Lecture02 人工神经网络用于石油工程
人工智能在石油工程领域应用及影响
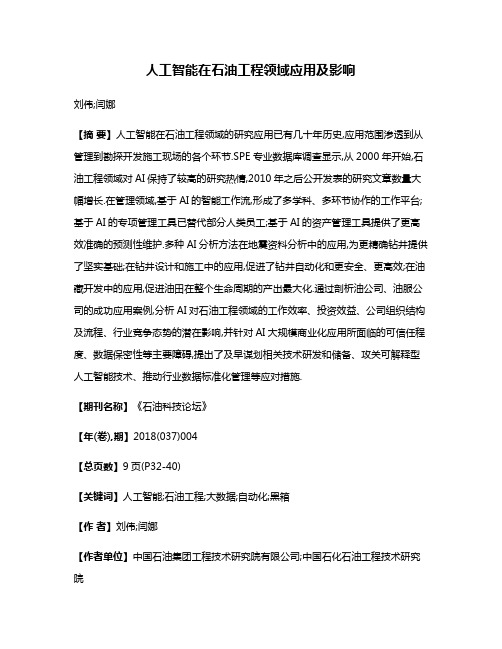
人工智能在石油工程领域应用及影响刘伟;闫娜【摘要】人工智能在石油工程领域的研究应用已有几十年历史,应用范围渗透到从管理到勘探开发施工现场的各个环节.SPE专业数据库调查显示,从2000年开始,石油工程领域对AI保持了较高的研究热情,2010年之后公开发表的研究文章数量大幅增长.在管理领域,基于AI的智能工作流,形成了多学科、多环节协作的工作平台;基于AI的专项管理工具已替代部分人类员工;基于AI的资产管理工具提供了更高效准确的预测性维护.多种AI分析方法在地震资料分析中的应用,为更精确钻井提供了坚实基础;在钻井设计和施工中的应用,促进了钻井自动化和更安全、更高效;在油藏开发中的应用,促进油田在整个生命周期的产出最大化.通过剖析油公司、油服公司的成功应用案例,分析AI对石油工程领域的工作效率、投资效益、公司组织结构及流程、行业竞争态势的潜在影响,并针对AI大规模商业化应用所面临的可信任程度、数据保密性等主要障碍,提出了及早谋划相关技术研发和储备、攻关可解释型人工智能技术、推动行业数据标准化管理等应对措施.【期刊名称】《石油科技论坛》【年(卷),期】2018(037)004【总页数】9页(P32-40)【关键词】人工智能;石油工程;大数据;自动化;黑箱【作者】刘伟;闫娜【作者单位】中国石油集团工程技术研究院有限公司;中国石化石油工程技术研究院【正文语种】中文【中图分类】F273.1;F416.2Gartner公司在界定及分析颠覆性技术方面具有丰富经验,在其2017年发布的报告中显示,人工智能(Artificial Intelligence,简称AI)类新兴技术在成熟度曲线上快速移动,正处于曲线的巅峰位置,与之相适应的数字化平台类技术在曲线上处于上升期,与之相匹配的商业生态扩展类技术区块链等技术有望在未来5~10年产生变革性影响。
美国国会下属的政府问责局(GAO,前身是美国总审计局)对AI的发展前景进行专题调研,认为即使人工智能技术停止前进,由今天的人工智能引发的变革仍将产生广泛而深远的影响[1]。
神经网络在石油测井解释中的应用综述
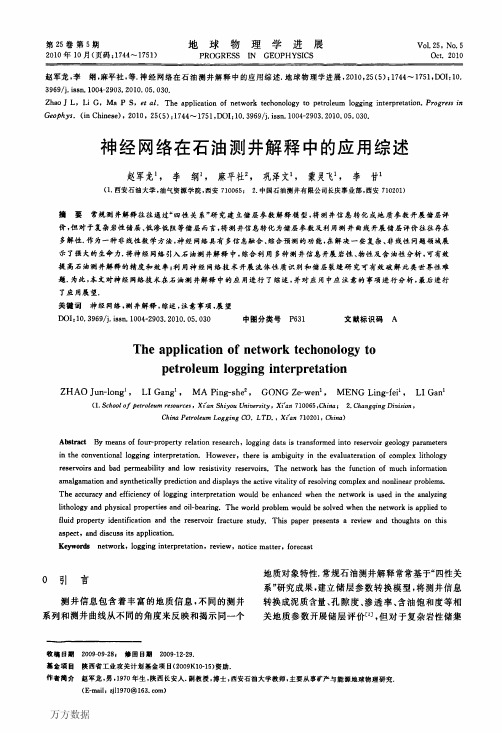
图1研究采用的神经网络模型(引自文献[281)
Fig.1 The neural network model
BP神经网络在石油测井解释中的应用
2.1在测井资料标准化方面的应用 目前,测井资料标准化的方法很多,归纳起来 有:测井曲线迭加法、岩心孑L隙度检验法、同类测井 曲线对比法、标准层测井响应对比法、测井参数二、 三维交会图法、均值方差法、克里金变异函数法、趋 势面分析法等.这些方法多为手工校正法,各有其优 缺点. 神经网络(ANN)与传统方法相比,表现出: (1)能够识别带有噪声或变形的输入模式. (2)具有很强的自适应学习能力,通过对样本的 学习,掌握模式变换的内在规律,进行判别分类和参
Logging
CO.LTD.,Xi'an 710201,China)
Abstract
By
means of four-property relation research,logging data is transformed into reservoir geology parameters
is ambiguity in the evaluateration of function of complex lithology much information
Zhao
J L,Li G,Ma P S,et a1.The application of network techonology
tO
petroleum logging interpretation.Progress in
Geophys.(inChinese),2010,25(5):1744~1751,DOI:10.3969/j.issn.1004-2903.2010.05.030.
人工神经网络自学习方法在大港滩海油田的应用
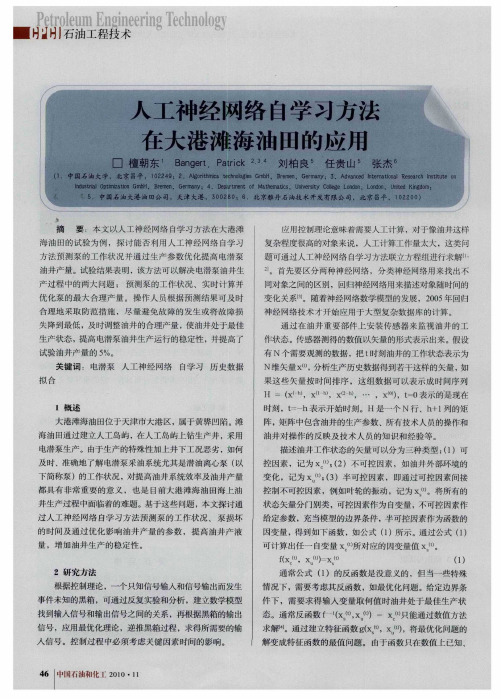
该方法实施过程 中还通过建立一 口与真实情况相同 的虚拟油井,将优化设计后的多种方案对油井进行模拟 ,
从模 拟 结果 中选 出最 佳 方 案应 用于 真 实油 井 。 这 种 方法
15 0
i
.
相对于石油软件建立的模型 ,更精确 、包含的数据量更 多、实用性更强,与人工设计模型相比,建模速度快 、 适
通 过在 油 井 重要 部 件 上 安 装传 感 器来 监 视 油 井的 工
失降到最低, 及时调整油井的合理产量 , 使油井处于最佳
生产状 态 , 高 电潜 泵油 井生 产运行 的稳 定性 , 提 并提 高 了
作状 态 。 感器 测得 的数 值 以矢量 的形 式表示 出来 。假设 传 有 N个需 要观 测的数 据 , t 把 时刻油 井 的工作状 态表 示为
I
。
方 法 预测 泵 的工 作状 况 并通 过生 产 参 数优 化 提高 电潜 泵
油井产量。试验结果表明, 该方法可以解决电潜泵油井生
产过 程 中的 两大 问题 : 预 测 泵 的工 作状 况 、 实时 计算 并 优化 泵 的最 大 合理 产 量 。 操 作 人员 根据 预 测 结 果可 及 时
变 化 ,记 为 X“;3 )( )半 可控 因 素 ,即通 过可 控因 素间 接 控 制不可 控 因素 ,例 如叶 轮的 振动 ,记 为 X“ 。将 所有 的 ) 状 态矢量 分 f ̄类 , 控 因素作 为 自变 量 ,不可控 因素 作 qt 可 ]
给定参数 , 充当模型的边界条件, 半可控因素作为函数的
因变量 , 到如 下 函数 , 公 式 ( ) 示 。 过公 式 ( ) 得 如 1所 通 1 可计算 出任一 自变 量 x( 对 应的 因变 量值 x( 所 。 ”
人工神经网络在石油勘探中的应用
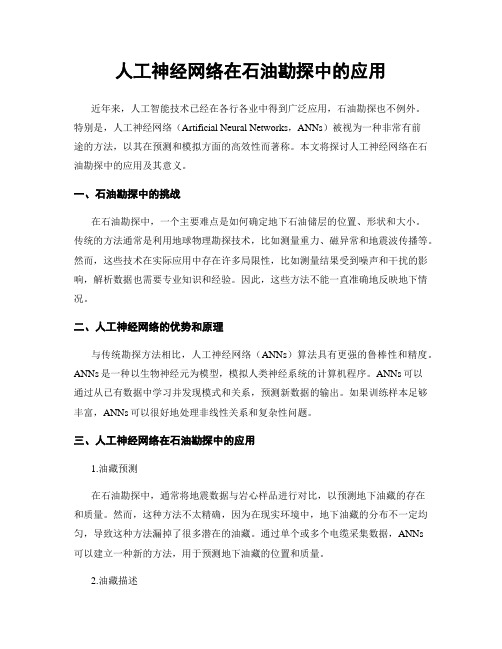
人工神经网络在石油勘探中的应用近年来,人工智能技术已经在各行各业中得到广泛应用,石油勘探也不例外。
特别是,人工神经网络(Artificial Neural Networks,ANNs)被视为一种非常有前途的方法,以其在预测和模拟方面的高效性而著称。
本文将探讨人工神经网络在石油勘探中的应用及其意义。
一、石油勘探中的挑战在石油勘探中,一个主要难点是如何确定地下石油储层的位置、形状和大小。
传统的方法通常是利用地球物理勘探技术,比如测量重力、磁异常和地震波传播等。
然而,这些技术在实际应用中存在许多局限性,比如测量结果受到噪声和干扰的影响,解析数据也需要专业知识和经验。
因此,这些方法不能一直准确地反映地下情况。
二、人工神经网络的优势和原理与传统勘探方法相比,人工神经网络(ANNs)算法具有更强的鲁棒性和精度。
ANNs是一种以生物神经元为模型,模拟人类神经系统的计算机程序。
ANNs可以通过从已有数据中学习并发现模式和关系,预测新数据的输出。
如果训练样本足够丰富,ANNs可以很好地处理非线性关系和复杂性问题。
三、人工神经网络在石油勘探中的应用1.油藏预测在石油勘探中,通常将地震数据与岩心样品进行对比,以预测地下油藏的存在和质量。
然而,这种方法不太精确,因为在现实环境中,地下油藏的分布不一定均匀,导致这种方法漏掉了很多潜在的油藏。
通过单个或多个电缆采集数据,ANNs可以建立一种新的方法,用于预测地下油藏的位置和质量。
2.油藏描述石油勘探人员经常需要对地下的油藏进行描述和建模,以便更好地开采。
这通常需要大量的地球物理测量和工程技术,但由于数据收集不完整,这种模型描述常常存在不确定性。
ANNs可以利用现有测量数据,并根据数据层次结构和特征提取来生成更准确和具有代表性的模型。
3.油藏分析在油藏分析中,石油勘探人员需要了解油藏内部的构成和特征,以确定更好开采油藏的方法。
这通常需要从地下采集数据和从地表进行实验室分析,并对数据进行特征提取和处理。
人工神经网络技术在油气田开发中的应用综述
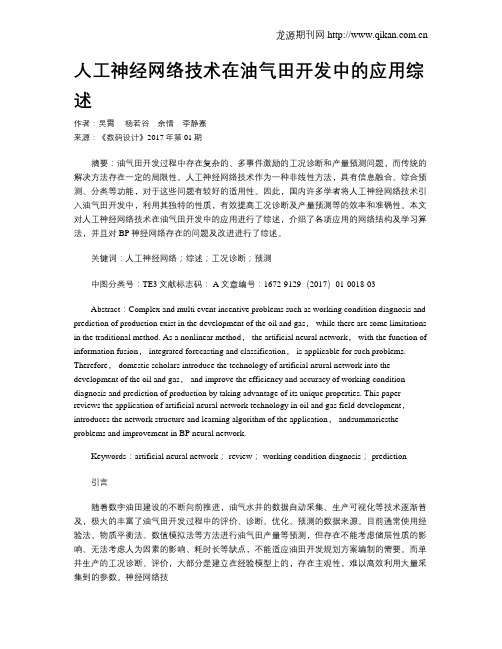
人工神经网络技术在油气田开发中的应用综述作者:吴霄杨若谷余情李静嘉来源:《数码设计》2017年第01期摘要:油气田开发过程中存在复杂的、多事件激励的工况诊断和产量预测问题,而传统的解决方法存在一定的局限性。
人工神经网络技术作为一种非线性方法,具有信息融合、综合预测、分类等功能,对于这些问题有较好的适用性。
因此,国内许多学者将人工神经网络技术引入油气田开发中,利用其独特的性质,有效提高工况诊断及产量预测等的效率和准确性。
本文对人工神经网络技术在油气田开发中的应用进行了综述,介绍了各项应用的网络结构及学习算法,并且对BP神经网络存在的问题及改进进行了综述。
关键词:人工神经网络;综述;工况诊断;预测中图分类号:TE3文献标志码: A文章编号:1672-9129(2017)01-0018-03Abstract:Complex and multi event incentive problems such as working condition diagnosis and prediction of production exist in the development of the oil and gas, while there are some limitations in the traditional method. As a nonlinear method, the artificial neural network, with the function of information fusion, integrated forecasting and classification, is applicable for such problems. Therefore, domestic scholars introduce the technology of artificial neural network into the development of the oil and gas, and improve the efficiency and accuracy of working condition diagnosis and prediction of production by taking advantage of its unique properties. This paper reviews the application of artificial neural network technology in oil and gas field development,introduces the network structure and learning algorithm of the application, andsummariesthe problems and improvement in BP neural network.Keywords:artificial neural network; review; working condition diagnosis; prediction引言随着数字油田建设的不断向前推进,油气水井的数据自动采集、生产可视化等技术逐渐普及,极大的丰富了油气田开发过程中的评价、诊断、优化、预测的数据来源。
人工神经网络在石油水压裂过程仿真中的应用
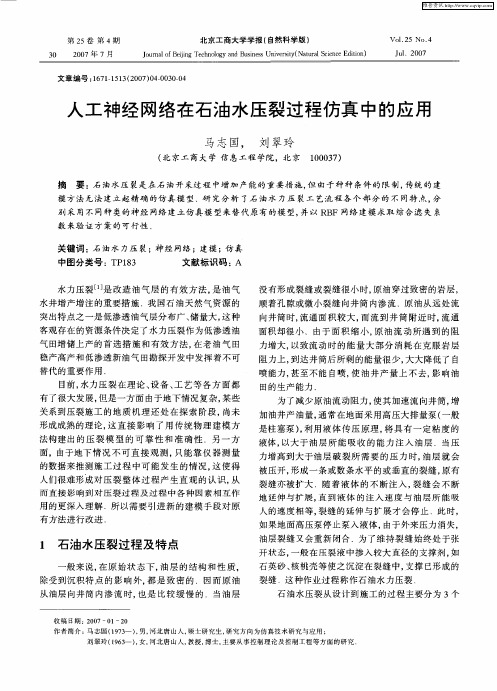
模 方法 无法 建立起 精确 的仿 真模 型 ,研 究分 析 了石 油 水 力 压 裂工 艺流 程 各 个部 分 的不 同特 点 , 分 别 采 用不 同种 类的神 经 网络 建 立仿真 模 型来替 代原 有的模 型 , 以 R F网络 建模 求取 综合 滤 失 系 并 B
维普资讯
第2 5卷 第 4期
3 0 20 0 7年 7月
北京工商大学学报 ( 自然 科 学 版 )
Junlf ei eho g dBs e n esyN t aSi c E i n orao Bi gTcnl y n ui s u i rt( a rlc ne di ) j n o a ns v i u e t o
维普资讯
第2 5卷 第 4期
马志国等 :人工神经网络在石油水压裂过程仿真 中的应用
客观 存 在的 资源条件 决 定 了水力 压裂 作为 低渗透 油
顺着 孔 隙或微 小裂缝 向井筒 内渗 流 , 油从远 处 流 原
向井筒 时, 通面 积 较大 , 流 到井 筒 附近 时 , 通 流 而 流 面积 却很 小 ,由于 面 积 缩 小 , 油 流 动 所 遇 到 的 阻 原 力增 大 , 以致 流动 时 的 能 量大 部 分 消 耗 在克 服 岩 层 阻 力上 , 到达 井筒 后所 剩 的能量很 少 , 大大 降低 了 自 喷 能力 , 甚至 不能 自喷 , 油 井 产量 上 不 去 , 响 油 使 影
地 延伸 与扩展 , 到液 体 的 注入 速 度 与 油 层所 能 吸 直
用 的更深 入理 解 .所 以需要 引进 新 的建 模 手段 对 原
人工神经网络在石油工业中的应用及未来发展趋势探讨
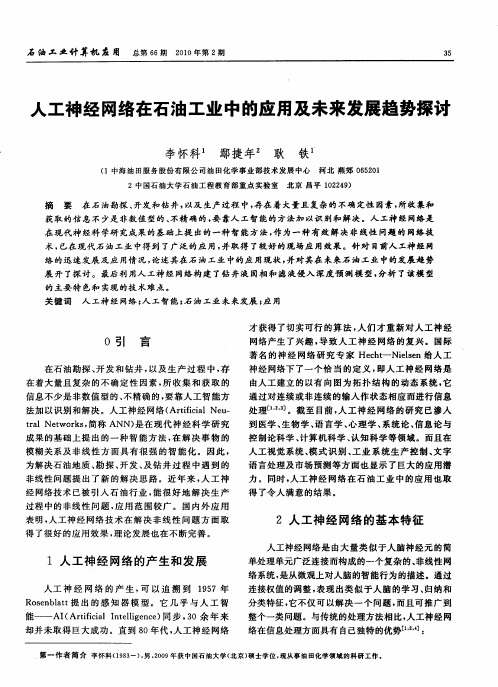
网络产 生 了兴趣 , 导致 人 工 神 经 网 络 的复 兴 。国际 著名 的 神 经 网 络研 究 专 家 Heh - Ni sn给人 工 et ee l 神经 网络下 了一 个恰 当 的定 义 , 即人 工神 经 网络 是 由人工 建立 的 以有 向 图为 拓 扑 结 构 的动 态 系统 , 它
成 果 的基 础 上提 出 的一 种 智 能方 法 , 解 决 事 物 的 在
通 过对 连续或 非连 续 的输入 作状 态相应 而进行信 息 处 理[ 矗 。截至 目前 , 1 ] 人工 神 经 网络 的研 究 已渗 入 到 医学 、 物 学 、 言学 、 理 学 、 统论 、 息论 与 生 语 心 系 信
() 1 并行结 构 和并行 处 理 人 工 神 经 网络 的计 算 功 能 分 布 在 多 个神 经 元
(0 油井 水力 压裂[ ,] 1) 22 01
不难 看 出 , 述这 些 应 用 领域 与 石 油工 业 的 发 上 展 紧密相 关 , 这将 为实 现 石 油 工业 的智 能 化 和 现代 化带 来新 的活 力和应 用前 景 。
术, 已在现 代石 油 工业 中得 到 了广泛 的应 用 , 并取得 了较 好 的现场应 用 效果 。针 对 目前人 工神 经 网
络的 迅速 发展 及应 用情 况 , 论述 其在 石 油工业 中的应 用现状 , 并对其 在 未来石 油 工业 中的发展 趋势
展 开 了探 讨 。最后利 用人 工神 经 网络 构建 了钻 井液 固相 和滤 液侵 入 深 度预 测 模 型 , 分析 了该模 型
连接权值 的调 整 , 现 出类 似 于人 脑 的学 习 、 表 归纳 和
分类特征 , 它不 仅可 以解 决 一个 问题 , 且可 推广 到 而
人工神经网络在油藏数据训练中的应用
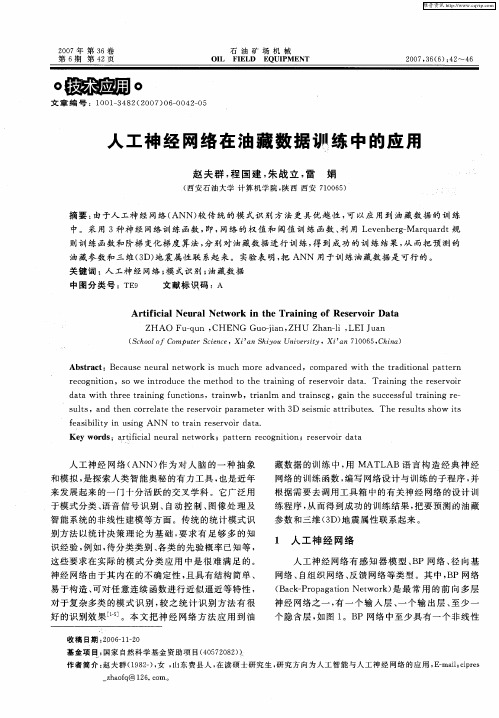
Ab t a t s r c :Be a e ne r lne wo k i u h mor d nc d,c m p r d wih t e t a ii a a t r c us u a t r S m c e a va e o a e t h r d ton lp t e n r c nii n,S ntod e t e ho o t r i ng ofr s r oi t . Tr i n he r s r o r e og to O we i r uc he m t d t he t ani e e v rda a ani g t e e v i da a wih t r e t a n ng f t t h e r i i unci s,t a n ton r i wb,ti nl a r i c ra m nd t a ns g,gan t uc e s ult ani e i he s c s f r i ng r — s ls,a he or e a e t e e vo rpa a e e t ut nd t n c r lt her s r i r m t rwih 3D es i t rbu e .Ther s ls s w t s im ca t i t s e u t ho is f a i iiy i sng ANN o t a n r s r oi t . e s b lt n u i t r i e e v r da a Ke r y wo ds:a tfca u a t r riii lne r lne wo k;p t e n r c gn to a t r e o ii n;r s r i a a e e vo r d t
- 1、下载文档前请自行甄别文档内容的完整性,平台不提供额外的编辑、内容补充、找答案等附加服务。
- 2、"仅部分预览"的文档,不可在线预览部分如存在完整性等问题,可反馈申请退款(可完整预览的文档不适用该条件!)。
- 3、如文档侵犯您的权益,请联系客服反馈,我们会尽快为您处理(人工客服工作时间:9:00-18:30)。
17
What are ANN Used for?
Hale Waihona Puke Brain modeling Models of human development – help children with developmental problems Simulations of adult performance – aid our understanding of how the brain works Neuropsychological models – suggest remedial actions for brain damaged patients Real world applications Financial modeling – predicting stocks, shares, currency exchange rates Time series prediction – climate, weather, airline marketing tactician Computer games – intelligent agents, backgammon, first person shooters Control systems – autonomous adaptable robots, microwave controllers Pattern recognition – speech recognition, hand-writing recognition, sonar signals Data analysis – data compression, data mining Noise reduction – function approximation, ECG noise reduction Bioinformatics – protein secondary structure, DNA sequencing Petroleum Engineering -------------------- WVU -----------------------
From a practical point of view, an ANN is just a parallel computational system consisting of many simple processing elements connected together in a specific way in order to perform a particular task.
Artificial Neural Networks and Reservoir Engineering Applications
Dr. Guojian Cheng
Department of Petroleum Engineering, West Virginia University School of Computer Science, Xi’an Shiyou University gucheng@, gjcheng.xsyu@
16
What are ANN Used for?
Brain modeling: The scientific goal of building models of how real brains work
This can potentially help us understand the nature of human intelligence, formulate better teaching strategies, or better remedial actions for brain damaged patients.
2
Lecture Topics
1. 2. 3. 4. 5.
6.
7.
An Overview of Computational Intelligence Applications for Petroleum Reservoir Engineering Artificial Neural Networks and Reservoir Engineering Applications Support Vector Machines and Reservoir Engineering Applications Fuzzy System and Reservoir Engineering Applications Evolutionary Computation and Reservoir Engineering Applications Swarm Intelligence and Reservoir Engineering Applications Hybrid Intelligence System and Reservoir Engineering Applications
12
Perceptron Computing
13
Perceptron Computing
14
What are ANN?
Neural Networks (NNs) are networks of neurons, as found in real our brains. Artificial Neurons are crude approximations of the biological neurons. They may be physical devices, or purely mathematical constructs. Artificial Neural Networks (ANNs) are networks of Artificial Neurons. They may be physical devices, or simulated on conventional computers.
4
Biological Neurons vs. Artificial Neurons
Biological Neurons encode their outputs or activations as a series of brief electrical pulses (i.e. spikes or action potentials). Dendrites are the receptive zones that receive activation from other neurons. Cell body (soma) of the neuron’s processes the incoming activations and converts them into output activations. Axons are transmission lines that send activation to other neurons. Synapses allow weighted transmission of signals (using neurotransmitters) between axons and dendrites to build up large neural networks.
6
Activation functions of a neuron
Step function
Y
Sign function Sigmoid function Linear function
Y Y Y
+1
0 -1 X
+1
0 -1 X
1
0 -1 X
1
0 -1 X
1, if X 0 sign 1, if X 0 sigmoid step Y Y Y 0, if X 0 1, if X 0
15
Why Study ANN ?
They are extremely powerful computational devices (Turing equivalent, universal computers) Massive parallelism makes them very efficient They can learn and generalize from training data They are particularly fault tolerant – this is equivalent to the “graceful degradation” found in biological systems
Main References: 1. Michael Negnevitsky, “Artificial Intelligence: A Guide to Intelligent Systems (2nd Edition)", Addison Wesley,2004,ISBN: 0321204662 2. Arthur K. Kordon, "Applying Computational Intelligence: How to Create Value", Springer,2009, ISBN: 3540699104 3. / 4. / 5. /
Artificial System Building: The engineering goal of building efficient systems for real world applications
This may make machines more powerful, relieve humans of tedious tasks, and may even improve upon human performance.