Edge-choosability and total-choosability of planar graphs with no adjacent 3-cycles
Complex_Dynamical_Systems_Theory
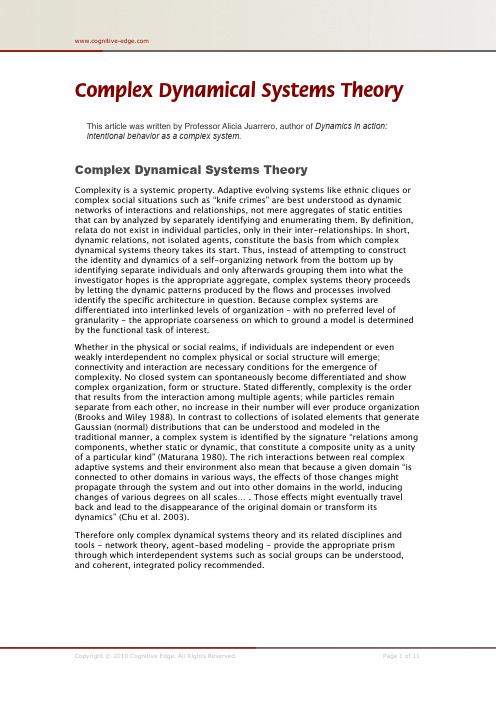
Complex Dynamical Systems Theory This article was written by Professor Alicia Juarrero, author of Dynamics in action:intentional behavior as a complex system.Complex Dynamical Systems TheoryComplexity is a systemic property. Adaptive evolving systems like ethnic cliques or complex social situations such as “knife crimes” are best understood as dynamic networks of interactions and relationships, not mere aggregates of static entities that can by analyzed by separately identifying and enumerating them. By definition, relata do not exist in individual particles, only in their inter-relationships. In short, dynamic relations, not isolated agents, constitute the basis from which complex dynamical systems theory takes its start. Thus, instead of attempting to construct the identity and dynamics of a self-organizing network from the bottom up by identifying separate individuals and only afterwards grouping them into what the investigator hopes is the appropriate aggregate, complex systems theory proceeds by letting the dynamic patterns produced by the flows and processes involved identify the specific architecture in question. Because complex systems aredi ff erentiated into interlinked levels of organization – with no preferred level of granularity - the appropriate coarseness on which to ground a model is determined by the functional task of interest.Whether in the physical or social realms, if individuals are independent or even weakly interdependent no complex physical or social structure will emerge; connectivity and interaction are necessary conditions for the emergence of complexity. No closed system can spontaneously become di ff erentiated and show complex organization, form or structure. Stated di ff erently, complexity is the order that results from the interaction among multiple agents; while particles remain separate from each other, no increase in their number will ever produce organization (Brooks and Wiley 1988). In contrast to collections of isolated elements that generate Gaussian (normal) distributions that can be understood and modeled in the traditional manner, a complex system is identified by the signature “relations among components, whether static or dynamic, that constitute a composite unity as a unity of a particular kind” (Maturana 1980). The rich interactions between real complex adaptive systems and their environment also mean that because a given domain “is connected to other domains in various ways, the e ff ects of those changes might propagate through the system and out into other domains in the world, inducing changes of various degrees on all scales… . Those e ff ects might eventually travel back and lead to the disappearance of the original domain or transform its dynamics” (Chu et al. 2003).Therefore only complex dynamical systems theory and its related disciplines and tools - network theory, agent-based modeling - provide the appropriate prism through which interdependent systems such as social groups can be understood, and coherent, integrated policy recommended.BoundariesA complex dynamical system’s internal structure consists in the patterns that result from particular objects and the interactions among them. But unlike those systems characterized by linear processes that can be e ff ectively isolated from environmental influence, the external structure or boundary conditions of complex systems are as much as part of the complex system as the internal structure; the interactions between the components and the environment, that is, “the set of all [interactions not components of the system] that act or are acted on by components of [the system]” (Bunge 1979) provides the system with a causally e ff ective external structure. Although the environment of interest is thus not the total environment but the environment that a ff ects and is a ff ected by the thing in question, the feedback provides complex systems with a contextual embeddedness that makes the boundaries of complex systems typically fuzzy and di ffi cult to demarcate.From a complexity science point of view, therefore, ethnic cliques and situations such as “knife crime”, understood as dynamic “structures of process,” are not bounded by physical or geographic boundaries. In the case of ethnicity, for example, the dynamic structure of a group no doubt extends spatially into both the group’s diaspora a well as the local communities; insofar as ancient traditions, rites and rituals continue to inform and influence present practices, the dynamical system we identify as an ethnic group also extends back in time to pre-diaspora and tribal culture.The Causality of Complex SystemsThis deep contextual embeddedness of complex systems presents additionaldi ffi culties for researchers: feedback and interactions to/from embedding domains can spread causally (not as e ffi cient causes but as context-sensitive constraints), thereby expanding the domain of the system in question and propagating unforeseen side-e ff ects uncontrollably (Chu et al.). Due to the interactions that constitute them, complex adaptive systems show not only nonlinear e ff ects, but also what is often called causal spread (Wheeler and Clark 1999), a form of causalitydi ff erent from that of the more commonly understood e ffi cient causality.The connectivity and interaction required for complex systems to self-organize, and which provides them with their contextuality and causal e ffi cacy, are best understood in terms of context-sensitive constraints (Juarrero 1999) not classical billiard-ball-like (e ffi cient) causality. First order, context-dependent constraints such as nonlinear interactions like positive feedback loops and catalysts make individuals or particles strongly interdependent by altering their marginal probability. Feedback relations with the environment recalibrate the internal dynamics of complex systems to incoming signals. Doing so embeds the system in its contextual setting by e ff ectively importing the environment into the system’s very dynamical structure. Positive feedback is a temporal context-dependent constraint insofar as it incorporates the past into a system’s present structure. Because the presence of a catalyst changes the probability of a reaction’s occurrence, catalysts also function as contextual constraints insofar as they incorporate the environment into a system’s present structure. Thus individuals or organizations who play the role of social catalysts and serve as media for feedback loops are physical embodiments of bottom-up constraints that link other individuals and organizations together and embed –tightly link—their dynamic organization to its environment and its history such that the newly formed global structure is no longer independent of either.By embodying context-sensitive dependencies, feedback and catalysts are bottom-up constraints that render a system constrained by its own past experience and its environment. Complex dynamical systems thus embody the initial conditions under which they were created; their origin and trajectory constrains their future development and evolution. Because such exquisite sensitivity to initial conditions is one of the hallmarks of complex adaptive systems, these dynamical processes are also essentially historical; in Prigogine’s words, “they carry their history on their backs,” that is, their internal structure reflects their history. Accordingly, self-organizing networks are “path-dependent.” Any methodology that purports to understand a given complex system while at the same time ignoring or not fully understanding either its trajectory or the overall context in which it is embedded is bound to fail. The e ff ects of context-dependent constraints, therefore, are described by conditional, not marginal, probabilities. They are, in other words, functional constraints.Once closure of first-order context sensitive constraints occurs, the resulting global dynamics presents characteristics that aggregates or sums of individuals do not; in technical terms, context-sensitive constraints are enabling constraints insofar as they precipitate the emergence of a global dynamics with an expanded phase space. The dynamic whole has greater degrees of freedom than its components individually – a narrative can tell you more than a Q&A form can. Self-organizing networks described in stories are thus multi-level dynamical systems with emergent properties that are irreducible to their component particles. These characteristics will be ignored and missed if the analytic focus is limited solely to compartmentalized components studied in isolation from each other.Qua emergent wholes, complex systems function as the boundary conditions that actively influence the behavior of their components. Insofar as individuals – children or adults - envision themselves as caught up in a particular narrative structure, we will be able to foresee their constrained behavior. Top down, narratives act as limiting constraints that restrict the degrees of freedom of their components. Whereas from a traditional mechanistic, atomistic point of view such influence was impossible, complex dynamical systems theory allows us to understand such interlevel causal relationships – ubiquitous in social systems - in a scientifically respectable way. In complex adaptive systems, interactions among individuals weave together a story; and once a narrative coalesces in the minds of an individual, or a culture in turn, and as a global system, it actively influences the behavior of the components that make it up. Only complexity science theory provides the tools to understand this kind of bottom-up and top-down causation typical of the collective behavior of human organizations. When combined with narratives as Cognitive-Edge’s SenseMaker® allows, policy makers acquire an indispensible tool with which to map current social patterns and anticipate future trends. Without an appreciation of such global dynamics it is impossible to fully understand the inter-level organizational dynamics of social groups: interacting individuals create stories which then loop back down and alter the behavior of the very individuals that constitute them.Power LawsThe relationship between (on the one hand) the context-sensitive constraints that make complex self organization possible, and the power laws that describe such systems on the other has become clearer thanks to the research of e.g. Barabasi (2002, 2003). Since many complex systems give evidence of the same dynamics atwork on multiple levels of organization (i.e., they tend to be self-similar across levels), scalability is often a central element of complexity science. Through children’s narratives it is therefore possible to capture the dynamics of an overall ethnic or social group. Because power laws are frequently “indicative of correlated, cooperative phenomena between groups of interacting agents” (Cook et al. 2004), students of complex human systems recognize that in lieu of Gaussian statistics, linear regression models, normal distributions, etc., they must model their subject matter using the more unfamiliar tools of organizational dynamics, including Pareto distributions, fractal geometries, and the like. Since extreme cases and situations are much more important than average cases and situations to most students of the human sciences, managers, policy makers, analysts and social scientists ignore power laws (which show fat or long tails, infinite variance, unstable confidence intervals, etc.) at their own peril.Game TheoryApplying game theory to human complex systems, exploring rational choice strategies over time, and investigating the basis of social cooperation, are just a few examples of the increasing pervasiveness of the complexity approach. In each case, the situation is treated as an evolving dynamical system with global properties that emerge from the local interactions among the participants, and between the participants and the context in which they are embedded. Such simulation modeling can capture otherwise intractable nonlinear e ff ects and thereby reveal global patterns that would have been previously out of reach.Once the usefulness of simulation models became clear, the Asian Development Bank, for example, dropped its opposition to a centuries-old management practice when Lansing’s computer model of the complex Balinese irrigation system showed the functional role of traditional water temples bore a “close resemblance to computer simulations of optimal solutions” (Lansing 2000).AttractorsAttractors are typical patterns of dynamical, interdependent behaviors of limited dimensionality and carved out from a much larger space of possible patterns and dimensions. These global structural patterns, which emerge from interactions among the system’s components through phase space, can be characterized as emergent collectives. Social networks can be characterized and studied as attractors.Ergodic behavior patterns describe what are called a system’s attractors. Only two attractors were thought to exist: (1) The dynamics of a grandfather clock’s pendulum describe a point attractor that draws the bob to a single point in phase space regardless of its original position. Equilibrium models assume that all systems they describe are of this sort; traditional economic models were equilibrium models. Not all processes can be understood as near-equilibrium and drawn into a point attractor; ecological research revealed that predator-prey relationships described a di ff erent type of attractor, (2) a periodic attractor. Unlike phenomena characterized by point attractors, predator-prey distribution, for example, typically repeat regularly in a continuous, periodic loop. It was not until the last quarter of the twentieth century that a third type of attractor, so-called strange, chaotic or complex attractors, were discovered: patterns of behavior so convoluted that it is di ffi cult todiscern any order at all; complex human systems can often be characterized as complex attractors, of which social networks are one example.Complex attractors surprised scientists when they discovered that far from being chaotic in the old sense of the word, these complex systems are characterized by a high-dimensional degree of order. Never exactly repeating, the trajectories they trace nevertheless stay within certain bounds. Far from being chaotic in the old sense of the term, these complex behavior patterns provide evidence of highly complex, context-dependent dynamic forms of organization.Attractor LandscapesIn the 1930s biologist Sewall Wright (1932) developed a model of fitness landscapes intended to capture the processes natural selection by visualizing the “switch and trigger mechanisms” that precipitate a change in a system’s evolutionary trajectory. More recently, thanks to the development of computer simulation models, the dependencies and constraints embodied by attractors can also be visualized as three dimensional adaptive landscapes depicting a series of changes in a system’s relative stability and instability over time. The increased probability that a system will occupy a particular state can be represented visually as a landscape’s wells, dips or valleys that embody attractor states and behaviors; the deeper the valley the greater the propensity of its being visited and the stronger the entrainment its attractor represents. In contrast sharp peaks are saddle points representing states and behaviors from which the system shies away. These landscape features capture the impact of context-sensitive constraints over time. The set of all states that end up in a particular attractor constitutes the attractor basin; di ff erent basins are separated from each other by basin boundaries or separatrices. A system’s identity at a particular point in time captures the signature probability distribution of its dynamics – its unique adaptive landscape, so to speak. The most useful image of complex systems is its phase space portrait: its state space carved up into basins of attraction and changing over time.Since all social phenomena are complex systems it becomes extremely important for makers of social policy to be able to map these convoluted relationships as accurately as possible. Doing so allows policy makers to map a situation’s relative volatility, as well as to explore which changes to which parameters will make the situation more or less stable. Complex dynamical mapping of this sort thus provides an invaluable visual aid in phase shift prediction. Although by their very naturecomplex systems resist precise predictability,dynamical landscapes and the mathematicalsoftware that create these visual aids alsoshow decision-makers the range of “adjacentpossible” successor states an unstablesituation is likely to tip into.Dynamic landscapes depicting a series ofchanges of relative stability and instabilityover time provide a very useful way ofvisualizing the contextual and historicalconstraints embodied in the convolutedbehavior patterns described by strangeattractors. By tweaking the various parametersand filters that produce the landscapes,dynamical mapping with SenseMaker®software can provide decision-makers, forexample, with evidence of the presence of “astable pattern overall, except for those groupsthat rank high on the combination of two scales, “retributive justice” and “anger.” These dynamical landscapes also provide evidence of probable and improbable “successor states” to a given situation, information that can be invaluable, for example, for designing a particular governmental advertisement campaigns on crime prevention etc. Dynamical mapping can prove that the intended network is possible, that it can be built; it can also providing guidance on the most appropriate criteria with which to design the most e ff ective network – or disrupt a noxious one. For example, one city’s current landscape might show that it is possible to build a particular network that assists community leaders in precipitating a particular desirable phase change with respect to criminal activity– or, conversely, it can provide decision makers with information that aids and enhances the status quo. Because complex dynamical systems are uniquely individuated, dynamical systems mapping can also provide decision makers with information about whether or not the same advertisement campaign will be as e ff ective in a di ff erent city, or a di ff erent country.If a system could access every alternative with the same frequency as every other – that is, randomly – its landscape would be smooth and flat, portraying an object or a situation with no propensities or dispositions, that is, with no attractors. In contrast, the increased probability that a real system will occupy a particular state can be represented as wells – dips or valleys in a landscape – that embody attractor states and behaviors that the system is more likely to occupy. The deeper the valley the greater the propensity of being visited and the stronger the entrainment of its attractor. Dynamic landscapes thus provide governmental leaders with information about how entrenched a set of attitudes or behavior patterns are, and how best to go about preserving or changing them.Topologically, ridges separating basins of attraction are called separatrices or repellers. Sharp peaks are saddle points representing states and behaviors from which the system shies away and in all likelihood will not access; the probability of their occurrence is low or nonexistent. But if a decision-maker discovers that a system is perched on a saddle point, he can rest assured that it won’t remain in that condition very long. The height of the saddle point separating one attractor from another thus also represents the unlikelihood that the system will switch to another attractor given its history, current dynamics, and the environment. Landscape valleys thus provide decision-makers with a very good indication of whether or not a systemis locked-in to that particular condition, and what the likely “adjacent possibles” might be. The steeper the attractor’s separatrix walls, the greater the improbability of the system’s making the transition. On the other hand, the deeper the valley, the stronger the attractor’s pull, and so the stronger the perturbation that would be needed to dislodge the system from that behavior pattern. Similarly, the broader the floor of a valley the greater the variability in states and behaviors that the attractor allows under its control; conversely, the narrower the valley the more specific the attractor, that is, the fewer the states and behaviors it countenances.Complex systems theory tells us that a landscape’s valleys and peaks are neither static givens nor external control mechanisms through which we can force change. They are not determinants operating as Newtonian forces. Instead they represent constrained pathways that have been constructed and continue to be modified as a result of persistent interactions between the dynamical system and its environment. Landscapes that incorporate dynamics also provide decision makers with information about the likely direction of change, and of the critical parameters that can influence the direction of that change.Co-evolutionPredator-prey relationships taught us that the dynamical landscape of a complex system, to continue with the topographical metaphor, is not fixed. A predator will evolve better eyesight to see its prey, but the prey will evolve a disguise, negating the eyesight advantage. Thus “the landscape peak the predator attempted to climb has moved from under its feet, the fitness peak has shifted, the landscape has deformed due to the changes in the prey. This “coevolution” means that the fitness landscape seen by one creature is a dynamic, ever changing map dependent upon the actions of everything else in its surroundings. This is true for occupants of an ecosystem or a social group. It is a highly non-linear, closely coupled system - attractors that vary in both shape and position over time” (Lucas). Co-evolution with their natural and social environment is even more so of human systems than it is of animals. In the case of human beings we are always referring, therefore, to complex adaptive systems.In other words, since fitness is a relative term (relative to an environmental niche), changes in a (natural, social) niche alter the fitness of the individuals and species within it; in turn, changes in the relative distribution of types of individuals and species within a niche will alter the characteristics of the niche. Thus complex adaptive systems are best characterized as adapting and co-evolving with their environment.Stability versus Resilience: The Importance of Micro-diversityComplex dynamical systems theory explains the di ff erence between stability and resilience. A stable system fluctuates minimally outside its stable attractor, to which it quickly returns when perturbed. Stable systems are typically brittle; they disintegrate if highly stressed. Resilient systems, on the other hand, might fluctuate wildly but have the capacity to modify their structure so as to adapt and evolve. Resilient, robust systems are also called meta-stable. Co-evolution selects for resilience, not stability.Complex adaptive systems are typically resilient. And notoriously robust to random perturbations – but exquisitely vulnerable to targeted interventions, as we will see below.Understanding what causes resilience or robustness is a central issue for analysts and policy makers. For purposes of Cultural Mapping it is particularly important to understand which specific features of the dynamical relationships that make up the knife crime statistics in the city of XYZ make the situation robust or resilient; it is important, that is, to identify the system’s dynamics that allow likely participants to adapt in response to either their own dynamics or perturbations from the outside, and thereby to evolve and persist as a network, despite the removal or incarceration of many of their members. This understanding also points to avenues for intervention by the appropriate authorities. Although still a young science, complex adaptive systems theory has begun to make inroads into understanding (1) the conditions that allow these structures evolve over time in response both to their own internal dynamics and in interaction with the environment; (2) the conditions that facilitate robustness and resilience; and (3) the most e ff ective points of intervention. Jackson & Watts (2002) note that in a network context, path resistance or network resilience is equivalent to “how many errors or mutations are needed to get from some given network to an improving path leading to another network.” Peter Allen defines microdiversity more broadly than simply errors or mutations, as “a measure of the number of qualitatively di ff erent types of entity present corresponding to individuals with di ff erent attributes.” (Garnsey & McGlade 2006, 23). Chu et al. (2003) call such systemic di ff erentiation “inhomogeneity”; they too consider it a hallmark of complexity, as do Carlson & Doyle (2002). In an important article that echoes this general point, the U.S. Naval Academy’s Robert Artigiani demonstrates through two military examples that the best way to deal with unpredictable complex systems is by organizing the system so it is maximally adaptive – when leadership cannot solve the problem in advance because no one knows what the problems will be, it is important to build systems that can solve the problem for themselves. Microdiversity in the sense of internal di ff erentiation is one way to do just that. Allen, who worked extensively with Nobel Laureate Ilya Prigogine in Brussels during the earliest years of this science, has also extensively studied how micro-diversity within a natural or social system drives the qualitative changes that occur in these systems and structures over time. Allen demonstrates that if a particular variation increases an organism’s fitness, natural selection will favor that variation; following the landscape metaphor, evolutionary change – i.e., increased adaptation to the environment - is tantamount to hill-climbing.Allen’s early experiments demonstrated that hill-climbing occurs “as a result of processes of ‘di ff usion’ in character space. Using di ff usion models, Allen’s research also establishes that it is micro-diversity or internal di ff erentiation that confers resilience. Further experiments conducted by Allen and his team at Cranfield University (UK) subsequently confirmed that successful “evolution will be driven by the amount of diversity generation to which it leads. Evolution selects for an appropriate capacity to evolve [more exploration and innovation in novel situations; less exploration in established conditions], and this will be governed by the balance between the costs of experimental ‘failures’… and the improved performance capabilities discovered by the exploration.” The conclusion Allen draws from the research is that “organizations or individuals that can adapt and transform themselves, do so as a result of the generation of micro-diversity and the interactions with micro-contextualities” (emphasis added). The system’s complex regulatory feedback and dynamics also stop cascading failures and enable thesystem to survive (Carlson & Doyle 2002). Incorporating narrative research into dynamical landscapes is a unique and powerful tool to understand and influence social systems.Understanding how information and influence disseminate throughout a social group is a key component that cuts across storylines and issues. To the extent thatdi ff usion processes identify a property that is the inverse of robustness (both pertain to the way influence or information disseminates through, or is blocked, within particular communities by components that are di ff erent – by social mavericks, ine ff ect), identifying features in a social landscape that promote desirable robustness and resilience is a central task of any decision-maker’s mission.Fail-Safe versus Safe-FailThinkers in the field of public policy have traditionally counseled what might be called a fail-safe strategy. From Plato to Marx, the goal was always to design forms of social organization that, because they were ideal, would remain forever in equilibrium. The traditional goal of public policy makers, in other words, has been stability, the minimization of fluctuations. In stark contrast to this approach, ecologist C. S. Holling argues convincingly that if the notion of resilience applies to society at all, it counsels instead a safe-fail strategy that assumes from the outset that failures will occur despite the best-laid plans. A safe-fail strategy is one “that optimizes a cost of failure and even assures that there are periodic ‘minifailures’ to prevent evolution of inflexibility” (Holling 1976; Juarrero-Roque 1991). It is clear that Allen’s thesis - that evolution evolves to maximize evolvability - is another way of making the same point. Social policy should pursue a goal of resilience, not stability. As this new science develops, valuable lessons are derived from studying dynamical landscapes and the networks described in cluster graphs for the way weak ties, high betweenness links, micro-diversity, and other similar features contribute to the robustness and resilience of complex adaptive networks. In turn, these insights are can inform social organization management.。
EDGE应用层以及各项指标计算方法简介

EDGE应用层速率以及RLC层速率计算方法如下:先将测试文件转成FMT格式。
打开tems6.1.4软件,然后在标签栏中选择Logfile中的Export Logfile:点击Export Logfile出现界面后,点击带有+号的Add order ,在Add Export Order界面里对相关的选项进行设定。
在Format 中选择Text file ,在Setup中选择Available IEs项中的Data 里的“App Throughput DL(kbit/s)并添加到右边。
(App Throughput DL为应用层的下载速率)在Available IEs中选择GSM中的RLC Throughput DL(kbit/s)并添加到右边。
(RLC ThroughputDL为RLC的下载速率):按“确定”键后再设定Input files 中的Browse file。
Browse file是指要将转成FMT格式的测试文件。
Browse directory则是指将所转化的文件放于你所指定的文件夹中。
把上述的相关选项设置好后,按下“OK“键。
按下“OK“键后回到Export Logfile界面,按按键。
至此,测试文件转换成FMT格式。
用EXCEL将FMT格式文件打开,将着将下载速率不为零的筛选出来,再求平均值,就是该应用层和RLC层的下载速率。
统计MCS的比例打开tems6.1.4软件,然后在标签栏中选择Logfile中的Export Logfile:点击Export Logfile出现界面后,点击带有+号的Add order ,在Add Export Order界面里Mapinfo Tab-file如下图所示:接着单击SEPUP进入Mapinfo Tap-file的界面如下图所示:接着单击Load选择相应的MEX文件,在右边的边框上会出现你所需要的指标,如下图所示:接着单击确定键。
出现如下图所示的界面:接着单击Browse file选择你要统计的文件,在Browse dir里选择输出结果放的路径,按下“OK“键后回到Export Logfile界面,按按键。
Autodesk Nastran 2023 参考手册说明书
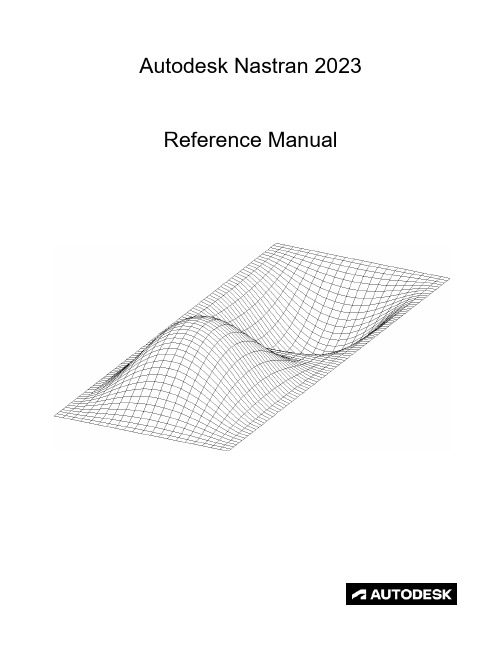
FILESPEC ............................................................................................................................................................ 13
DISPFILE ............................................................................................................................................................. 11
File Management Directives – Output File Specifications: .............................................................................. 5
BULKDATAFILE .................................................................................................................................................... 7
tobit模型的拟合优度

tobit模型的拟合优度以tobit模型的拟合优度为题,进行创作一、什么是tobit模型拟合优度?Tobit模型是一种用于处理存在截断(censored)或右侧截断(right-censored)数据的统计模型。
在某些情况下,我们无法观测到完整的数据,而是只能观测到其上限或下限。
Tobit模型通过最大似然估计来估计模型参数,并评估模型对数据的拟合程度,即拟合优度。
评估tobit模型的拟合优度主要使用两个指标:似然比比较和贝叶斯信息准则(BIC)。
1. 似然比比较:通过比较拟合tobit模型和只包含截断值的模型,计算似然比统计量。
如果统计量显著不为零,即拟合tobit模型比只包含截断值的模型更好,说明tobit模型对数据的拟合程度较好。
2. BIC:贝叶斯信息准则是一种模型选择准则,它考虑了模型的复杂度和拟合优度。
BIC值越小,说明模型对数据的拟合越好。
三、如何提高tobit模型的拟合优度?1. 改进模型:可以尝试不同的变量组合或添加交互项,以提高模型的拟合优度。
此外,还可以考虑使用其他统计模型来拟合数据,例如零膨胀模型或混合效应模型。
2. 增加样本量:增加样本量可以提高模型的拟合优度。
如果可能的话,可以尝试收集更多的数据以提高模型的准确性。
3. 检查模型假设:检查模型的假设是否合理,例如正态分布假设、线性关系假设等。
如果假设不成立,可以考虑使用非参数方法或拟合其他适合的模型。
四、结语Tobit模型的拟合优度是评估模型对数据的拟合程度的重要指标。
通过比较似然比统计量和BIC值,可以评估模型的拟合程度并进行模型选择。
通过改进模型、增加样本量和检查模型假设,可以进一步提高tobit模型的拟合优度。
在实际应用中,我们应该根据具体问题和数据情况选择合适的评估指标和改进方法,以获得更好的拟合结果。
自动定向准则

自动定向准则自动定向准则是指在人工智能和机器学习领域中,利用算法和数据来自动调整和优化模型的参数,以达到最佳的性能和精度。
这些准则通常由专业的数据科学家、机器学习工程师或数据分析师制定,并在模型训练过程中应用。
自动定向准则有以下三个主要方面:1. 超参数调整超参数是指影响模型训练过程的参数,例如学习速率、批量大小、迭代次数等。
这些参数不会随着训练数据的改变而改变,需要手动设置。
自动定向准则可以通过搜索算法来寻找最佳的超参数组合,从而提高模型性能。
2. 模型选择在机器学习中,有多种不同类型的模型可供选择,例如线性回归、决策树、随机森林等。
每种模型都有其独特的优点和缺点。
自动定向准则可以帮助选择最适合特定问题的模型类型,并优化其性能。
3. 特征选择特征是指用于训练模型的输入变量。
在某些情况下,使用所有可用特征可能会导致过拟合或其他问题。
自动定向准则可以帮助确定哪些特征对于模型的性能最重要,并在训练过程中仅使用这些特征。
为了实现自动定向准则,通常需要使用一种称为“网格搜索”的技术。
网格搜索是一种穷尽搜索方法,它尝试所有可能的超参数组合,并选择具有最佳性能的组合。
这种方法虽然可靠,但计算成本非常高,因为它需要测试大量的超参数组合。
另一种更高效的自动定向准则技术是随机搜索。
随机搜索从超参数空间中随机选择一组超参数,并评估其性能。
然后它会继续随机选择并评估其他组合,直到找到最佳的超参数组合或达到预设的时间限制。
除了网格搜索和随机搜索之外,还有其他自动定向准则技术可供选择,例如贝叶斯优化、遗传算法等。
这些技术都有其独特的优点和缺点,并且在不同类型的问题上表现不同。
总之,自动定向准则是一种强大而有效的工具,在人工智能和机器学习领域中得到广泛应用。
通过利用算法和数据来自动优化模型性能,它可以帮助数据科学家和机器学习工程师更快地开发出高性能的模型,并提高他们的工作效率。
找理想工作的英语作文
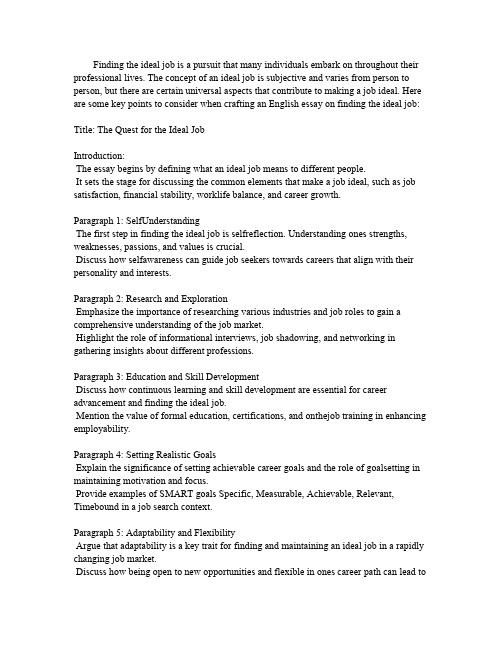
Finding the ideal job is a pursuit that many individuals embark on throughout their professional lives.The concept of an ideal job is subjective and varies from person to person,but there are certain universal aspects that contribute to making a job ideal.Here are some key points to consider when crafting an English essay on finding the ideal job:Title:The Quest for the Ideal JobIntroduction:The essay begins by defining what an ideal job means to different people.It sets the stage for discussing the common elements that make a job ideal,such as job satisfaction,financial stability,worklife balance,and career growth.Paragraph1:SelfUnderstandingThe first step in finding the ideal job is selfreflection.Understanding ones strengths, weaknesses,passions,and values is crucial.Discuss how selfawareness can guide job seekers towards careers that align with their personality and interests.Paragraph2:Research and ExplorationEmphasize the importance of researching various industries and job roles to gain a comprehensive understanding of the job market.Highlight the role of informational interviews,job shadowing,and networking in gathering insights about different professions.Paragraph3:Education and Skill DevelopmentDiscuss how continuous learning and skill development are essential for career advancement and finding the ideal job.Mention the value of formal education,certifications,and onthejob training in enhancing employability.Paragraph4:Setting Realistic GoalsExplain the significance of setting achievable career goals and the role of goalsetting in maintaining motivation and focus.Provide examples of SMART goals Specific,Measurable,Achievable,Relevant, Timebound in a job search context.Paragraph5:Adaptability and FlexibilityArgue that adaptability is a key trait for finding and maintaining an ideal job in a rapidly changing job market.Discuss how being open to new opportunities and flexible in ones career path can lead tounexpected but fulfilling job roles.Paragraph6:The Importance of NetworkingElaborate on the power of networking in uncovering job opportunities that may not be publicly advertised.Describe how building professional relationships can provide support,mentorship,and access to job leads.Paragraph7:WorkLife BalanceDiscuss the importance of finding a job that allows for a healthy balance between work and personal life.Mention how worklife balance contributes to overall wellbeing and job satisfaction.Paragraph8:Persistence and ResilienceEmphasize the need for persistence and resilience in the job search process,especially when facing rejections or setbacks.Provide tips on maintaining a positive attitude and learning from each job search experience.Conclusion:Summarize the key points discussed in the essay.Conclude by reiterating that finding the ideal job is a personal journey that requires selfawareness,continuous learning,and adaptability.Final Thoughts:Encourage readers to stay true to their values and aspirations while pursuing their ideal job.End on a hopeful note,suggesting that with determination and the right approach, anyone can find a job that brings them fulfillment and satisfaction.Remember to use clear,concise language and provide concrete examples to illustrate your points.The essay should be wellstructured,with a logical flow of ideas that guides the reader through your argument.。
从创意到创业智慧树知到答案章节测试2023年湖南师范大学
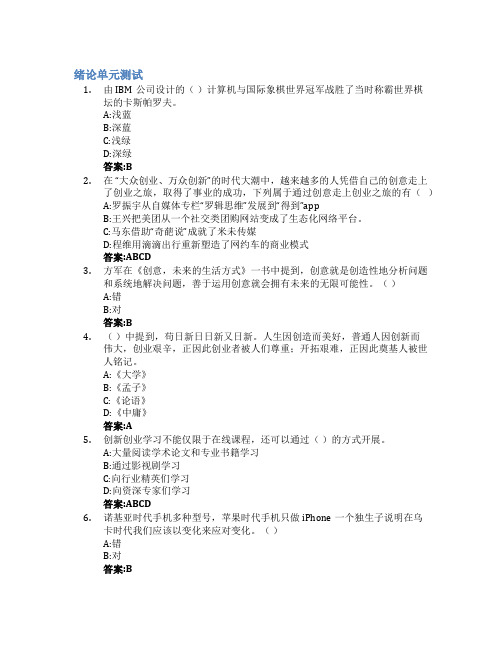
绪论单元测试1.由IBM公司设计的()计算机与国际象棋世界冠军战胜了当时称霸世界棋坛的卡斯帕罗夫。
A:浅蓝B:深蓝C:浅绿D:深绿答案:B2.在“大众创业、万众创新”的时代大潮中,越来越多的人凭借自己的创意走上了创业之旅,取得了事业的成功,下列属于通过创意走上创业之旅的有()A:罗振宇从自媒体专栏“罗辑思维”发展到“得到”appB:王兴把美团从一个社交类团购网站变成了生态化网络平台。
C:马东借助“奇葩说”成就了米未传媒D:程维用滴滴出行重新塑造了网约车的商业模式答案:ABCD3.方军在《创意,未来的生活方式》一书中提到,创意就是创造性地分析问题和系统地解决问题,善于运用创意就会拥有未来的无限可能性。
()A:错B:对答案:B4.()中提到,苟日新日日新又日新。
人生因创造而美好,普通人因创新而伟大,创业艰辛,正因此创业者被人们尊重;开拓艰难,正因此奠基人被世人铭记。
A:《大学》B:《孟子》C:《论语》D:《中庸》答案:A5.创新创业学习不能仅限于在线课程,还可以通过()的方式开展。
A:大量阅读学术论文和专业书籍学习B:通过影视剧学习C:向行业精英们学习D:向资深专家们学习答案:ABCD6.诺基亚时代手机多种型号,苹果时代手机只做iPhone一个独生子说明在乌卡时代我们应该以变化来应对变化。
()A:错B:对答案:B第一章测试1.企业其实是企业家个性的外化,()决定了企业的发展方向。
()A:创业者对自身的了解B:创业者的资本C:创业者的心态D:创业者的目标答案:A2.创业的三把钥匙包括()A:“你能做什么”B:“你是谁”C:“你到哪里去”D:“你从哪里来”答案:BCD3.经济上的成功是创业的原因,但绝不是创业的结果。
()A:对B:错答案:B4.饿了么曾推出过以下哪些项目()A:蜂鸟系统B:未来餐厅C:火箭系统D:风车系统答案:AB5.大学生创业热情高、社会经验少,很难一次性取得成功或者取得大得成功。
()A:错B:对答案:B6.()决定了创业发展方向和关键时候的决策方式()A:个人喜好B:创新思维C:价值观和目标D:认知方式答案:C7.创业者可以从同行身上获得灵感,这是()型的创业。
决策树算法原理(三种最优属性划分方法)
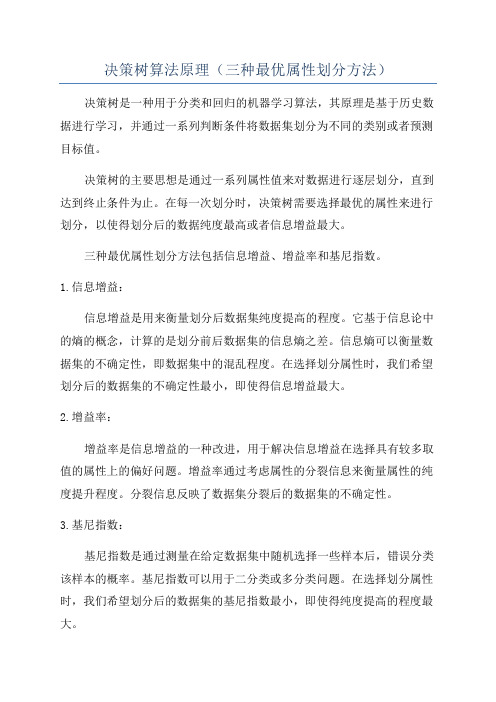
决策树算法原理(三种最优属性划分方法)决策树是一种用于分类和回归的机器学习算法,其原理是基于历史数据进行学习,并通过一系列判断条件将数据集划分为不同的类别或者预测目标值。
决策树的主要思想是通过一系列属性值来对数据进行逐层划分,直到达到终止条件为止。
在每一次划分时,决策树需要选择最优的属性来进行划分,以使得划分后的数据纯度最高或者信息增益最大。
三种最优属性划分方法包括信息增益、增益率和基尼指数。
1.信息增益:信息增益是用来衡量划分后数据集纯度提高的程度。
它基于信息论中的熵的概念,计算的是划分前后数据集的信息熵之差。
信息熵可以衡量数据集的不确定性,即数据集中的混乱程度。
在选择划分属性时,我们希望划分后的数据集的不确定性最小,即使得信息增益最大。
2.增益率:增益率是信息增益的一种改进,用于解决信息增益在选择具有较多取值的属性上的偏好问题。
增益率通过考虑属性的分裂信息来衡量属性的纯度提升程度。
分裂信息反映了数据集分裂后的数据集的不确定性。
3.基尼指数:基尼指数是通过测量在给定数据集中随机选择一些样本后,错误分类该样本的概率。
基尼指数可以用于二分类或多分类问题。
在选择划分属性时,我们希望划分后的数据集的基尼指数最小,即使得纯度提高的程度最大。
以上三种最优属性划分方法在决策树学习的过程中都有着重要的应用。
在实际应用中,决策树算法可以通过先验知识或者经验来选择最优属性划分方法。
此外,由于决策树算法在高维数据上容易过拟合的问题,可以通过剪枝等技术来避免过拟合。
决策树算法的优势在于易于理解和解释,但也存在局限性,比如对于处理缺失数据、处理连续数据和处理类别不平衡等问题仍然存在挑战。
- 1、下载文档前请自行甄别文档内容的完整性,平台不提供额外的编辑、内容补充、找答案等附加服务。
- 2、"仅部分预览"的文档,不可在线预览部分如存在完整性等问题,可反馈申请退款(可完整预览的文档不适用该条件!)。
- 3、如文档侵犯您的权益,请联系客服反馈,我们会尽快为您处理(人工客服工作时间:9:00-18:30)。
a r X i v :m a t h /0512518v 2 [m a t h .C O ] 21 J u n 2008Edge-choosability and total-choosability ofplanar graphs with no adjacent 3-cyclesDaniel W.Cranston ∗dcransto@ June 21,2008Abstract Let G be a planar graph with no two 3-cycles sharing an edge.We show that if ∆(G )≥9,then χ′l (G )=∆(G )and χ′′l (G )=∆(G )+1.We also show that if ∆(G )≥6,then χ′l (G )≤∆(G )+1and if ∆(G )≥7,then χ′′l (G )≤∆(G )+2.All of these results extend to graphs in the projective plane and when ∆(G )≥7the results also extend to graphs in the torus and Klein bottle.This second edge-choosability result improves on work of Wang and Lih and of Zhang and Wu.All of our results use the discharging method to prove structural lemmas about the existence of subgraphs with small degree-sum.For example,we prove that if G is a planar graph with no two 3-cycles sharing an edge and with ∆(G )≥7,then G has an edge uv with d (u )≤4and d (u )+d (v )≤∆(G )+2.All of our proofs yield linear-time algorithms that produce the desired colorings.MSC :05C15,05C10Keywords :List coloring,edge coloring,total coloring,Vizing’s Conjecture 1Introduction All our graphs are finite and without loops or multiple edges.Let G be a plane graph.We use E (G ),V (G ),F (G ),∆(G ),and δ(G )to denote the edge set,vertex set,face set,maximum degree,and minimum degree of G ,respectively.When the graph is clear from context,we use ∆,rather than ∆(G ).We use “j -face”and “j -vertex”to mean faces and vertices of degree j .The degree of a face f is the number of edges along the boundary of f ,with each cut-edge being counted twice.The degree of a face f and the degree of a vertex v are denoted by d (f )and d (v ).We say a face f or vertex v is large when d (f )≥5or d (v )≥5.We use triangle to mean 3-cycle.We use kite to mean a subgraph of G formed by two 3-cycles that share an edge.We use element to mean vertex or face.A proper total-coloring of G is an assignment of a label to each element so that no two incident oradjacent elements receive the same label.We call these labels colors .A proper k -total-coloring is a proper total-coloring that uses no more than k colors.A total assignment L is a function on E (G )∪V (G )that assigns each element x a list L (x )of colors available for use on that element.An L -total-coloring is a proper total-coloring with the additional constraint that each element receives a color appearing in its assigned list.We say that a graph G is k -total-choosable if G has a proper L -total-coloring whenever |L (x )|≥k for every x ∈E (G )∪V (G ).The total chromatic number of G ,denoted χ′′(G ),is the least integer k such that G is k -total-colorable.The list total chromatic number of G ,denoted χ′′l (G ),is the least integer k such that G is k -total-choosable.In particular,note that χ′′(G )≤χ′′l (G ).The list edge chromatic number χ′l (G )is defined similarly in terms of coloring only edges;the ordinary edge chromatic number is denoted χ′(G ).Probably the most fundamental and important result about the edge chromatic number of graphs is:Theorem1.(Vizing’s Theorem;Vizing[18,19]and Gupta[8])χ′(G)≤∆(G)+1.Vizing conjectured that Theorem1could be strengthened by proving the same bound for the list edge chromatic number:Conjecture2.(Vizing’s Conjecture;see[12])χ′l(G)≤∆(G)+1.The most famous open problem about list edge-coloring is the List Coloring Conjecture.Bollob´a s and Harris[2]believed that Vizing’s conjecture could be further strengthened to give:Conjecture3.(List Coloring Conjecture;Bollob´a s and Harris[2])χ′l(G)=χ′(G).We give a brief summary of previous results on list edge-coloring;for a more thorough treatment,we recommend Graph Coloring Problems[10].Borodin,Kostochka,and Woodall[6]proved that the List Coloring Conjecture holds for planar graphs with∆≥12.Vizing’s Conjecture is easy to prove when∆≤2. Harris[9]and Juvan et al.[11]confirmed the conjecture when∆=3and∆=4,respectively.Borodin proved Vizing’s Conjecture for planar graphs with∆≥9[3].Wang and Lih[20]proved that Vizing’s Conjecture holds for a planar graph G when∆≥6and G has no two triangles sharing a vertex.Zhang and Wu[22] proved that Vizing’s Conjecture holds for a planar graph G when∆≥6and G has no4-cycles.We improve these results in several ways.In Section2,we prove structural results for use in Section4 and Section5,where we prove our main results.For simplicity,we state each of our results only for planar graphs.However,in Section3we show that each result can be extended to the projective plane and that most of the results can also be extended to the torus and Klein bottle.In Section4,we show that Vizing’s Conjecture holds for a planar graph that contains no kites and has∆≥6.This is a strengthening of the result of Wang and Lih[20]and the result of Zhang and Wu[22].We also show that the List Coloring Conjecture holds for a planar graph that contains no kites and has∆≥9.In Section5we prove results about list total coloring,which we describe below.Less is known about the total chromatic number than the edge chromatic number.Vizing and Behzad conjectured an analogue to Vizing’s Theorem:Conjecture4.(Total Coloring Conjecture;Vizing[18]and Behzad[1])χ′′(G)≤∆(G)+2.The Total Coloring Conjecture was proved for∆=3by Rosenfeld[16]and also by Vijayaditya[17]. For∆=4and∆=5it was proved by Kostochka[13,14,15].For planar graphs,much more is known. Borodin[4]proved the Total Coloring Conjecture for∆≥9.Yap[21,10]observed that the cases of the Total Coloring Conjecture when∆=7or∆=8follow from a short argument that uses the4-Color Theorem and the fact thatχ′(G)=∆for planar graphs when∆≥7.Borodin,Kostochka,and Woodall[6]showed that χ′′(G)=∆+1for∆≥12.The list total chromatic number seems to have been relatively unstudied until Borodin,Kostochka,and Woodall conjectured the following:Conjecture5.(Total List Coloring Conjecture;Borodin,Kostochka,Woodall[6])χ′′l(G)=χ′′(G).For a planar graph with∆(G)≥12,they showed the stronger resultχ′′l(G)=χ′′(G)=∆+1.We note that Borodin’s proof of the Total Coloring Conjecture for planar graphs with∆(G)≥9in fact shows that χ′′l(G)≤∆+2.Almost all of our proofs forχ′l(G)can easily be adapted to give results forχ′′l(G).Again,we consider planar graphs with no kites.In Section5,we show that if∆≥9,thenχ′′l(G)=∆+1.We also show that if∆≥7,thenχ′′l(G)≤∆+2.22Structure of planar graphs with no triangles sharing an edge Before we prove structural results about planar graphs with no kites,we mention a theorem of O.V.Borodin. Theorem6.(Borodin[5])Let G be a plane graph with no kites.The following statements are valid and all numerical parameters are best possible.(i)δ(G)≤4;(ii)Ifδ(G)≥3,then there are adjacent vertices x,y such that d(x)+d(y)≤9;(iii)ifδ(G)≥3,then there is either an i-face where4≤i≤9or10-face incident with ten3-vertices and adjacent tofive triangles.We will not directly use Theorem6,although part(ii)is very similar to some of our lemmas.Theorem7.If graph G is planar,G contains no kites,and G has∆≥7,then G has an edge uv with d(u)≤4and d(u)+d(v)≤∆+2.Proof:Assume G is a counterexample.Clearly,δ(G)≥3;furthermore,each3-vertex is only adjacent to ∆-vertices.We use a discharging argument.We assign to each element x an initial chargeµ(x)=d(x)−4. The initial charges have sum x∈V∪F(d(x)−4)=−8.We use the following two discharging rules,applied simultaneously at all vertices and faces in a single discharging phase:(R1)Each large vertex gives a charge of1/2to each incident triangle.(R2)Each∆-vertex gives a charge of1/3to each adjacent3-vertex.To reach a contradiciton,we show that for every element the new chargeµ∗is nonnegative.Consider an arbitrary face f.•If d(f)=3,then at least two of the vertices incident to f are large;otherwise,we have d(u)+d(v)≤4+4≤∆+2.Thusµ∗(f)≥−1+2(1/2)=0.•If d(f)≥4,thenµ∗(f)=µ(f)≥0.Consider an arbitrary vertex v.•If d(v)=3,thenµ∗(v)=−1+3(1/3)=0,since each neighbor of v is a∆-vertex.•If d(v)=4,thenµ∗(v)=µ(v)=0.•If d(v)=5,then v is incident to at most2triangles,soµ∗(v)≥1−2(1/2)=0.•If6≤d(v)≤∆−1,then v is incident to at most d(v)/2triangles.Thusµ∗(v)≥d(v)−4−d(v)2=3d(v)2)−(d(v)−t)(13−4−t2,we getµ∗(v)≥7d(v)(i)G has an edge uv with d(u)+d(v)≤8.(ii)G has a4-face uvwx with d(u)=d(w)=3.(iii)G has a6-vertex incident to three triangles;two of these triangles are of type(6,6,3)and the third is of type(6,6,3),(6,5,4),or(6,6,4).Proof:Assume G is a counterexample.For every edge uv,G must have d(u)+d(v)≥9.Thus,δ(G)≥3. We use a discharging argument.We assign to each element x an initial chargeµ(x)=d(x)−4.We use the following three discharging rules:(R1)Each large face f gives a charge of1/2to each incident3-vertex.(R2)Each5-vertex v gives a charge of1/2to each incident triangle.(R3)Each6-vertex v•gives a charge of1/3to each adjacent3-vertex that is not incident to any large face.•gives a charge of1/6to each adjacent3-vertex that is incident to a large face.•gives a charge of1/2to each incident triangle that is incident to a3-vertex or a4-vertex.•gives a charge of1/3to each incident triangle that is not incident to a3-vertex or a4-vertex.Now we show that for every element the new chargeµ∗is nonnegative.Consider an arbitrary face f.•If d(f)=3,then we consider two cases.If f is incident to a3-vertex or a4-vertex,thenµ∗(f)=−1+2(1/2)=0.If f is not incident to a3-vertex or a4-vertex,thenµ∗(f)≥−1+3(1/3)=0.•If d(f)=4,thenµ∗(f)=µ(f)=0.•If d(f)=5,thenµ∗(f)≥1−2(1/2)=0.•If d(f)≥6,thenµ∗(f)≥d(f)−4−d(f)=3d(f)2◦If v is incident to three triangles,then we consider two cases.If at most one of the triangles istype(6,6,3),thenµ∗(v)≥2−3(1/2)−1/3>0.Furthermore,if two of the triangles incident tov are type(6,6,3)but the third triangle is not incident to any vertex of degree at most4,thenµ∗(v)=2−2(1/2)−2(1/3)−1(1/3)=0.If two of the triangles are of type(6,6,3)and thethird triangle is incident to a vertex of degree at most4,then condition(iii)of the lemma holds.We will apply Theorem7and Lemma8to get ourfirst result about edge-choosability.To prove the (∆+1)-edge-choosability of a planar graph G that has∆≥6and that contains no kites,we remove one or more edges of G,inductively color the resulting subgraph,then extend the coloring to G.Intuitively, Theorem7and Lemma8do the“hard work.”However,it is still convenient to prove the following lemma, which we will apply to the subgraphs of G that arise from this process.Lemma9.Let G be a planar graph that contains no kites.If∆≤5,then G has an edge uv with d(u)+d(v)≤8.If∆=6,then G has an edge uv with d(u)+d(v)≤9.Proof:If∆≤4,then each edge uv satisfies d(u)+d(v)≤2∆≤8.In that case,the lemma holds trivially.If∆=6,then the result follows from(ii)of Theorem6.So we must prove the lemma for the case ∆=5.We use a discharging argument.Assume G is a counterexample.For every edge uv,G must have d(u)+d(v)≥∆+4.Thus,δ(G)≥4.We assign to each element x an initial chargeµ(x)=d(x)−4.We use a single discharging rule:(R1)Every large vertex v gives a charge of1/2to each incident triangle.Now we show that for every element the new chargeµ∗is nonnegative.Consider an arbitrary face f.•If d(f)=3,then f is incident to at least two large vertices,soµ∗(f)≥−1+2(1/2)=0.•If d(f)≥4,thenµ∗(f)=µ(f)≥0.Consider an arbitrary vertex v.•If d(v)=4,thenµ∗(v)=µ(v)=0.•If d(v)=5,thenµ∗(v)≥1−2(1/2)=0. Note that the case when∆=6in Lemma9follows easily from(ii)in Theorem6;however,we reproved it above because in Section3we will adapt the proof of Lemma9to the projective plane,torus,and Klein bottle.Before we state the next theorem,we need a new definition.A k-alternating cycle is an even cycle v1w1v2w2...v l w l with d(w i)=k.This definition was introduced by Borodin in1989[4].Since then,it has been used to prove many coloring results(for example,[3]).Theorem10.If graph G is planar,G contains no kites,and∆≥9,then at least one of the following two conditions holds:(i)G has an edge uv with d(u)≤4and d(u)+d(v)≤∆+1.(ii)G has a2-alternating cycle v1w1v2w2...v k w k.Proof:Assume G is a counterexample.Clearly,δ(G)≥2.Our proof will use a discharging argument,but first we show that if G is a counterexample to Theorem10,then G has more∆-vertices than2-vertices.Let H be the subgraph of G formed by all edges with one endpoint of degree2and the other endpoint of degree∆.Form H from H by contracting one of the two edges incident to each vertex of degree2(recall that each neighbor of a2-vertex in G is a∆-vertex).Each2-vertex in G corresponds to an edge in H5and each vertex in H corresponds to a∆-vertex in G.So G has more∆-vertices than2-vertices unless |E( H)|≥|V( H)|.If|E( H)|≥|V( H)|,then H contains a cycle.However,a cycle in H corresponds to a2-alternating cycle in G.Such a cycle in G satisfies condition(ii)and shows that G is not a counterexample to Theorem10. So,G has more∆-vertices than2-vertices.We assign to each element x an initial chargeµ(x)=d(x)−4.In addition to the vertices and edges,we create a bank that can give and receive charge.The bank has initial charge0.As with the vertices and edges, we must verify that thefinal charge of the bank is nonnegative.We use the following three discharging rules: (R1)Each∆-vertex and(∆−1)-vertex v gives a charge of1/3to each adjacent2-vertex or3-vertex.(R2)Each large vertex v gives a charge of1/2to each incident triangle.(R3)Each∆-vertex gives a charge of4/3to the bank.Each2-vertex takes a charge of4/3from the bank.The only rule that effects the bank’s charge is(R3).Since G has more∆-vertices than2-vertices,the bank’s final charge is positve.Now we show that for every element the new chargeµ∗is nonnegative.Consider an arbitrary face f.•If d(f)=3,then since at least one endpoint of each edge is large,at least two of the vertices incident to f are large.Thusµ∗(f)≥−1+2(1/2)=0.•If d(f)≥4,thenµ∗(f)=µ(f)≥0.Consider an arbitrary vertex v.•If d(v)=2,thenµ∗(v)=−2+2(1/3)+4/3=0.•If d(v)=3,thenµ∗(v)=−1+3(1/3)=0.•If d(v)=4,thenµ∗(v)=µ(v)=0.•If d(v)=5,then v is incident to at most2triangles,soµ∗(v)≥1−2(1/2)=0.•If6≤d(v)≤∆−2,then v is incident to at most d(v)2(1 4−4>0.•If d(v)=∆−1,then let t be the number of triangles incident to v.For each triangle incident to v, at most one of the vertices of that triangle has degree3.Thus,if v is incident to t triangles,thenµ∗(v)≥d(v)−4−t(13)=2d(v)6.Since t≤d(v)12d(v)−4.This expression is positive when d(v)≥8.•If d(v)=∆,then let t be the number of triangles incident to v.For each triangle incident to v, at most one of the vertices of that triangle has degree3.Thus,if v is incident to t triangles,thenµ∗(v)≥d(v)−4−42)−(d(v)−t)(13−166.Since t≤⌊d(v)positive charge.In Theorem10,the bank has positive charge.In Lemma9,when∆=6,each vertex of degree∆has positive charge.Since we cannot prove Lemma9for the torus and the Klein bottle when ∆=5,we prove a weaker result:Lemma11.Let G be a graph with no kites that is embedded in a surface with nonnegative Euler charac-teristic.If∆≤6,then G has an edge uv with d(u)+d(v)≤9.Proof:If∆≤4,the result holds,since4+4<9.If∆∈{5,6},we use the same discharging argument as in Lemma6.We have already shown thatµ∗(x)≥0for each element x;now we must show there exists some element x such thatµ∗(x)>0.If∆=6,then each vertex v of degree6hasµ∗(v)>0.Suppose∆=5.If the lemma fails,then G must be5-regular.If G contains no triangles,then each5-vertex has positive charge;if G contains a triangle f, then since G is5-regular,µ∗(f)=−1+3(1/2)=1/2>0.We now have the necessary tools to prove our main results.In the next section we prove two theorems about edge-choosability.In the following section we prove two theorems about total-choosability.For simplicity,we state these theorems only for planar graphs,but after each proof we note which results hold for other surfaces.4Application to Edge-ChoosabilityTheorem12.Let G be a planar graph that contains no kites.If∆=5,thenχ′l (G)≤∆+1.If∆=5,thenχ′l(G)≤∆+2.Proof:Let G be a connected graph.Harris[9]and Juvan et al.[11]showed that G is(∆+1)-edge-choosable when∆=3and∆=4,respectively(even for nonplanar graphs).Thus,we only need to prove the theorem when∆≥5.We consider separately the three cases∆=5,∆=6,and∆≥7.In each case we proceed by induction on the number of edges.The theorem holds trivially if|E(G)|≤7.Note that if d(u)+d(v)≤k, then edge uv is adjacent to at most k−2other edges.We use this fact frequently in the proof.Suppose∆(G)=5.Let H be a subgraph of G.Since∆(H)≤5,Lemma9implies that H has an edge uv with d(u)+d(v)≤8.By hypothesis,χ′l(H−uv)≤7.Since edge uv is adjacent to at most six edges in H,we can extend the coloring to edge uv.Suppose∆(G)≥7.Let H be a subgraph of G.Since∆(H)≤∆(G),Theorem7and Lemma9together imply that H has an edge uv with d(u)+d(v)≤∆(G)+2.By hypothesis,χ′l(H−uv)≤∆(G)+1.Since edge uv is adjacent to at most∆(G)edges in H,we can extend the coloring to edge uv.Suppose∆(G)=6.Let H be a subgraph of G.By Lemmas8and9,we know that one of the three conditions from Lemma8holds for H.We show that in each case we can remove some set of edges E, inductively color the graph H− E,then extend the coloring to E.(i)If H has an edge uv with d(u)+d(v)≤8,then by hypothesisχ′l(H−uv)≤7.Since at most6colors are prohibited from use on uv,we can extend the coloring to uv.(ii)If H has a4-face uvwx with d(u)=d(w)=3,then let C={uv,vw,wx,xu}.By hypothesis χ′l(H−C)≤7.Since each of the four uncolored edges of C has at most5colors prohibited,there are at least two colors available to use on each edge of C.Sinceχ′l(C)=2,we can extend the coloring to C.(It is well-known for every even cycle C thatχ′l(C)=2;for example,this was shown by Erd˝o s,Rubin,and Taylor[7].)(iii)If G has a6-vertex incident to3triangles,two of type(6,6,3)and the third of type(6,6,3),(6,5,4), or(6,6,4),then we assume the third triangle is type(6,6,4),since this is the most restrictive case.LetˆE be the set of edges of all three triangles,plus one additional edge incident to a vertex of degree3in one of the triangles.By hypothesis,χ′l(G− E)≤7.We show that we can extend the coloring to E.The ten edges of E are shown in Figure1,along with the number of colors available to use on each edge. We use L(e)to denote the list of colors available for use on edge e after we have chosen colors for all the7••••••••a |3b |2c |6d |3e |1f |5g |3h |3i |7j |263646366Figure 1.The ten remaining uncolored edges.The number at each vertex is the degree of that vertex in G .The number on each edge is the number of colors available to use on that edge after we have chosen colors for all edges not pictured.edges not shown in Figure 1.Since |L (g )|+|L (j )|>|L (h )|,either there exists some color α∈L (g )∩L (j )or there exists some color α∈(L (g )∪L (j ))\L (h ).If α∈L (g )∩L (j ),we use color αon edges g and j .Otherwise there exists α∈( L (g )∪L (j ))\L (h ).In this case,use color αon g or j ,then use some other available color on whichever of g and j is uncolored.In either case,we can now color the rest of the edges in the order:e,d,a,b,f,c,i,h .This completes the proof for the case ∆(G )=6. The results in Theorem 12easily extend to the projective plane for all values of ∆;they also extend to the torus and Klein bottle when ∆≥7.Theorem 13.If G is planar,G contains no kites,and ∆(G )≥9,then χ′l (G )=∆(G ).Proof:Since edges with a common endpoint must receive distinct colors,χ′l (G )≥∆(G ).So we need to prove that χ′l (G )≤∆(G ).By induction on the number of edges,we prove that if H is a subgraph of G ,then χ′l (H )≤∆(G ).Our base case is when ∆(H )≤8.The result holds for the base case by Theorem 12.Assume that ∆(H )≥9.By Theorem 10at least one of the following two conditions holds:(i)H has an edge uv with d (u )+d (v )≤∆(H )+1.(ii)H has a 2-alternating cycle.Suppose condition (i)holds.By hypothesis,χ′l (H −uv )≤∆(G ).Since d (u )+d (v )≤∆(H )+1≤∆(G )+1,we have at least one color available to extend the coloring to uv .Suppose condition (ii)holds.Let C be the even cycle.By hypothesis,χ′l (H −C )≤∆(G ).After coloring H −C ,each edge of C has at least two colors available.Since even cycles are 2-choosable,we can extend the coloring to C .Theorem 13easily extends to the projective plane,Klein bottle,and torus.5Application to Total-ChoosabilityTheorem 14.If G is a planar graph with no kites and ∆≥7,then χ′′l (G )≤∆+2.Proof:Our proof is by induction on the number of edges in G .(Our base case is all planar graphs with at most 4edges.)By Theorem 4and Lemma 8,every subgraph of G contains an edge uv with d (u )≤4and d (u )+d (v )≤∆+2.By induction,we have a total coloring for G −uv .Now we uncolor u ;since edge uv is adjacent to at most ∆colored edges and one colored vertex,we can color uv .Since d (u )≤4,u is incident and adjacent to at most 2d (u )≤8colored elements;thus,we can color u .8Theorem14extends to the projective plane,Klein bottle,and torus.(G)=∆+1.Theorem15.If G is a planar graph with no kites and∆≥9,thenχ′′lProof:Our proof is by induction on the number of edges in G.Our base case is all subgraphs H of G with ∆(H)∈{7,8}.By Theorem14,each subgraph H satisfiesχ′′l(H)≤∆(H)+2≤10≤∆(G)+1.Our induction step is as follows.By Lemma10,every subgraph H of G with∆(H)≥9satisfies one of the following two conditions:(i)H has an edge uv with d(u)≤4and d(u)+d(v)≤∆+1or(ii)H has a 2-alternating cycle.Suppose that H satisfies(i).By the induction hypothesis,we have a total coloring of H−uv.To extend this coloring to uv,uncolor vertex u;since edge uv is adjacent to at most∆(H)−1colored edges and one colored vertex,we can extend the coloring to uv.Finally,since vertex u is adjacent and incident to at most 2d H(u)≤8colored elements,we can color u.Suppose instead that H satisfies(ii).Let C denote the2-alternating cycle v1w1v2w2...v k w k.By the induction hypothesis,we have a total coloring of H−E(C).To extend the coloring to E(C),wefirst uncolor each2-vertex w i on C.Now each edge in E(C)has at least two colors available.Since even cycles are 2-choosable(and equivalently2-(edge-choosable)),we can color the edges of C.Finally,we can color each 2-vertex w i,since each such vertex is incident and adjacent to only4colored elements.Theorem15easily extends to the projective plane,Klein bottle,and torus.6AcknowledgementsDoug West clarified the arguments and improved the exposition.David Bunde offered many suggestions that improved the exposition.Oleg Borodin taught a lecture course on discharging at UIUC during the Spring 2005semester.Without that course,this paper would not have been possible.Thanks to an anonymous referee,who provided two very useful references.Thanks most of all to my Lord and Savior,Jesus Christ.References[1]M.Behzad,Graphs and their chromatic numbers,Ph.D.thesis,Michigan State University,1965.[2]B.Bollab´a s and A.J.Harris,List-colorings of graphs,Graphs Combin.,1(1985)115–127.[3]O.V.Borodin,Generalization of a theorem of Kotzig and a prescribed coloring of the edges of planargraphs,Mathematical Notes of the Academy of Sciences of the USSR,48(1990)1186–1190.[4]O.V.Borodin,On the total coloring of planar graphs,J.reine angew.Math.,394(1989)180–185.[5]O.V.Borodin,Structural properties of plane graphs without adjacent triangles and an application to3-colorings,J.Graph Theory,21(1996)183–186.[6]O.V.Borodin and A.V.Kostochka and D.R.Woodall,List Edge and List Total Colourings of Multi-graphs,b.Theory B,71(1997)184–204.[7]P.Erd˝o s,A.L.Rubin,and H.Taylor,Choosability in graphs,Proceedings of the West Coast Conferenceon Combinatorics,Graph Theory and Computing,Arcata,California,1979,Congressus Numeratium, 26(1980)125–157.[8]R.P.Gupta,The chromatic index and the degree of a graph(Abstract66T-429),Notices Amer.Math.Soc.,13(1966)719.[9]A.J.Harris,Problems and conjectures in extremal graph theory,Ph.D.Dissertation,Cambridge Univer-sity,UK,1984.9[10]T.R.Jensen and B.Toft,Graph Coloring Problems.John Wiley&Sons,1995.[11]M.Juvan,B.Mohar,and R.Skrekovski,Graphs of degree4are5-edge-choosable,J.Graph Theory,32(1999)250–264.[12]A.V.Kostochka,List edge chromatic number of graphs with large girth,Discrete Math.,101(1992)189–201.[13]A.V.Kostochka,The total coloring of a multigraph with maximal degree4,Discrete Math.,17(1977)161–163.[14]A.V.Kostochka,The total chromatic number of a multigraph with maximal degree5is at most7,Discrete Math.,162(1996)199–214.[15]A.V.Kostochka,Exact upper bound for the total chromatic number of a graph,Proc.24th InternationalWiss.Koll.,Tech.Hochsch.Ilmenau,(1979)33–36.[in Russian][16]M.Rosenfeld,On the total coloring of certain graphs,Israel J.Math.,9(1971)396–402.[17]N.Vijayaditya,On total chromatic number of a graph,J.London Math.Soc.,3(1971)405–408.[18]V.G.Vizing,On an estimate of the chromatic class of a p-graph,Diskret.Analiz.,3(1964)25–30.[19]V.G.Vizing,Critical graphs with a given chromatic class.,Diskret.Analiz.,5(1965)9–17.[20]W.Wang and K.Lih,Choosability and edge choosability of planar graphs without intersecting triangles,SIAM J.Discrete Math.15(2002)538–545.[21]H.P.Yap,Total-colourings of graphs,Manuscript,1989.[22]L.Zhang and B.Wu,Edge choosability of planar graphs without small cycles,Discrete Math.,283(2004)289–293.10。