Efficient Kernel Machines Using the Improved Fast Gauss Transform
人工智能生态相关的专业名词解释
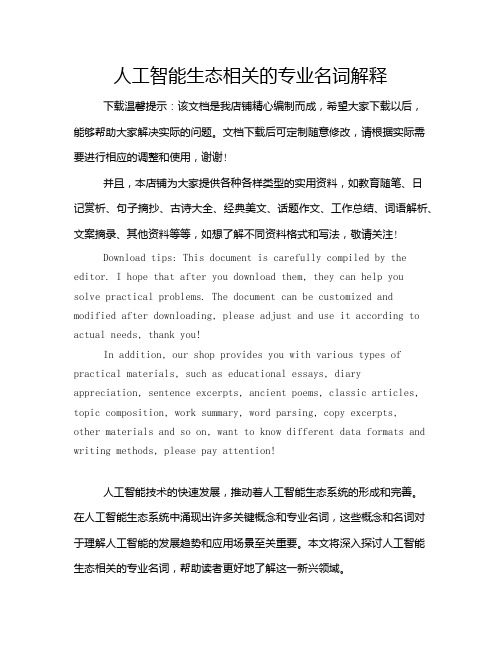
人工智能生态相关的专业名词解释下载温馨提示:该文档是我店铺精心编制而成,希望大家下载以后,能够帮助大家解决实际的问题。
文档下载后可定制随意修改,请根据实际需要进行相应的调整和使用,谢谢!并且,本店铺为大家提供各种各样类型的实用资料,如教育随笔、日记赏析、句子摘抄、古诗大全、经典美文、话题作文、工作总结、词语解析、文案摘录、其他资料等等,如想了解不同资料格式和写法,敬请关注!Download tips: This document is carefully compiled by the editor. I hope that after you download them, they can help yousolve practical problems. The document can be customized and modified after downloading, please adjust and use it according to actual needs, thank you!In addition, our shop provides you with various types of practical materials, such as educational essays, diary appreciation, sentence excerpts, ancient poems, classic articles, topic composition, work summary, word parsing, copy excerpts,other materials and so on, want to know different data formats and writing methods, please pay attention!人工智能技术的快速发展,推动着人工智能生态系统的形成和完善。
基于RPMDE-MKSVM的锂离子电池剩余使用寿命预测
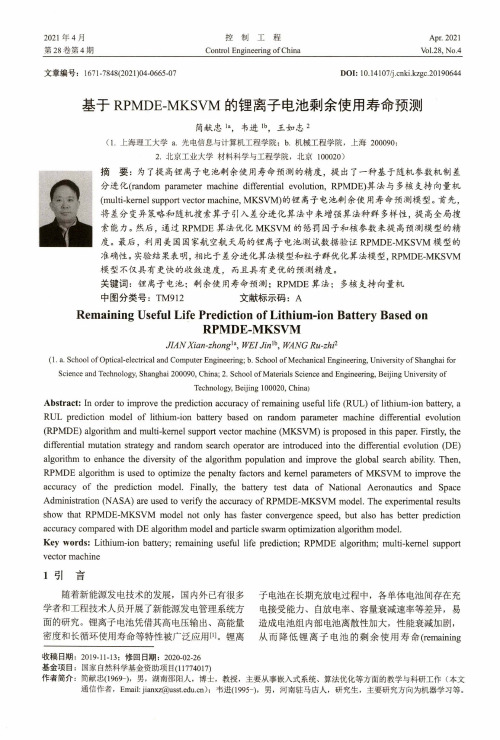
2021年4月第28卷第4期控制工程Control Engineering of ChinaApr.2021Vol.28,No.4文章编号:1671-7848(2021)04-0665-07DOI: 10.14107/ki.kzgc.20190644基于R PM D E-M K S V M的锂离子电池剩余使用寿命预测简献忠l a,韦进l b,王如志2(1.上海理工大学a.光电信息与计算机工程学院;b.机械工程学院,上海200090;2.北京工业大学材料科学与工程学院,北京100020)摘要:为了提高锂离子电池剩余使用寿命预测的精度,提出了一种基于随机参数机制差分进化(random parameter machine differential evolution,R P M D E)算法与多核支持向量机(multi-kernel support vector machine,M K S V M)的锂离子电池剩余使用寿命预测模型。
首先,将差分变异策略和随机搜索算子引入差分进化算法中来增强算法种群多样性,提高全局搜索能力。
然后,通过R P M D E算法优化M K S V M的惩罚因子和核参数来提高预測模型的精度。
最后,利用美国国家航空航天局的锂离子电池測试数据验证R P M D E-M K S V M模型的准确性。
实验结果表明,相比于差分进化算法模型和粒子群优化算法模型,R P M D E-M K S V M模型不仅具有更快的收敛速度,而且具有更优的预測精度。
关键词:锂离子电池;剩余使用寿命预测;R P M D E算法;多核支持向量机中图分类号:T M912 文献标示码:ARemaining Useful Life Prediction of Lithium-ion Battery Based onRPMDE-MKSVMJ I A N X i a n-z h o n g x\ W E I J i n l b,W A N G R u-zhi2(1.a.School of Optical-electrical and Computer Engineering;b.School of Mechanical Engineering,University of Shanghai forScience and Technology,Shanghai200090, China;2.School of Materials Science and Engineering,Beijing University ofTechnology,Beijing 100020, China)Abstract:In order to improve the prediction accuracy of remaining useful life(R U L)of lithium-ion battery,a R U L prediction model of lithium-ion battery based on ran d o m parameter machine differential evolution (R P M D E)algorithm and multi-kernel support vector machine(M K S V M)is proposed in this paper.Firstly,the differential mutation strategy and rand o m search operator are introduced into the differential evolution (D E) algorithm to enhance the diversity of the algorithm population and improve the global search ability.T h e n, R P M D E algorithm is used to optimize the penalty factors and kernel parameters of M K S V M to improve the accuracy of the prediction m o d e l.Finally,the battery test data of National Aeronautics and Space Administration(N A S A)are used to verify the accuracy of R P M D E-M K S V M m o d e l.T h e experimental results s h o w that R P M D E-M K S V M model not only has faster convergence speed,but also has better prediction accuracy compared with D E algorithm model and particle s w a r m optimization algorithm m o d e l.K e y w o r d s:Lithium-ion battery;remaining useful life prediction;R P M D E algorithm;multi-kernel support vector machineOi引言随着新能源发电技术的发展,国内外已有很多 学者和工程技术人员开展了新能源发电管理系统方 面的研究。
人脸表情识别英文参考资料
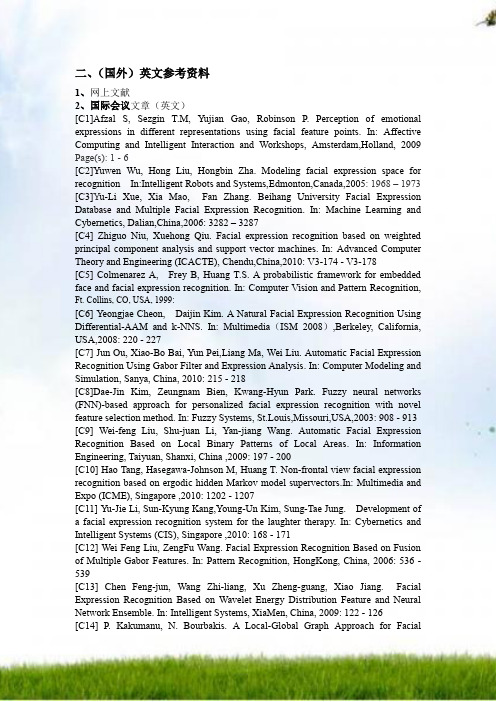
二、(国外)英文参考资料1、网上文献2、国际会议文章(英文)[C1]Afzal S, Sezgin T.M, Yujian Gao, Robinson P. Perception of emotional expressions in different representations using facial feature points. In: Affective Computing and Intelligent Interaction and Workshops, Amsterdam,Holland, 2009 Page(s): 1 - 6[C2]Yuwen Wu, Hong Liu, Hongbin Zha. Modeling facial expression space for recognition In:Intelligent Robots and Systems,Edmonton,Canada,2005: 1968 – 1973 [C3]Y u-Li Xue, Xia Mao, Fan Zhang. Beihang University Facial Expression Database and Multiple Facial Expression Recognition. In: Machine Learning and Cybernetics, Dalian,China,2006: 3282 – 3287[C4] Zhiguo Niu, Xuehong Qiu. Facial expression recognition based on weighted principal component analysis and support vector machines. In: Advanced Computer Theory and Engineering (ICACTE), Chendu,China,2010: V3-174 - V3-178[C5] Colmenarez A, Frey B, Huang T.S. A probabilistic framework for embedded face and facial expression recognition. In: Computer Vision and Pattern Recognition, Ft. Collins, CO, USA, 1999:[C6] Yeongjae Cheon, Daijin Kim. A Natural Facial Expression Recognition Using Differential-AAM and k-NNS. In: Multimedia(ISM 2008),Berkeley, California, USA,2008: 220 - 227[C7]Jun Ou, Xiao-Bo Bai, Yun Pei,Liang Ma, Wei Liu. Automatic Facial Expression Recognition Using Gabor Filter and Expression Analysis. In: Computer Modeling and Simulation, Sanya, China, 2010: 215 - 218[C8]Dae-Jin Kim, Zeungnam Bien, Kwang-Hyun Park. Fuzzy neural networks (FNN)-based approach for personalized facial expression recognition with novel feature selection method. In: Fuzzy Systems, St.Louis,Missouri,USA,2003: 908 - 913 [C9] Wei-feng Liu, Shu-juan Li, Yan-jiang Wang. Automatic Facial Expression Recognition Based on Local Binary Patterns of Local Areas. In: Information Engineering, Taiyuan, Shanxi, China ,2009: 197 - 200[C10] Hao Tang, Hasegawa-Johnson M, Huang T. Non-frontal view facial expression recognition based on ergodic hidden Markov model supervectors.In: Multimedia and Expo (ICME), Singapore ,2010: 1202 - 1207[C11] Yu-Jie Li, Sun-Kyung Kang,Young-Un Kim, Sung-Tae Jung. Development of a facial expression recognition system for the laughter therapy. In: Cybernetics and Intelligent Systems (CIS), Singapore ,2010: 168 - 171[C12] Wei Feng Liu, ZengFu Wang. Facial Expression Recognition Based on Fusion of Multiple Gabor Features. In: Pattern Recognition, HongKong, China, 2006: 536 - 539[C13] Chen Feng-jun, Wang Zhi-liang, Xu Zheng-guang, Xiao Jiang. Facial Expression Recognition Based on Wavelet Energy Distribution Feature and Neural Network Ensemble. In: Intelligent Systems, XiaMen, China, 2009: 122 - 126[C14] P. Kakumanu, N. Bourbakis. A Local-Global Graph Approach for FacialExpression Recognition. In: Tools with Artificial Intelligence, Arlington, Virginia, USA,2006: 685 - 692[C15] Mingwei Huang, Zhen Wang, Zilu Ying. Facial expression recognition using Stochastic Neighbor Embedding and SVMs. In: System Science and Engineering (ICSSE), Macao, China, 2011: 671 - 674[C16] Junhua Li, Li Peng. Feature difference matrix and QNNs for facial expression recognition. In: Control and Decision Conference, Yantai, China, 2008: 3445 - 3449 [C17] Yuxiao Hu, Zhihong Zeng, Lijun Yin,Xiaozhou Wei, Jilin Tu, Huang, T.S. A study of non-frontal-view facial expressions recognition. In: Pattern Recognition, Tampa, FL, USA,2008: 1 - 4[C18] Balasubramani A, Kalaivanan K, Karpagalakshmi R.C, Monikandan R. Automatic facial expression recognition system. In: Computing, Communication and Networking, St. Thomas,USA, 2008: 1 - 5[C19] Hui Zhao, Zhiliang Wang, Jihui Men. Facial Complex Expression Recognition Based on Fuzzy Kernel Clustering and Support Vector Machines. In: Natural Computation, Haikou,Hainan,China,2007: 562 - 566[C20] Khanam A, Shafiq M.Z, Akram M.U. Fuzzy Based Facial Expression Recognition. In: Image and Signal Processing, Sanya, Hainan, China,2008: 598 - 602 [C21] Sako H, Smith A.V.W. Real-time facial expression recognition based on features' positions and dimensions. In: Pattern Recognition, Vienna,Austria, 1996: 643 - 648 vol.3[C22] Huang M.W, Wang Z.W, Ying Z.L. A novel method of facial expression recognition based on GPLVM Plus SVM. In: Signal Processing (ICSP), Beijing, China, 2010: 916 - 919[C23] Xianxing Wu; Jieyu Zhao; Curvelet feature extraction for face recognition and facial expression recognition. In: Natural Computation (ICNC), Yantai, China, 2010: 1212 - 1216[C24]Xu Q Z, Zhang P Z, Yang L X, et al.A facial expression recognition approach based on novel support vector machine tree. In Proceedings of the 4th International Symposium on Neural Networks, Nanjing, China, 2007: 374-381.[C25] Wang Y B, Ai H Z, Wu B, et al. Real time facial expression recognition with adaboost.In: Proceedings of the 17th International Conference on Pattern Recognition , Cambridge,UK, 2004: 926-929.[C26] Guo G, Dyer C R. Simultaneous feature selection and classifier training via linear programming: a case study for face expression recognition. In: Proceedings of the 2003 IEEE Computer Society Conference on Computer Vision and Pattern Recognition, Madison, W isconsin, USA, 2003,1: 346-352.[C27] Bourel F, Chibelushi C C, Low A A. Robust facial expression recognition using a state-based model of spatially-localised facial dynamics. In: Proceedings of the 5th IEEE International Conference on Automatic Face and Gesture Recognition, Washington, DC, USA, 2002: 113-118·[C28] Buciu I, Kotsia I, Pitas I. Facial expression analysis under partial occlusion. In: Proceedings of the 2005 IEEE International Conference on Acoustics, Speech, and Signal Processing, Philadelphia, PA, USA, 2005,V: 453-456.[C29] ZHAN Yong-zhao,YE Jing-fu,NIU De-jiao,et al.Facial expression recognition based on Gabor wavelet transformation and elastic templates matching. Proc of the 3rd International Conference on Image and Graphics.Washington DC, USA,2004:254-257.[C30] PRASEEDA L V,KUMAR S,VIDYADHARAN D S,et al.Analysis of facial expressions using PCA on half and full faces. Proc of ICALIP2008.2008:1379-1383.[C31] LEE J J,UDDIN M Z,KIM T S.Spatiotemporal human facial expression recognition using Fisher independent component analysis and Hidden Markov model [C]//Proc of the 30th Annual International Conference of IEEE Engineering in Medicine and Biology Society.2008:2546-2549.[C32] LITTLEWORT G,BARTLETT M,FASELL. Dynamics of facial expression extracted automatically from video. In: Proceedings of IEEE Conference on Computer Vision and Pattern Recognition, Workshop on Face Processing inVideo, Washington DC,USA,2006:80-81.[C33] Kotsia I, Nikolaidis N, Pitas I. Facial Expression Recognition in Videos using a Novel Multi-Class Support Vector Machines Variant. In: Acoustics, Speech and Signal Processing, Honolulu, Hawaii, USA, 2007: II-585 - II-588[C34] Ruo Du, Qiang Wu, Xiangjian He, Wenjing Jia, Daming Wei. Facial expression recognition using histogram variances faces. In: Applications of Computer Vision (WACV), Snowbird, Utah, USA, 2009: 1 - 7[C35] Kobayashi H, Tange K, Hara F. Real-time recognition of six basic facial expressions. In: Robot and Human Communication, Tokyo , Japan,1995: 179 - 186 [C36] Hao Tang, Huang T.S. 3D facial expression recognition based on properties of line segments connecting facial feature points. In: Automatic Face & Gesture Recognition, Amsterdam, The Netherlands, 2008: 1 - 6[C37] Fengjun Chen, Zhiliang Wang, Zhengguang Xu, Donglin Wang. Research on a method of facial expression recognition.in: Electronic Measurement & Instruments, Beijing,China, 2009: 1-225 - 1-229[C38] Hui Zhao, Tingting Xue, Linfeng Han. Facial complex expression recognition based on Latent DirichletAllocation. In: Natural Computation (ICNC), Yantai, Shandong, China, 2010: 1958 - 1960[C39] Qinzhen Xu, Pinzheng Zhang, Wenjiang Pei, Luxi Yang, Zhenya He. An Automatic Facial Expression Recognition Approach Based on Confusion-Crossed Support Vector Machine Tree. In: Acoustics, Speech and Signal Processing, Honolulu, Hawaii, USA, 2007: I-625 - I-628[C40] Sung Uk Jung, Do Hyoung Kim, Kwang Ho An, Myung Jin Chung. Efficient rectangle feature extraction for real-time facial expression recognition based on AdaBoost.In: Intelligent Robots and Systems, Edmonton,Canada, 2005: 1941 - 1946[C41] Patil K.K, Giripunje S.D, Bajaj P.R. Facial Expression Recognition and Head Tracking in Video Using Gabor Filter .In: Emerging Trends in Engineering and Technology (ICETET), Goa, India, 2010: 152 - 157[C42] Jun Wang, Lijun Yin, Xiaozhou Wei, Yi Sun. 3D Facial Expression Recognition Based on Primitive Surface Feature Distribution.In: Computer Vision and PatternRecognition, New York, USA,2006: 1399 - 1406[C43] Shi Dongcheng, Jiang Jieqing. The method of facial expression recognition based on DWT-PCA/LDA.IN: Image and Signal Processing (CISP), Yantai,China, 2010: 1970 - 1974[C44] Asthana A, Saragih J, Wagner M, Goecke R. Evaluating AAM fitting methods for facial expression recognition. In: Affective Computing and Intelligent Interaction and Workshops, Amsterdam,Holland, 2009:1-8[C45] Geng Xue, Zhang Youwei. Facial Expression Recognition Based on the Difference of Statistical Features.In: Signal Processing, Guilin, China, 2006[C46] Metaxas D. Facial Features Tracking for Gross Head Movement analysis and Expression Recognition.In: Multimedia Signal Processing, Chania,Crete,GREECE, 2007:2[C47] Xia Mao, YuLi Xue, Zheng Li, Kang Huang, ShanWei Lv. Robust facial expression recognition based on RPCA and AdaBoost.In: Image Analysis for Multimedia Interactive Services, London, UK, 2009: 113 - 116[C48] Kun Lu, Xin Zhang. Facial Expression Recognition from Image Sequences Based on Feature Points and Canonical Correlations.In: Artificial Intelligence and Computational Intelligence (AICI), Sanya,China, 2010: 219 - 223[C49] Peng Zhao-yi, Wen Zhi-qiang, Zhou Yu. Application of Mean Shift Algorithm in Real-Time Facial Expression Recognition.In: Computer Network and Multimedia Technology, Wuhan,China, 2009: 1 - 4[C50] Xu Chao, Feng Zhiyong, Facial Expression Recognition and Synthesis on Affective Emotions Composition.In: Future BioMedical Information Engineering, Wuhan,China, 2008: 144 - 147[C51] Zi-lu Ying, Lin-bo Cai. Facial Expression Recognition with Marginal Fisher Analysis on Local Binary Patterns.In: Information Science and Engineering (ICISE), Nanjing,China, 2009: 1250 - 1253[C52] Chuang Yu, Yuning Hua, Kun Zhao. The Method of Human Facial Expression Recognition Based on Wavelet Transformation Reducing the Dimension and Improved Fisher Discrimination.In: Intelligent Networks and Intelligent Systems (ICINIS), Shenyang,China, 2010: 43 - 47[C53] Stratou G, Ghosh A, Debevec P, Morency L.-P. Effect of illumination on automatic expression recognition: A novel 3D relightable facial database .In: Automatic Face & Gesture Recognition and Workshops (FG 2011), Santa Barbara, California,USA, 2011: 611 - 618[C54] Jung-Wei Hong, Kai-Tai Song. Facial expression recognition under illumination variation.In: Advanced Robotics and Its Social Impacts, Hsinchu, Taiwan,2007: 1 - 6[C55] Ryan A, Cohn J.F, Lucey S, Saragih J, Lucey P, De la Torre F, Rossi A. Automated Facial Expression Recognition System.In: Security Technology, Zurich, Switzerland, 2009: 172 - 177[C56] Gokturk S.B, Bouguet J.-Y, Tomasi C, Girod B. Model-based face tracking for view-independent facial expression recognition.In: Automatic Face and Gesture Recognition, Washington, D.C., USA, 2002: 287 - 293[C57] Guo S.M, Pan Y.A, Liao Y.C, Hsu C.Y, Tsai J.S.H, Chang C.I. A Key Frame Selection-Based Facial Expression Recognition System.In: Innovative Computing, Information and Control, Beijing,China, 2006: 341 - 344[C58] Ying Zilu, Li Jingwen, Zhang Youwei. Facial expression recognition based on two dimensional feature extraction.In: Signal Processing, Leipzig, Germany, 2008: 1440 - 1444[C59] Fengjun Chen, Zhiliang Wang, Zhengguang Xu, Jiang Xiao, Guojiang Wang. Facial Expression Recognition Using Wavelet Transform and Neural Network Ensemble.In: Intelligent Information Technology Application, Shanghai,China,2008: 871 - 875[C60] Chuan-Yu Chang, Yan-Chiang Huang, Chi-Lu Yang. Personalized Facial Expression Recognition in Color Image.In: Innovative Computing, Information and Control (ICICIC), Kaohsiung,Taiwan, 2009: 1164 - 1167[C61] Bourel F, Chibelushi C.C, Low A.A. Robust facial expression recognition using a state-based model of spatially-localised facial dynamics. In: Automatic Face and Gesture Recognition, Washington, D.C., USA, 2002: 106 - 111[C62] Chen Juanjuan, Zhao Zheng, Sun Han, Zhang Gang. Facial expression recognition based on PCA reconstruction.In: Computer Science and Education (ICCSE), Hefei,China, 2010: 195 - 198[C63] Guotai Jiang, Xuemin Song, Fuhui Zheng, Peipei Wang, Omer A.M. Facial Expression Recognition Using Thermal Image.In: Engineering in Medicine and Biology Society, Shanghai,China, 2005: 631 - 633[C64] Zhan Yong-zhao, Ye Jing-fu, Niu De-jiao, Cao Peng. Facial expression recognition based on Gabor wavelet transformation and elastic templates matching.In: Image and Graphics, Hongkong,China, 2004: 254 - 257[C65] Ying Zilu, Zhang Guoyi. Facial Expression Recognition Based on NMF and SVM. In: Information Technology and Applications, Chengdu,China, 2009: 612 - 615 [C66] Xinghua Sun, Hongxia Xu, Chunxia Zhao, Jingyu Yang. Facial expression recognition based on histogram sequence of local Gabor binary patterns. In: Cybernetics and Intelligent Systems, Chengdu,China, 2008: 158 - 163[C67] Zisheng Li, Jun-ichi Imai, Kaneko M. Facial-component-based bag of words and PHOG descriptor for facial expression recognition.In: Systems, Man and Cybernetics, San Antonio,TX,USA,2009: 1353 - 1358[C68] Chuan-Yu Chang, Yan-Chiang Huang. Personalized facial expression recognition in indoor environments.In: Neural Networks (IJCNN), Barcelona, Spain, 2010: 1 - 8[C69] Ying Zilu, Fang Xieyan. Combining LBP and Adaboost for facial expression recognition.In: Signal Processing, Leipzig, Germany, 2008: 1461 - 1464[C70] Peng Yang, Qingshan Liu, Metaxas, D.N. RankBoost with l1 regularization for facial expression recognition and intensity estimation.In: Computer Vision, Kyoto,Japan, 2009: 1018 - 1025[C71] Patil R.A, Sahula V, Mandal A.S. Automatic recognition of facial expressions in image sequences: A review.In: Industrial and Information Systems (ICIIS), Mangalore, India, 2010: 408 - 413[C72] Iraj Hosseini, Nasim Shams, Pooyan Amini, Mohammad S. Sadri, Masih Rahmaty, Sara Rahmaty. Facial Expression Recognition using Wavelet-Based Salient Points and Subspace Analysis Methods.In: Electrical and Computer Engineering, Ottawa, Canada, 2006: 1992 - 1995[C73][C74][C75][C76][C77][C78][C79]3、英文期刊文章[J1] Aleksic P.S., Katsaggelos A.K. Automatic facial expression recognition using facial animation parameters and multistream HMMs.IEEE Transactions on Information Forensics and Security, 2006, 1(1):3-11[J2] Kotsia I,Pitas I. Facial Expression Recognition in Image Sequences Using Geometric Deformation Features and Support Vector Machines. IEEE Transactions on Image Processing, 2007, 16(1):172 – 187[J3] Mpiperis I, Malassiotis S, Strintzis M.G. Bilinear Models for 3-D Face and Facial Expression Recognition. IEEE Transactions on Information Forensics and Security, 2008,3(3) : 498 - 511[J4] Sung J, Kim D. Pose-Robust Facial Expression Recognition Using View-Based 2D+3D AAM. IEEE Transactions on Systems and Humans, 2008 , 38 (4): 852 - 866 [J5]Yeasin M, Bullot B, Sharma R. Recognition of facial expressions and measurement of levels of interest from video. IEEE Transactions on Multimedia, 2006, 8(3): 500 - 508[J6] Wenming Zheng, Xiaoyan Zhou, Cairong Zou, Li Zhao. Facial expression recognition using kernel canonical correlation analysis (KCCA). IEEE Transactions on Neural Networks, 2006, 17(1): 233 - 238[J7]Pantic M, Patras I. Dynamics of facial expression: recognition of facial actions and their temporal segments from face profile image sequences. IEEE Transactions on Systems, Man, and Cybernetics, Part B: Cybernetics, 2006, 36(2): 433 - 449[J8] Mingli Song, Dacheng Tao, Zicheng Liu, Xuelong Li, Mengchu Zhou. Image Ratio Features for Facial Expression Recognition Application. IEEE Transactions on Systems, Man, and Cybernetics, Part B: Cybernetics, 2010, 40(3): 779 - 788[J9] Dae Jin Kim, Zeungnam Bien. Design of “Personalized” Classifier Using Soft Computing Techniques for “Personalized” Facial Expression Recognition. IEEE Transactions on Fuzzy Systems, 2008, 16(4): 874 - 885[J10] Uddin M.Z, Lee J.J, Kim T.-S. An enhanced independent component-based human facial expression recognition from video. IEEE Transactions on Consumer Electronics, 2009, 55(4): 2216 - 2224[J11] Ruicong Zhi, Flierl M, Ruan Q, Kleijn W.B. Graph-Preserving Sparse Nonnegative Matrix Factorization With Application to Facial Expression Recognition.IEEE Transactions on Systems, Man, and Cybernetics, Part B: Cybernetics, 2011, 41(1): 38 - 52[J12] Chibelushi C.C, Bourel F. Hierarchical multistream recognition of facial expressions. IEE Proceedings - Vision, Image and Signal Processing, 2004, 151(4): 307 - 313[J13] Yongsheng Gao, Leung M.K.H, Siu Cheung Hui, Tananda M.W. Facial expression recognition from line-based caricatures. IEEE Transactions on Systems, Man and Cybernetics, Part A: Systems and Humans, 2003, 33(3): 407 - 412[J14] Ma L, Khorasani K. Facial expression recognition using constructive feedforward neural networks. IEEE Transactions on Systems, Man, and Cybernetics, Part B: Cybernetics, 2004, 34(3): 1588 - 1595[J15] Essa I.A, Pentland A.P. Coding, analysis, interpretation, and recognition of facial expressions. IEEE Transactions on Pattern Analysis and Machine Intelligence, 1997, 19(7): 757 - 763[J16] Anderson K, McOwan P.W. A real-time automated system for the recognition of human facial expressions. IEEE Transactions on Systems, Man, and Cybernetics, Part B: Cybernetics, 2006, 36(1): 96 - 105[J17] Soyel H, Demirel H. Facial expression recognition based on discriminative scale invariant feature transform. Electronics Letters 2010, 46(5): 343 - 345[J18] Fei Cheng, Jiangsheng Yu, Huilin Xiong. Facial Expression Recognition in JAFFE Dataset Based on Gaussian Process Classification. IEEE Transactions on Neural Networks, 2010, 21(10): 1685 – 1690[J19] Shangfei Wang, Zhilei Liu, Siliang Lv, Yanpeng Lv, Guobing Wu, Peng Peng, Fei Chen, Xufa Wang. A Natural Visible and Infrared Facial Expression Database for Expression Recognition and Emotion Inference. IEEE Transactions on Multimedia, 2010, 12(7): 682 - 691[J20] Lajevardi S.M, Hussain Z.M. Novel higher-order local autocorrelation-like feature extraction methodology for facial expression recognition. IET Image Processing, 2010, 4(2): 114 - 119[J21] Yizhen Huang, Ying Li, Na Fan. Robust Symbolic Dual-View Facial Expression Recognition With Skin Wrinkles: Local Versus Global Approach. IEEE Transactions on Multimedia, 2010, 12(6): 536 - 543[J22] Lu H.-C, Huang Y.-J, Chen Y.-W. Real-time facial expression recognition based on pixel-pattern-based texture feature. Electronics Letters 2007, 43(17): 916 - 918[J23]Zhang L, Tjondronegoro D. Facial Expression Recognition Using Facial Movement Features. IEEE Transactions on Affective Computing, 2011, pp(99): 1[J24] Zafeiriou S, Pitas I. Discriminant Graph Structures for Facial Expression Recognition. Multimedia, IEEE Transactions on 2008,10(8): 1528 - 1540[J25]Oliveira L, Mansano M, Koerich A, de Souza Britto Jr. A. Selecting 2DPCA Coefficients for Face and Facial Expression Recognition. Computing in Science & Engineering, 2011, pp(99): 1[J26] Chang K.I, Bowyer W, Flynn P.J. Multiple Nose Region Matching for 3D Face Recognition under Varying Facial Expression. Pattern Analysis and Machine Intelligence, IEEE Transactions on2006, 28(10): 1695 - 1700[J27] Kakadiaris I.A, Passalis G, Toderici G, Murtuza M.N, Yunliang Lu, Karampatziakis N, Theoharis T. Three-Dimensional Face Recognition in the Presence of Facial Expressions: An Annotated Deformable Model Approach.IEEE Transactions on Pattern Analysis and Machine Intelligence, 2007, 29(4): 640 - 649[J28] Guoying Zhao, Pietikainen M. Dynamic Texture Recognition Using Local Binary Patterns with an Application to Facial Expressions. IEEE Transactions on Pattern Analysis and Machine Intelligence, 2007, 29(6): 915 - 928[J29] Chakraborty A, Konar A, Chakraborty U.K, Chatterjee A. Emotion Recognition From Facial Expressions and Its Control Using Fuzzy Logic. IEEE Transactions on Systems, Man and Cybernetics, Part A: Systems and Humans, 2009, 39(4): 726 - 743 [J30] Pantic M, RothkrantzL J.M. Facial action recognition for facial expression analysis from static face images. IEEE Transactions on Systems, Man, and Cybernetics, Part B: Cybernetics, 2004, 34(3): 1449 - 1461[J31] Calix R.A, Mallepudi S.A, Bin Chen, Knapp G.M. Emotion Recognition in Text for 3-D Facial Expression Rendering. IEEE Transactions on Multimedia, 2010, 12(6): 544 - 551[J32]Kotsia I, Pitas I, Zafeiriou S, Zafeiriou S. Novel Multiclass Classifiers Based on the Minimization of the Within-Class Variance. IEEE Transactions on Neural Networks, 2009, 20(1): 14 - 34[J33]Cohen I, Cozman F.G, Sebe N, Cirelo M.C, Huang T.S. Semisupervised learning of classifiers: theory, algorithms, and their application to human-computer interaction. IEEE Transactions on Pattern Analysis and Machine Intelligence, 2004, 26(12): 1553 - 1566[J34] Zafeiriou S. Discriminant Nonnegative Tensor Factorization Algorithms. IEEE Transactions on Neural Networks, 2009, 20(2): 217 - 235[J35] Zafeiriou S, Petrou M. Nonlinear Non-Negative Component Analysis Algorithms. IEEE Transactions on Image Processing, 2010, 19(4): 1050 - 1066[J36] Kotsia I, Zafeiriou S, Pitas I. A Novel Discriminant Non-Negative Matrix Factorization Algorithm With Applications to Facial Image Characterization Problems. IEEE Transactions on Information Forensics and Security, 2007, 2(3): 588 - 595[J37] Irene Kotsia, Stefanos Zafeiriou, Ioannis Pitas. Texture and shape information fusion for facial expression and facial action unit recognition . Pattern Recognition, 2008, 41(3): 833-851[J38]Wenfei Gu, Cheng Xiang, Y.V. Venkatesh, Dong Huang, Hai Lin. Facial expression recognition using radial encoding of local Gabor features and classifier synthesis. Pattern Recognition, In Press, Corrected Proof, Available online 27 May 2011[J39] F Dornaika, E Lazkano, B Sierra. Improving dynamic facial expression recognition with feature subset selection. Pattern Recognition Letters, 2011, 32(5): 740-748[J40] Te-Hsun Wang, Jenn-Jier James Lien. Facial expression recognition system based on rigid and non-rigid motion separation and 3D pose estimation. Pattern Recognition, 2009, 42(5): 962-977[J41] Hyung-Soo Lee, Daijin Kim. Expression-invariant face recognition by facialexpression transformations. Pattern Recognition Letters, 2008, 29(13): 1797-1805[J42] Guoying Zhao, Matti Pietikäinen. Boosted multi-resolution spatiotemporal descriptors for facial expression recognition . Pattern Recognition Letters, 2009, 30(12): 1117-1127[J43] Xudong Xie, Kin-Man Lam. Facial expression recognition based on shape and texture. Pattern Recognition, 2009, 42(5):1003-1011[J44] Peng Yang, Qingshan Liu, Dimitris N. Metaxas Boosting encoded dynamic features for facial expression recognition . Pattern Recognition Letters, 2009,30(2): 132-139[J45] Sungsoo Park, Daijin Kim. Subtle facial expression recognition using motion magnification. Pattern Recognition Letters, 2009, 30(7): 708-716[J46] Chathura R. De Silva, Surendra Ranganath, Liyanage C. De Silva. Cloud basis function neural network: A modified RBF network architecture for holistic facial expression recognition. Pattern Recognition, 2008, 41(4): 1241-1253[J47] Do Hyoung Kim, Sung Uk Jung, Myung Jin Chung. Extension of cascaded simple feature based face detection to facial expression recognition. Pattern Recognition Letters, 2008, 29(11): 1621-1631[J48] Y. Zhu, L.C. De Silva, C.C. Ko. Using moment invariants and HMM in facial expression recognition. Pattern Recognition Letters, 2002, 23(1-3): 83-91[J49] Jun Wang, Lijun Yin. Static topographic modeling for facial expression recognition and analysis. Computer Vision and Image Understanding, 2007, 108(1-2): 19-34[J50] Caifeng Shan, Shaogang Gong, Peter W. McOwan. Facial expression recognition based on Local Binary Patterns: A comprehensive study. Image and Vision Computing, 2009, 27(6): 803-816[J51] Xue-wen Chen, Thomas Huang. Facial expression recognition: A clustering-based approach. Pattern Recognition Letters, 2003, 24(9-10): 1295-1302 [J52] Irene Kotsia, Ioan Buciu, Ioannis Pitas. An analysis of facial expression recognition under partial facial image occlusion. Image and Vision Computing, 2008, 26(7): 1052-1067[J53] Shuai Liu, Qiuqi Ruan. Orthogonal Tensor Neighborhood Preserving Embedding for facial expression recognition. Pattern Recognition, 2011, 44(7):1497-1513[J54] Eszter Székely, Henning Tiemeier, Lidia R. Arends, Vincent W.V. Jaddoe, Albert Hofman, Frank C. Verhulst, Catherine M. Herba. Recognition of Facial Expressions of Emotions by 3-Year-Olds. Emotion, 2011, 11(2): 425-435[J55] Kathleen M. Corcoran, Sheila R. Woody, David F. Tolin. Recognition of facial expressions in obsessive–compulsive disorder. Journal of Anxiety Disorders, 2008, 22(1): 56-66[J56] Bouchra Abboud, Franck Davoine, Mô Dang. Facial expression recognition and synthesis based on an appearance model. Signal Processing: Image Communication, 2004, 19(8): 723-740[J57] Teng Sha, Mingli Song, Jiajun Bu, Chun Chen, Dacheng Tao. Feature level analysis for 3D facial expression recognition. Neurocomputing, 2011,74(12-13) :2135-2141[J58] S. Moore, R. Bowden. Local binary patterns for multi-view facial expression recognition . Computer Vision and Image Understanding, 2011, 15(4):541-558[J59] Rui Xiao, Qijun Zhao, David Zhang, Pengfei Shi. Facial expression recognition on multiple manifolds. Pattern Recognition, 2011, 44(1):107-116[J60] Shyi-Chyi Cheng, Ming-Yao Chen, Hong-Yi Chang, Tzu-Chuan Chou. Semantic-based facial expression recognition using analytical hierarchy process. Expert Systems with Applications, 2007, 33(1): 86-95[J71] Carlos E. Thomaz, Duncan F. Gillies, Raul Q. Feitosa. Using mixture covariance matrices to improve face and facial expression recognitions. Pattern Recognition Letters, 2003, 24(13): 2159-2165[J72]Wen G,Bo C,Shan Shi-guang,et al. The CAS-PEAL large-scale Chinese face database and baseline evaluations.IEEE Transactions on Systems,Man and Cybernetics,part A:Systems and Hu-mans,2008,38(1):149-161.[J73] Yongsheng Gao,Leung ,M.K.H. Face recognition using line edge map.IEEE Transactions on Pattern Analysis and Machine Intelligence,2002,24:764-779. [J74] Hanouz M,Kittler J,Kamarainen J K,et al. Feature-based affine-invariant localization of faces.IEEE Transactions on Pat-tern Analysis and Machine Intelligence,2005,27:1490-1495.[J75] WISKOTT L,FELLOUS J M,KRUGER N,et al.Face recognition by elastic bunch graph matching.IEEE Trans on Pattern Analysis and Machine Intelligence,1997,19(7):775-779.[J76] Belhumeur P.N, Hespanha J.P, Kriegman D.J. Eigenfaces vs. fischerfaces: recognition using class specific linear projection.IEEE Trans on Pattern Analysis and Machine Intelligence,1997,15(7):711-720[J77] MA L,KHORASANI K.Facial Expression Recognition Using Constructive Feedforward Neural Networks. IEEE Transactions on Systems, Man and Cybernetics, Part B,2007,34(3):1588-1595.[J78][J79][J80][J81][J82][J83][J84][J85][J86][J87][J88][J89][J90]4、英文学位论文[D1]Hu Yuxiao. Three-dimensional face processing and its applications in biometrics:[Ph.D dissertation]. USA,Urbana-Champaign: University of Illinois, 2008。
CSP共空间模式的介绍
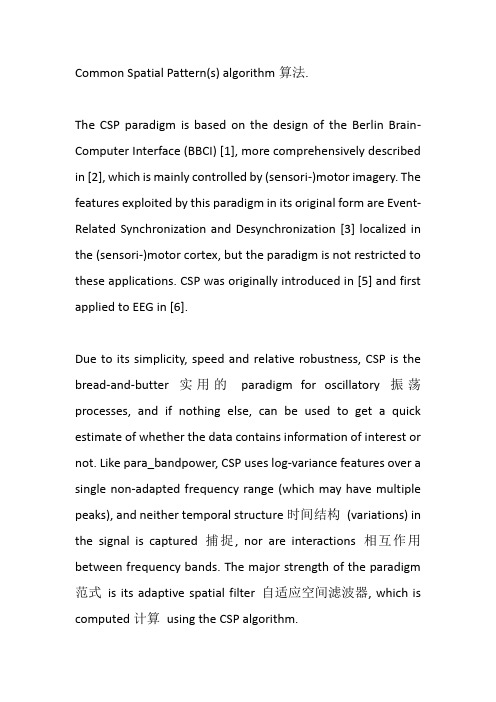
Common Spatial Pattern(s) algorithm算法.The CSP paradigm is based on the design of the Berlin Brain-Computer Interface (BBCI) [1], more comprehensively described in [2], which is mainly controlled by (sensori-)motor imagery. The features exploited by this paradigm in its original form are Event-Related Synchronization and Desynchronization [3] localized in the (sensori-)motor cortex, but the paradigm is not restricted to these applications. CSP was originally introduced in [5] and first applied to EEG in [6].Due to its simplicity, speed and relative robustness, CSP is the bread-and-butter实用的paradigm for oscillatory振荡processes, and if nothing else, can be used to get a quick estimate of whether the data contains information of interest or not. Like para_bandpower, CSP uses log-variance features over a single non-adapted frequency range (which may have multiple peaks), and neither temporal structure时间结构(variations) in the signal is captured捕捉, nor are interactions 相互作用between frequency bands. The major strength of the paradigm 范式is its adaptive spatial filter自适应空间滤波器, which is computed计算using the CSP algorithm.The paradigm is implemented实施as a standard sequence of signal (pre-)processing (spatial/spectral光谱filtering), feature extraction, and machine learning. The first preprocessing预处理step is frequency filtering, followed by an adaptively learned spatial filter (which is the defining propery定义的性能of the paradigm), followed by log-variance feature extraction and finally a (usually simple) machine learning step applied to the log-variance features. The spatial filtering projects the channels of the original signal down to a small set of (usually 4-6) surrogate代理channels, where the (linear) mapping is optimized线性映射被优化such that the variance in these channels is maximally informative w.r.t. to the prediction预测task. The CSP filters can be obtained from the per-class signal covariance matrices协方差矩阵by solving a generalized eigenvalue problem广义特征值问题(of the form [V,D]=eig(Cov1,Cov1+Cov2)). CSP can also be applied to independent components to rate评价their importance or for better artifact 工件robustness鲁棒性. A wide range of classifiers分类can be used with CSP features, the most commonly used one being LDA狄利克雷/一个集合概率模型. There exists a large corpus语料库of CSP variants and extensions变换与拓展, mostly to give better control over spectral filtering, including multiband多波段的CSP (para_multiband_csp), Spectrally Weighted CSP (para_speccsp)光谱加权CSP, Invariant CSP, Common Spatio-Spectral Patterns (CSSP), Common Sparse Spectral Spatial Pattern (CSSSP), Regularized CSP,【不变的CSP,普通的时空光谱模式(CSSP),普通的稀疏频谱空间格局(CSSP),正则CSP】and several others. A more advanced (but also computationally 计算more costly) paradigm范式than CSP is the Dual-Augmented Lagrange Paradigm双增强拉格朗日范式(para_dal/para_dal_hf). The length of the data epoch数据纪元and the choice of a frequency band (defaulting默认to motor imagery time scales时间尺度and frequency ranges) are the parameters参数that are most commonly tuned to调谐the task, both of which can also be found via a small parameter 参数search.Some application areas include detection of major brain rhythm modulations主要的大脑节奏调制(e.g. alpha, beta), for example related to relaxation/stress, aspects of workload, sensori-motor imagery, visual processing vs. idling and other idle-rhythm-related questions, or emotion recognition视觉处理与空转和其他空闲的节奏相关的问题,或情感识别。
基于改进_YOLOv5_的车辆与行人目标识别方法

引用格式:张青春, 吴峥, 周玲, 等. 基于改进 YOLOv5 的车辆与行人目标识别方法[J]. 中国测试,2023, 49(7): 82-88. ZHANG Qingchun, WU Zheng, ZHOU Ling, et al. Vehicle and pedestrian target detection method based on improved YOLOv5[J]. China Measurement & Test, 2023, 49(7): 82-88. DOI: 10.11857/j.issn.1674-5124.2022060008基于改进 YOLOv5 的车辆与行人目标识别方法张青春, 吴 峥, 周 玲, 刘晓洋(淮阴工学院自动化学院,江苏 淮安 223001)摘 要: 为改进无人驾驶技术部署困难问题,该文提出一种基于YOLOv5的目标识别算法。
算法引入Ghost Bottleneck 模块,CBAM 模块,将网络参数压缩,减少模型整体的计算量,同时提升模型推理速度;将原始模型CIoU 损失函数替换为α-IoU 损失函数,使得模型训练过程中更快收敛;用DWConv 层替代原有网络中Neck 部分的Conv 层,可大大降低网络参数数量以及运算成本,同时可提取不同大小的特征信息,提升模型在物体识别方面的鲁棒性。
实验证明,原始模型的参数量为7 095 906,权重文件为14.4 MB ,达到86.11%,检测速度为30.30 f/s;改进后模型参数量缩减为2 546 136,参数量减少了64.12%,权重文件为5.8 MB ,权重文件大小减小了59.72%,达到89.44%,检测速度提升至40.00 f/s ,检测速度提升了32.01%。
将改进后的模型部署至RK3399嵌入式移动端,算法识别速度较原始算法提升了约35.04%。
关键词: 无人驾驶; 目标检测; YOLOv5; Ghost Bottleneck; CBAM; α-IoU 中图分类号: P391.4; TN911.73文献标志码: A文章编号: 1674–5124(2023)07–0082–07Vehicle and pedestrian target detection methodbased on improved YOLOv5ZHANG Qingchun, WU Zheng, ZHOU Ling, LIU Xiaoyang (Huaiyin Institute of Technology, Huaian 223001, China)Abstract : In order to improve the deployment difficulties of unmanned technology, this paper proposes a target detection algorithm based on YOLOv5. The algorithm introduces the Ghost Bottleneck module and the CBAM module. It reduces the overall computational effort of the model by compressing the network parameters and increases the speed of model inference. It replaces the original model CIoU loss function with an α-IoU loss function, so that the model converges faster during training. This paper replaces the Conv layer in the Neck part of the original network with the DWConv layer. It can reduce the number of network parameters as well as the computational cost, while extracting feature information of different sizes. To a certain extent, it can improve the robustness of the model in terms of object recognition. It was demonstrated experimentally that the original model had a parametric count of 7 095 detection speed of 30.30 frames/s. After the model improvement, the number of parameters of the model was reduced to 2 546 136, the amount of parameters was reduced by 64.12%, the weight file was 5.8 MB, the收稿日期: 2022-06-02;收到修改稿日期: 2022-08-23基金项目: 江苏省研究生科研与实践创新计划项目(SJCX21_1507)作者简介: 张青春(1964-),男,江苏盱眙县人,教授,研究方向为智能检测技术、物联网应用技术、移动机器人、虚拟仪器技术等。
辐照灭菌的过程控制指南(美国医疗器械促进协会)AAMI TIR 29-2002

AAMI TIR29:2002技术信息报告辐照灭菌过程控制指南AAMI 美国医疗器械促进协会(Association for the Advancement of MEDICALInstrumentation)AAMI 技术信息报告AAMI TIR29:2002辐照灭菌过程控制指南Approved 16 July 2002 by美国医疗器械促进协会摘要: 本技术信息报告增加了ANSI/AAMI/ISO 11137所界定的光子,电子束灭菌的剂量场的建立和规范,过程确认,和常规控制等辐射灭菌。
尽管轫致辐射的要求相似,但在这项工作开始的时候缺乏关于轫致辐射装置的设计和运行的经验。
所以轫致辐射不包括在此指南之内。
关键词: 辐射剂量场, 过程确认, 日常加工,剂量确认美国医疗器械促进协会技术信息报告信息技术报告是美国医疗器械促进协会标准局的刊物,它是为特殊的医疗技术提供。
提交到信息技术报告的材料需要更多专家的意见,发表的信息也得是用的,因为很多行业都急切需要它。
信息技术报告与标准和操作规程建议,读者应该理解这些文件的不同之处。
标准和工业标准由正式的委员会通过收集所有正确的意见和观点,此过程由美国医疗器械促进协会标准局和美国国际标准机构完成。
信息技术报告作为一个标准审核的过程不是一样。
但是,信息技术报告由技术委员会和美国医疗器械促进协会标准出版社发布。
另外一个不同的地方,尽管标准和信息技术报告都需要定期审查,一个标准必须经过重申,修改,或撤回,通常每五年或十年需要正式的被认可。
对于信息技术报告来说,美国医疗器械促进协会和技术委员会达成一致,规定自出版日期五年后(作为一个周期)进行审查报告是否有用,检查信息是否切题和具有实用性,如果信息没有实用性了,此信息技术报告就被删掉。
信息技术报告肯发展,因为它比标准和操作规程建议能更好响应基础安全和性能问题。
或者说因为达成共识是非常困难甚至不可能。
信息技术报告与标准不同,它允许在技术问题上由不同的观点。
增强Kernel学习优化最大边缘投影的人脸识别

增强Kernel学习优化最大边缘投影的人脸识别郑翔;鲜敏;马勇【期刊名称】《计算机应用与软件》【年(卷),期】2015(000)009【摘要】针对传统的流形学习算法通常只考虑样本类内几何结构而忽略类间判别信息的问题,提出一种基于增强核学习的最大边缘投影(MMP)算法。
首先使用基于增强核学习非线性扩展的MMP采集人脸图像的非线性结构;然后利用核变换技术加强原始输入核函数的判别能力,并且借助于特征向量选择算法改善算法的计算效率;最后,利用基于乘性规则训练的支持向量机完成人脸的识别。
在Yale、ORL、PIE三大通用人脸数据库的组合数据集及AR上的实验验证了该算法的有效性。
实验结果表明,相比其他几种核学习算法,该算法取得了更好的识别效果。
%For the problem that traditional manifold learning methods usually consider intra-class geometry structure only but ignore the discriminative information of inter-classes,we propose an enhanced kernel learning-based maximum margin projection (MMP)algorithm. Firstly,we use MMP nonlinearly extended by enhanced kernel learning to collect the nonlinear structure of face image.Then,we use kernel transformation technology to enhance the discriminant ability of original inputted kernel function,and improve the computation efficiency of the proposed algorithm by feature vector selection algorithm.Finally,we use support vector machine trainedby multiplicative rules to finish the face recognition.The effectiveness ofthe proposed algorithm is verified by the experiments on AR and thecombination datasets of three com-mon face databases Yale,ORL and PIE.Experimental results show that the proposed algorithm has better recognition efficiency comparing with several other advanced approaches based on kernel learning.【总页数】5页(P314-318)【作者】郑翔;鲜敏;马勇【作者单位】四川工程职业技术学院计算机科学技术系四川德阳618000;四川工程职业技术学院计算机科学技术系四川德阳618000;四川工程职业技术学院计算机科学技术系四川德阳618000【正文语种】中文【中图分类】TP391【相关文献】1.基于优化投影矩阵的人脸识别技术研究 [J], 于爱华;白煌;孙斌斌;侯北平2.最大边缘准则图形嵌入在人脸识别中的应用 [J], 宋宇翔;胡伟3.基于加权最大类间边缘准则的人脸识别 [J], 秦春霞;任文杰;贺长伟;王欣4.最小化总投影误差优化一元回归分类的人脸识别 [J], 潘锋5.用于人脸识别的半监督优化局部保持投影 [J], 杨晓梅因版权原因,仅展示原文概要,查看原文内容请购买。
(2008)Dimensionality reduction: A comparative review
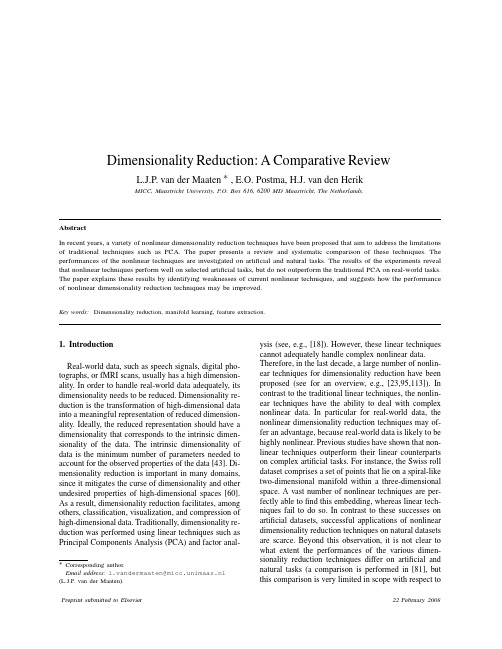
L.J.P. van der Maaten ∗ , E.O. Postma, H.J. van den Herik
MICC, Maastricht University, P.O. Box 616, 6200 MD Maastricht, The Netherlands.
22 February 2008
the number of techniques and tasks that are addressed). Motivated by the lack of a systematic comparison of dimensionality reduction techniques, this paper presents a comparative study of the most important linear dimensionality reduction technique (PCA), and twelve frontranked nonlinear dimensionality reduction techniques. The aims of the paper are (1) to investigate to what extent novel nonlinear dimensionality reduction techniques outperform the traditional PCA on real-world datasets and (2) to identify the inherent weaknesses of the twelve nonlinear dimenisonality reduction techniques. The investigation is performed by both a theoretical and an empirical evaluation of the dimensionality reduction techniques. The identification is performed by a careful analysis of the empirical results on specifically designed artificial datasets and on the real-world datasets. Next to PCA, the paper investigates the following twelve nonlinear techniques: (1) multidimensional scaling, (2) Isomap, (3) Maximum Variance Unfolding, (4) Kernel PCA, (5) diffusion maps, (6) multilayer autoencoders, (7) Locally Linear Embedding, (8) Laplacian Eigenmaps, (9) Hessian LLE, (10) Local Tangent Space Analysis, (11) Locally Linear Coordination, and (12) manifold charting. Although our comparative review includes the most important nonlinear techniques for dimensionality reduction, it is not exhaustive. In the appendix, we list other important (nonlinear) dimensionality reduction techniques that are not included in our comparative review. There, we briefly explain why these techniques are not included. The outline of the remainder of this paper is as follows. In Section 2, we give a formal definition of dimensionality reduction. Section 3 briefly discusses the most important linear technique for dimensionality reduction (PCA). Subsequently, Section 4 describes and discusses the selected twelve nonlinear techniques for dimensionality reduction. Section 5 lists all techniques by theoretical characteristics. Then, in Section 6, we present an empirical comparison of twelve techniques for dimensionality reduction on five artificial datasets and five natural datasets. Section 7 discusses the results of the experiments; moreover, it identifies weaknesses and points of improvement of the selected nonlinear techniques. Section 8 provides our conclusions. Our main conclusion is that the focus of the research community should shift towards nonlocal techniques for dimensionality reduction with objective functions that can be optimized well in practice (such as PCA, Kernel PCA, and autoencoders).
- 1、下载文档前请自行甄别文档内容的完整性,平台不提供额外的编辑、内容补充、找答案等附加服务。
- 2、"仅部分预览"的文档,不可在线预览部分如存在完整性等问题,可反馈申请退款(可完整预览的文档不适用该条件!)。
- 3、如文档侵犯您的权益,请联系客服反馈,我们会尽快为您处理(人工客服工作时间:9:00-18:30)。
1
Introduction
Kernel based methods, including support vector machines [16], regularization networks [5] and Gaussian process [18], have attracted much attention in machine learning. The solid theoretical foundations and good practical performance of the kernel methods make them very popular. However one major drawback of the kernel methods is their scalability. Kernel methods require O(N 2 ) storage and O(N 3 ) operations for direct methods, or O(N 2 ) operations per iteration for iterative methods, which is impractical for large datasets. To deal with this scalability problem, many approaches have been proposed, including the Nystr¨ om method [19], sparse greedy approximation [13, 12], low rank kernel approximation [3] and reduced support vector machines [9]. All these try to find a reduced subset of the original dataset using either random selection or greedy approximation. There is no guarantee on the approximation of the kernel matrix in a deterministic sense. An assumption of these methods is that most eigenvalues of the kernel matrix are zero. This is not always true and its violation results in either performance degradation or negligible reduction in computational time or memory. We explore a deterministic method to speed up kernel machines using the improved fast Gauss transform (IFGT) [20, 21]. The kernel machine is solved iteratively using the conjugate gradient method, where the dominant computation is the matrix-vector product which we accelerate using the IFGT. Rather than approximating the kernel matrix by a low-rank representation, we approximate the matrix-vector product by the improved fast Gauss transform to any desired precision. The total computational and storage costs are of linear order in the size of the dataset. We present the application of the IFGT to kernel methods in the context of the Regularized Least-Squares Classification (RLSC) [11, 10], though the approach is general and can be extended to other kernel methods.
2
Regularized Least-Squares Classification
The RLSC algorithm [11, 10] solves the binary classification problems in Reproducing Kernel Hilbert Space (RKHS) [17]: given N training samples in d-dimensional space xi ∈
Efficient Kernel Machines Using the Improved Fast Gauss Transform
Changjiang Yang, Ramani Duraiswami and Larry Davis Department of Computer Science University of Maryland College Park, MD 20742 {yangcj,ramani,lsd}@
N
fλ (x) =
i=1
ci K (x, xi ).
(2)
If the loss function V is the hinge function, V (y, f ) = (1 − yf )+ , where (τ )+ = τ for τ > 0 and 0 otherwise, then the minimization of (1) leads to the popular Support Vector Machines which can be solved using quadratic programming. If the loss function V is the square-loss function, V (y, f ) = (y − f )2 , the minimization of (1) leads to the so-called Regularized Least-Squares Classification which requires only the solution of a linear system. The algorithm has been rediscovered several times and has many different names [11, 10, 4, 15]. In this paper, we stick to the term “RLSC” for consistency. It has been shown in [11, 4] that RLSC achieves comparable accuracy in binary classification problems to the popular SVMs. If we substitute (2) into (1), and denote c = [c1 , . . . , cN ]T , K = K (xi , xj ), we can find the solution of (1) by solving the linear system (K + λ′ I )c = y where λ′ = λN , I is the identity matrix, and y = [y1 , . . . , yN ]T . There are many choices for the kernel function K . The Gaussian is a good kernel for classification and is used in many applications. If a Gaussian kernel is applied, as shown in [10], the classification problem can be solved by the solution of a linear system, i.e., Regularized Least-Squares Classification. A direct solution of the linear system will require O(N 3 ) computation and O(N 2 ) storage, which is impractical even for problems of moderate size. Algorithm 1 Regularized Least-Squares Classification Require: Training dataset SN = (xi , yi )N i=1 . ′ 2 2 1. Choose the Gaussian kernel: K (x, x′ ) = e− x−x /σ . N 2. Find the solution as f (x) = i=1 ci K (x, xi ), where c satisfies the linear system (3). 3. Solve the linear system (3). An effective way to solve the large-scale linear system (3) is to use iterative methods. Since the matrix K is symmetric, we consider the well-known conjugate gradient method. The conjugate gradient method solves the linear system (3) by iteratively performing the matrix-vector multiplication K c. If rank (K ) = r, then the conjugate gradient algorithm converges in at most r +1 steps. Only one matrix-vector multiplication and 10N arithmetic operations are required per iteration. Only four N -vectors are required for storage. So the computational complexity is O(N 2 ) for low-rank K and the storage requirement is O(N 2 ). While this represents an improvement for most problems, the rank of the matrix may not be small, and moreover the quadratic storage and computational complexity are still too high for large datasets. In the following sections, we present an algorithm to reduce the complexity to linear order. (3)