ADVANCED SPECTRAL METHODS FOR CLIMATIC
基于导数光谱的小麦冠层叶片含水量反演汇编
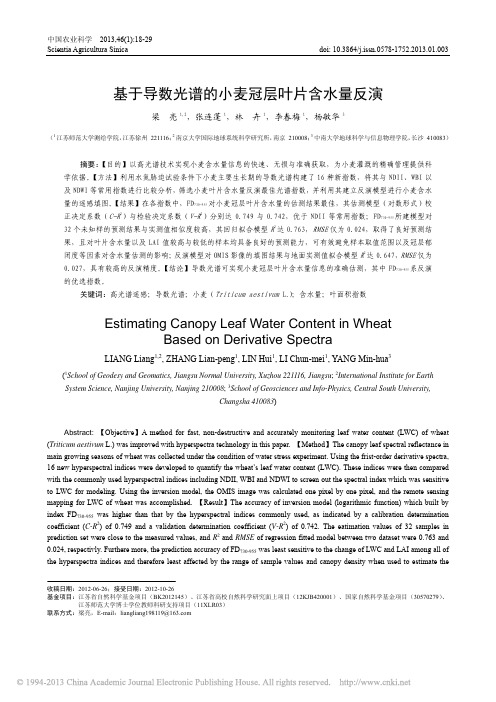
中国农业科学 2013,46(1):18-29Scientia Agricultura Sinica doi: 10.3864/j.issn.0578-1752.2013.01.003基于导数光谱的小麦冠层叶片含水量反演梁 亮1,2,张连蓬1,林 卉1,李春梅1,杨敏华3(1江苏师范大学测绘学院,江苏徐州 221116;2南京大学国际地球系统科学研究所,南京 210008;3中南大学地球科学与信息物理学院,长沙 410083)摘要:【目的】以高光谱技术实现小麦含水量信息的快速、无损与准确获取,为小麦灌溉的精确管理提供科学依据。
【方法】利用水氮胁迫试验条件下小麦主要生长期的导数光谱构建了16种新指数,将其与NDII、WBI以及NDWI等常用指数进行比较分析,筛选小麦叶片含水量反演最佳光谱指数,并利用其建立反演模型进行小麦含水量的遥感填图。
【结果】在各指数中,FD730-955对小麦冠层叶片含水量的估测结果最佳,其估测模型(对数形式)校正决定系数(C-R2)与检验决定系数(V-R2)分别达0.749与0.742,优于NDII等常用指数;FD730-955所建模型对32个未知样的预测结果与实测值相似度较高,其回归拟合模型R2达0.763,RMSE仅为0.024,取得了良好预测结果,且对叶片含水量以及LAI值较高与较低的样本均具备良好的预测能力,可有效避免样本取值范围以及冠层郁闭度等因素对含水量估测的影响;反演模型对OMIS影像的填图结果与地面实测值拟合模型R2达0.647,RMSE仅为0.027,具有较高的反演精度。
【结论】导数光谱可实现小麦冠层叶片含水量信息的准确估测,其中FD730-955系反演的优选指数。
关键词:高光谱遥感;导数光谱;小麦(Triticum aestivum L.);含水量;叶面积指数Estimating Canopy Leaf Water Content in WheatBased on Derivative SpectraLIANG Liang1,2, ZHANG Lian-peng1, LIN Hui1, LI Chun-mei1, YANG Min-hua3(1School of Geodesy and Geomatics, Jiangsu Normal University, Xuzhou 221116, Jiangsu; 2International Institute for EarthSystem Science, Nanjing University, Nanjing 210008; 3School of Geosciences and Info-Physics, Central South University,Changsha 410083)Abstract: 【Objective】A method for fast, non-destructive and accurately monitoring leaf water content (LWC) of wheat(Triticum aestivum L.) was improved with hyperspectra technology in this paper. 【Method】The canopy leaf spectral reflectance inmain growing seasons of wheat was collected under the condition of water stress experiment. Using the frist-order derivative spectra,16 new hyperspectral indices were developed to quantify the wheat’s leaf water content (LWC). These indices were then comparedwith the commonly used hyperspectral indices including NDII, WBI and NDWI to screen out the spectral index which was sensitiveto LWC for modeling. Using the inversion model, the OMIS image was calculated one pixel by one pixel, and the remote sensingmapping for LWC of wheat was accomplished. 【Result】The accuracy of inversion model (logarithmic function) which built byindex FD730-955 was higher than that by the hyperspectral indices commonly used, as indicated by a calibration determinationcoefficient (C-R2) of 0.749 and a validation determination coefficient (V-R2) of 0.742. The eatimation values of 32 samples inprediction set were close to the measured values, and R2 and RMSE of regression fitted model between two dataset were 0.763 and0.024, respectivly. Furthere more, the prediction accuracy of FD730-955 was least sensitive to the change of LWC and LAI among all ofthe hyperspectra indices and therefore least affected by the range of sample values and canopy density when used to estimate the收稿日期:2012-06-26;接受日期:2012-10-26基金项目:江苏省自然科学基金项目(BK2012145)、江苏省高校自然科学研究面上项目(12KJB420001)、国家自然科学基金项目(30570279)、江苏师范大学博士学位教师科研支持项目(11XLR03)联系方式:梁亮,E-mail:liangliang198119@1期梁亮等:基于导数光谱的小麦冠层叶片含水量反演 19LWC of wheat. The R2 and RMSE of the fitting model for the inversion and measured values were 0.635 and 0.027, respectively, andindicated the similarity between the inversion and measured value was high. 【Conclusion】It is possible to eatimate LWC of wheatby derivative hyperspectra with a high accuracy, and FD730-955 is an optimal index for modeling.Key words: hyperspectra remote sensing; derivative spectrum; wheat (Triticum aestivum L.); leaf water content (LWC); leafarea index (LAI)0 引言【研究意义】叶片含水量是植株水分状况良好的度量和诊断指标,实时、快速地获取叶片含水量信息是进行作物长势监测以及灌溉调控的前提[1]。
bed

Chebyshev super spectral viscosity method for a fluidizedbed modelScott A.SarraDepartment of Mathematics,Marshall University,One John Marshall Drive,Huntington,WV 25755-2560,USAReceived 7May 2002;received in revised form 30October 2002;accepted 16December 2002AbstractA Chebyshev super spectral viscosity method and operator splitting are used to solve a hyperbolic system of con-servation laws with a source term modeling a fluidized bed.The fluidized bed displays a slugging behavior which corresponds to shocks in the solution.A modified Gegenbauer postprocessing procedure is used to obtain a solution which is free of oscillations caused by the Gibbs–Wilbraham phenomenon in the spectral viscosity solution.Conser-vation is maintained by working with unphysical negative particle concentrations.Ó2003Elsevier Science B.V.All rights reserved.Keywords:Chebyshev collocation;Super spectral viscosity;Pseudospectral;Gibbs–Wilbraham phenomenon;Edge detection;Gegenbauer postprocessing1.IntroductionFluidized beds are used in the chemical and fossil fuel processing industries to mix particulate solids and fluids (gases or liquids).A typical fluidized bed consists of a vertically oriented chamber,a bed of par-ticulate solids,and a fluid flow distributor at the bottom the chamber.The fluid flows upward through the particles creating a force that counteracts gravity at which time a state of minimum fluidization is reached.Stronger gas inflows (more than is necessary to maintain minimum fluidization)lead to pockets of gas,or equivalently low particle concentrations,resembling bubbles in a liquid traveling upward through the particles.Each rising bubble pushes a large amount of mass in front of it.Particles move downward through and around the rising bubble until it reaches the top of the bed.A settled bed is reestablished and the cycle repeats.Each set of upward moving particles is referred to as a slug.In this paper we consider only one-dimensional flow.Physically,this corresponds to flow in a narrow diameter fluidized bed.The fluidized bed model was originally solved numerically in [6]by finite difference methods.An exact solution to the homogeneous system with Riemann initial conditions has beendevel-Journal of Computational Physics 186(2003)630–/locate/jcpE-mail address:scott@.0021-9991/03/$-see front matter Ó2003Elsevier Science B.V.All rights reserved.doi:10.1016/S0021-9991(03)00089-5oped in[7].Thefluidized bed model can be put in the form of system of conservation laws with a source term asu tþfðuÞx¼bðuÞ:ð1ÞSpectral viscosity methods have been successfully applied to homogeneous systems of conservation laws. We use operator splitting to extend the methods to nonhomogeneous systems of conservation laws.If discontinuities are present in the solutions,the spectral viscosity approximations will be contaminated by the Gibbs–Wilbraham phenomenon,but the spectral viscosity solution may be postprocessed to obtain a better approximation.While the spectral viscosity is applied to the solution at every time level,postpro-cessing is only done at times for which a‘‘clean’’solution is desired.Several methods exist for postpro-cessing spectral approximations.They include spectral mollification[12,25,26,36]methods which involve using a two-parameterfilter,the Gegenbauer Reconstruction Procedure(GRP),and a recently developed Fourier–Pad e-based algorithm[9].Spectral mollification is a fairly robust method which may be used with or without the knowledge of edge locations.However,it will only recover spectral accuracy up to within a neighborhood of discontinuity locations.The GRP is capable of recovering spectral accuracy at every point,even at the locations of the discontinuities.In this paper,all numerical examples have been postprocessed using the GRP.One of our goals was to examine if the GRP,which has shown great promise on some simple examples,could be used to successfully postprocess PDE solutions which were either more detailed than piecewise linear or if the method could be used to postprocess solutions containing varying subintervals of detail.The solutions in the previous ap-plications[15,32]consisted of homogeneous features throughout the computational domain which allowed the parameters of the postprocessing method to be chosen globally.Thefluidized bed solutions contain features of varying detail throughout the computational domain and a different strategy must be used to choose the postprocessing parameters.Additionally,we examine what remains to be done if the GRP is to be used as a‘‘black box’’postprocessing method for spectral approximations.This paper is organized as follows:In Section2,the Chebyshev collocation method and super spectral viscosity methods are reviewed.Section3summarizes a method to locate edges in the spectral viscosity approximations.Edge locations will be necessary to apply the postprocessing procedure.Section4describes the GRP for non-periodic functions.Section5describes thefluidized bed model.Numerical results are presented in Section6.2.Chebyshev super spectral viscosity methodThe standard collocation points for a Chebyshev collocation(pseudospectral)method are usually de-fined byx j¼Àcosp jN;j¼0;1;...;N:ð2ÞThese points are extrema of the N th order Chebyshev polynomial,T kðxÞ¼cosðk arccosðxÞÞ:ð3ÞThe points are often labeled the Chebyshev–Gauss–Lobatto(CGL)points,a name which alludes to the points role in certain quadrature formulas.The CGL points cluster quadratically around the endpoints and are less densely distributed in the interior of the domain.The Chebyshev collocation method is based on assuming that an unknown PDE solution,u,can be represented by a global,interpolating,Chebyshev partial sum,S.A.Sarra/Journal of Computational Physics186(2003)630–651631u NðxÞ¼X Nn¼0a n T nðxÞ:ð4ÞThe discrete Chebyshev coefficients,a n,are defined bya n¼2N1c nX Nn¼0uðx jÞT nðx jÞc j;where c j¼2when j¼0;N;1otherwise:ð5ÞDerivatives of u at the collocation points are approximated by the derivative of the interpolating polynomial evaluated at the collocation points.Thefirst derivative,for example,is defined by,d u d x ¼X Nn¼0að1ÞnT nðxÞ:ð6ÞSince að1ÞNþ1¼0and að1ÞN¼0,the non-zero derivative coefficients can be computed in decreasing order by therecurrence relation:c n að1Þn ¼að1Þnþ2þ2ðnþ1Þa nþ1;n¼NÀ1;...;1;0:ð7ÞThe transform pair given by Eqs.(4)or(6)and(5)can be efficiently computed by a fast cosine transform. Equivalently,the interpolating polynomial and its derivatives can be computed in physical space using matrix multiplication[4].Special properties of the Chebyshev basis allow for differentiation via parity matrix multiplication[3](even–odd decomposition[33]),which can be performed by using slightly more than half as manyfloating point operations as standard matrix multiplication.More detailed information may be found in the standard references[4,10,11,18,19,37].After the spectral evaluation of spatial derivatives,the system of ordinary differential equationsd ud t¼Fðu;tÞresults,where u is the vector containing the unknown PDE solution at the collocation points.The system is typically integrated by a second,third,or fourth-order explicit Runge–Kutta method to advance the so-lution in time.A coordinate transformation may be necessary either to map a computational interval to½a;b from the interval½À1;1 ,or to redistribute the collocation points within an interval for the purpose of giving high resolution to regions of very rapid change.Popular maps used to redistribute the CGL points(2)are the Kosloff/Tal-Ezer map[27]x¼gðn;cÞ¼arcsinðcnÞarcsinðcÞ;ð8Þthe center map[1]x¼gðn;cÞ¼ð1:0ÀcÞn3þcn;ð9Þand the two parameter tangent map[2]x¼gðn;c;lÞ¼x0þtanðdnþxÞc;ð10Þwhere j¼arctanðcð1ÀlÞÞ,c¼arctanðcð1þlÞÞ,d¼0:5ðjþcÞ,x¼0:5ðjÀcÞ,and x0¼À1þ2ðlÀaÞ=ðbÀaÞ.632S.A.Sarra/Journal of Computational Physics186(2003)630–651If n denotes the original variable and x¼gðnÞthe new variable,then after a change of variable is performed Eq.(1)becomesu tþ1g0ðnÞfðuÞx¼bðuÞ:ð11ÞIf the PDE solution contains shocks,the spectral collocation method will not converge to the correct entropy solution[35].In this case,a spectrally small viscosity term must be added in order to stabilize the approximation and ensure convergence to the entropy solution.This can be done without sacrificing spectral accuracy and can be accomplished in several different ways,with each way being labeled a par-ticular type of spectral viscosity method.We have used the super spectral viscosity(SSV)method of[28], which for a conservation law in one space dimension,can be stated aso o t u Nþoo xfðu NÞ¼eðÀ1Þsþ1Q2s u N;ð12Þwhere the viscosity operator is given byQ¼ffiffiffiffiffiffiffiffiffiffiffiffiffi1Àx2p oo x:ð13ÞIt was shown in[28]that if e¼CN1À2s,with the parameter C chosen large enough to ensure stability and such that06C6N1=2,and with the parameter s chosen such that s6lnðNÞand allowing s to grow with N, that bounded solutions of(12)will converge to the correct entropy solution in the case bðuÞ¼0.Except for the ranges mentioned in order to ensure convergence to the entropy solution,the parameters s and C are problem dependent,depending mainly on the strength of the shocks involved.A direct implementation of(12)amounts to adding2s spatial derivatives to the equation.This would introduce additional stiffness which would severely limit the stable time step and increase the computational work involved by requiring the computation of higher order derivatives.Hence,the practical implementation of the SSV method is an important issue.The efficient implementation offthe SSV method wasfirst addressed in[8],where the authors recognized that the SSV method could be implemented as a spectralfilter.This fact is based on the examination of the viscosity operator Q2applied to the Chebyshev polynomial(3),T kðxÞ.Q2T kðxÞ¼ffiffiffiffiffiffiffiffiffiffiffiffiffi1Àx2p oo xffiffiffiffiffiffiffiffiffiffiffiffiffi1Àx2p oo xT kðxÞ¼Àk2T kðxÞ:ð14ÞAs a result of applying the viscosity operator to the Chebyshev polynomials,it can be noticed that the Chebyshev polynomials are the eigenfunctions of the operator Q2with eigenvalues k2.Expanding the viscosity term,which is the right-hand side of(12),we notice thateðÀ1Þsþ1Q2s u N¼ÀCNX Nk¼0kN2sa kðtÞT kðxÞ:ð15ÞIf we implement the SSV method via time splitting where in thefirst step we solveo o t u Nþoo xfðu NÞ¼0ð16Þand in the second step we solveo o t u N¼eðÀ1Þsþ1Q2s u N;ð17ÞS.A.Sarra/Journal of Computational Physics186(2003)630–651633the second equation,(17),in the split step can be written aso o tX Nk¼0a kðtÞT kðxÞ"#¼ÀCNX Nk¼0kN2sa kðtÞT kðxÞ;which can be solved analytically.Over one time step,the analytical solution modifies the Chebyshev co-efficients asa kðtþD tÞ¼a kðtÞexpðÀCN D tðk=NÞ2sÞ:Thus,the exact solution of the SSV split step can be written as thefiltered partial sumu NðxÞ¼X Nk¼0rkNa kðtÞT kðxÞ;ð18Þwherer k N¼expÀakNis an exponentialfilter of strength a and order b as described in[38].The Chebyshev SSV method is seen to be equivalent to applying the exponentialfilter with b¼2s and a¼CN D t.The method can be implemented with little additional cost.It should be stressed that while the SSV method is being implemented via the exponentialfiltering framework,that it is not a b th orderfilter as it does not meet the requirements set forth in[38].The amount of damping of the high modes is significantly less with the SSV method than with the application of a b th order exponentialfilter.An application of a b th order exponentialfilter typically takes a¼Àln e where e is machine zero(on a32-bit machine using double precisionfloating point operations, e¼2À52and lnðeÞ’À36:0437).Fig.1compares two exponentialfilters of different orders with an appli-cation of thefilter with the parameters set as a¼0:032and b¼4,which are possible settings that may be used if thefiltering framework is used to implement the SSV method.634S.A.Sarra/Journal of Computational Physics186(2003)630–6513.Edge detectionThe GRP recovers spectral accuracy up to the discontinuity points in each smooth subinterval of a piecewise analytic function.Thus,the GRP needs the exact location of discontinuities,or edges,in the function.If a PDE solution is being postprocessed and the solution contains rarefaction waves,disconti-nuities in the first derivative of the function will exist and need to be located as well.The method used to find the edges originated in [14]for periodic and non-periodic functions.The method is specialized to approximations of functions by Chebyshev methods and is summarized below.Denote the location of discontinuities as a j .Let½f ðx Þ:¼f ðx þÞÀf ðx ÀÞdenote a local jump in the function and defineue ðx Þ¼p ffiffiffiffiffiffiffiffiffiffiffiffiffi1Àx 2p N X N k ¼0a k d d x T k ðx Þ;ð19Þwhered d x T k ðx Þ¼k sin ðk arccos ðx ÞÞffiffiffiffiffiffiffiffiffiffiffiffiffi1Àx 2p :Essentially,we are looking at the derivative of the spectral projection of the numerical solution to de-termine the location of the discontinuities.The series ue ðx Þhas the convergence propertiesue ðx Þ!O ð1=N Þwhen x ¼a j ;½f ða j Þwhen x ¼a j :The series converges to both the height and direction of the jump at the location of a discontinuity.However,for the GRP,we only need the locations and magnitudes of the jumps,not the directions.While a graphical examination of the series ue ðx Þverifies that the series does have the desired convergence prop-erties,an additional step is needed to numerically pinpoint the location of the discontinuities.For that purpose,make a non-linear enhancement to the edge series asun ðx Þ¼N Q =2½ue ðx Þ Q :The values,un ðx Þ,will serve to amplify the separation of scales which has taken place in (19).The series has the convergence propertiesun ðx Þ!O ðN ÀQ =2Þwhen x ¼a j ;N Q =2½½f ða j Þ Q when x ¼a j :By choosing Q >1we enhance the separation between the O ð½1=N Q =2Þpoints of smoothness and the O ðN Q =2Þpoints of discontinuity.The parameter J ,whose value will be problem dependent,is a critical threshold value.Finally,redefine ue ðx Þasue ðx Þ¼j ue ðx Þj if un ðx Þ>J ;0otherwise :With Q large enough,one ends up with an edge detector ue ðx Þ¼0at all x except at the discontinuities x ¼a j .Only those edges with amplitude larger than J 1=Q ffiffiffiffiffiffiffiffiffi1=N p will be detected.S.A.Sarra /Journal of Computational Physics 186(2003)630–651635Often the series ue is slow to converge in the area of a discontinuity and the nonlinear enhancement has difficulty pinpointing the exact location of the edge.If an additional parameter,g,is added to the procedure this problem can be overcome in a simple manner.The parameter specifies that only one edge may be located in the intervalðx½iÀg ;x½iþg Þ,i¼0;...;N,with appropriate one sided intervals being considered near boundaries.The correct edge will be the maximum of ue in this subinterval.The value of g is problem-dependent and is best chosen after the edge detection procedure has been applied once.The edge detection parameters J,Q,and g,are all problem-dependent.Various combinations of the parameters may be used to successfully locate edges represented by jumps of a magnitude in a certain range.4.Gegenbauer reconstructionThe truncation error decays exponentially as N increases when spectral methods are used to approximate smooth functions.However,the situation changes when the function is discontinuous as the spectral ap-proximation no longer converges in the maximum norm.This is known as the Gibbs–Wilbraham phe-nomenon.Several methods exist for removing or reducing the effects of the Gibbs–Wilbraham phenomenon from spectral approximations.Most however,such as spectral mollification[17,36],only recover spectral accuracy up to within a neighborhood of each discontinuity.To date,the most powerful postprocessing method seems to be the GRP which is capable of recovering spectral accuracy up to and including at the location of discontinuities.Although the GRP has been shown to produce remarkable results on some simple problem,the method lacks robustness due to the fact that two parameters,for which an optimal choice for is currently not known,must be specified.The GRP was developed in[20–24]for the purpose of recovering exponential accuracy at all points, including at the discontinuities themselves,from the knowledge of a spectral partial sum of a discontinuous, but piecewise analytic function.While the SSV solution serves as a highly accurate approximation to the exact spectral partial sum,only partial theoretical justification can be found concerning using the GRP as a postprocessing method for the SSV solution.However,numerical results indicate that spectral accuracy can be achieved by applying the GRP to the SSV solution of homogeneous systems of conservation laws[12,15]. The same can be said about the edge detection method,as the theoretical results are limited to locating the jump discontinuities of a piecewise smooth function uðxÞ.However,numerical evidence also advocates applying the edge detection method to the SSV solution.The GRP works by expanding the function in another basis,the Gibbs complementary basis,via knowledge of the known Chebyshev coefficients and the location of discontinuities.The Chebyshev partial sums are projected onto a space spanned by the Gegenbauer polynomials.The associated weight functions increasingly emphasize information away from the discontinuities as the number of included modes grow. The approximation converges exponentially in the new basis even though it only converged very slowly in the original basis due to the Gibbs–Wilbraham phenomenon.The choice of a Gibbs complementary basis isthe Ultraspherical or Gegenbauer polynomials,C kn .The Gegenbauer polynomials are orthogonal polyno-mials of order n which satisfyZ1À1ð1Àx2ÞkÀ1=2C kkðxÞC knðxÞd x¼h kn;k¼n;0;k¼n;where(for k P0)h k n ¼p1=2C knð1ÞCðkþð1=2ÞÞCðkÞðnþkÞ636S.A.Sarra/Journal of Computational Physics186(2003)630–651withC k n ð1Þ¼Cðnþ2kÞn!Cð2kÞ:Whether the Gegenbauer basis is the optimal choice as the Gibbs complementary basis for the Chebyshev basis remains an open question.In other words,it may be possible to construct another basis in which the slowing converging Chebyshev approximation could be expanded in to obtain an approximation with better convergence properties than those of the Gegenbauer approximation.However,it is shown in[24] that the Gegenbauer basis is a Gibbs complementary basis for the Chebyshev basis.The Gegenbauer expansion of a function uðxÞ;x2½À1;1 isuðxÞ¼X1l¼0b f klC klðxÞ;where the continuous Gegenbauer coefficients,b f k l,of uðxÞareb f k l ¼1h klZ1À1ð1Àx2ÞkÀ1=2C klðxÞuðxÞd x:ð20ÞSince we do not know the function uðxÞ,implementing the GRP requires obtaining an exponentiallyaccurate approximation,b g kl ,to thefirst m coefficients b f k l in the Gegenbauer expansion from thefirst Nþ1Chebyshev coefficients of uðxÞ.The approximate Gegenbauer coefficients are defined as the integralb g k l¼1hl Z1À1ð1Àx2ÞkÀ1=2C klðxÞu NðxÞd x;ð21Þwhere u N is the Chebyshev partial sum(4).The integral should be evaluated by Gauss–Lobatto quadraturein order to insure sufficient accuracy.The coefficients b g kl are now used in the partial Gegenbauer sum toapproximate the original function asuðxÞ%u km ðxÞ¼X ml¼0b g k l C k lðxÞ:In practice,there will be discontinuities in the interval½À1;1 and the reconstruction must be done on each subinterval½a;b in which the solution remains smooth.To accomplish the reconstruction on each subinterval,define a local variable for each subinterval as xðnÞ¼ nþd where ¼ðbÀaÞ=2,d¼ðbþaÞ=2 and n j¼cosðp j=NÞ:The reconstruction in each subinterval is then accomplished byu k; m ð nþdÞ¼X ml¼0b g k ðlÞC k lðnÞ;whereb g k ðlÞ¼1hl ZÀ11ð1Àn2ÞkÀ1=2C klðnÞu Nð nþdÞd n:Notice that we have used collocation points on the entire interval½À1;1 to build the approximation in ½a;b .This is referred to as a global–local approach[22].The global–local approach seems to be best when postprocessing PDE solutions where u N is obtained from the time evolution of the PDE solution.The point values uðx iÞmay not be accurate,but the global interpolating polynomial u NðxÞis accurate.S.A.Sarra/Journal of Computational Physics186(2003)630–651637In order to show that the GRP yields uniform exponential accuracy for the approximation,it is nec-essary to select k and m such that k¼m¼b N,where b<2e=ð27ð1þ1=2pÞÞ,and p is the distance from ½À1;1 to the nearest singularity in the complex plane,in each subinterval where the function being re-constructed is assumed to be analytic[24].It is not necessary,and usually not advisable,to choose k¼m.In practice,we are often more concerned with obtaining results for afixed N,rather than achieving an ex-ponential convergence rate.If the function to be postprocessed consists homogeneous features,the reconstruction parameters can be successfully chosen as k¼k k N and m¼k m N for each subinterval where k k and k m are user chosen, globally applied parameters.We refer to this strategy as the global approach.In all previous applications of the GRP in the literature,the method was applied to such functions and it was possible to chose the parameters in this way[12,13].However,in problems with solutions with varying detail throughout the computational domain,the reconstruction parameters may need to be chosen independently in each subinterval[30].We refer to this strategy as the local approach.To date there is no known method to choose optimal values of the reconstruction parameters m and k.The parameters remain very problem dependent.Work is under way on choosing optimal parameters and results will be reported in a future paper.5.Fluidized bed equationsThe variable x denotes the vertical height in the bed.Let aðx;tÞdenote the concentration of particles by volume,vðx;tÞthe particle velocity,and mðx;tÞ¼aðx;tÞvðx;tÞthe particle momentum.The parameter a0is the concentration of particles at equilibrium(when v¼0)and a p is the packing concentration which sets an upper limit for a where a2ð0;1Þ.The parameter a0u denotes the particle concentration corresponding to the critical state dividing linearly stable and unstable states(the particle concentration at minimumflu-idization).The constant s¼3:5ð1Àa0uÞ2:5ða pÀa0uÞis related to the linear stability of the equilibrium solutions which correspond to states of uniformfluidization.The model can be put in the form of a system of conservation laws with a source term asa tþm x¼0;ð22Þm tþðm2=aþFðaÞÞx¼bða;mÞ;ð23ÞwhereFðaÞ¼s2aþs2a2paÀa pþ2s2a p lnðj aÀa p jÞ:The function bða;mÞin the source term is given bybða;mÞ¼Àaþa JÀm ð1ÀaÞ;where J¼ð1Àa0Þ3:5represents the total volumetricflux through the bed.Increasing J(or decreasing a0) corresponds to turning up the inflowing gas.Values a0<a0u correspond to large gasfluxes and have been shown to produce unstable states corresponding to slug-like solutions.Values a0>a0u give rise to stable states.From a mathematical point of view,the non-homogeneous system of conservation laws coincides with the Euler equations for an isentropic gasflow,subject to volumetric forces.The variables a,v,and FðaÞplay the role of density,velocity,and pressure respectively,in the Euler equations.638S.A.Sarra/Journal of Computational Physics186(2003)630–651S.A.Sarra/Journal of Computational Physics186(2003)630–6516395.1.Vacuums and unphysical particle concentrationsA vacuum is said to exist at a collocation point if the particle concentration is zero.Numerically,we will assume that a vacuum exists at a grid point if the concentration is either zero or it is very small (j a j<thres).The system becomes meaningless at vacuum points as m2=a is either undefinedða¼0Þor produces unrealistic valuesðj a j<thresÞ.At each vacuum point encountered in the numerical method,the corresponding values of v,and therefore m,are set equal to zero at that collocation point rather than using the spurious valueðj a j<thresÞor NaN valueða¼0Þ.Values of a such that j a j<thres are retained and not set to zero.Stable approximations by the spectral method always produced a<a p.In the spectral method,a must be allowed to take negative values even though a negative concentration in not physically meaningful,as this information is used in the GRP to postprocess the result.When it was attempted to artificially force the spectral method to work only with a>0,the quality of the postpro-cessed solution was adversely affected.More importantly,even though the spectral collocation method is conservative,if for a<0,a was redefined as a¼0,the conservative properties of the method were destroyed and the method started producing mass.If the values of a were allowed to be negative,the method was conservative and mass was preserved to as many as six decimal places.In all reported re-sults,the parameter thres was taken to be thres¼0:001.After postprocessing the solution,all concen-trations are such that a P0.6.Numerical resultsAll examples were postprocessed using the spectral signal processing[31]suite.In the reported results we have used a0u¼0:55and a p¼0:6.6.1.Homogeneous systemThefirst two problems solve the homogeneous system with Riemann initial data so that the Chebyshev SSV method with GRP postprocessing may be validated against an exact solution.Ourfirst example consists of a left-moving shock wave and a right moving rarefaction wave.The initial conditions are vðx;0Þ¼0for all x in a domain of½À0:2;0:2 and aðx;0Þ¼0:3if x<0and aðx;0Þ¼0:55if x P0.Fig.2shows the solution advanced to time t¼0:5with a fourth-order Runge–Kutta method.The grid consists of64points distributed by map(9)with c¼0:25.The use of the coordinate map has the effect of placing more points in the center of the domain.The SSV parameters used were C¼1and s¼4which produced a viscosity parameter of e¼CN1À2s¼2:27EÀ13(or a¼CN D t¼0:16and b¼8in the expo-nentialfilter).The rarefaction wave is characterized by the solution having a discontinuousfirst derivative,thus edge detection must be applied to thefirst derivative of the solution in addition to the solution itself.The edge detection procedure with Q¼1and J¼1locates jumps of magnitude greater than0.125.With these settings,the edge detection procedure locates edges in the function and thefirst derivative of the function at x¼À0:0331,x¼0:0331,and x¼0:1374.We were unable to get good postprocessed results by specifying the reconstruction parameters globally through the parameters k k and k m.Global parameter specification failed due to the solution containing three intervals of piecewise constant values and a fourth intervalð0:033;0:1374Þconsisting of a function requiring different reconstruction parameters.Good results were obtained by specifying the GRP parameters locally in each smooth subinterval as listed in Table1.The postprocessed solution in Fig.3.。
EN 61853-1-2011 PV module performance testing and energy rating - Part 1

ICS 27.160
EN 61853-1
March 2011
English version
Photovoltaic (PV) module performance testing and energy rating Part 1: Irradiance and temperature performance measurements and power rating (IEC 61853-1:2011)
-2-
Foreword
The text of document 82/613/FDIS, future edition 1 of IEC 61853-1, prepared by IEC TC 82, Solar photovoltaic energy systems, was submitted to the IEC-CENELEC parallel vote and was approved by CENELEC as EN 61853-1 on 2011-03-02. Attention is drawn to the possibility that some of the elements of this document may be the subject of patent rights. CEN and CENELEC shall not be held responsible for identifying any or all such patent rights. The following dates were fixed: – latest date by which the EN has to be implemented at national level by publication of an identical national standard or by endorsement – latest date by which the national standards conflicting with the EN have to be withdrawn Annex ZA has been added by CENELEC. __________
ICESat-2_数据背景光子特性及滤波方法研究

第 31 卷第 5 期2023 年 3 月Vol.31 No.5Mar. 2023光学精密工程Optics and Precision EngineeringICESat-2数据背景光子特性及滤波方法研究谢欢1,2*,黄佩琪1,徐琪1,叶丹1,孙媛1,栾奎峰3,刘世杰1,2,童小华1,2(1.同济大学测绘与地理信息学院,上海 200092;2.上海市航天测绘遥感与空间探测重点实验室,上海 200092;3.上海海洋大学海洋科学学院,上海 201306)摘要:ICESat-2(Ice, Cloud and Land Elevation Satellite-2)是世界首颗采用光子计数模式的激光测高卫星,可快速获得高精度、大尺度地面三维数据。
光子探测机制使得数据中除了地面信号外,还包含大气散射等背景信号,需要通过滤波才能获得地形等信息。
为分析ICESat-2背景和信号光子的分布特点及点云滤波算法的效果和适用性,本文首先选取了六种地表覆盖类型(城市、海冰、沙漠、植被、海洋及冰盖/冰川)及不同观测条件的数据,对其背景光子率进行统计分析。
分析结果表明:白天观测数据的背景光子率平均为106(点/秒)数量级,远高于夜晚观测数据的背景光子率——104(点/秒)数量级,弱波束的背景光子率与强波束背景光子率相当,六种地表覆盖类型中,冰盖/冰川的背景光子率最高。
然后,根据统计结果筛选出21组测高数据,并选取七种具有代表性的点云滤波对其进行去噪实验,分析精度后得出结论:改进局部密度法的去噪效果最佳,算法召回率、精准度和F值均大于0.90,算法较为稳定。
最后,对所选取各滤波算法的精度、特点与适用性等性质进行了总结与分析,可为后续该数据的使用和滤波算法的选择提供参考。
关键词:ICESat-2;激光测高;光子计数激光雷达;点云去噪;背景光子率中图分类号:TP391.4 文献标识码:A doi:10.37188/OPE.20233105.0631Research on background photon characteristics and filteringmethods for ICESat-2 dataXIE Huan1,2*,HUANG Peiqi1,XU Qi1,YE Dan1,SUN Yuan1,LUAN Kuifeng3,LIU Shijie1,2,TONG Xiaohua1,2(1.College of Surveying and Geo-informatics, Tongji University, Shanghai 200092, China;2.Shanghai Key Laboratory for Planetary Mapping and Remote Sensing for Deep Space Exploration,Shanghai 200092, China;3.College of Marine Sciences, Shanghai Ocean University, Shanghai 201306, China)* Corresponding author, E-mail: huanxie@Abstract: ICESat-2 is the world's first laser altimetry satellite to use photon counting technology. It pro⁃vides high-precision,three-dimensional,large-scale ground data that can be obtained rapidly.Altimetry data obtained using photon detection systems generally contain ground signals and background signals,such as atmospheric scattering, which must be filtered to obtain ground information. To analyze the distri⁃文章编号1004-924X(2023)05-0631-13收稿日期:2022-06-15;修订日期:2022-07-05.基金项目:国家自然科学基金项目(No.41822106);上海市教育发展基金会曙光计划项目(No.18SG22)第 31 卷光学精密工程bution characteristics of ICESat-2 background and signal photons and the performance of the point cloud filtering algorithm,we selected data from six types of land cover (city,sea ice,desert,vegetation,ocean and ice sheet (or glacier))in different observation conditions.A statistical analysis of the back⁃ground rate was then performed. The results show that the average background rate of daytime observa⁃tion data is 106 points per second, which is much higher than that of nighttime observation data (104 points per second). The background rate of the weak beam is equivalent to that of the strong beam. Among the six types of land cover, the ice sheet (or glacier) has the highest background rate. Based on the statistical results,21 datasets and seven representative point cloud filters were selected for denoising experiments. After analyzing the accuracy, we can conclude that the improved local density method with recall, accura⁃cy,and F-measure values greater than 0.90 has the best denoising performance and is relatively stable. Finally, the performance, characteristics, and applicability of the seven filtering algorithms were summa⁃rized and analyzed. This can serve as a reference for the subsequent data use and selection of filtering algo⁃rithms.Key words: ICESat-2; laser altimetry; photon counting LiDAR; photon denoising; background rate1 引言激光测高卫星由于速度快、精度高、节省人力物力[1]等优势,成为了当今获取大范围地表三维信息的重要工具之一[2]。
redfit
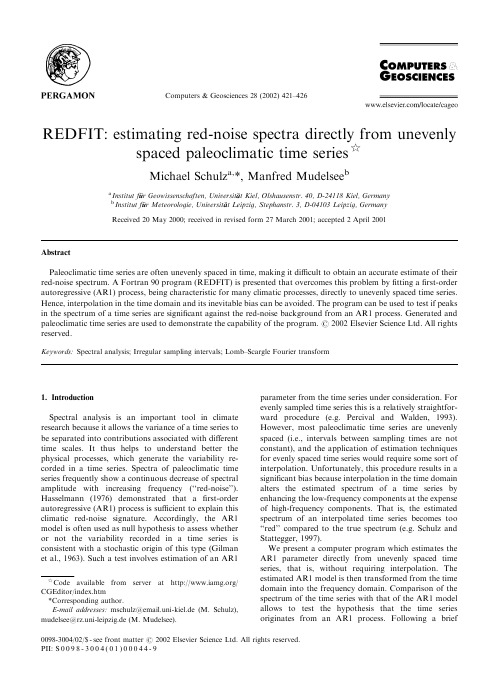
Computers&Geosciences28(2002)421–426REDFIT:estimatingred-noise spectra directly from unevenly spaced paleoclimatic time series$Michael Schulz a,*,Manfred Mudelsee ba Institut f.u r Geowissenschaften,Uni v ersit.a t Kiel,Olshausenstr.40,D-24118Kiel,Germanyb Institut f.u r Meteorolo g ie,Uni v ersit.a t Leipzi g,Stephanstr.3,D-04103Leipzi g,GermanyReceived20May2000;received in revised form27March2001;accepted2April2001AbstractPaleoclimatic time series are often unevenly spaced in time,makingit difficult to obtain an accurate estimate of their red-noise spectrum.A Fortran90program(REDFIT)is presented that overcomes this problem byfitting afirst-order autoregressive(AR1)process,being characteristic for many climatic processes,directly to unevenly spaced time series. Hence,interpolation in the time domain and its inevitable bias can be avoided.The program can be used to test if peaks in the spectrum of a time series are significant against the red-noise background from an AR1process.Generated and paleoclimatic time series are used to demonstrate the capability of the program.r2002Elsevier Science Ltd.All rights reserved.Keywords:Spectral analysis;Irregular sampling intervals;Lomb–Scargle Fourier transform1.IntroductionSpectral analysis is an important tool in climateresearch because it allows the variance of a time series tobe separated into contributions associated with differenttime scales.It thus helps to understand better thephysical processes,which generate the variability re-corded in a time series.Spectra of paleoclimatic timeseries frequently show a continuous decrease of spectralamplitude with increasingfrequency(‘‘red-noise’’).Hasselmann(1976)demonstrated that afirst-orderautoregressive(AR1)process is sufficient to explain thisclimatic red-noise signature.Accordingly,the AR1model is often used as null hypothesis to assess whetheror not the variability recorded in a time series isconsistent with a stochastic origin of this type(Gilmanet al.,1963).Such a test involves estimation of an AR1parameter from the time series under consideration.Forevenly sampled time series this is a relatively straightfor-ward procedure(e.g.Percival and Walden,1993).However,most paleoclimatic time series are unevenlyspaced(i.e.,intervals between samplingtimes are notconstant),and the application of estimation techniquesfor evenly spaced time series would require some sort ofinterpolation.Unfortunately,this procedure results in asignificant bias because interpolation in the time domainalters the estimated spectrum of a time series byenhancingthe low-frequency components at the expenseof high-frequency components.That is,the estimatedspectrum of an interpolated time series becomes too‘‘red’’compared to the true spectrum(e.g.Schulz andStattegger,1997).We present a computer program which estimates theAR1parameter directly from unevenly spaced timeseries,that is,without requiringinterpolation.Theestimated AR1model is then transformed from the timedomain into the frequency parison of thespectrum of the time series with that of the AR1modelallows to test the hypothesis that the time seriesoriginates from an AR1process.Following a brief $Code available from server at /CGEditor/index.htm*Correspondingauthor.E-mail addresses:mschulz@email.uni-kiel.de(M.Schulz),mudelsee@rz.uni-leipzig.de(M.Mudelsee).0098-3004/02/$-see front matter r2002Elsevier Science Ltd.All rights reserved.PII:S0098-3004(01)00044-9description of the numerical procedure and its imple-mentation in a computer program,we apply the program to a synthetic time series and a paleoclimatic record.2.MethodA discrete AR1process r for times t i ði ¼1;2;y ;N Þwith arbitrary spacingis g iven by (Robinson,1977)r ðt i Þ¼r i r ðt i @1Þþe ðt i Þ;ð1Þr i ¼exp @ðt i @t i @1Þ=t ÀÁ:The constant t is the characteristic time scale of the AR1process (a measure of its ‘‘memory’’)and e indicates ‘‘white’’Gaussian noise with zero mean and variance s 2e 1@exp @2ðt i @t i @1Þ=t ÀÁ.This value of s 2e en-sures that the AR1process is stationary and has unit variance.The spectrum G rr ðf j Þcorrespondingto the time-domain process of Eq.(1)is (e.g.Percival and Walden,1993)G rr ðf j Þ¼G 01@r 21@2r cos ðp f j =f Nyq Þþr 2ð2Þwhere f j denotes discrete frequency up to the Nyquist frequency f Nyq (cf.Schulz and Stattegger,1997)and G 0is the average spectral amplitude.The ‘‘average autocor-relation coefficient’’r is calculated from the arithmetic mean of the samplingintervals D t ¼ðt N @t 1Þ=ðN @1Þas r exp @D t =t ÀÁ.The unknown value of t is estimated from an unevenly spaced time series usingthe least-squares algorithm devised by Mudelsee (2002).The spectrum of an irregularly spaced time series is determined without the need for interpolation by means of the Lomb–Scargle Fourier transform (Lomb,1976;Scargle,1982,1989).Schulz and Stattegger (1997)presented a computer program for this purpose which makes additional use of the so-called Welch-overlapped-seg-ment-averaging (WOSA)procedure (Welch,1967).This algorithms splits a time series into n 50segments which overlap by 50%,the final spectral estimate is derived from averaging the n 50periodograms.With an estimate for t as well as an appropriate value for G 0it should then be possible to overlay the red-noise spectrum after Eq.(2)and the spectrum estimated from the data.Provided that the probability distribution of G rr at each frequency follows a w 2distribution (e.g.Percival and Walden,1993),it is finally possible to test if the data spectrum is consistent with a red-noise model.Unfortunately,this approach is hampered by an inherent aspect of the Lomb–Scargle Fourier transform:in contrast to the classical Fourier transform,the individual Lomb–Scargle Fourier components are not necessarily independent of each other and,as aconsequence,an estimated spectrum based on the Lomb–Scargle transform may be biased (Lomb,1976;Scargle,1982).In particular,spectral amplitudes at the high-frequency end of a spectrum are often over-estimated.Therefore,a red-noise spectrum Eq.(2)which is based on an unbiased estimate of t for a given time series will not necessarily coincide with the ‘‘Lomb–Scargle spectrum’’of the same time series.We therefore seek for a bias correction for the Lomb–Scargle Fourier transform.3.Numerical procedureThe systematic deviation between a theoretical red-noise spectrum Eq.(2)and one estimated from an unevenly spaced time series by means of the Lomb–Scargle Fourier transform depends on the distribution of the samplingtimes in the interval [t 1,t N ](Lomb,1976;Scargle,1982).For some arbitrary distribution of samplingtimes the lack of an analytical solution for the deviation prevents a direct bias correction of a Lomb–Scargle spectrum.To circumvent this problem,we turn to a Monte–Carlo technique.Based on the actual samplingtimes,an ensemble of N sim AR1time series is generated after Eq.(1)with fixed t .The deviation of the average spectrum of the ensemble from the known theoretical spectrum is then employed for the required bias correction.The computational steps to obtain a red-noise spectrum of an unevenly spaced time series x ðt i Þwhich is consistent with the estimated value of t are as follows:1.Estimate t from x ðt i Þusingthe time-domain algorithm of Mudelsee (2002).If more than one WOSA segment is used for spectral analysis (n 50>1),an average value for t is calculated from t estimates for each individual segment.The individual t estimates (Mudel-see,in press)are bias corrected,based on the number of data points in each WOSA segment.2.Estimate spectrum #Gxx ðf j Þof x ðt i Þin the interval [0,f Nyq ]usingthe Lomb–Scarg le Fourier transform as described in Schulz and Stattegger (1997).Determine thearea under #Gxx ðf j Þwhich is an estimate for the variance of x ðt i Þ.3.Monte Carlo simulation loop.Repeat N sim times*create AR1time series accordingto Eq.(1),using the samplingtimes of the input data ðt i Þ,the estimated t ,and an independent set of e ðt i Þfor each simulation*estimate spectrum of the generated AR1time series,#Grr ðf j ÞM.Schulz,M.Mudelsee /Computers &Geosciences 28(2002)421–426422*scale #Grr ðf j Þsuch that the area under the spectrum is identical to the area under #Gxx ðf j ÞDetermine arithmetic mean of the N sim independent red-noise spectral estimates #Grr ðf j Þ .4.Calculate theoretical AR1spectrum G rr ðf j Þfor theestimated value of t (Eq.(2)).(Note that G rr ðf j Þis not affected by the bias of the Lomb–Scargle Fourier transform,because the critical parameter t is estimated in the time domain.)5.Select G 0(see Eq.(2))such that the area underG rr ðf j Þis identical to the area under #Gxx ðf j Þ.(This step is required since the true noise variance of the process under consideration is unknown.)6.Calculate a correction factor c ðf j Þfor the bias adjustment of the Lomb–Scargle spectrum as c ðf j Þ¼#Grr ðf j Þ=G rr ðf j Þ:ing c ðf j Þ,determine a bias-corrected version ofthe spectrum of the data as #G 0xx ðf j Þ¼#G xx ðf j Þ=c ðf j Þ:8.For assessingthe statistical sig nificance of a spectralpeak,the upper confidence interval of the AR1noise is calculated for various significance levels (based on w 2distribution;degrees of freedom depend on the actual spectral analysis setting;cf.Schulz and Stattegger,1997).In addition,significance levels are calculated from percentiles of the Monte Carlo ensemble.9.Check appropriateness of the AR1model to describe x ðt i Þby testingthe equality of G rr ðf j Þand #G 0xxðf j Þusinga non-parametric runs test (Bendat and Piersol,1986).The assumptions underlyingthis procedure are:(i)The noise background recorded in a time series can indeed be approximated by an AR1process (tested in step 9),that is,the potential effect of non-AR1signal components (e.g.harmonic signals)can be neglected.Although it would be possible to identify and subtract harmonic signal components prior to estimating t (see Mann and Lees,1996for evenly spaced time series),this approach may fail if there are quasi-periodic signals (e.g.narrow-band noise),which often occur in climatic time series.For most practical problems such refinement is unwarranted because such signals cover only a small portion of the entire frequency range and have only a marginal effect on the estimated value of t (Gilman et al.,1963).Situations in which non-AR1features doaffectthe estimation of t can be identified by visual inspection of the resultingred-noise spectrum and the runs test of step9.(ii)The distribution of data points alongthe time axis is not too clustered(Horne and Baliunas,1986).A computer program(REDFIT)that performs the above steps is freely available via anonymous ftp from ftp.rz.uni-kiel.de(file:/pub/sfb313/mschulz/redfit35.zip) or from the IAMG server.The zip-archive includes Fortran90source code,binaries for Windows95(or above),program documentation and examplefiles. The program offers the same functionality for uni-variate spectral analysis as the SPECTRUM program (Schulz and Stattegger,1997)and uses the same format for inputfiles.To cope better with the computational demand of the Monte–Carlo simulation,the program is command-line driven and can therefore be run in batch mode.4.Example computationsThefirst test signal is a pure AR1process after Eq.(1) with t¼15yr and N¼324data points(Fig.1A).The uneven time axis is generated by treating the time interval between subsequent samplingtimes as a random variable following a gamma distribution with3degreesof freedom(which is a geologically realistic model;Schulz and Stattegger,1997).The estimated value for tis15yr(90%confidence interval:10o t o20yr).Theuncorrected Lomb–Scargle spectrum of the AR1timeseries,#G xxðf jÞ,does not show the characteristic red-noise shape,instead spectral amplitudes increase slightly forf>0:09(1/yr)(Fig.1B).As expected,the same holdstrue for the mean,#G rrðf jÞ,of the N sim¼1000simu-lated red-noise spectra(Fig.1B).Compared to thetheoretical spectrum of the generated AR1process,G rrðf jÞ,(based on estimated value of t)the Lomb–Scargle Fourier transform clearly overestimates thespectral amplitudes for a large part of the spectrum(Fig.1B).Applying the bias correction(steps6and7)results in a spectral estimate#G0xxðf jÞwhich is,of course, consistent with G rrðf jÞ(Fig.1B).At the low-frequency end of the spectrum we observe that#G0xxðf jÞ>#G xxðf jÞ. This effect is caused by thefinite length of the time series,which leads to an underestimation of the spectral amplitudes for periods exceedingthe leng th of the time series(independently of the spectral-analysis technique beingused and the spacingof the time axis).Thus,as a side effect,the bias correction accounts also for this problem inherent in all spectral analysistechniques.In the second example,we investigate the glacial part of the oxygen-isotope record from the GISP2ice core from Greenland(Grootes and Stuiver,1997;Fig.2A), which reflects,to a large extent,air temperature above Greenland.In the initial step of the analysis we determine whether or not the spectrum of this time series is consistent with a red-noise model.Based on the periodogram of the time series(n50¼1;rectangular window;cf.Schulz and Stattegger,1997)and N sim¼1000Monte–Carlo simulations,the runs test indicates that the AR1model is indeed appropriate to character-ize this record(5%significance level).The estimated mean value of t is310yr with90%confidence interval 240o t o380yr.Next we test if any non-AR1compo-nents can be identified in the time series.For this purpose we repeat the analysis,but increase the number of WOSA segments in the spectral analysis in order to obtain a consistent spectral estimate(we refer the reader to Schulz and Stattegger,1997for details of the spectral-analysis technique).Setting n50¼4and selectinga Welch spectral window to reduce spectral leakage results in the spectrum depicted in Fig.2B.We scale the theoretical red-noise spectrum by an appropriate per-centile of the w2-probability distribution to obtain a false-alarm level,which marks the maximum spectral amplitude expected if the time series would have been generated by an AR1process.Accordingly,spectral peaks exceedingthe false-alarm level indicate non-AR1 components in a time series,and should be considered significant.We follow Thomson(1990)and select a false-alarm level ofð121=nÞÂ100%,where n is the number of data points in each WOSA segment.For the example at hand,a false-alarm level of99.6%results.At this level the spectrum indicates the presence of a single peak at f¼1=ð1470yrÞwhich is not consistent with the red-noise model.This spectral peak is associated with the so-called Dansgaard–Oeschger oscillations,the dominant mode of millennial-scale climatefluctuations during the last glacial period(e.g.Grootes and Stuiver, 1997).However,care should be taken when interpreting these results because the assumption of weak stationar-ity of the time series may be violated.5.ConclusionsWe present a computer program(REDFIT)for testingwhether or not the red-noise shape,often observed in paleoclimatic time series,is consistent with the generation by afirst-order autoregressive(AR1) process.In contrast to existingapproaches,REDFIT allows direct processingof unevenly spaced time series and,hence,the usual prerequisite of data interpolation is not required.Since interpolation of an unevenly spaced time series is equivalent to low-passfiltering,reddening of an estimated spectrum will result and consequently a biased test result may be the outcome.As an aside,by correctingfor the effect of correlation between Lomb–Scargle Fourier components,the program removes the bias of this Fourier transform for unevenly spaced data.A real-world example demonstrates the capability of REDFIT to detect spectral feature not consistent with an AR1origin.Although REDFIT indicates whether or not the main assumption(i.e.,adequacy of the AR1 model)is violated,the program should not be used as black-box tool without checkingthe structure of a time series prior to its analysis.AcknowledgementsThis work received support from the BMBF ‘Klimaforschungsprogramm’(MS)and DFG Research Grant MU1595/1-1(MM).ReferencesBendat,J.S.,Piersol, A.G.,1986.Random Data,2nd edn.Wiley,New York,566pp.Gilman,D.L.,Fuglister,F.J.,Mitchel Jr.,J.M.,1963.On the power spectrum of red noise.Journal of the Atmospheric Sciences20(2),182–184.Grootes,P.M.,Stuiver,M.,1997.Oxygen18/16variability in Greenland snow and ice with10@3–105-year time resolution.Journal of Geophysical Research102(C12), 26455–26470.Hasselmann,K.,1976.Stochastic climate models:Part I.Theory.Tellus28(6),473–485.Horne,J.H.,Baliunas,S.L.,1986.A prescription for period analysis of unevenly sampled time series.The Astrophysical Journal302(2),757–763.Lomb,N.R.,1976.Least-squares frequency analysis of unequally spaced data.Astrophysics and Space Science39, 447–462.Mann,M.E.,Lees,J.M.,1996.Robust estimation of back-ground noise and signal detection in climatic time series.Climatic Change33(3),409–445.Mudelsee,M.,2002.TAUEST:a computer program for estimatingpersistence in unevenly spaced weather/climate time puters a Geosciences,28(1)69–72. Percival, D.B.,Walden, A.T.,1993.Spectral Analysis for Physical Applications.Cambridge University Press,Cam-bridge,583pp.Robinson,P.M.,1977.Estimation of a time series model from unequally spaced data.Stochastic Processes and their Applications6,9–24.Scargle,J.D.,1982.Studies in astronomical time series analysis.II.Statistical aspects of spectral analysis of unevenly spaced data.The Astrophysical Journal263(2), 835–853.Scargle,J.D.,1989.Studies in astronomical time series analysis.III.Fourier transforms,autocorrelation functions,and cross-correlation functions of unevenly spaced data.The Astrophysical Journal343(2),874–887.M.Schulz,M.Mudelsee/Computers&Geosciences28(2002)421–426425Schulz,M.,Stattegger,K.,1997.SPECTRUM:Spectral analysis of unevenly spaced paleoclimatic time series.Computers a Geosciences23(9),929–945.Thomson,D.J.,1990.Time series analysis of Holocene climate data.Philosophical Transactions of the Royal Society of London.Series A330,601–616.Welch,P.D.,1967.The use of fast Fourier transform for the estimation of power spectra:a method based on time averaging over short,modified periodograms.IEEE Transactions on Audio and Electroacoustics 15(2),70–73.M.Schulz,M.Mudelsee/Computers&Geosciences28(2002)421–426 426。
“蒸发悖论”在秦岭南北地区的探讨

“蒸发悖论”在秦岭南北地区的探讨蒋冲;王飞;刘思洁;穆兴民;李锐;刘焱序【摘要】潜在蒸散量(ET0)是大气蒸发的估计值,已经广泛应用于灌溉管理和无实测蒸发资料地区的估算.分析ET0的时空变化是研究水资源对气候变化响应的基础工作,同时对于农业水资源的优化利用也具有重要意义.根据秦岭南北47个气象站1960-2011年逐日数据,利用FAO Penman-Monteith公式计算出各站的潜在蒸散量(ET0),研究了气温、降水与ET0之间的长期变化趋势关系,对导致ET0下降的主要原因进行了讨论,着重对秦岭南北地区是否存在“蒸发悖论”进行验证.结果表明:(1)秦岭南北整体气温经历了先降后升的变化过程,1993年为突变年份,1960-1993年的降温速率和1994-2011年的升温速率均表现出由南向北递减的规律,1960-2011年整体升温速率由北向南递减.(2)1979年和1993年是ET0变化的转折点,以1979和1993为界ET0经历了“升—降—降”的变化阶段.1960-1979年仅汉水流域和巴巫谷地存在“蒸发悖论”现象,1980-1993、1994-2011和1960-2011年3个时段区域整体和各子区均发现了“蒸发悖论”现象.秋季后18a 和52a整体以及冬季前34a和52a整体均存在“蒸发悖论”现象,冬季最为明显.(3)近52年整体降水表现出不显著的下降趋势,相较于年尺度,夏季降水与ET0逆向变化趋势更为明显.(4)年尺度上,太阳辐射(日照时数)下降引起的潜热通量减少是造成ET0下降即“蒸发悖论”现象的主要原因.季节尺度,春季ET0下降的主导因素为风速,其它季节均为太阳辐射(日照时数).【期刊名称】《生态学报》【年(卷),期】2013(033)003【总页数】12页(P844-855)【关键词】秦岭南北;潜在蒸散量;蒸发悖论;气温;降水【作者】蒋冲;王飞;刘思洁;穆兴民;李锐;刘焱序【作者单位】西北农林科技大学资源环境学院,杨凌712100;西北农林科技大学资源环境学院,杨凌712100;中国科学院水利部水土保持研究所,杨凌712100;北京大学遥感与地理信息系统研究所,北京100871;西北农林科技大学资源环境学院,杨凌712100;中国科学院水利部水土保持研究所,杨凌712100;西北农林科技大学资源环境学院,杨凌712100;中国科学院水利部水土保持研究所,杨凌712100;陕西师范大学旅游与环境学院,西安710062【正文语种】中文潜在蒸散量(ET0)是指在一定气象条件下水分供应不受限制时,某一固定下垫面土壤蒸发量和植物蒸腾量的总和,它是实际蒸散量的理论上限,也是计算实际蒸散量的基础,又被称之为参考作物蒸散量[1-4]。
ICESat-2 ATL03数据预处理及校正方法

〇 引言
近些年来,星 载 激 光 雷 达 凭 借 其 全 天 时 、高空间 分 辨 率 以 及 覆 盖 范 围 广 的 特 点 ,成 为 多 个 领 域 研 究 的 有效工具。2003年 1 月,美 国 N A S A 发射激光测高仪 系统 Geoscience Laser Altimeter System(GLAS),搭 载 于 以 冰 、云 和 陆 地 高 程 卫 星 (Ice, Cloud and land Elevation Satellite, 丨CESat) 平 台 。该 系 统 被 用 于 测 量 冰被地形,同 时 也 监 测 云 层 和 大 气 的 特 性 ,提供云层 高度和厚度,有 助 于 提 高 短 期 天 气 及 气 候 预 报 能 力 。 2003年 到 2009年 在 轨 期 间 ,收 集 的 测 高 数 据 促 使 科学家对格陵兰岛和南极冰盖的总体质量变化有了 更 为 精 确 的 估 计 [1]。 为 了 延 续 ICESat的后续观测任 务 ,N A S A 于 2018年 9 月 1 5 日发射了地形激光测高 仪 系 统 ( Advanced Topographic Laser Altimeter System, ATLAS),搭 载 于 以 冰 、云 和 陆 地 高 程 卫 星 2 号 (The Ice, Cloud, and Land Elevation Satellite-2, ICESat-2)平 台 。ATLAS/ICESat-2将帮助科学家研究地球冰冻圈 发 生 变 化 的 原 因 和 幅 度 ;测 量 地 球 温 带 和 热 带 区 域 的 高程信息,并 对 全 球 森 林 植 被 信 息 进 行 评 估 [2]。不同 于 GLAS/ICESat 全 波 形 记 录 ,ATLAS/ICESat-2 采用 更 为 灵 敏 的 单 光 子 探 测 器 ,以 10 kH z的重复频率发 射 532 nm激 光 脉 冲 ,通 过 获 取 高 密 度 光 子 计 数 回 波 数 据 ,实 现 高 精 度 全 球 观 测 [\
城市热岛气候
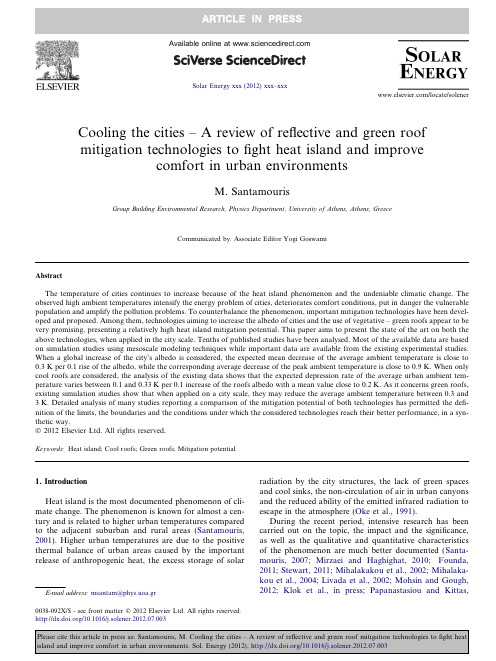
Cooling the cities –A review of reflective and green roof mitigation technologies to fight heat island and improvecomfort in urban environmentsM.SantamourisGroup Building Environmental Research,Physics Department,University of Athens,Athens,GreeceCommunicated by:Associate Editor Yogi GoswamiAbstractThe temperature of cities continues to increase because of the heat island phenomenon and the undeniable climatic change.The observed high ambient temperatures intensify the energy problem of cities,deteriorates comfort conditions,put in danger the vulnerable population and amplify the pollution problems.To counterbalance the phenomenon,important mitigation technologies have been devel-oped and proposed.Among them,technologies aiming to increase the albedo of cities and the use of vegetative –green roofs appear to be very promising,presenting a relatively high heat island mitigation potential.This paper aims to present the state of the art on both the above technologies,when applied in the city scale.Tenths of published studies have been analysed.Most of the available data are based on simulation studies using mesoscale modeling techniques while important data are available from the existing experimental studies.When a global increase of the city’s albedo is considered,the expected mean decrease of the average ambient temperature is close to 0.3K per 0.1rise of the albedo,while the corresponding average decrease of the peak ambient temperature is close to 0.9K.When only cool roofs are considered,the analysis of the existing data shows that the expected depression rate of the average urban ambient tem-perature varies between 0.1and 0.33K per 0.1increase of the roofs albedo with a mean value close to 0.2K.As it concerns green roofs,existing simulation studies show that when applied on a city scale,they may reduce the average ambient temperature between 0.3and 3K.Detailed analysis of many studies reporting a comparison of the mitigation potential of both technologies has permitted the defi-nition of the limits,the boundaries and the conditions under which the considered technologies reach their better performance,in a syn-thetic way.Ó2012Elsevier Ltd.All rights reserved.Keywords:Heat island;Cool roofs;Green roofs;Mitigation potential1.IntroductionHeat island is the most documented phenomenon of cli-mate change.The phenomenon is known for almost a cen-tury and is related to higher urban temperatures compared to the adjacent suburban and rural areas (Santamouris,2001).Higher urban temperatures are due to the positive thermal balance of urban areas caused by the important release of anthropogenic heat,the excess storage of solarradiation by the city structures,the lack of green spaces and cool sinks,the non-circulation of air in urban canyons and the reduced ability of the emitted infrared radiation to escape in the atmosphere (Oke et al.,1991).During the recent period,intensive research has been carried out on the topic,the impact and the significance,as well as the qualitative and quantitative characteristics of the phenomenon are much better documented (Santa-mouris,2007;Mirzaei and Haghighat,2010;Founda,2011;Stewart,2011;Mihalakakou et al.,2002;Mihalaka-kou et al.,2004;Livada et al.,2002;Mohsin and Gough,2012;Klok et al.,in press;Papanastasiou and Kittas,0038-092X/$-see front matter Ó2012Elsevier Ltd.All rights reserved./10.1016/j.solener.2012.07.003E-mail address:msantam@phys.uoa.gr/locate/solenerSolar Energy xxx (2012)xxx–xxx2012).Higher urban temperatures increase the energy con-sumption for cooling and raise the peak electricity demand (Hassid et al.,2000;Cartalis et al.,2001;Santamouris et al.,2001;Kolokotroni et al.,2012;Hirano and Fujita,in press;Akbari and Konopacki,2004;Akbari et al.,1992).As men-tioned by Santamouris et al.(2001),heat island in the city of Athens,Greece,doubles the cooling load of buildings and almost triples their peak electricity demand,while decreasing the Coefficient of Performance (COP),of mechanical cooling systems up to 25%.According to Akbari et al.(1992),for US cities with population larger than 100,000the peak electricity load will increase 1.5–2%for every 1°F increase in temperature.The cooling energy increase is accompanied by intensification of pollu-tion patterns in cities and increase of ozone concentrations (Stathopoulou et al.,2008;Sarrat et al.,2006;Taha,2008a ),while the ecological footprint of the cities is increased (Santamouris et al.,2007a ),the outdoor thermal comfort conditions deteriorate (Pantavou et al.,2011),the thermal stress in low income dwellings is increased,the indoor thermal comfort levels are seriously decreased and health problems are intensified (Sakka et al.,2012;Luber and McGeehin,2008).Research carried out recently has permitted the develop-ment of technological measures to counterbalance the impact of heat island (Rosenfeld et al.,1995;Akbari et al.,2001;Adnot et al.,2007;Kuttler,2011;Gaitani et al.,2007).Mitigation techniques aim to balance the ther-mal budget of cities by increasing thermal losses and decreasing the corresponding gains.Among the more important of the proposed techniques are those targeting to increase the albedo of the urban environment,to expand the green spaces in cities and to use the natural heat sinks in order to dissipate the excess heat (Akbari et al.,2005a;Julia et al.,2009;Mihalakakou et al.,1994).Recent real scale applications involving the use of the above mitigation techniques have resulted into very important climatic ben-efits and a serious reduction of the heat island strength (Gaitani et al.,2011;Fintikakis et al.,2011;Santamouris et al.,in press,2012).Roofs present a very high fraction of the exposed urban area.Estimations given in Akbari and Rose (2008),for four American cities,show that the roof area fraction varies from 20%to 25%for less or more dense cities.Based on these findings and considering that urban areas occupy almost 1%of all land,it is estimated that the total roof area of the urban world is close to 3.8Â1011m 2(Akbari et al.,2009a ).However,according to Jacobson et al.(2007),the above assumption regarding the size of urban areas is about 2.26times higher than it is estimated using an anal-ysis of satellite data.Given that the available free ground area in the urban environment is quite limited and of very high economic value,it is relatively difficult to implement large scale mit-igation technologies on the ground surface of cities.At the same time,urbanization decreases the proportion of spaces dedicated to plants and trees or other mitigation infrastruc-tures because of new building developments (Mathieu et al.,2007;Smith,2010).On the contrary,roofs provide an excellent space to apply mitigation techniques,given that the relevant cost is limited,while the corresponding techniques are associated to important energy savings for the buildings.Two are the more important mitigation technologies associated to roofs:(a)Those aiming to increase the albedo of the roofs,known as cool or reflective roofs (Zinzi,2010;Akbari and Levinson,2008;Synnefa and Santamouris,in press )those that propose roofs partially or completed cov-ered with vegetation,known as green roofs or living roofs (Theodosiou,2009;Santamouris et al.,2007b;Sfakianaki et al.,2009).Both technologies can lower the surface tem-peratures of roofs and thus to decrease the corresponding sensible heat flux to the atmosphere.Cool or reflective roofs are typically white and present a high albedo.Products used in cool roofs are single ply or liquid applied (Mac Cracken,2009).Typical liquid applied products involve white paints,elastomeric,polyurethane or acrylic coatings.Examples of white single ply products involve EPDM (Ethylene-Propylenediene-Tetrolymer Membrane)PVC (Polyvinyl Chloride),CPE (Chlorinated Polyethylene),CPSE (Chlorosulfonated Polyethylene),and TPO (Thermoplastic Polyolefin)materials (Mac Crac-ken,2009).A review of the recent developments on the field of liquid applied materials used in reflective roofs is given in Santamouris et al.(2011).The first generation of materials used in cool roofs consisted of natural materials quite eas-ily found in the nature characterized by a relatively high albedo,rarely higher than 0.75(Doulos et al.,2004;Bretz et al.,1992;Reagan and Acklam,1979),while the second generation was based on the development of artificial white materials designed to present very high albedo values close or higher than 0.85(Synnefa et al.,2006;Kolokotsa et al.,2012;Santamouris et al.,2008).In a later,third phase of development,colored high reflective materials have been developed.The overall idea was to develop colored materi-als presenting a high reflectivity value in the infrared spec-trum (Levinson et al.,2005a,2005b;Synnefa et al.,2007a ).The specific materials were characterized by a much higher global reflectivity than the conventional ones of the same color and were associated to important energy savings when used in building roofs or urban infrastructures (Synnefa et al.,2007b,2011;Santamouris et al.,2007c ).Quite recently,fourth generation reflective materials based on nanotechnological additives like thermochromic paints and tiles (Ma et al.,2001,2002;Karlessi et al.,2009),or PCM doped cool materials (Karlessi et al.,2011;Zhang et al.,2007;Pasupathy et al.,2008;Cabeza et al.,2007)have been developed and likely to be used for future cool roof applications.Many studies have been performed in order to identify the cooling potential and the possible improvements of indoor thermal comfort caused by cool roofs (Synnefa et al.,in press;Akbari et al.,2005a,2005b;Levinson2M.Santamouris /Solar Energy xxx (2012)xxx–xxxet al.,2005c;Tengfang et al.,2012;Kolokotsa et al.,in press;Romeo and Zinzi,in press;Kolokotroni et al.,in press;Bozonnet et al.,2011;Boixo et al.,2012;Takebay-ashi et al.,in press ).Energy benefits vary mainly as a func-tion of the climatic conditions and the characteristics of the building.Typically,peak summer indoor temperatures may decrease up to 2°C in moderately insulated buildings while cooling loads reductions may range between 10%and 40%.At the same time,the heating penalty may range between 5%and 10%as a function of the local climate and building characteristics.In parallel,important simulation studies have been car-ried out to identify the heat island mitigation potential of cool roofs (Savio et al.,2006;Synnefa et al.,2008;Menon et al.,2010;Jacobson and Ten Hoeve,2012).Low temper-atures at the roof level,decrease the sensible heat flux to the atmosphere and add to the mitigation of the urban heat island.Most of the studies have been carried out in USA,using mesoscale simulation models.The specific results of the above studies are discussed in the following chapters.Increase of the green spaces in cities,contribute to decrease the urban surface and ambient temperatures and mitigate heat island effect.Studies reported by Gill et al.(2007),show that an increase by 10%of the urban green in Manchester,UK,could amortize the predicted increase by 4K,of the ambient temperature over the next 80years.Green or living roofs are partially or fully covered by veg-etation and a growing medium over a waterproofing mem-brane.There are two main types of green roofs:Extensive roofs which are light and are covered by a thin layer of veg-etation and intensive roofs which are heavier and can sup-port small trees and shrubs.Green roofs present a variety of advantages like storm water runoffmanagement,increased roof materials durability,decreased energy con-sumption,possible better air quality and noise reduction,offer space for urban wildlife and increased mitigation of urban heat island (Parizotto and Lamberts,2011;Mentens et al.,2006;Teemusk and Mander,2009;Rowe,2010;Ren-terghem and Botteldooren,2011;Brenneisen,2006;Pataki et al.,2011).Several experimental and theoretical studies have been performed to identify the energy conservation potential of green roofs (Kumar and Kaushik,2005;Alexandri and Jones,2007;Wong et al.,2003a;Theodosiou,2003;Eumorfopoulou and Aravantinos,1998;Jaffal et al.,2012;Spala et al.,2008;Takakura et al.,2000;Castleton et al.,2010).The specific energy benefits depend on the local climate,the green roof design and more importantly on the specific building characteristics.Given that in green roofs heat transfer benefits are mainly provided through latent heat processes,the performance of the system is higher in dry climates.In parallel,the thickness and the thermal characteristics of the vegetative roof largely define its U value and the corresponding transfer of heat to the building,while the type and characteristics of the plants (LAI),define the shading levels and the transfer of radia-tion through the layers.Finally,watering is important asit determines the latent heat release and regulates the ther-mal balance of the roof.However,the building characteris-tics also define the possible contribution of green roofs.In non-insulated buildings the impact of green roofs is much higher than in insulated ones.It is evident that the better the insulation of the roof,the lower the contribution of the green roof.In parallel,the characteristics of the energy load of the building define the specific contribution of the roof system.In buildings presenting a high part of their energy load because of the ventilation gains or losses,inter-nal or solar gains,green roofs have a limited contribution.On the contrary,in buildings where the energy load is due to the heat transfer though the opaque parts of the enve-lope,vegetative roofs may contribute significantly to reduce heating and cooling loads.Existing studies per-formed for various types of buildings,green roof character-istics and climatic zones,show that expected reduction of the annual energy load may vary between 1%and 40%in extreme cases.In reality,in well insulated modern buildings the energy contribution of green roofs is quite modest.Although the possible energy contribution of green and reflective roofs is a quite well investigated area,the avail-able information on the possible mitigation potential of both technologies is relatively limited (Cameron et al.,2012).Most of the existing studies are based on mesoscale simulation modeling and the given results depend com-pletely on the specific regional characteristics and the assumptions made,while very few experimental studies are available.Some of the reported studies attempt to com-pare the climatic potential of the two roof technologies either using simulation or experimental techniques.How-ever,most of the studies are building and climatic specific and it is quite difficult to extract general conclusions,although the provided results are very useful.The objective of the present paper is to review,in a crit-ical way,the available scientific information on the mitiga-tion potential of reflective and green roofs.Also,to combine and analyze the existing theoretical and experi-mental data,compare and homogenize the results and if possible,to provide general conclusions and suggestions.2.Increasing the albedo in the city:the role of cool roofs It is well known and documented that large scale change of albedo has a serious impact on the local peak ambient temperature.Multiyear observations reported in Campra et al.(2008),show an important temperature reduction (À0.3K/decade),because of the massive construction of high albedo greenhouses through the Almeria area in Spain.Several simulation studies have been carried out to investigate the impact of various albedo related mitigation techniques on the possible reduction of ambient tempera-ture.Most of the works evaluate the impact of a general increase of the local albedo considering in a combined way cool roofs,cool pavements roadways and parking lots,while few studies consider and evaluate just the mitigation impact of reflective roofs.In parallel,many of the mitiga-M.Santamouris /Solar Energy xxx (2012)xxx–xxx3tion studies investigate the combined impact of several and different mitigation technologies,i.e.increase of the local albedo and vegetative cover without splitting the results about the specific contribution of each technique (Taha et al.,1999).The present paper considers only studies aim-ing either to calculate the influence of reflective roofs or to evaluate the combined impact of a general albedo change.2.1.Mitigation potential of cool roofsEvaluation of the heat island mitigation potential of cool roofs in a city is a quite new scientific subject.Four specific studies are currently available focusing on the potential decrease of the ambient temperature because of the increase of roof albedo (Savio et al.,2006;Synnefa et al.,2008;Menon et al.,2010;Jacobson and Ten Hoeve,2012).Two of the studies (Savio et al.,2006;Synnefa et al.,2008),examine the local impact of cool roofs in New York,US and Athens Greece,while the studies reported by Menon et al.(2010)and Jacobson and Ten Hoeve (2012),investigate the climatic impact of reflective roof on a plan-etary scale.In Savio et al.(2006),the impact of cool roofs on the potential ambient temperature decrease at 2m height above ground,has been evaluated for New York city,US,using simulations performed with the Penn State/NCAR MM5regional climate model (Grell et al.,1994).Runs were performed for the period of three heat waves during the summer of 2002.An average solar reflectivity equal to 0.5was used.It was calculated that the daily aver-age temperature decrease in the various parts of the city ranges between 0.18K and 0.36K.In parallel,the average 3PM reduction of peak ambient temperature ranged between 0.31K and 0.62K as a function of the character-istics of the considered areas.The climatic impact of cool roofs has been simulated for the city of Athens,Greece (Synnefa et al.,2008).The study has been performed using the MM5climate model (Grell et al.,1994),for the 15th of August 2005.Two modified albedo scenarios were considered:a moderate one,where the albedo of the roofs was increased from 0.18to 0.63,and an extreme one where the final albedo of the building rooftops was considered 0.85.It was calculated that for the moderate increase of the albedo,the ambient temperature depression at 2m height at 12:00LST varied between 0.5and 1.5K.For the extreme case of albedo increase,the ambient temperature reduction varied between 1K and 2.2K.The temperature depression found to start at 9:00LST and stopped at around 20:00LST.Simulations were carried out using the CSCRC model (Yoshida et al.,2000;Hong et al.,2004),to calculate the impact of cool roofs in medium and high rise neighbor-hoods with medium and high rise buildings (average height 28.6and 67.4m respectively).The albedo of the roofs was changed from 0.2to 0.5.It was calculated that the decrease of the ambient temperature at street level was 0.1K and 0.12K for the areas of high and medium height rise build-ings.It is evident that the impact of reflective roofs on the ambient temperature at street level is seriously reduced when the height of buildings where cool roofs are applied is great.The characteristics of the NY and Athens studies are summarized in Table 1.The expected rate of temperature depression per 0.1increase of the albedo of roofs ranges between 0.1and 0.19K for New York and 0.11–0.33K for Athens.The average temperature depression for both studies is close to 0.2K per 0.1increase of roof albedo,while the corresponding minimum and maximum tempera-ture depression are 0.02K and 0.41K,respectively.Simulations have been carried out using the GATOR–GCMOM model (Jacobson et al.,2007),to calculate the climatic impact of cool roofs on a planetary scale.The solar albedo of all roofs was increased from 0.12to 0.65.This corresponds to an overall urban albedo increase by 0.147.It is reported that a worldwide conversion to cool roofs will contribute to decrease populated weighted tem-peratures by 0.02K but to increase the overall earth tem-perature by 0.07K.The results of this study suggesting an overall global warming because of the possible conver-sion of roofs to white have been discussed in Oleson et al.(2008a,b),raising several concerns about the assump-tions and the results of this publication.Another similar simulation study aiming to evaluate the worldwide heat island mitigation potential of reflective roofs is reported in Menon et al.(2010).The study was car-ried out using the urban canyon model CLMU coupled with other models (Oleson et al.,2008a,b ).It was consid-ered that the albedo of roofs increases up to 0.9.It is calcu-lated that the daily maximum urban temperatures decrease by 0.6K while the daily minimum ambient temperature decreased by 0.3K.This corresponds to a decrease in the urban diurnal temperature range of 0.3K.2.2.Increasing the albedo of cities –mitigation potential One of the first evaluations of the climatic impact of reflective surfaces was published in Sailor (1995).The authors simulated the impact of albedo modification in Los Angeles,USA.They have used the Colorado State University Mesoscale Model (Mahrer and Pielke,1977).It was assumed that the albedo of downtown surfaces was increased by 0.14while the corresponding increase for the entire basin was close to 0.08.It was calculated that the considered albedo change reduced ambient tempera-tures by at least 0.5K over the majority of the area,while the peak urban temperatures reduction was 1.4K near downtown LA.A second study carried out for the same city,Los Ange-les,USA,is described in Rosenfeld et al.(1995).The Col-orado State University Mesoscale Model (Mahrer and Pielke,1977),was also used.The authors assumed an increase of the average albedo by 0.13,and in particular from 0.13to 0.26for an area of 100,000km 2,in which over 20%of the land was covered by artificial surfaces.Flat and4M.Santamouris /Solar Energy xxx (2012)xxx–xxxsloped roofs accounted for about 30%of the area.For the flat roofs it was considered that the albedo changes from 0.25to 0.75while for the sloped roofs from 0.25to 0.6.It was calculated that the peak impact of the albedo change occurs in the early afternoon and the potential cooling exceeds 3K at 3pm.Simulations carried out under differ-ent boundary conditions indicate that the expected peak summertime temperature reductions were between 2and 4K.In another study of the same group (Rosenfeld et al.,1998),the cooling potential of a possible albedo change in the city of Los Angeles was evaluated.It was considered that in almost 1250Km 2of roof surface the albedo was increased by 0.35and in particular from 0.15to 0.5.It was also considered that in 1250km 2of pavements,the albedo was increased by 0.25,from 0.05to 0.30.It was cal-culated that the achieved temperature reduction during the peak period was close to 1.5K.Important energy and pol-lution benefits were also estimated.Research carried out by Millstein and Menon (2011),has investigated the impact of albedo change on the tem-perature status of various American lstein and Menon used the Weather Research and Forecasting model (Skamarock et al.,2008).Cool roofs and cool pavement technologies were considered.It was assumed that roofs and pavements represent 25%and 35%of the urban area respectively,while the considered albedo increase for roofs and pavements were +0.25and +0.15,respectively.The average albedo increase for the whole considered area ranges between 0.0and 0.115.It was calculated that cool roofs and cool pavements contribute to decrease average afternoon summertime temperatures by 0.11–0.53K.For some of the studied urban locations not statistically signif-icant temperature reduction was found.Simulations are carried out using the mesoscale model MM5(Grell et al.,1994)to estimate the impact of a possi-ble increase of albedo in Philadelphia,US (Sailor et al.,2002).It was reported that an increase of the local albedo by 0.1is responsible for an average daytime ambient tem-perature depression of about 0.3–0.5K.A simulation study aiming to investigate the impact of various mitigation technologies for the city of Atlanta,US is described in Zhou and Shepherd (2010).The Weather Research and Forecasting WRF-NOAH land surface model was used (Skamarock et al.,2008).Considering an increase of the local albedo by 100%(0.15–0.30),it was cal-culated that the ambient temperature depression was almost negligible.On the contrary,when the local albedo rises to 0.45,which corresponds to an increase by 200%,the peak ambient temperature in the city decreases by 2.5K.Simulations have been carried out to investigate the impact of a moderate increase of local albedo in Houston,US,using the mesoscale model MM5(Taha,2008b ).Roof albedo increased from 0.1to 0.3,wall albedo from 0.25to 0.3and paved surface albedo from 0.08to 0.2.It is found that the change of the albedo may decrease peak tempera-T a b l e 1C h a r a c t e r i s t i c s o f t h e N e w Y o r k a n d A t h e n s s t u d i e s o n t h e m i t i g a t i o n p o t e n t i a l o f c o o l r o o f s .R e f e r e n c e w o r kA r e a o f a p p l i c a t i o nS i m u l a t i o n t o o lF i n a l a l b e d oR e s u l t s –m i t i g a t i o n p o t e n t i a lP e a k t e m p e r a t u r e d e p r e s s i o n p e r 0.1a l b e d o i n c r e a s eS a v i o e t a l .(2006)N e w Y o r k C i t y U SP e n n S t a t e /N C A R M M 50.5T h e d a i l y a v e r a g e t e m p e r a t u r e r e d u c t i o n r a n g e s b e t w e e n 0.18K a n d 0.36K .T h e a v e r a g e 3P M r e d u c t i o n o f p e a k a m b i e n t t e m p e r a t u r e r a n g e d b e t w e e n 0.31K a n d 0.62K 0.10–0.19KS y n n e f a e t a l .(2008)A t h e n s ,G r e e c e M M 5S c e n a r i o a :0.63S c e n a r i o a :T h e a m b i e n t t e m p e r a t u r e d e p r e s s i o n a t 2m h e i g h t a t 12:00L S T v a r i e d b e t w e e n 0.5a n d 1.5K S c e n a r i o a :0.11–0.33KS c e n a r i o b :0.85S c e n a r i o b :T h e a m b i e n t t e m p e r a t u r e d e c r e a s e v a r i e d b e t w e e n 1K a n d 2.2KS c e n a r i o b :0.15–0.33KM.Santamouris /Solar Energy xxx (2012)xxx–xxx5tures up to 3.5K,while in one occasion ambient tempera-tures increase by up to 1.5K.The average temperature reduction varies from site to site and ranges between 0.3and 0.4K in residential urban areas.Cooling occurs during daytime and heating during the night.A similar simulation study aiming to evaluate the cli-matic impact of various mitigation techniques is described in Lynn et al.(2009).The study refers to the Metropolitan New York Area,US,and is carried out using mainly the MM5mesoscale simulation model.As it concerns the eval-uation of the scenario related to the increase of the urban albedo,it was considered that the albedo of the impervious surfaces changes from 0.15to 0.5.It is found that peak ambient temperature at 2m height for 12:00LST 14th August 2001,decreases between 0.25and 0.5K,while a daily average temperature decrease is close to 0.2–0.3K.Simulations studies have been performed to investigate the impact of various mitigation techniques for several cit-ies of California (Taha,2008c ).The study has been carried out using the PSU/NCAR MM5simulation tool (Dudhia,1993).Two scenarios related to the albedo change were considered and evaluated.The first scenario considered a moderate increase of the urban reflectivity while the second one a stronger increase.In particular,for the existing situ-ation,albedos ranged from 0.117to 0.152,for the moder-ate albedo change scenario from 0.18to 0.252,while for the last scenario from 0.199to 0.374.Data were calculated for Los Angeles,Pomona and San Fernando Valley.It was found that a moderate change of the urban albedo may decrease peak ambient temperatures by up to 1K,whileconcerning the possible albedo change and the correspond-ing decrease of the average ambient temperature are plot-ted.All data given in Millstein and Menon (2011)are also included.As shown,data correlate quite well in a lin-ear regression given below indicating that an albedo change of 0.1in urban areas decreases the average ambient temper-ature by 0.3K:ATD ¼a ALBINð1Þwhere a =3.11,ATD is the average temperature decrease and ALBIN the albedo increase (0<ALBIN <1).The R 2of the regression is equal to 0.85.The calculated relation between the possible albedo change and the average ambient temperature decrease is slightly higher to the one given in Synnefa et al.(2008)for Athens,Greece (2.4<a <2.85).This is logical as the Athens study considered only reflective roofs and not a general albedo change.In parallel,it is slightly lower than the one calculated in Menon et al.(2010)between the albedo change and the average urban surface temperature.The calculated reduction of the peak ambient tempera-ture ranges between 1and 3.5K.The estimated decrease of the peak ambient temperature per 0.1of albedo change varies between 0.57and 2.3K.Fig.2plots the existing data concerning the possible albedo change and the correspond-ing decrease of the peak ambient temperature.A linear relation between the two parameters has been calculated,although the correlation coefficient is not quite high because of the important scattering of the data.It is found that for an albedo change of 0.1in urban areas the peak between the possible albedo change and the corresponding decrease of the average ambient temperature M.Santamouris /Solar Energy xxx (2012)xxx–xxx 7。
- 1、下载文档前请自行甄别文档内容的完整性,平台不提供额外的编辑、内容补充、找答案等附加服务。
- 2、"仅部分预览"的文档,不可在线预览部分如存在完整性等问题,可反馈申请退款(可完整预览的文档不适用该条件!)。
- 3、如文档侵犯您的权益,请联系客服反馈,我们会尽快为您处理(人工客服工作时间:9:00-18:30)。
ADVANCED SPECTRAL METHODS FOR CLIMATIC TIME SERIESM.Ghil,1M.R.Allen,2M.D.Dettinger,3K.Ide,1D.Kondrashov,1M.E.Mann,4A.W.Robertson,1A.Saunders,1Y.Tian,1F.Varadi,1and P.Yiou5Received28August2000;revised3July2001;accepted18September2001;published XX Month2001.[1]The analysis of univariate or multivariate time series provides crucial information to describe,understand, and predict climatic variability.The discovery and im-plementation of a number of novel methods for extract-ing useful information from time series has recently revitalized this classicalfield of study.Considerable progress has also been made in interpreting the infor-mation so obtained in terms of dynamical systems the-ory.In this review we describe the connections between time series analysis and nonlinear dynamics,discuss sig-nal-to-noise enhancement,and present some of the novel methods for spectral analysis.The various steps,as well as the advantages and disadvantages of these meth-ods,are illustrated by their application to an important climatic time series,the Southern Oscillation Index.This index captures major features of interannual climate vari-ability and is used extensively in its prediction.Regional and global sea surface temperature data sets are used to illustrate multivariate spectral methods.Open questions and further prospects conclude the review.I NDEX T ERMS: 1620Climate dynamics(3309);3220Nonlinear dynam-ics;4522El Nin˜o;9820Techniques applicable in three or morefields;K EYWORDS:climate;dynamical systems;El Nin˜o;prediction;spectral analysis;time seriesCONTENTS1.Introduction and Motivation 01.1.Analysis in the time domain versus thespectral domain 01.2.Time series and nonlinear dynamics 02.Enhancing the Signal-to-Noise(S/N)Ratio 02.1.Motivation for singular spectrumanalysis(SSA) 02.2.Decomposition and reconstruction 02.3.Monte Carlo SSA 02.4.Multiscale SSA and wavelet analysis 03.Spectral Analysis Methods 03.1.Generalities and definitions 03.2.Classical spectral estimates 03.3.Maximum entropy method(MEM) 03.4.Multitaper method(MTM)..............04.Multivariate Methods.. 04.1.Principal oscillation patterns(POPs) 04.2.Multichannel SSA 05.Summary and Perspectives 05.1.Summary 05.2.Implications for understanding 05.3.Implications for prediction 0Appendix A:SSA,Spatial EOFs,and the Karhu-nen-Loe`ve Theorems 0A1.Spatial and temporal EOFs 0plementary windows for M-SSA 01.INTRODUCTION AND MOTIVATION[2]A time series provides useful information about the physical,biological,or socioeconomic system that produced it.The purpose of time series analysis is to determine some of the system’s key properties by quan-tifying certain features of the time series.These proper-ties can then help understand and predict the system’s future behavior.[3]To illustrate the ideas and methods reviewed here, we shall turn to one of the best known climatic time series.This time series is made up of monthly values of the Southern Oscillation Index(SOI).It will be intro-duced in section2.2and is shown in Figure2there. [4]At this point we merely notice that physical pro-1Department of Atmospheric Sciences and Institute ofGeophysics and Planetary Physics,University of California,Los Angeles,Los Angeles,California,USA.2Space Science and Technology Department,RutherfordAppleton Laboratory,Chilton,Didcot,England.3U.S.Geological Survey,San Diego,California,USA.4Department of Environmental Sciences,University of Vir-ginia,Charlottesville,Virginia,USA.5Laboratoire des Sciences du Climat et de l’Environnement,UMR CEA-CNRS,Gif-sur-Yvette,France.Copyright2002by the American Geophysical Union.Reviews of Geophysics,40,1/Month2002pages1-1–1-41 8755-1209/02/2000RG000092$15.00DOI10.1029/2001RG000092●1-1●1-2●Ghil et al.:CLIMATIC TIME SERIES ANALYSIS40,1/REVIEWS OF GEOPHYSICS TABLE1.Glossary of the Principal Symbols aSymbol Definition Method SectionA k(t)k th principal component(PC)of{X(t)}SSA 2.2A k b(t)k th local PC of{X(t)}at time b SSA 2.4A(t),A n continuous-and discrete-time envelope function MTM 3.4A k k th multichannel PC SSA 4.2a dilation factor of(x)3(x/a)WLTs 2.4a1lag-one autoregression coefficient SSA 2.3 {a j}true regression coefficients with order M1 {aˆj}estimates of a j with order MЈMEM 3.3 B,Bˆ(f0)true and estimated amplitude of oscillation(at frequency f0)MTM 3.4 B,Btrue and estimated dynamics matrix(with lag)POP 4.1b translation of(x)3(xϪb)WLTs 2.4b k(f)weighting function MTM 3.4C R covariance of surrogate data for{X(t)}SSA 2.3C(R)reduced covariance SSA Appendix C()lag covariance matrix of{X(t)}with lagPOP 4.1C X covariance of{X˜(t)}SSA 2.2C X grand covariance matrix SSA 4.2D trajectory matrix of{X˜(t)}in the univariate case 2.2D˜trajectory matrix of{X˜l(t)}in the multivariate case SSA 4.2d dimension of underlying attractor 2.1d(t)white noise vector in continuous time POP 4.1E k eigenvector matrix of C˜X or T˜X SSA 4.2F i right-hand sides of ODEs 2.1F(f)F test ratio MTM 3.4f frequency SSA 2.3{f k}discrete sequence of frequencies BT 3.2f N Nyquist frequency 2.3f R Rayleigh frequency MTM 3.4f0fixed frequency of pure sinusoid MTM 3.4 G()Green’s function associated with B at lagPOP 4.1 {g j}smoothing weights for SX(f k)BT 3.2I interval for dilations a WLTs 2.4i time index SSA 2.4i imaginary unit BT 3.4K number of tapers MTM 3.4a set of indices used in reconstruction SSA 2.2 L number of channels POP 4.1 M order of autoregression1 M embedding dimension 2.1 M⌬t window width SSA 2.2 M t normalization factor for R(t)SSA 2.2 MЈorder of MEM MEM 3.3 N length of{X(n⌬t)}1 NЈlength of{X˜(n⌬t)} 2.1 Nnormalization factor for T X SSA 4.2 P(f)cumulative power spectrum 3.1 p integer bandwidth parameter MTM 3.4 Q lag-zero covariance matrix of d(t)POP 4.1 R(t)reconstructed component(RC)of{X(t)}for a setSSA 2.2 Rb(t)th local RC of{X(t)}at time b SSA 2.4 R k k th multichannel RC SSA 4.2 r lag-one autocorrelation SSA 2.3 S(f)power spectrum of AR(1)process SSA 2.3 S0average of S(f)SSA 2.3 S X(f),SˆX(f)true and estimated periodogram BT 3.2 S˜X(f)correlogram BT 3.2 SX(f k),SញX(f)direct and indirect estimate of S X(f)BT 3.2 S X(f),SˆX(f)true and estimated(by{aˆj})power spectrum BT 3.2 Sˆk(f)k th eigenspectrum MTM 3.4 S r(f),S w(f)high-resolution and adaptively weighted multitaper spectrum MTM 3.4 T X grand block matrix for covariances SSA 4.2 t continuous time(tʦޒ)or discrete time(tʦޚϩ)1⌬t sampling rate1(continued)cesses usually operate in continuous time.Most mea-surements,though,are done and recorded in discrete time.Thus the SOI time series,as well as most climatic and other geophysical time series,are available in dis-crete time.1.1.Analysis in the Time Domain Versus the Spectral Domain[5]Two basic approaches to time series analysis are associated with the time domain or the spectral domain. We present them atfirst in the linear context in which the physical sciences have operated for most of the last two centuries.In this context the physical system can be described by a linear ordinary differential equation (ODE),or a system of such equations,subject to addi-tive random forcing.[6]It goes well beyond the scope of this review paper to introduce the concepts of random variables,stochastic processes,and stochastic differential equations.We re-fer the interested reader to Feller[1968,1971]for the former two concepts and to Arnold[1974]and Schuss [1980]for the latter.Many of the standard books on classical spectral methods that are cited in sections3.1 and3.2also contain good elementary introductions to stochastic processes in discrete and,sometimes,contin-uous time.[7]We concentrate here on time series in discrete time and consider thereforefirst the simple case of a scalar,linear ordinary difference equation with random forcing,X͑tϩ1͒ϭjϭ1M a j X͑tϪMϩj͒ϩ͑t͒.(1)Its constant coefficients a j determine the solutions X(t) at discrete times tϭ0,1,⅐⅐⅐,n,⅐⅐⅐.In(1)the random forcing(t)is assumed to be white in time,i.e.,uncor-related from t to tϩ1,and Gaussian at each t,with constant variance equal to unity.In the Yule[1927]and Walker[1931]method for the time domain approach, one computes the coefficients a j and the variance2 from a realization of X having length N,{X(t)Ϻ1ՅtՅN}.[8]This method is discussed further in section3.3 below,where(1)is treated as an autoregressive(AR) process of order M,which we denote by AR(M).The notation used in the present paper is summarized in Table1.The table lists the main symbols in alphabeticalTABLE1.Continued.Symbol Definition Method Sectionn⌬t discretely sampled time1U k(f)discrete Fourier transform of w k(t)MTM 3.4W sliding-window length of wavelet SSA 2.4W(a,b)wavelet transform of{X(t)}using b-translated and a-dilated((tϪb)/a)SSA 2.4W(k)lag window for SញX(f)with smoothing parameterBT 3.2W m(k)Bartlett window for SញX(f)with window length m BT 3.2w k(t)k th taper MTM 3.4Xˆ(t)observed time series1X n X(n⌬t)1X(p)p th-order differentiation of X(t)with respect to time t,d p X/dt p1{X(t)}univariate time series in tʦޒor tʦޚϩ1X˜(t)M-dimensional augmented vector of X(t) 2.1X0mean of{X(t)}SSA 2.3X I(t)reconstructed X(t)WLTs 2.4X˜(t),X˜(n⌬t)continuous-and discrete-time reconstructed signal MTM 3.4{X(t)}multivariate time series in tʦޒor tʦޚϩPOP 4.1{X˜l}multichannel augmented vector of{X˜(t)}SSA 4.2Yˆk(f)discrete Fourier transform of{X(t)w k(t)}MTM 3.4␣time-scale ratio SSA 2.4(t)additive noise MTM 3.4explained contribution to variance MTM 3.4k,⌳X k th eigenvalue and eigenvalue matrix of C X SSA 2.2⌳R projection of C R onto E X SSA 2.3k weight of k th taper MTM 3.4number of degrees of freedom of spectrum MTM 3.4(t)random forcing1k,E X k th eigenvector and eigenvector matrix of C X SSA 2.22variance of random forcing1characteristic delay time SSA 2.3X(k),ˆX(k)true and estimated lag k autocorrelation function of X(t)BT 3.2(x)mother wavelet of variable x WLTs 2.4unexplained contribution to variance MTM 3.4a Method and section number correspond to where the symbol appearsfirst or is used differently from previous sections.40,1/REVIEWS OF GEOPHYSICS Ghil et al.:CLIMATIC TIME SERIES ANALYSIS●1-3order and indicates the section where each symbol is introducedfirst.This facilitates the comparison between the methods we review,since the literature of each method tends to use its own notation.[9]The spectral domain approach is motivated by the observation that the most regular,and hence predict-able,behavior of a time series is to be periodic.This approach then proceeds to determine the periodic com-ponents embedded in the time series by computing the associated periods,amplitudes,and phases,in this order.[10]The classical implementation of the spectral do-main approach is based on the Bochner-Khinchin-Wie-ner theorem[Box and Jenkins,1970],which states that the lag autocorrelation function of a time series and its spectral density are Fourier transforms of each other. Hannan’s[1960]introduction to this approach and its implementation excels by its brevity and clarity;the so-called Blackman-Tukey implementation is presented in section3.2below.We shall use here both the more mathematical term of spectral density and the term of power spectrum,often used in the scientific and engi-neering literature,according to whether the context is more theoretical or more applied.[11]The remainder of this review is organized as follows.Section2deals mainly with signal-to-noise(S/N) ratio enhancement and introduces singular spectrum analysis(SSA)as an important andflexible tool for this enhancement.Connections between SSA and empirical orthogonal functions(EOFs)are outlined in Appendix A.Statistical tests for the reliability of SSA results are also discussed in this section,along with connections to wavelet analysis.[12]In section3we present,in succession,three methods of spectral analysis:Fourier transform based, maximum entropy,and multitaper.Both sections2and3 use the SOI time series for the purposes of illustrating the methods“in action.”In section4the multivariate extensions of the maximum entropy method and of single-channel SSA are introduced,and a few additional applications are mentioned or illustrated.The review concludes with a section on open questions,from the point of view of both the methodology and its applica-tions.1.2.Time Series and Nonlinear Dynamics[13]Before proceeding with the technical details,we give in this section a quick perspective on the“nonlinear revolution”in time series analysis.In the1960s and 1970s the scientific community found out that much of the irregularity in observed time series,which had tra-ditionally been attributed to the above mentioned ran-dom“pumping”of a linear system by infinitely many (independent)degrees of freedom(DOF),could be generated by the nonlinear interaction of a few DOF [Lorenz,1963;Smale,1967;Ruelle and Takens,1971]. This realization of the possibility of deterministic aperi-odicity or“chaos”[Gleick,1987]created quite a stir.[14]The purpose of this review is to describe briefly some of the implications of this change in outlook for time series analysis,with a special emphasis on climatic time series.Many general aspects of nonlinear time series analysis are reviewed by Drazin and King[1992], Ott et al.[1994],and Abarbanel[1996].We concentrate here on those aspects that deal with regularities and have proven most useful in studying climatic variability.[15]A connection between deterministically chaotic time series and the nonlinear dynamics generating them was attempted fairly early in the young history of“chaos theory.”The basic idea was to consider specifically a scalar,or univariate,time series with apparently irregu-lar behavior,generated by a deterministic or stochastic system.This time series could be exploited,so the think-ing went,in order to ascertain,first,whether the under-lying system has afinite number of DOF.An upper bound on this number would imply that the system is deterministic,rather than stochastic,in nature.Next,we might be able to verify that the observed irregularity arises from the fractal nature of the deterministic sys-tem’s invariant set,which would yield a fractional,rather than integer,value of this set’s dimension.Finally,one could maybe reconstruct the invariant set or even the equations governing the dynamics from the data. [16]This ambitious program[Packard et al.,1980; Roux et al.,1980;Ruelle,1981]relied essentially on the method of delays,based in turn on the Whitney[1936] embedding lemma and the Man˜e´[1981]and Takens [1981]theorems.Wefirst describe an easy connection between a univariate and a multivariate time series. [17]Let us assume that the univariate time series is the solution of a scalar nonlinear ODE of order p,X͑p͒ϭG͑X͑pϪ1͒,...,X͒.(2) This scalar higher-dimensional equation is equivalent to the following system offirst-order ODEs:X˙iϭF i͑X1,...,X j,...,X p͒,1Յi,jՅp;(3) here X˙ϵdX/dtϵX(1)and X(p)ϵd p X/dt p.It suffices to writeXϵX1,X˙1ϭX2,...,X˙pϪ1ϭX p,X˙pϭG͑X1,...,X p͒,(4) so that F1ϭX2,F2ϭX3,⅐⅐⅐,F pϭG.In other words, the successive derivatives of X(t)can be thought of as the component of a vector Xϵ(X1,⅐⅐⅐,X p).The Euclidean spaceޒp in which the vector XϭX(t)evolves is called the phase space of thefirst-order system(3).[18]Let us now change the point of view and consider system(3)for arbitrary right-hand sides F i(X),rather than for the specific F i given by(4).Such an ODE system represents a fairly general description of a dif-ferentiable dynamical system in continuous time[Ar-nold,1973,1983].We are interested atfirst in the case in which only a single time series Xˆ(t)is known,where XˆϭX i for somefixed component iϭi0or Xˆis some sufficiently smooth function of all the components X i.1-4●Ghil et al.:CLIMATIC TIME SERIES ANALYSIS40,1/REVIEWS OF GEOPHYSICSFor the solutions of such a system to be irregular,i.e., other than(asymptotically)steady or periodic,three or more DOF are necessary.Can one then go from(3)to (2)just as easily as in the opposite direction?The answer,in general is no;hence a slightly more sophisti-cated procedure needs to be applied.[19]This procedure is called the method of delays, and it tries,in some sense,to imitate the Yule-Walker inference of(1)from the time series{X(t)Ϻtϭ1,⅐⅐⅐, N}.First of all,one acknowledges that the data X(t)are typically given at discrete times tϭn⌬t only.Next,one admits that it is hard to actually get the right-hand sides F i;instead,one attempts to reconstruct the invariant set on which the solutions of(3)that satisfy certain con-straints lie.[20]In the case of conservative,Hamiltonian systems [Lichtenberg and Lieberman,1992],there are typically unique solutions through every point in phase space. The irregularity in the solutions’behavior is associated with the intricate structure of cantori[Wiggins,1988], complicated sets of folded tori characterized by a given energy of the solutions lying on them.These cantori have,in particular,finite and fractional dimension,being self-similar fractals[Mandelbrot,1982].[21]Mathematically speaking,however,Hamiltonian systems are structurally unstable[Smale,1967]in the function space of all differentiable dynamical systems. Physically speaking,on the other hand,“open”systems, in which energy is gained externally and dissipated in-ternally,abound in nature.Therefore climatic time se-ries,as well as most other time series from nature or the laboratory,are more likely to be generated by forced dissipative systems[Lorenz,1963;Ghil and Childress, 1987,chapter5].The invariant sets associated with ir-regularity here are“strange attractors”[Ruelle and Tak-ens,1971],toward which all solutions tend asymptoti-cally;that is,long-term irregular behavior in such systems is associated with these attractors.These objects are also fractal,although rigorous proofs to this effect have been much harder to give than in the case of Hamiltonian cantori[Guckenheimer and Holmes,1983; Lasota and Mackey,1994].[22]Man˜e´[1981],Ruelle[1981],and Takens[1981] had the idea,developed further by Sauer et al.[1991], that a single observed time series Xˆ(t)(where XˆϭX i0 or,more generally,Xˆϭ(X1(t),⅐⅐⅐,X p(t)))could be used to reconstruct the attractor of a forced dissipative system.The basis for this reconstruction idea is essen-tially the fact that such a solution covers the attractor densely;that is,as time increases,it will pass arbitrarily close to any point on the attractor.Time series observed in the natural environment,however,havefinite length and sampling rate,as well as significant measurement noise.[23]The embedding idea has been applied therefore most successfully to time series generated numerically or by laboratory experiments in which sufficiently long se-ries could be obtained and noise was controlled better than in nature.Broomhead and King[1986a],for in-stance,successfully applied SSA to the reconstruction of the Lorenz[1963]attractor.As we shall see,for climate and other geophysical time series,it might be possible to attain a more modest goal:to describe merely a“skele-ton”of the attractor that is formed by a few robust periodic orbits.[24]In the climate context,Lorenz[1969]had already pointed out a major stumbling block for applying the attractor reconstruction idea to large-scale atmospheric motions.While using more classical statistical methods, he showed that the recurrence time of sufficiently good analogs for weather maps was of the order of hundreds of years,at the spatial resolution of the observational network then available for the Northern Hemisphere.[25]The next best target for demonstrating from an observed time series the deterministic cause of its irreg-ularity was to show that the presumed system’s attractor had afinite and fractional dimension.Various dimen-sions,metric and topological,can be defined[Kaplan and Yorke,1979;Farmer et al.,1983];among them,the one that became the most popular,since easiest to compute,was the correlation dimension[Grassberger and Procaccia,1983].In several applications,its compu-tation proved rather reliable and hence useful.Climatic time series,however,tended again to be rather too short and noisy for comfort(see,for instance,Ruelle[1990] and Ghil et al.[1991]for a review of this controversial topic).[26]A more robust connection between classical spec-tral analysis and nonlinear dynamics seems to be pro-vided by the concept of“ghost limit cycles.”The road to chaos[Eckmann,1981]proceeds from stable equilibria, orfixed points,through stable periodic solutions,or limit cycles,and on through quasiperiodic solutions lying on tori,to strange attractors.Thefixed points and limit cycles are road posts on this highway from the simple to the complex.That is,even after having lost their stability to successively more complex and realistic solutions, these simple attractors still play a role in the observed spatial patterns and the time series generated by the system.[27]A“ghostfixed point”is afixed point that has become unstable in one or a few directions in phase space.Still,the system’s trajectories will linger near it for extended time intervals[Legras and Ghil,1985].Like-wise,a ghost limit cycle is a closed orbit that has become slightly unstable but is visited,again and again,by the system’s trajectories[Kimoto and Ghil,1993].[28]Consider the periodic solution shown in Figure 1a as embedded in Euclidean three-dimensional phase space.It is neutrally stable in the direction tangent to itself,while in the plane perpendicular to this tangent it is asymptotically stable in one direction and unstable in the other,as shown in the Poincare´section of Figure1b. In a multidimensional phase space it is plausible that the directions of stability are numerous or even infinite in number.The directions of instability,however,would40,1/REVIEWS OF GEOPHYSICS Ghil et al.:CLIMATIC TIME SERIES ANALYSIS●1-5still be few in number,for parameter values not too far from those at which the Hopf bifurcation that gave rise to the limit cycle in the first place occurs.Hence solu-tions of the full system would easily be attracted to this barely unstable limit cycle,follow it closely for one or a few turns,be ejected from its neighborhood,only to return later,again and again.[29]The analogous picture for a ghost fixed point was illustrated in detail for an atmospheric model with 25DOF by Legras and Ghil [1985;see also Ghil and Chil-dress ,1987,Figures 6.12and 6.18].Thus the “ghosts ”of fixed points and limit cycles leave their imprint on the system ’s observed spatiotemporal behavior.[30]The episodes during which the system trajectory circles near a ghost limit cycle result in nearly periodic segments of the time series and hence contribute to a spectral peak with that period.This concept was illus-trated using 40years of an atmospheric multivariate time series by Kimoto and Ghil [1993]for the so-called in-traseasonal oscillations of the Northern Hemisphere [see also Ghil and Mo ,1991a].We shall show in the subsequent sections of the present review,in particular section 2.2,how this concept can be generalized to associate multiple spectral peaks with a robust skeleton of the attractor,as proposed by Vautard and Ghil [1989].2.ENHANCING THE SIGNAL-TO-NOISE (S/N)RATIO2.1.Motivation for Singular Spectrum Analysis (SSA)[31]SSA is designed to extract information from short and noisy time series and thus provide insight into the unknown or only partially known dynamics of the un-derlying system that generated the series [Broomheadand King ,1986a;Fraedrich ,1986;Vautard and Ghil ,1989].We outline here the method for univariate time series and generalize for multivariate ones in section 4.2.[32]The analogies between SSA and spatial EOFs are summarized in Appendix A,along with the basis of both in the Karhunen-Loe `ve theory of random fields and of stationary random processes.Multichannel SSA (see section 4.2)is numerically analogous to the extended EOF (EEOF)algorithm of Weare and Nasstrom [1982].The two different names arise from the origin of the former in the dynamical systems analysis of univariate time series,while the latter had its origins in the princi-pal component analysis of meteorological fields.The two approaches lead to different methods for the choice of key parameters,such as the fixed or variable window width,and hence to differences in the way of interpret-ing results.[33]The starting point of SSA is to embed a time series {X (t )Ϻt ϭ1,⅐⅐⅐,N }in a vector space of dimen-sion M ,i.e.,to represent it as a trajectory in the phase space of the hypothetical system that generated {X (t )}.In concrete terms this is equivalent to representing the behavior of the system by a succession of overlapping “views ”of the series through a sliding M -point window.[34]Let us assume,for the moment,that X (t )is anobservable function Xˆ(t )of a noise-free system ’s depen-dent variables X i (t ),as de fined in (4),and that the function that maps the p variables {X i (t )Ϻi ϭ1,⅐⅐⅐,p }into the single variable X (t )has certain properties that make it generic in the dynamical systems sense of Smale [1967].Assume,moreover,that M Ͼ2d ϩ1,where d is the dimension of the underlying attractor on which the system evolves,and that d is known and finite.If so,then the representation of the system in the “delay coordinates ”described in (5)below will share key topo-logical properties with a representation in any coordi-nate system.This is a consequence of Whitney ’s [1936]embedding lemma and indicates the potential value of SSA in the qualitative analysis of the dynamics of non-linear systems [Broomhead and King ,1986a,1986b;Sauer et al .,1991].The quantitative interpretation of SSA results in terms of attractor dimensions is fraught with dif ficulties,however,as pointed out by a number of authors [Broomhead et al .,1987;Vautard and Ghil ,1989;Palus ˘and Dvor ˘a ´k ,1992].[35]We therefore use SSA here mainly (1)for data-adaptive signal-to-noise (S/N)enhancement and associ-ated data compression and (2)to find the attractor ’s skeleton,given by its least unstable limit cycles (see again Figure 1above).The embedding procedure ap-plied to do so constructs a sequence {X ˜(t )}of M -dimensional vectors from the original time series X ,by using lagged copies of the scalar data {X (t )Ϻ1Յt ՅN },X ˜͑t ͒ϭ͑X ͑t ͒,X ͑t ϩ1͒,...,X ͑t ϩM Ϫ1͒͒;(5)the vectors X ˜(t )are indexed by t ϭ1,⅐⅐⅐,N Ј,where N ЈϭN ϪM ϩ1.Figure 1.Schematic diagram of a ghost limit cycle.Figure 1a is a perspective sketch of the limit cycle (bold curve)in a three-dimensional Euclidean space.Light solid curves indicate two distinct trajectories that approach the limit cycle in a direction in which it is stable and leave its neighborhood in an unstable direction.Figure 1b is a sketch of the projection of four representative trajectories (light curves)onto a Poincare ´section (i.e.,a plane intersecting transversally the limit cycle)in a neighborhood of the limit cycle.The figure ’s mutually per-pendicular directions (bold lines)of stability (inward pointing arrows)and instability (outward pointing arrows)can form,in general,an arbitrary angle with each other.The shape of such a limit cycle need not be elliptic,as shown in the figure,except near the Hopf bifurcation point where it arises.Reprinted from Ghil and Yiou [1996]with permission of Springer-Verlag.1-6●Ghil et al.:CLIMATIC TIME SERIES ANALYSIS 40,1/REVIEWS OF GEOPHYSICS[36]SSA allows one to unravel the information em-bedded in the delay-coordinate phase space by decom-posing the sequence of augmented vectors thus obtained into elementary patterns of behavior.It does so by providing data-adaptivefilters that help separate the time series into components that are statistically inde-pendent,at zero lag,in the augmented vector space of interest.These components can be classified essentially into trends,oscillatory patterns,and noise.As we shall see,it is an important feature of SSA that the trends need not be linear and that the oscillations can be amplitude and phase modulated.[37]SSA has been applied extensively to the study of climate variability,as well as to other areas in the phys-ical and life sciences.The climatic applications include the analysis of paleoclimatic time series[Vautard and Ghil,1989;Yiou et al.,1994,1995],interdecadal climate variability[Ghil and Vautard,1991;Allen and Smith, 1994;Plaut et al.,1995;Robertson and Mechoso,1998],as well as interannual[Rasmusson et al.,1990;Keppenne and Ghil,1992]and intraseasonal[Ghil and Mo,1991a, 1991b]oscillations.SSA algorithms and their properties have been investigated further by Penland et al.[1991],Allen[1992],Vautard et al.[1992],and Yiou et al.[2000]. The SSA Toolkitfirst documented by Dettinger et al. [1995a]was built,largely but not exclusively,around this technique.2.2.Decomposition and Reconstruction[38]In this section we illustrate the fundamental SSA formulae with the classical example of a climatic time series,the Southern Oscillation Index(SOI).SOI is a climatic index connected with the recurring El Nin˜o conditions in the tropical Pacific.It is defined usually as the difference between the monthly means of the sea level pressures at Tahiti and at Darwin(Australia).We use this definition and the monthly data given in the archive /pacs/additional_ analyses/soi.html.[39]The SOI data in this archive are based on the time series at each of the two stations being deseason-alized and normalized[Ropelewski and Jones,1987].The seasonal cycle is removed by subtracting the average of the values for each calendar month over a reference interval,in this case1951–1980.The residues from this operation,called monthly anomalies in the climatologi-cal literature,are then normalized with respect to the standard deviation computed over the same interval.[40]The archived SOI data for1866–1997are from the Climate Research Unit of the University of East Anglia,and those for1998–1999are from the Climate Prediction Center of the U.S.National Centers for En-vironmental Prediction(NCEP).They are obtained by taking the difference between the anomalies at Tahiti and those at Darwin and dividing by the standard devi-ation of this difference over the same30-year reference interval.The time interval we consider here goes from January1942to June1999,during which no observations are missing at either station;this yields Nϭ690raw data points.Note that this raw SOI time series is cen-tered and normalized over the reference interval1951–1980,but not over the entire interval of interest.We show in Figure2the SOI obtained from this raw data set.It actually has meanϪ0.0761and standard deviation equal to1.0677.All subsequentfigures,however,use a version of the series that has been correctly centered over the interval January1942to June1999.[41]SSA is based on calculating the principal direc-tions of extension of the sequence of augmented vectors {X˜(t)Ϻtϭ1,⅐⅐⅐,NЈ}in phase space.The MϫM covariance matrix C X can be estimated directly from the data as a Toeplitz matrix with constant diagonals;that is, its entries c ij depend only on the lag͉iϪj͉[cf.Vautard and Ghil,1989]:c ijϭ1NϪ͉iϪj͉tϭ1NϪ͉iϪj͉X͑t͒X͑tϩ͉iϪj͉͒.(6)The eigenelements{(k,k)Ϻkϭ1,⅐⅐⅐,M}of C X are then obtained by solvingC Xkϭkk.(7) The eigenvaluek equals the partial variance in the directionk,and the sum of thek,i.e.,the trace of C X, gives the total variance of the original time series X(t).[42]An equivalent formulation of(7),which will prove useful further on,is given by forming the MϫM matrix E X that has the eigenvectorsk as its columns and the diagonal matrix⌳X whose elements are the eigen-valuesk,in decreasing order:E X t C X E Xϭ⌳X;(8) Figure2.Variations of the Southern Oscillation Index(SOI) between January1942and June1999.Time on the abscissa is in calendar years,and SOI on the ordinate is normalized by its standard deviation.40,1/REVIEWS OF GEOPHYSICS Ghil et al.:CLIMATIC TIME SERIES ANALYSIS●1-7。