(1974).The effect of serial correlation on the performance of CUSUM tests-Part I.Technometrics,vol.16,no.1,pp.103-112.H.L.J ONES(1973).Failure Detection in Linear Systems.Ph.D.Thesis,Dept.Aeronautics and Astronautics, MIT,Cambridge,MA.R.H.J ONES,D.H.C ROWELL and L.E.K APUNIAI(1970).Change detection model for serially correlated multivariate data.Biometrics,vol.26,no2,pp.269-280.M.J URGUTIS(1984).Comparison of the statistical properties of the estimates of the change times in an autoregressive process.In Statistical Problems of Control,Issue65,Vilnius,pp.234-243(in Russian).T.K AILATH(1980).Linear rmation and System Sciences Series,Prentice Hall,Englewood Cliffs,NJ.L.V.K ANTOROVICH and V.I.K RILOV(1958).Approximate Methods of Higher Analysis.Interscience,New York.S.K ARLIN and H.M.T AYLOR(1975).A First Course in Stochastic Processes,2d ed.Academic Press,New York.S.K ARLIN and H.M.T AYLOR(1981).A Second Course in Stochastic Processes.Academic Press,New York.D.K AZAKOS and P.P APANTONI-K AZAKOS(1980).Spectral distance measures between gaussian pro-cesses.IEEE Trans.Automatic Control,vol.AC-25,no5,pp.950-959.K.W.K EMP(1958).Formula for calculating the operating characteristic and average sample number of some sequential tests.Jal Royal Statistical Society,vol.B-20,no2,pp.379-386.K.W.K EMP(1961).The average run length of the cumulative sum chart when a V-mask is used.Jal Royal Statistical Society,vol.B-23,pp.149-153.K.W.K EMP(1967a).Formal expressions which can be used for the determination of operating character-istics and average sample number of a simple sequential test.Jal Royal Statistical Society,vol.B-29,no2, pp.248-262.K.W.K EMP(1967b).A simple procedure for determining upper and lower limits for the average sample run length of a cumulative sum scheme.Jal Royal Statistical Society,vol.B-29,no2,pp.263-265.D.P.K ENNEDY(1976).Some martingales related to cumulative sum tests and single server queues.Stochas-tic Processes and Appl.,vol.4,pp.261-269.T.H.K ERR(1980).Statistical analysis of two-ellipsoid overlap test for real time failure detection.IEEE Trans.Automatic Control,vol.AC-25,no4,pp.762-772.T.H.K ERR(1982).False alarm and correct detection probabilities over a time interval for restricted classes of failure detection algorithms.IEEE rmation Theory,vol.IT-24,pp.619-631.T.H.K ERR(1987).Decentralizedfiltering and redundancy management for multisensor navigation.IEEE Trans.Aerospace and Electronic systems,vol.AES-23,pp.83-119.Minor corrections on p.412and p.599 (May and July issues,respectively).R.A.K HAN(1978).Wald’s approximations to the average run length in cusum procedures.Jal Statistical Planning and Inference,vol.2,no1,pp.63-77.R.A.K HAN(1979).Somefirst passage problems related to cusum procedures.Stochastic Processes and Applications,vol.9,no2,pp.207-215.R.A.K HAN(1981).A note on Page’s two-sided cumulative sum procedures.Biometrika,vol.68,no3, pp.717-719.B IBLIOGRAPHY433 V.K IREICHIKOV,V.M ANGUSHEV and I.N IKIFOROV(1990).Investigation and application of CUSUM algorithms to monitoring of sensors.In Statistical Problems of Control,Issue89,Vilnius,pp.124-130(in Russian).G.K ITAGAWA and W.G ERSCH(1985).A smoothness prior time-varying AR coefficient modeling of non-stationary covariance time series.IEEE Trans.Automatic Control,vol.AC-30,no1,pp.48-56.N.K LIGIENE(1980).Probabilities of deviations of the change point estimate in statistical models.In Sta-tistical Problems of Control,Issue83,Vilnius,pp.80-86(in Russian).N.K LIGIENE and L.T ELKSNYS(1983).Methods of detecting instants of change of random process prop-erties.Automation and Remote Control,vol.44,no10,Part II,pp.1241-1283.J.K ORN,S.W.G ULLY and A.S.W ILLSKY(1982).Application of the generalized likelihood ratio algorithm to maneuver detection and estimation.Proc.American Control Conf.,Arlington,V A,pp.792-798.P.R.K RISHNAIAH and B.Q.M IAO(1988).Review about estimation of change points.In Handbook of Statistics(P.R.Krishnaiah,C.R.Rao,eds.),vol.7,Elsevier,New York,pp.375-402.P.K UDVA,N.V ISWANADHAM and A.R AMAKRISHNAN(1980).Observers for linear systems with unknown inputs.IEEE Trans.Automatic Control,vol.AC-25,no1,pp.113-115.S.K ULLBACK(1959).Information Theory and Statistics.Wiley,New York(also Dover,New York,1968). K.K UMAMARU,S.S AGARA and T.S¨ODERSTR¨OM(1989).Some statistical methods for fault diagnosis for dynamical systems.In Fault Diagnosis in Dynamic Systems-Theory and Application(R.Patton,P.Frank,R. Clark,eds.).International Series in Systems and Control Engineering,Prentice Hall International,London, UK,pp.439-476.A.K USHNIR,I.N IKIFOROV and I.S AVIN(1983).Statistical adaptive algorithms for automatic detection of seismic signals-Part I:One-dimensional case.In Earthquake Prediction and the Study of the Earth Structure,Naouka,Moscow(Computational Seismology,vol.15),pp.154-159(in Russian).L.L ADELLI(1990).Diffusion approximation for a pseudo-likelihood test process with application to de-tection of change in stochastic system.Stochastics and Stochastics Reports,vol.32,pp.1-25.T.L.L A¨I(1974).Control charts based on weighted sums.Annals Statistics,vol.2,no1,pp.134-147.T.L.L A¨I(1981).Asymptotic optimality of invariant sequential probability ratio tests.Annals Statistics, vol.9,no2,pp.318-333.D.G.L AINIOTIS(1971).Joint detection,estimation,and system identifirmation and Control, vol.19,pp.75-92.M.R.L EADBETTER,G.L INDGREN and H.R OOTZEN(1983).Extremes and Related Properties of Random Sequences and Processes.Series in Statistics,Springer,New York.L.L E C AM(1960).Locally asymptotically normal families of distributions.Univ.California Publications in Statistics,vol.3,pp.37-98.L.L E C AM(1986).Asymptotic Methods in Statistical Decision Theory.Series in Statistics,Springer,New York.E.L.L EHMANN(1986).Testing Statistical Hypotheses,2d ed.Wiley,New York.J.P.L EHOCZKY(1977).Formulas for stopped diffusion processes with stopping times based on the maxi-mum.Annals Probability,vol.5,no4,pp.601-607.H.R.L ERCHE(1980).Boundary Crossing of Brownian Motion.Lecture Notes in Statistics,vol.40,Springer, New York.L.L JUNG(1987).System Identification-Theory for the rmation and System Sciences Series, Prentice Hall,Englewood Cliffs,NJ.。
Rough Approximation of a perference Relation by Dominance Relations
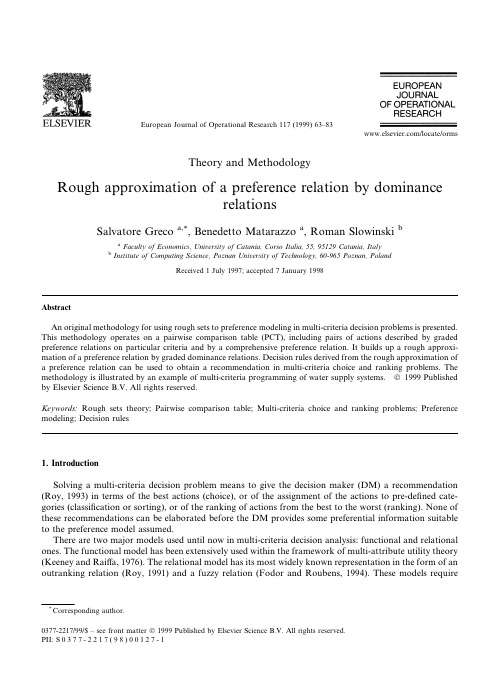
SPSS词汇中英文对照

SPSS词汇(中英文对照) Absolute deviation, 绝对离差Absolute number, 绝对数Absolute residuals, 绝对残差Acceleration array, 加速度立体阵Acceleration in an arbitrary direction, 任意方向上的加速度Acceleration normal, 法向加速度Acceleration space dimension, 加速度空间的维数Acceleration tangential, 切向加速度Acceleration vector, 加速度向量Acceptable hypothesis, 可接受假设Accumulation, 累积Accuracy, 准确度Actual frequency, 实际频数Adaptive estimator, 自适应估计量Addition, 相加Addition theorem, 加法定理Additivity, 可加性Adjusted rate, 调整率Adjusted value, 校正值Admissible error, 容许误差Aggregation, 聚集性Alternative hypothesis, 备择假设Among groups, 组间Amounts, 总量Analysis of correlation, 相关分析Analysis of covariance, 协方差分析Analysis of regression, 回归分析Analysis of time series, 时间序列分析Analysis of variance, 方差分析Angular transformation, 角转换ANOVA (analysis of variance), 方差分析ANOVA Models, 方差分析模型Arcing, 弧/弧旋Arcsine transformation, 反正弦变换Area under the curve, 曲线面积AREG , 评估从一个时间点到下一个时间点回归相关时的误差ARIMA, 季节和非季节性单变量模型的极大似然估计Arithmetic grid paper, 算术格纸Arithmetic mean, 算术平均数Arrhenius relation, 艾恩尼斯关系Assessing fit, 拟合的评估Associative laws, 结合律Asymmetric distribution, 非对称分布Asymptotic bias, 渐近偏倚Asymptotic efficiency, 渐近效率Asymptotic variance, 渐近方差Attributable risk, 归因危险度Attribute data, 属性资料Attribution, 属性Autocorrelation, 自相关Autocorrelation of residuals, 残差的自相关Average, 平均数Average confidence interval length, 平均置信区间长度Average growth rate, 平均增长率Bar chart, 条形图Bar graph, 条形图Base period, 基期Bayes' theorem , Bayes定理Bell-shaped curve, 钟形曲线Bernoulli distribution, 伯努力分布Best-trim estimator, 最好切尾估计量Bias, 偏性Binary logistic regression, 二元逻辑斯蒂回归Binomial distribution, 二项分布Bisquare, 双平方Bivariate Correlate, 二变量相关Bivariate normal distribution, 双变量正态分布Bivariate normal population, 双变量正态总体Biweight interval, 双权区间Biweight M-estimator, 双权M估计量Block, 区组/配伍组BMDP(Biomedical computer programs), BMDP统计软件包Boxplots, 箱线图/箱尾图Breakdown bound, 崩溃界/崩溃点Canonical correlation, 典型相关Caption, 纵标目Case-control study, 病例对照研究Categorical variable, 分类变量Catenary, 悬链线Cauchy distribution, 柯西分布Cause-and-effect relationship, 因果关系Cell, 单元Censoring, 终检Center of symmetry, 对称中心Centering and scaling, 中心化和定标Central tendency, 集中趋势Central value, 中心值CHAID -χ2 Automatic Interaction Detector, 卡方自动交互检测Chance, 机遇Chance error, 随机误差Chance variable, 随机变量Characteristic equation, 特征方程Characteristic root, 特征根Characteristic vector, 特征向量Chebshev criterion of fit, 拟合的切比雪夫准则Chernoff faces, 切尔诺夫脸谱图Chi-square test, 卡方检验/χ2检验Choleskey decomposition, 乔洛斯基分解Circle chart, 圆图Class interval, 组距Class mid-value, 组中值Class upper limit, 组上限Classified variable, 分类变量Cluster analysis, 聚类分析Cluster sampling, 整群抽样Code, 代码Coded data, 编码数据Coding, 编码Coefficient of contingency, 列联系数Coefficient of determination, 决定系数Coefficient of multiple correlation, 多重相关系数Coefficient of partial correlation, 偏相关系数Coefficient of production-moment correlation, 积差相关系数Coefficient of rank correlation, 等级相关系数Coefficient of regression, 回归系数Coefficient of skewness, 偏度系数Coefficient of variation, 变异系数Cohort study, 队列研究Column, 列Column effect, 列效应Column factor, 列因素Combination pool, 合并Combinative table, 组合表Common factor, 共性因子Common regression coefficient, 公共回归系数Common value, 共同值Common variance, 公共方差Common variation, 公共变异Communality variance, 共性方差Comparability, 可比性Comparison of bathes, 批比较Comparison value, 比较值Compartment model, 分部模型Compassion, 伸缩Complement of an event, 补事件Complete association, 完全正相关Complete dissociation, 完全不相关Complete statistics, 完备统计量Completely randomized design, 完全随机化设计Composite event, 联合事件Composite events, 复合事件Concavity, 凹性Conditional expectation, 条件期望Conditional likelihood, 条件似然Conditional probability, 条件概率Conditionally linear, 依条件线性Confidence interval, 置信区间Confidence limit, 置信限Confidence lower limit, 置信下限Confidence upper limit, 置信上限Confirmatory Factor Analysis , 验证性因子分析Confirmatory research, 证实性实验研究Confounding factor, 混杂因素Conjoint, 联合分析Consistency, 相合性Consistency check, 一致性检验Consistent asymptotically normal estimate, 相合渐近正态估计Consistent estimate, 相合估计Constrained nonlinear regression, 受约束非线性回归Constraint, 约束Contaminated distribution, 污染分布Contaminated Gausssian, 污染高斯分布Contaminated normal distribution, 污染正态分布Contamination, 污染Contamination model, 污染模型Contingency table, 列联表Contour, 边界线Contribution rate, 贡献率Control, 对照Controlled experiments, 对照实验Conventional depth, 常规深度Convolution, 卷积Corrected factor, 校正因子Corrected mean, 校正均值Correction coefficient, 校正系数Correctness, 正确性Correlation coefficient, 相关系数Correlation index, 相关指数Correspondence, 对应Counting, 计数Counts, 计数/频数Covariance, 协方差Covariant, 共变Cox Regression, Cox回归Criteria for fitting, 拟合准则Criteria of least squares, 最小二乘准则Critical ratio, 临界比Critical region, 拒绝域Critical value, 临界值Cross-over design, 交叉设计Cross-section analysis, 横断面分析Cross-section survey, 横断面调查Crosstabs , 交叉表Cross-tabulation table, 复合表Cube root, 立方根Cumulative distribution function, 分布函数Cumulative probability, 累计概率Curvature, 曲率/弯曲Curvature, 曲率Curve fit , 曲线拟和Curve fitting, 曲线拟合Curvilinear regression, 曲线回归Curvilinear relation, 曲线关系Cut-and-try method, 尝试法Cycle, 周期Cyclist, 周期性D test, D检验Data acquisition, 资料收集Data bank, 数据库Data capacity, 数据容量Data deficiencies, 数据缺乏Data handling, 数据处理Data manipulation, 数据处理Data processing, 数据处理Data reduction, 数据缩减Data set, 数据集Data sources, 数据来源Data transformation, 数据变换Data validity, 数据有效性Data-in, 数据输入Data-out, 数据输出Dead time, 停滞期Degree of freedom, 自由度Degree of precision, 精密度Degree of reliability, 可靠性程度Degression, 递减Density function, 密度函数Density of data points, 数据点的密度Dependent variable, 应变量/依变量/因变量Dependent variable, 因变量Depth, 深度Derivative matrix, 导数矩阵Derivative-free methods, 无导数方法Design, 设计Determinacy, 确定性Determinant, 行列式Determinant, 决定因素Deviation, 离差Deviation from average, 离均差Diagnostic plot, 诊断图Dichotomous variable, 二分变量Differential equation, 微分方程Direct standardization, 直接标准化法Discrete variable, 离散型变量DISCRIMINANT, 判断Discriminant analysis, 判别分析Discriminant coefficient, 判别系数Discriminant function, 判别值Dispersion, 散布/分散度Disproportional, 不成比例的Disproportionate sub-class numbers, 不成比例次级组含量Distribution free, 分布无关性/免分布Distribution shape, 分布形状Distribution-free method, 任意分布法Distributive laws, 分配律Disturbance, 随机扰动项Dose response curve, 剂量反应曲线Double blind method, 双盲法Double blind trial, 双盲试验Double exponential distribution, 双指数分布Double logarithmic, 双对数Downward rank, 降秩Dual-space plot, 对偶空间图DUD, 无导数方法Duncan's new multiple range method, 新复极差法/Duncan新法Effect, 实验效应Eigenvalue, 特征值Eigenvector, 特征向量Ellipse, 椭圆Empirical distribution, 经验分布Empirical probability, 经验概率单位Enumeration data, 计数资料Equal sun-class number, 相等次级组含量Equally likely, 等可能Equivariance, 同变性Error, 误差/错误Error of estimate, 估计误差Error type I, 第一类错误Error type II, 第二类错误Estimand, 被估量Estimated error mean squares, 估计误差均方Estimated error sum of squares, 估计误差平方和Euclidean distance, 欧式距离Event, 事件Event, 事件Exceptional data point, 异常数据点Expectation plane, 期望平面Expectation surface, 期望曲面Expected values, 期望值Experiment, 实验Experimental sampling, 试验抽样Experimental unit, 试验单位Explanatory variable, 说明变量Exploratory data analysis, 探索性数据分析Explore Summarize, 探索-摘要Exponential curve, 指数曲线Exponential growth, 指数式增长EXSMOOTH, 指数平滑方法Extended fit, 扩充拟合Extra parameter, 附加参数Extrapolation, 外推法Extreme observation, 末端观测值Extremes, 极端值/极值F distribution, F分布F test, F检验Factor, 因素/因子Factor analysis, 因子分析Factor Analysis, 因子分析Factor score, 因子得分Factorial, 阶乘Factorial design, 析因试验设计False negative, 假阴性False negative error, 假阴性错误Family of distributions, 分布族Family of estimators, 估计量族Fanning, 扇面Fatality rate, 病死率Field investigation, 现场调查Field survey, 现场调查Finite population, 有限总体Finite-sample, 有限样本First derivative, 一阶导数First principal component, 第一主成分First quartile, 第一四分位数Fisher information, 费雪信息量Fitted value, 拟合值Fitting a curve, 曲线拟合Fixed base, 定基Fluctuation, 随机起伏Forecast, 预测Four fold table, 四格表Fourth, 四分点Fraction blow, 左侧比率Fractional error, 相对误差Frequency, 频率Frequency polygon, 频数多边图Frontier point, 界限点Function relationship, 泛函关系Gamma distribution, 伽玛分布Gauss increment, 高斯增量Gaussian distribution, 高斯分布/正态分布Gauss-Newton increment, 高斯-牛顿增量General census, 全面普查GENLOG (Generalized liner models), 广义线性模型Geometric mean, 几何平均数Gini's mean difference, 基尼均差GLM (General liner models), 一般线性模型Goodness of fit, 拟和优度/配合度Gradient of determinant, 行列式的梯度Graeco-Latin square, 希腊拉丁方Grand mean, 总均值Gross errors, 重大错误Gross-error sensitivity, 大错敏感度Group averages, 分组平均Grouped data, 分组资料Guessed mean, 假定平均数Half-life, 半衰期Hampel M-estimators, 汉佩尔M估计量Happenstance, 偶然事件Harmonic mean, 调和均数Hazard function, 风险均数Hazard rate, 风险率Heading, 标目Heavy-tailed distribution, 重尾分布Hessian array, 海森立体阵Heterogeneity, 不同质Heterogeneity of variance, 方差不齐Hierarchical classification, 组内分组Hierarchical clustering method, 系统聚类法High-leverage point, 高杠杆率点HILOGLINEAR, 多维列联表的层次对数线性模型Hinge, 折叶点Histogram, 直方图Historical cohort study, 历史性队列研究Holes, 空洞HOMALS, 多重响应分析Homogeneity of variance, 方差齐性Homogeneity test, 齐性检验Huber M-estimators, 休伯M估计量Hyperbola, 双曲线Hypothesis testing, 假设检验Hypothetical universe, 假设总体Impossible event, 不可能事件Independence, 独立性Independent variable, 自变量Index, 指标/指数Indirect standardization, 间接标准化法Individual, 个体Inference band, 推断带Infinite population, 无限总体Infinitely great, 无穷大Infinitely small, 无穷小Influence curve, 影响曲线Information capacity, 信息容量Initial condition, 初始条件Initial estimate, 初始估计值Initial level, 最初水平Interaction, 交互作用Interaction terms, 交互作用项Intercept, 截距Interpolation, 内插法Interquartile range, 四分位距Interval estimation, 区间估计Intervals of equal probability, 等概率区间Intrinsic curvature, 固有曲率Invariance, 不变性Inverse matrix, 逆矩阵Inverse probability, 逆概率Inverse sine transformation, 反正弦变换Iteration, 迭代Jacobian determinant, 雅可比行列式Joint distribution function, 分布函数Joint probability, 联合概率Joint probability distribution, 联合概率分布K means method, 逐步聚类法Kaplan-Meier, 评估事件的时间长度Kaplan-Merier chart, Kaplan-Merier图Kendall's rank correlation, Kendall等级相关Kinetic, 动力学Kolmogorov-Smirnove test, 柯尔莫哥洛夫-斯米尔诺夫检验Kruskal and Wallis test, Kruskal及Wallis检验/多样本的秩和检验/H检验Kurtosis, 峰度Lack of fit, 失拟Ladder of powers, 幂阶梯Lag, 滞后Large sample, 大样本Large sample test, 大样本检验Latin square, 拉丁方Latin square design, 拉丁方设计Leakage, 泄漏Least favorable configuration, 最不利构形Least favorable distribution, 最不利分布Least significant difference, 最小显著差法Least square method, 最小二乘法Least-absolute-residuals estimates, 最小绝对残差估计Least-absolute-residuals fit, 最小绝对残差拟合Least-absolute-residuals line, 最小绝对残差线Legend, 图例L-estimator, L估计量L-estimator of location, 位置L估计量L-estimator of scale, 尺度L估计量Level, 水平Life expectance, 预期期望寿命Life table, 寿命表Life table method, 生命表法Light-tailed distribution, 轻尾分布Likelihood function, 似然函数Likelihood ratio, 似然比line graph, 线图Linear correlation, 直线相关Linear equation, 线性方程Linear programming, 线性规划Linear regression, 直线回归Linear Regression, 线性回归Linear trend, 线性趋势Loading, 载荷Location and scale equivariance, 位置尺度同变性Location equivariance, 位置同变性Location invariance, 位置不变性Location scale family, 位置尺度族Log rank test, 时序检验Logarithmic curve, 对数曲线Logarithmic normal distribution, 对数正态分布Logarithmic scale, 对数尺度Logarithmic transformation, 对数变换Logic check, 逻辑检查Logistic distribution, 逻辑斯特分布Logit transformation, Logit转换LOGLINEAR, 多维列联表通用模型Lognormal distribution, 对数正态分布Lost function, 损失函数Low correlation, 低度相关Lower limit, 下限Lowest-attained variance, 最小可达方差LSD, 最小显著差法的简称Lurking variable, 潜在变量Main effect, 主效应Major heading, 主辞标目Marginal density function, 边缘密度函数Marginal probability, 边缘概率Marginal probability distribution, 边缘概率分布Matched data, 配对资料Matched distribution, 匹配过分布Matching of distribution, 分布的匹配Matching of transformation, 变换的匹配Mathematical expectation, 数学期望Mathematical model, 数学模型Maximum L-estimator, 极大极小L 估计量Maximum likelihood method, 最大似然法Mean, 均数Mean squares between groups, 组间均方Mean squares within group, 组内均方Means (Compare means), 均值-均值比较Median, 中位数Median effective dose, 半数效量Median lethal dose, 半数致死量Median polish, 中位数平滑Median test, 中位数检验Minimal sufficient statistic, 最小充分统计量Minimum distance estimation, 最小距离估计Minimum effective dose, 最小有效量Minimum lethal dose, 最小致死量Minimum variance estimator, 最小方差估计量MINITAB, 统计软件包Minor heading, 宾词标目Missing data, 缺失值Model specification, 模型的确定Modeling Statistics , 模型统计Models for outliers, 离群值模型Modifying the model, 模型的修正Modulus of continuity, 连续性模Morbidity, 发病率Most favorable configuration, 最有利构形Multidimensional Scaling (ASCAL), 多维尺度/多维标度Multinomial Logistic Regression , 多项逻辑斯蒂回归Multiple comparison, 多重比较Multiple correlation , 复相关Multiple covariance, 多元协方差Multiple linear regression, 多元线性回归Multiple response , 多重选项Multiple solutions, 多解Multiplication theorem, 乘法定理Multiresponse, 多元响应Multi-stage sampling, 多阶段抽样Multivariate T distribution, 多元T分布Mutual exclusive, 互不相容Mutual independence, 互相独立Natural boundary, 自然边界Natural dead, 自然死亡Natural zero, 自然零Negative correlation, 负相关Negative linear correlation, 负线性相关Negatively skewed, 负偏Newman-Keuls method, q检验NK method, q检验No statistical significance, 无统计意义Nominal variable, 名义变量Nonconstancy of variability, 变异的非定常性Nonlinear regression, 非线性相关Nonparametric statistics, 非参数统计Nonparametric test, 非参数检验Nonparametric tests, 非参数检验Normal deviate, 正态离差Normal distribution, 正态分布Normal equation, 正规方程组Normal ranges, 正常范围Normal value, 正常值Nuisance parameter, 多余参数/讨厌参数Null hypothesis, 无效假设Numerical variable, 数值变量Objective function, 目标函数Observation unit, 观察单位Observed value, 观察值One sided test, 单侧检验One-way analysis of variance, 单因素方差分析Oneway ANOVA , 单因素方差分析Open sequential trial, 开放型序贯设计Optrim, 优切尾Optrim efficiency, 优切尾效率Order statistics, 顺序统计量Ordered categories, 有序分类Ordinal logistic regression , 序数逻辑斯蒂回归Ordinal variable, 有序变量Orthogonal basis, 正交基Orthogonal design, 正交试验设计Orthogonality conditions, 正交条件ORTHOPLAN, 正交设计Outlier cutoffs, 离群值截断点Outliers, 极端值OVERALS , 多组变量的非线性正规相关Overshoot, 迭代过度Paired design, 配对设计Paired sample, 配对样本Pairwise slopes, 成对斜率Parabola, 抛物线Parallel tests, 平行试验Parameter, 参数Parametric statistics, 参数统计Parametric test, 参数检验Partial correlation, 偏相关Partial regression, 偏回归Partial sorting, 偏排序Partials residuals, 偏残差Pattern, 模式Pearson curves, 皮尔逊曲线Peeling, 退层Percent bar graph, 百分条形图Percentage, 百分比Percentile, 百分位数Percentile curves, 百分位曲线Periodicity, 周期性Permutation, 排列P-estimator, P估计量Pie graph, 饼图Pitman estimator, 皮特曼估计量Pivot, 枢轴量Planar, 平坦Planar assumption, 平面的假设PLANCARDS, 生成试验的计划卡Point estimation, 点估计Poisson distribution, 泊松分布Polishing, 平滑Polled standard deviation, 合并标准差Polled variance, 合并方差Polygon, 多边图Polynomial, 多项式Polynomial curve, 多项式曲线Population, 总体Population attributable risk, 人群归因危险度Positive correlation, 正相关Positively skewed, 正偏Posterior distribution, 后验分布Power of a test, 检验效能Precision, 精密度Predicted value, 预测值Preliminary analysis, 预备性分析Principal component analysis, 主成分分析Prior distribution, 先验分布Prior probability, 先验概率Probabilistic model, 概率模型probability, 概率Probability density, 概率密度Product moment, 乘积矩/协方差Profile trace, 截面迹图Proportion, 比/构成比Proportion allocation in stratified random sampling, 按比例分层随机抽样Proportionate, 成比例Proportionate sub-class numbers, 成比例次级组含量Prospective study, 前瞻性调查Proximities, 亲近性Pseudo F test, 近似F检验Pseudo model, 近似模型Pseudosigma, 伪标准差Purposive sampling, 有目的抽样QR decomposition, QR分解Quadratic approximation, 二次近似Qualitative classification, 属性分类Qualitative method, 定性方法Quantile-quantile plot, 分位数-分位数图/Q-Q图Quantitative analysis, 定量分析Quartile, 四分位数Quick Cluster, 快速聚类Radix sort, 基数排序Random allocation, 随机化分组Random blocks design, 随机区组设计Random event, 随机事件Randomization, 随机化Range, 极差/全距Rank correlation, 等级相关Rank sum test, 秩和检验Rank test, 秩检验Ranked data, 等级资料Rate, 比率Ratio, 比例Raw data, 原始资料Raw residual, 原始残差Rayleigh's test, 雷氏检验Rayleigh's Z, 雷氏Z值Reciprocal, 倒数Reciprocal transformation, 倒数变换Recording, 记录Redescending estimators, 回降估计量Reducing dimensions, 降维Re-expression, 重新表达Reference set, 标准组Region of acceptance, 接受域Regression coefficient, 回归系数Regression sum of square, 回归平方和Rejection point, 拒绝点Relative dispersion, 相对离散度Relative number, 相对数Reliability, 可靠性Reparametrization, 重新设置参数Replication, 重复Report Summaries, 报告摘要Residual sum of square, 剩余平方和Resistance, 耐抗性Resistant line, 耐抗线Resistant technique, 耐抗技术R-estimator of location, 位置R估计量R-estimator of scale, 尺度R估计量Retrospective study, 回顾性调查Ridge trace, 岭迹Ridit analysis, Ridit分析Rotation, 旋转Rounding, 舍入Row, 行Row effects, 行效应Row factor, 行因素RXC table, RXC表Sample, 样本Sample regression coefficient, 样本回归系数Sample size, 样本量Sample standard deviation, 样本标准差Sampling error, 抽样误差SAS(Statistical analysis system ), SAS统计软件包Scale, 尺度/量表Scatter diagram, 散点图Schematic plot, 示意图/简图Score test, 计分检验Screening, 筛检SEASON, 季节分析Second derivative, 二阶导数Second principal component, 第二主成分SEM (Structural equation modeling), 结构化方程模型Semi-logarithmic graph, 半对数图Semi-logarithmic paper, 半对数格纸Sensitivity curve, 敏感度曲线Sequential analysis, 贯序分析Sequential data set, 顺序数据集Sequential design, 贯序设计Sequential method, 贯序法Sequential test, 贯序检验法Serial tests, 系列试验Short-cut method, 简捷法Sigmoid curve, S形曲线Sign function, 正负号函数Sign test, 符号检验Signed rank, 符号秩Significance test, 显著性检验Significant figure, 有效数字Simple cluster sampling, 简单整群抽样Simple correlation, 简单相关Simple random sampling, 简单随机抽样Simple regression, 简单回归simple table, 简单表Sine estimator, 正弦估计量Single-valued estimate, 单值估计Singular matrix, 奇异矩阵Skewed distribution, 偏斜分布Skewness, 偏度Slash distribution, 斜线分布Slope, 斜率Smirnov test, 斯米尔诺夫检验Source of variation, 变异来源Spearman rank correlation, 斯皮尔曼等级相关Specific factor, 特殊因子Specific factor variance, 特殊因子方差Spectra , 频谱Spherical distribution, 球型正态分布Spread, 展布SPSS(Statistical package for the social science), SPSS统计软件包Spurious correlation, 假性相关Square root transformation, 平方根变换Stabilizing variance, 稳定方差Standard deviation, 标准差Standard error, 标准误Standard error of difference, 差别的标准误Standard error of estimate, 标准估计误差Standard error of rate, 率的标准误Standard normal distribution, 标准正态分布Standardization, 标准化Starting value, 起始值Statistic, 统计量Statistical control, 统计控制Statistical graph, 统计图Statistical inference, 统计推断Statistical table, 统计表Steepest descent, 最速下降法Stem and leaf display, 茎叶图Step factor, 步长因子Stepwise regression, 逐步回归Storage, 存Strata, 层(复数)Stratified sampling, 分层抽样Stratified sampling, 分层抽样Strength, 强度Stringency, 严密性Structural relationship, 结构关系Studentized residual, 学生化残差/t化残差Sub-class numbers, 次级组含量Subdividing, 分割Sufficient statistic, 充分统计量Sum of products, 积和Sum of squares, 离差平方和Sum of squares about regression, 回归平方和Sum of squares between groups, 组间平方和Sum of squares of partial regression, 偏回归平方和Sure event, 必然事件Survey, 调查Survival, 生存分析Survival rate, 生存率Suspended root gram, 悬吊根图Symmetry, 对称Systematic error, 系统误差Systematic sampling, 系统抽样Tags, 标签Tail area, 尾部面积Tail length, 尾长Tail weight, 尾重Tangent line, 切线Target distribution, 目标分布Taylor series, 泰勒级数Tendency of dispersion, 离散趋势Testing of hypotheses, 假设检验Theoretical frequency, 理论频数Time series, 时间序列Tolerance interval, 容忍区间Tolerance lower limit, 容忍下限Tolerance upper limit, 容忍上限Torsion, 扰率Total sum of square, 总平方和Total variation, 总变异Transformation, 转换Treatment, 处理Trend, 趋势Trend of percentage, 百分比趋势Trial, 试验Trial and error method, 试错法Tuning constant, 细调常数Two sided test, 双向检验Two-stage least squares, 二阶最小平方Two-stage sampling, 二阶段抽样Two-tailed test, 双侧检验Two-way analysis of variance, 双因素方差分析Two-way table, 双向表Type I error, 一类错误/α错误Type II error, 二类错误/β错误UMVU, 方差一致最小无偏估计简称Unbiased estimate, 无偏估计Unconstrained nonlinear regression , 无约束非线性回归Unequal subclass number, 不等次级组含量Ungrouped data, 不分组资料Uniform coordinate, 均匀坐标Uniform distribution, 均匀分布Uniformly minimum variance unbiased estimate, 方差一致最小无偏估计Unit, 单元Unordered categories, 无序分类Upper limit, 上限Upward rank, 升秩Vague concept, 模糊概念Validity, 有效性VARCOMP (Variance component estimation), 方差元素估计Variability, 变异性Variable, 变量Variance, 方差Variation, 变异Varimax orthogonal rotation, 方差最大正交旋转Volume of distribution, 容积W test, W检验Weibull distribution, 威布尔分布Weight, 权数Weighted Chi-square test, 加权卡方检验/Cochran检验Weighted linear regression method, 加权直线回归Weighted mean, 加权平均数Weighted mean square, 加权平均方差Weighted sum of square, 加权平方和Weighting coefficient, 权重系数Weighting method, 加权法W-estimation, W估计量W-estimation of location, 位置W估计量Width, 宽度Wilcoxon paired test, 威斯康星配对法/配对符号秩和检验Wild point, 野点/狂点Wild value, 野值/狂值Winsorized mean, 缩尾均值Withdraw, 失访Youden's index, 尤登指数Z test, Z检验Zero correlation, 零相关Z-transformation, Z变换。
决奈达隆片说明书

- 1、下载文档前请自行甄别文档内容的完整性,平台不提供额外的编辑、内容补充、找答案等附加服务。
- 2、"仅部分预览"的文档,不可在线预览部分如存在完整性等问题,可反馈申请退款(可完整预览的文档不适用该条件!)。
- 3、如文档侵犯您的权益,请联系客服反馈,我们会尽快为您处理(人工客服工作时间:9:00-18:30)。
2
µ The variable xµ i (t) is the world-line coordinate of the i-th particle and pi is its corresponding momentum. The Lagrange multipliers λAij implement the constraints φAij = 0 and satisfy
†
Theory Group, Department of Physics The Univ. of Texas at Austin RLM 5208, Austin, TEXAS
♯
Departament d’Estructura i Constituents de la Mat` eria Universitat de Barcelona Diagonal, 647 E-08028 BARCELONA
λ1ji = λ1ij , The explicit form of φAij is φ1ij = 1 pi pj , 2 φ2ij = pi xj
λ3ji = λ3ij .
and
φ3ij =
1 xi xj . 2
(2.2)
These 2M 2 + M constraints close under Poisson bracket giving a realization of the Sp(2M ) algebra. It is useful to introduce a matrix notation for the coordinates and momenta of the particles r ¯ = p⊤ , −r ⊤ R= , R (2.3) p with x1 . r= . . , xM
the equations of motion (2.9) and transformation properties of the matter fields (2.6) can be written as ¯ − Az (∂ (∂ − Az )R = 0. (2.11) ¯)R = 0, This linear system of partial differential equations has an integrability condition Fz z ¯ = 0, which is equivalent to the transformation law of the Lagrange multipliers (2.7) under the identifications (2.10). These relations will continue to hold when we fix the gauge partially. The above discussion explains why a relativistic particle model becomes after partial gauge-fixing a model of matter with a non-linear W -symmetry. As we will see below it is useful to express the model in terms of lagrangian variables in order to construct the finite transformations of the model. If we write the momenta p in terms of the lagrangian variables p = A−1 (r ˙ − Br ) ≡ K, the action is now rewritten as S= The gauge transformations are δr = Aβ K + Bβ r, ˙ − [Λ, β ]. δΛ = β (2.14) dt 1 K ⊤ AK − r ⊤ Cr . 2 (2.13) (2.12)
1
(2.6) (2.7)
Bβ Aβ ⊤ −Cβ −Bβ
(2.8)
For a previous discussion of geometrical models and Yang-Mills gauge theories see [16].
3
and Aβ , Bβ , Cβ are the M × M matrices gauge parameters associated to the constraints φ1ij , φ2ij , φ3ij . The equations of motion of the matter fields are ˙ − ΛR = 0. R If we make the following identifications ¯ δ → ∂, β → Az ¯, Λ → Az , d → ∂, dt (2.10) (2.9)
In the last few years a lot of attention has been devoted to the study of W -algebras [1]. For recent update reviews see [2], [3] where extensive lists of references can be found. An interesting way to construct classical W -algebras is by the zero-curvature method [4], [5], [6],[7] [8]. If one constraints an Az gauge potential the residual gauge transformations can be obtained as a zero-curvature condition Fz z ¯ = 0. This zero-curvature condition is the integrability condition of a linear system of partial differential equations. As we will see this system can be related to the transformation properties and equations of motion of matter coupled to the gauge fields. In this letter we consider a relativistic model of M particles with an Sp(2M ) gauge group, the matter variables being the coordinates and momenta of the particles and the gauge variables being the Lagrange multipliers. We find that under some formal identifications between 2d gauge theories and 1d particle models, the equations of motion and transformation properties of the matter variables can be written as a system of partial differential equations whose integrability condition is precisely the zero-curvature condition Fz z ¯ = 0. This condition is equivalent to the transformation properties of the Lagrange multipliers. These relations continue to hold when we fix the gauge partially. This fact explains why a model of relativistic particles exhibits, after a partial gaugefixing, invariance under non-linear W -symmetry tranformations. In a sense, it can be understood as a coupling of matter to (world-line) W -gravity. The particle model is also useful for the construction of finite W -transformations. Finite transformations are necessary in order to understand completely the W -geometry [6], [8], [9], [10], [11], [12], [13], [14], [15]. The strategy is the following: we first construct the finite linear transformations of the Sp(2M ) model and then, by a partial gaugefixing at the finite level, we find residual finite W -transformations. In this way one avoids the direct integration of non-linear infinitesimal W -transformations. We will explicitly construct in this paper finite W -transformations obtained from the Sp(4) gauge group.