外文翻译---采用遗传算法优化加工夹具定位和加紧位置
薄壁盒形件真空吸附装夹变形的计算方法研究

薄壁盒形件真空吸附装夹变形的计算方法研究李勇;江磊;马术文;刘蕾;陈雪梅【摘要】为了较为准确地预测薄壁盒形件的装夹变形量,研究了不同误差形式下薄壁盒形件的真空吸附装夹变形趋势,设计了装夹变形数值求解和有限元仿真求解的具体流程,形成了比较完整的薄壁盒形件真空吸附装夹变形求解算法,通过实例验证了本方法的有效性,并为盒形类零件的装夹变形分析提供理论支持,为装夹误差控制及夹具设计提供参考.【期刊名称】《机械制造与自动化》【年(卷),期】2018(047)003【总页数】5页(P49-52,62)【关键词】盒形件;装夹变形;真空吸附;有限元仿真【作者】李勇;江磊;马术文;刘蕾;陈雪梅【作者单位】西南交通大学机械工程学院,四川成都610031;西南交通大学机械工程学院,四川成都610031;西南交通大学机械工程学院,四川成都610031;成都飞机工业(集团)有限责任公司制造工程部,四川成都610092;成都飞机工业(集团)有限责任公司制造工程部,四川成都610092【正文语种】中文【中图分类】TH161+.30 引言薄壁类零件由于刚度较差,在装夹时容易产生变形。
装夹变形是加工误差的主要来源,最终会影响零件的加工精度,严重者会引起加工缺陷,导致零件报废。
因此,研究薄壁类零件的装夹变形和优化装夹方案对控制和提高加工精度具有重要的意义。
为了减小装夹变形对薄壁件精度的影响,提高其加工质量,众多研究者主要从装夹变形预测、控制,装夹方案优化,装夹方式的选择等方面做了大量的研究。
秦国华等针对薄壁件的装夹布局方案,利用有限元方法获取神经网络训练样本,提出了基于神经网络与遗传算法的装夹变形“分析-预测-控制”方法[1-2]。
于金等利用有限元模拟了薄壁框体类零件在铣削加工中不同装夹位置的变形,得出了最终的优化装夹布局方案,并做了相关的验证试验[3]。
王军等借助数值模拟方法对铝合金薄壁壳体件装夹方案进行了优选,分析了在集中载荷与均布载荷作用下,装夹位置、装夹顺序及加载方式对其变形的影响[4]。
(完整word版)外文翻译-遗传算法

What is a genetic algorithm?●Methods of representation●Methods of selection●Methods of change●Other problem—solving techniquesConcisely stated,a genetic algorithm (or GA for short)is a programming technique that mimics biological evolution as a problem-solving strategy。
Given a specific problem to solve, the input to the GA is a set of potential solutions to that problem,encoded in some fashion,and a metric called a fitness function that allows each candidate to be quantitatively evaluated. These candidates may be solutions already known to work,with the aim of the GA being to improve them, but more often they are generated at random.The GA then evaluates each candidate according to the fitness function. In a pool of randomly generated candidates,of course,most will not work at all, and these will be deleted. However,purely by chance, a few may hold promise — they may show activity,even if only weak and imperfect activity,toward solving the problem.These promising candidates are kept and allowed to reproduce. Multiple copies are made of them, but the copies are not perfect;random changes are introduced during the copying process. These digital offspring then go on to the next generation,forming a new pool of candidate solutions,and are subjected to a second round of fitness evaluation。
基于改进遗传算法的数控加工中心机床工艺路线优化
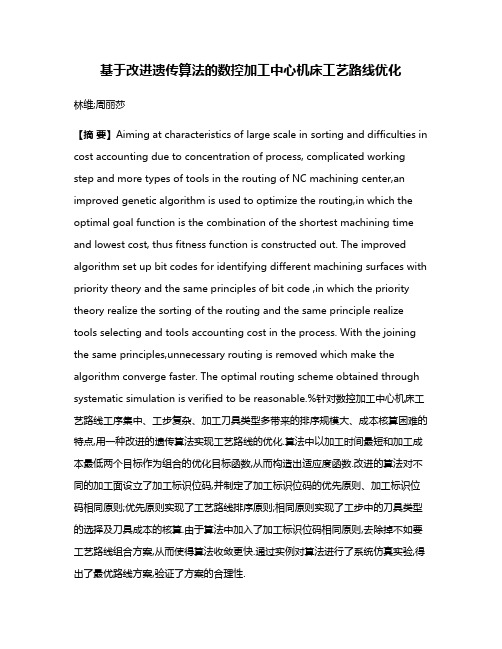
基于改进遗传算法的数控加工中心机床工艺路线优化林维;周丽莎【摘要】Aiming at characteristics of large scale in sorting and difficulties in cost accounting due to concentration of process, complicated working step and more types of tools in the routing of NC machining center,an improved genetic algorithm is used to optimize the routing,in which the optimal goal function is the combination of the shortest machining time and lowest cost, thus fitness function is constructed out. The improved algorithm set up bit codes for identifying different machining surfaces with priority theory and the same principles of bit code ,in which the priority theory realize the sorting of the routing and the same principle realize tools selecting and tools accounting cost in the process. With the joining the same principles,unnecessary routing is removed which make the algorithm converge faster. The optimal routing scheme obtained through systematic simulation is verified to be reasonable.%针对数控加工中心机床工艺路线工序集中、工步复杂、加工刀具类型多带来的排序规模大、成本核算困难的特点,用一种改进的遗传算法实现工艺路线的优化.算法中以加工时间最短和加工成本最低两个目标作为组合的优化目标函数,从而构造出适应度函数.改进的算法对不同的加工面设立了加工标识位码,并制定了加工标识位码的优先原则、加工标识位码相同原则;优先原则实现了工艺路线排序原则;相同原则实现了工步中的刀具类型的选择及刀具成本的核算.由于算法中加入了加工标识位码相同原则,去除掉不如要工艺路线组合方案,从而使得算法收敛更快.通过实例对算法进行了系统仿真实验,得出了最优路线方案,验证了方案的合理性.【期刊名称】《机械设计与制造》【年(卷),期】2011(000)007【总页数】3页(P175-177)【关键词】改进遗传算法;数控加工中心机床;工艺路线优化;加工标识位码【作者】林维;周丽莎【作者单位】贵州大学,机械工程学院,贵阳,550003;贵州大学,机械工程学院,贵阳,550003【正文语种】中文【中图分类】TH16;TG6591 引言制定数控加工中心机床零件的工艺路线时要求在一次装夹下完成尽可能多的工序,利用传统的编制工艺经验很难得到一个最优路线。
遗传算法 课题申报书范文
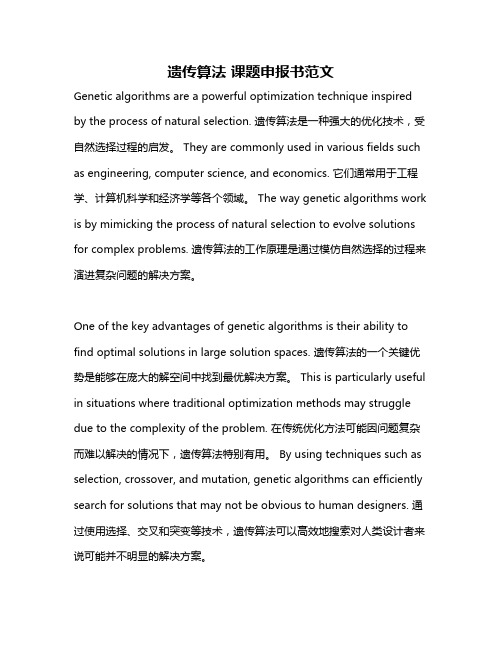
遗传算法课题申报书范文Genetic algorithms are a powerful optimization technique inspired by the process of natural selection. 遗传算法是一种强大的优化技术,受自然选择过程的启发。
They are commonly used in various fields such as engineering, computer science, and economics. 它们通常用于工程学、计算机科学和经济学等各个领域。
The way genetic algorithms work is by mimicking the process of natural selection to evolve solutions for complex problems. 遗传算法的工作原理是通过模仿自然选择的过程来演进复杂问题的解决方案。
One of the key advantages of genetic algorithms is their ability to find optimal solutions in large solution spaces. 遗传算法的一个关键优势是能够在庞大的解空间中找到最优解决方案。
This is particularly useful in situations where traditional optimization methods may struggle due to the complexity of the problem. 在传统优化方法可能因问题复杂而难以解决的情况下,遗传算法特别有用。
By using techniques such as selection, crossover, and mutation, genetic algorithms can efficiently search for solutions that may not be obvious to human designers. 通过使用选择、交叉和突变等技术,遗传算法可以高效地搜索对人类设计者来说可能并不明显的解决方案。
遗传算法中英文对照外文翻译文献
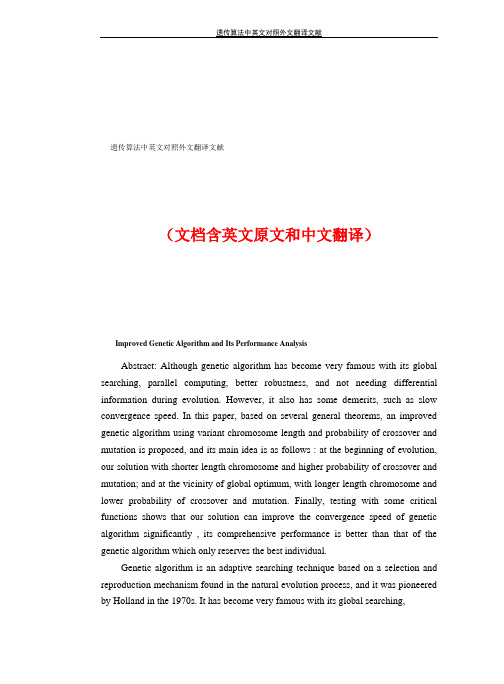
遗传算法中英文对照外文翻译文献遗传算法中英文对照外文翻译文献(文档含英文原文和中文翻译)Improved Genetic Algorithm and Its Performance AnalysisAbstract: Although genetic algorithm has become very famous with its global searching, parallel computing, better robustness, and not needing differential information during evolution. However, it also has some demerits, such as slow convergence speed. In this paper, based on several general theorems, an improved genetic algorithm using variant chromosome length and probability of crossover and mutation is proposed, and its main idea is as follows : at the beginning of evolution, our solution with shorter length chromosome and higher probability of crossover and mutation; and at the vicinity of global optimum, with longer length chromosome and lower probability of crossover and mutation. Finally, testing with some critical functions shows that our solution can improve the convergence speed of genetic algorithm significantly , its comprehensive performance is better than that of the genetic algorithm which only reserves the best individual.Genetic algorithm is an adaptive searching technique based on a selection and reproduction mechanism found in the natural evolution process, and it was pioneered by Holland in the 1970s. It has become very famous with its global searching,________________________________ 遗传算法中英文对照外文翻译文献 ________________________________ parallel computing, better robustness, and not needing differential information during evolution. However, it also has some demerits, such as poor local searching, premature converging, as well as slow convergence speed. In recent years, these problems have been studied.In this paper, an improved genetic algorithm with variant chromosome length andvariant probability is proposed. Testing with some critical functions shows that it can improve the convergence speed significantly, and its comprehensive performance is better than that of the genetic algorithm which only reserves the best individual.In section 1, our new approach is proposed. Through optimization examples, insection 2, the efficiency of our algorithm is compared with the genetic algorithm which only reserves the best individual. And section 3 gives out the conclusions. Finally, some proofs of relative theorems are collected and presented in appendix.1 Description of the algorithm1.1 Some theoremsBefore proposing our approach, we give out some general theorems (see appendix)as follows: Let us assume there is just one variable (multivariable can be divided into many sections, one section for one variable) x £ [ a, b ] , x £ R, and chromosome length with binary encoding is 1.Theorem 1 Minimal resolution of chromosome isb 一 a2l — 1Theorem 3 Mathematical expectation Ec(x) of chromosome searching stepwith one-point crossover iswhere Pc is the probability of crossover.Theorem 4 Mathematical expectation Em ( x ) of chromosome searching step with bit mutation isE m ( x ) = ( b- a) P m 遗传算法中英文对照外文翻译文献Theorem 2 wi = 2l -1 2 i -1 Weight value of the ith bit of chromosome is(i = 1,2,・・・l )E *)= P c1.2 Mechanism of algorithmDuring evolutionary process, we presume that value domains of variable are fixed, and the probability of crossover is a constant, so from Theorem 1 and 3, we know that the longer chromosome length is, the smaller searching step of chromosome, and the higher resolution; and vice versa. Meanwhile, crossover probability is in direct proportion to searching step. From Theorem 4, changing the length of chromosome does not affect searching step of mutation, while mutation probability is also in direct proportion to searching step.At the beginning of evolution, shorter length chromosome( can be too shorter, otherwise it is harmful to population diversity ) and higher probability of crossover and mutation increases searching step, which can carry out greater domain searching, and avoid falling into local optimum. While at the vicinity of global optimum, longer length chromosome and lower probability of crossover and mutation will decrease searching step, and longer length chromosome also improves resolution of mutation, which avoid wandering near the global optimum, and speeds up algorithm converging.Finally, it should be pointed out that chromosome length changing keeps individual fitness unchanged, hence it does not affect select ion ( with roulette wheel selection) .2.3 Description of the algorithmOwing to basic genetic algorithm not converging on the global optimum, while the genetic algorithm which reserves the best individual at current generation can, our approach adopts this policy. During evolutionary process, we track cumulative average of individual average fitness up to current generation. It is written as1 X G x(t)= G f vg (t)t=1where G is the current evolutionary generation, 'avg is individual average fitness.When the cumulative average fitness increases to k times ( k> 1, k £ R) of initial individual average fitness, we change chromosome length to m times ( m is a positive integer ) of itself , and reduce probability of crossover and mutation, which_______________________________ 遗传算法中英文对照外文翻译文献________________________________can improve individual resolution and reduce searching step, and speed up algorithm converging. The procedure is as follows:Step 1 Initialize population, and calculate individual average fitness f avg0, and set change parameter flag. Flag equal to 1.Step 2 Based on reserving the best individual of current generation, carry out selection, regeneration, crossover and mutation, and calculate cumulative average of individual average fitness up to current generation 'avg ;f avgStep 3 If f vgg0 三k and Flag equals 1, increase chromosome length to m times of itself, and reduce probability of crossover and mutation, and set Flag equal to 0; otherwise continue evolving.Step 4 If end condition is satisfied, stop; otherwise go to Step 2.2 Test and analysisWe adopt the following two critical functions to test our approach, and compare it with the genetic algorithm which only reserves the best individual:sin 2 弋 x2 + y2 - 0.5 [1 + 0.01( 2 + y 2)]x, y G [-5,5]f (x, y) = 4 - (x2 + 2y2 - 0.3cos(3n x) - 0.4cos(4n y))x, y G [-1,1]22. 1 Analysis of convergenceDuring function testing, we carry out the following policies: roulette wheel select ion, one point crossover, bit mutation, and the size of population is 60, l is chromosome length, Pc and Pm are the probability of crossover and mutation respectively. And we randomly select four genetic algorithms reserving best individual with various fixed chromosome length and probability of crossover and mutation to compare with our approach. Tab. 1 gives the average converging generation in 100 tests.In our approach, we adopt initial parameter l0= 10, Pc0= 0.3, Pm0= 0.1 and k= 1.2, when changing parameter condition is satisfied, we adjust parameters to l= 30, Pc= 0.1, Pm= 0.01.From Tab. 1, we know that our approach improves convergence speed of genetic algorithm significantly and it accords with above analysis.2.2 Analysis of online and offline performanceQuantitative evaluation methods of genetic algorithm are proposed by Dejong, including online and offline performance. The former tests dynamic performance; and the latter evaluates convergence performance. To better analyze online and offline performance of testing function, w e multiply fitness of each individual by 10, and we give a curve of 4 000 and 1 000 generations for fl and f2, respectively.(a) onlineFig. 1 Online and offline performance of fl(a) online (b) onlineFig. 2 Online and offline performance of f2From Fig. 1 and Fig. 2, we know that online performance of our approach is just little worse than that of the fourth case, but it is much better than that of the second, third and fifth case, whose online performances are nearly the same. At the same time, offline performance of our approach is better than that of other four cases.3 ConclusionIn this paper, based on some general theorems, an improved genetic algorithmusing variant chromosome length and probability of crossover and mutation is proposed. Testing with some critical functions shows that it can improve convergence speed of genetic algorithm significantly, and its comprehensive performance is better than that of the genetic algorithm which only reserves the best individual.AppendixWith the supposed conditions of section 1, we know that the validation of Theorem 1 and Theorem 2 are obvious.Theorem 3 Mathematical expectation Ec(x) of chromosome searching step with one point crossover isb - a PEc(x) = 21 cwhere Pc is the probability of crossover.Proof As shown in Fig. A1, we assume that crossover happens on the kth locus, i. e. parent,s locus from k to l do not change, and genes on the locus from 1 to k are exchanged.During crossover, change probability of genes on the locus from 1 to k is 2 (“1” to “0” or “0” to “1”). So, after crossover, mathematical expectation of chromosome searching step on locus from 1 to k is1 chromosome is equal, namely l Pc. Therefore, after crossover, mathematical expectation of chromosome searching step isE (x ) = T 1 -• P • E (x ) c l c ckk =1Substituting Eq. ( A1) into Eq. ( A2) , we obtain 尸 11 b - a p b - a p • (b - a ) 1 E (x ) = T • P • — •• (2k -1) = 7c • • [(2z -1) ― l ] = ——— (1 一 )c l c 2 21 — 121 21 — 1 21 21 —1 k =1 lb - a _where l is large,-——-口 0, so E (x ) 口 -——P2l — 1 c 21 c 遗传算法中英文对照外文翻译文献 厂 / 、 T 1 T 1 b — a - 1E (x )="—w ="一• ---------- • 2 j -1 二 •ck2 j 2 21 -1 2j =1 j =1 Furthermore, probability of taking • (2k -1) place crossover on each locus ofFig. A1 One point crossoverTheorem 4 Mathematical expectation E m(")of chromosome searching step with bit mutation E m (x)—(b a)* P m, where Pm is the probability of mutation.Proof Mutation probability of genes on each locus of chromosome is equal, say Pm, therefore, mathematical expectation of mutation searching step is一i i - b —a b b- aE (x) = P w = P•—a«2i-1 = P•—a q2,-1)= (b- a) •m m i m 21 -1 m 2 i -1 mi=1 i=1一种新的改进遗传算法及其性能分析摘要:虽然遗传算法以其全局搜索、并行计算、更好的健壮性以及在进化过程中不需要求导而著称,但是它仍然有一定的缺陷,比如收敛速度慢。
中文翻译-通过夹具布局设计和夹紧力的优化控制变形
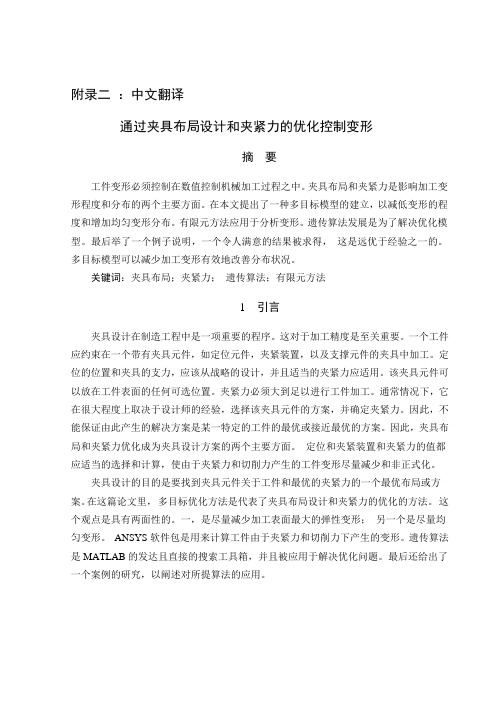
附录二:中文翻译通过夹具布局设计和夹紧力的优化控制变形摘要工件变形必须控制在数值控制机械加工过程之中。
夹具布局和夹紧力是影响加工变形程度和分布的两个主要方面。
在本文提出了一种多目标模型的建立,以减低变形的程度和增加均匀变形分布。
有限元方法应用于分析变形。
遗传算法发展是为了解决优化模型。
最后举了一个例子说明,一个令人满意的结果被求得,这是远优于经验之一的。
多目标模型可以减少加工变形有效地改善分布状况。
关键词:夹具布局;夹紧力;遗传算法;有限元方法1 引言夹具设计在制造工程中是一项重要的程序。
这对于加工精度是至关重要。
一个工件应约束在一个带有夹具元件,如定位元件,夹紧装置,以及支撑元件的夹具中加工。
定位的位置和夹具的支力,应该从战略的设计,并且适当的夹紧力应适用。
该夹具元件可以放在工件表面的任何可选位置。
夹紧力必须大到足以进行工件加工。
通常情况下,它在很大程度上取决于设计师的经验,选择该夹具元件的方案,并确定夹紧力。
因此,不能保证由此产生的解决方案是某一特定的工件的最优或接近最优的方案。
因此,夹具布局和夹紧力优化成为夹具设计方案的两个主要方面。
定位和夹紧装置和夹紧力的值都应适当的选择和计算,使由于夹紧力和切削力产生的工件变形尽量减少和非正式化。
夹具设计的目的是要找到夹具元件关于工件和最优的夹紧力的一个最优布局或方案。
在这篇论文里,多目标优化方法是代表了夹具布局设计和夹紧力的优化的方法。
这个观点是具有两面性的。
一,是尽量减少加工表面最大的弹性变形;另一个是尽量均匀变形。
ANSYS软件包是用来计算工件由于夹紧力和切削力下产生的变形。
遗传算法是MATLAB的发达且直接的搜索工具箱,并且被应用于解决优化问题。
最后还给出了一个案例的研究,以阐述对所提算法的应用。
2文献回顾随着优化方法在工业中的广泛运用,近几年夹具设计优化已获得了更多的利益。
夹具设计优化包括夹具布局优化和夹紧力优化。
King 和Hutter提出了一种使用刚体模型的夹具-工件系统来优化夹具布局设计的方法。
基于遗传算法的夹具布局和加紧力优化技术
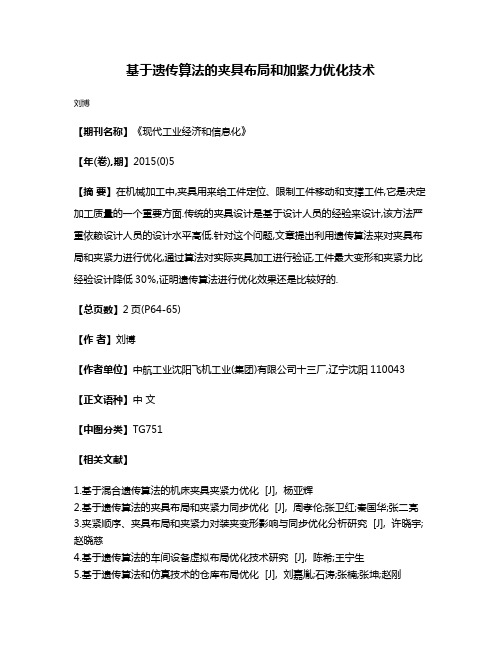
基于遗传算法的夹具布局和加紧力优化技术
刘博
【期刊名称】《现代工业经济和信息化》
【年(卷),期】2015(0)5
【摘要】在机械加工中,夹具用来给工件定位、限制工件移动和支撑工件,它是决定加工质量的一个重要方面.传统的夹具设计是基于设计人员的经验来设计,该方法严重依赖设计人员的设计水平高低.针对这个问题,文章提出利用遗传算法来对夹具布局和夹紧力进行优化,通过算法对实际夹具加工进行验证,工件最大变形和夹紧力比经验设计降低30%,证明遗传算法进行优化效果还是比较好的.
【总页数】2页(P64-65)
【作者】刘博
【作者单位】中航工业沈阳飞机工业(集团)有限公司十三厂,辽宁沈阳110043【正文语种】中文
【中图分类】TG751
【相关文献】
1.基于混合遗传算法的机床夹具夹紧力优化 [J], 杨亚辉
2.基于遗传算法的夹具布局和夹紧力同步优化 [J], 周孝伦;张卫红;秦国华;张二亮
3.夹紧顺序、夹具布局和夹紧力对装夹变形影响与同步优化分析研究 [J], 许晓宇;赵晓慈
4.基于遗传算法的车间设备虚拟布局优化技术研究 [J], 陈希;王宁生
5.基于遗传算法和仿真技术的仓库布局优化 [J], 刘嘉胤;石涛;张楠;张坤;赵刚
因版权原因,仅展示原文概要,查看原文内容请购买。
基于遗传算法的涂胶机器人装夹优化设计
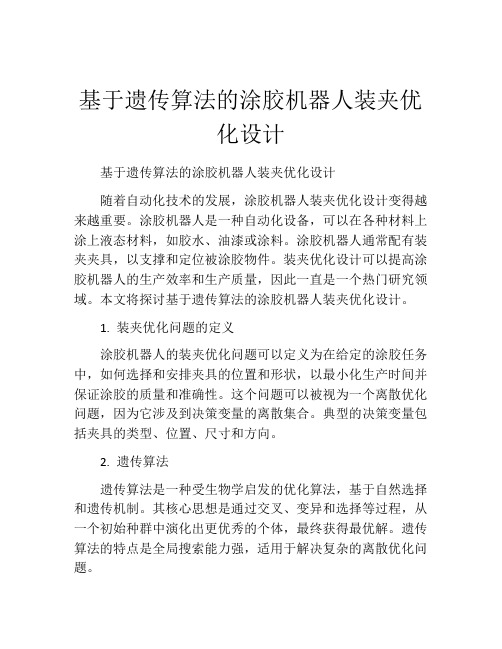
基于遗传算法的涂胶机器人装夹优化设计基于遗传算法的涂胶机器人装夹优化设计随着自动化技术的发展,涂胶机器人装夹优化设计变得越来越重要。
涂胶机器人是一种自动化设备,可以在各种材料上涂上液态材料,如胶水、油漆或涂料。
涂胶机器人通常配有装夹夹具,以支撑和定位被涂胶物件。
装夹优化设计可以提高涂胶机器人的生产效率和生产质量,因此一直是一个热门研究领域。
本文将探讨基于遗传算法的涂胶机器人装夹优化设计。
1. 装夹优化问题的定义涂胶机器人的装夹优化问题可以定义为在给定的涂胶任务中,如何选择和安排夹具的位置和形状,以最小化生产时间并保证涂胶的质量和准确性。
这个问题可以被视为一个离散优化问题,因为它涉及到决策变量的离散集合。
典型的决策变量包括夹具的类型、位置、尺寸和方向。
2. 遗传算法遗传算法是一种受生物学启发的优化算法,基于自然选择和遗传机制。
其核心思想是通过交叉、变异和选择等过程,从一个初始种群中演化出更优秀的个体,最终获得最优解。
遗传算法的特点是全局搜索能力强,适用于解决复杂的离散优化问题。
3. 基于遗传算法的涂胶机器人装夹优化设计基于遗传算法的涂胶机器人装夹优化设计通常包括以下步骤:(1)初始化种群初始种群可以根据涂胶任务和夹具要求自动生成。
种群中的个体包括夹具的类型、位置、尺寸和方向等信息。
(2)适应度函数适应度函数是用来评估每个个体的优劣程度的。
通常,适应度函数的值被定义为与涂胶任务相关的成本或时间,以及夹具的质量和准确性等方面的指标。
(3)选择选择操作是为了从当前种群中选出优良的个体。
通常,选择方法包括轮盘赌选择、竞争选择和排名选择等。
(4)交叉交叉操作是为了产生新的个体。
它通常涉及到两个个体的基因交换操作。
交叉操作可以通过单点交叉、两点交叉或均匀交叉等方法实现。
(5)变异变异操作是为了增加种群的多样性和随机性。
它通常涉及到单个基因的改变。
变异可以通过位变异、反转变异或插入变异等方法实现。
(6)终止条件终止条件是为了保证遗传算法在有限时间内获得较好的解。
- 1、下载文档前请自行甄别文档内容的完整性,平台不提供额外的编辑、内容补充、找答案等附加服务。
- 2、"仅部分预览"的文档,不可在线预览部分如存在完整性等问题,可反馈申请退款(可完整预览的文档不适用该条件!)。
- 3、如文档侵犯您的权益,请联系客服反馈,我们会尽快为您处理(人工客服工作时间:9:00-18:30)。
附录Machining fixture locating and clamping position optimization usinggenetic algorithmsNecmettin Kaya*Department of Mechanical Engineering, Uludag University, Go¨ru¨kle, Bursa 16059, Turkey Received 8 July 2004; accepted 26 May 2005Available online 6 September 2005AbstractDeformation of the workpiece may cause dimensional problems in machining. Supports and locators are used in order to reduce the error caused by elastic deformation of the workpiece. The optimization of support, locator and clamp locations is a critical problem to minimize the geometric error in workpiece machining. In this paper, the application of genetic algorithms (GAs) to the fixture layout optimization is presented to handle fixture layout optimization problem. A genetic algorithm based approach is developed to optimise fixture layout through integrating a finite element code running in batch mode to compute the objective function values for each generation. Case studies are given to illustrate the application of proposed approach. Chromosome library approach is used to decrease the total solution time. Developed GA keeps track of previously analyzed designs; therefore the numbers of function evaluations are decreased about 93%. The results of this approach show that the fixture layout optimization problems are multi-modal problems. Optimized designs do not have any apparent similarities although they provide very similar performances.Keywords: Fixture design; Genetic algorithms; Optimization1. IntroductionFixtures are used to locate and constrain a workpiece during a machining operation, minimizing workpiece and fixture tooling deflections due to clamping and cutting forces are critical to ensuring accuracy of the machining operation. Traditionally, machining fixtures are designed and manufactured through trial-and-error, which prove to be both expensive and time-consuming to the manufacturing process. To ensure a workpiece is manufactured according to specified dimensions and tolerances, it must be appropriately located and clamped, making it imperative to develop tools that will eliminate costly and time-consuming trial-and-error designs. Properworkpiece location and fixture design are crucial to product quality in terms of precision, accuracy and finish of the machined part.Theoretically, the 3-2-1 locating principle can satisfactorily locate all prismatic shaped workpieces. This method provides the maximum rigidity with the minimum number of fixture elements. To position a part from a kinematic point of view means constraining the six degrees of freedom of a free moving body (three translations and three rotations). Three supports are positioned below the part to establish the location of the workpiece on its vertical axis. Locators are placed on two peripheral edges and intended to establish the location of the workpiece on the x and y horizontal axes. Properly locating the workpiece in the fixture is vital to the overall accuracy and repeatability of the manufacturing process. Locators should be positioned as far apart as possible and should be placed on machined surfaces wherever possible. Supports are usually placed to encompass the center of gravity of a workpiece and positioned as far apart as possible to maintain its stability. The primary responsibility of a clamp in fixture is to secure the part against the locators and supports. Clamps should not be expected to resist the cutting forces generated in the machining operation.For a given number of fixture elements, the machining fixture synthesis problem is the finding optimal layout or positions of the fixture elements around the workpiece. In this paper, a method for fixture layout optimization using genetic algorithms is presented. The optimization objective is to search for a 2D fixture layout that minimizes the maximum elastic deformation at different locations of the workpiece. ANSYS program has been used for calculating the deflection of the part under clamping and cutting forces. Two case studies are given to illustrate the proposed approach.2. Review of related worksFixture design has received considerable attention in recent years. However, little attention has been focused on the optimum fixture layout design. Menassa and DeVries[1]used FEA for calculating deflections using the minimization of the workpiece deflection at selected points as the design criterion. The design problem was to determine the position of supports. Meyer and Liou[2] presented an approach that uses linear programming technique to synthesize fixtures for dynamic machining conditions. Solution for the minimum clamping forces and locator forces is given. Li and Melkote[3]used a nonlinear programming method to solve the layout optimization problem. The method minimizes workpiece location errors due to localized elasticdeformation of the workpiece. Roy andLiao[4]developed a heuristic method to plan for the best supporting and clamping positions. Tao et al.[5]presented a geometrical reasoning methodology for determining the optimal clamping points and clamping sequence for arbitrarily shaped workpieces. Liao and Hu[6]presented a system for fixture configuration analysis based on a dynamic model which analyses the fixture–workpiece system subject to time-varying machining loads. The influence of clamping placement is also investigated. Li and Melkote[7]presented a fixture layout and clamping force optimal synthesis approach that accounts for workpiece dynamics during machining. A combined fixture layout and clamping force optimization procedure presented.They used the contact elasticity modeling method that accounts for the influence of workpiece rigid body dynamics during machining. Amaral et al. [8] used ANSYS to verify fixture design integrity. They employed 3-2-1 method. The optimization analysis is performed in ANSYS. Tan et al. [9] described the modeling, analysis and verification of optimal fixturing configurations by the methods of force closure, optimization and finite element modeling.Most of the above studies use linear or nonlinear programming methods which often do not give global optimum solution. All of the fixture layout optimization procedures start with an initial feasible layout. Solutions from these methods are depending on the initial fixture layout. They do not consider the fixture layout optimization on overall workpiece deformation.The GAs has been proven to be useful technique in solving optimization problems in engineering [10–12]. Fixture design has a large solution space and requires a search tool to find the best design. Few researchers have used the GAs for fixture design and fixture layout problems. Kumar et al. [13] have applied both GAs and neural networks for designing a fixture. Marcelin[14]has used GAs to the optimization of support positions. Vallapuzha et al.[15]presented GA based optimization method that uses spatial coordinates to represent the locations of fixture elements. Fixture layout optimization procedure was implemented using MATLAB and the genetic algorithm toolbox. HYPERMESH and MSC/NASTRAN were used for FE model. Vallapuzha et al. [16] presented results of an extensive investigation into the relative effectiveness of various optimization methods. They showed that continuous GA yielded the best quality solutions. Li and Shiu [17] determined the optimal fixture configuration design for sheet metal assembly using GA. MSC/NASTRAN has been used for fitness evaluation. Liao [18] presented a method to automatically select the optimal numbers oflocators and clamps as well as their optimal positions in sheet metal assembly fixtures. Krishnakumar and Melkote [19] developed a fixture layout optimization technique that uses the GA to find the fixture layout that minimizes the deformation of the machined surface due to clamping and machining forces over the entire tool path. Locator and clamp positions are specified by node numbers. A built-in finite element solver was developed.Some of the studies do not consider the optimization of the layout for entire tool path and chip removal is not taken into account. Some of the studies used node numbers as design parameters.In this study, a GA tool has been developed to find the optimal locator and clamp positions in 2D workpiece. Distances from the reference edges as design parameters are used rather than FEA node numbers. Fitness values of real encoded GA chromosomes are obtained from the results of FEA. ANSYS has been used for FEA calculations. A chromosome library approach is used in order to decrease the solution time. Developed GA tool is tested on two test problems. Two case studies are given to illustrate the developed approach. Main contributions of this paper can be summarized as follows:(1) developed a GA code integrated with a commercial finite element solver;(2) GA uses chromosome library in order to decrease the computation time;(3) real design parameters are used rather than FEA node numbers;(4) chip removal is taken into account while tool forces moving on the workpiece.3. Genetic algorithm conceptsGenetic algorithms were first developed by John Holland. Goldberg [10] published a book explaining the theory and application examples of genetic algorithm in details. A genetic algorithm is a random search technique that mimics some mechanisms of natural evolution. The algorithm works on a population of designs. The population evolves from generation to generation, gradually improving its adaptation to the environment through natural selection; fitter individuals have better chances of transmitting their characteristics to later generations.In the algorithm, the selection of the natural environment is replaced by artificial selection based on a computed fitness for each design. The term fitness is used to designate the chromosome’s chances of survival and it is essentially the objective function of the optimization problem. The chromosomes that define characteristics of biological beings are replaced by strings of numerical values representing the designvariables.GA is recognized to be different than traditional gradient based optimization techniques in the following four major ways [10]:1. GAs work with a coding of the design variables and parameters in the problem, rather than with the actual parameters themselves.2. GAs makes use of population-type search. Many different design points are evaluated during each iteration instead of sequentially moving from one point to the next.3. GAs needs only a fitness or objective function value. No derivatives or gradients are necessary.4. GAs use probabilistic transition rules to find new design points for exploration rather than using deterministic rules based on gradient information to find these new points.4. Approach4.1. Fixture positioning principlesIn machining process, fixtures are used to keep workpieces in a desirable position for operations. The most important criteria for fixturing are workpiece position accuracy and workpiece deformation. A good fixture design minimizes workpiece geometric and machining accuracy errors. Another fixturing requirement is that the fixture must limit deformation of the workpiece. It is important to consider the cutting forces as well as the clamping forces. Without adequate fixture support, machining operations do not conform to designed tolerances. Finite element analysis is a powerful tool in the resolution of some of these problems [22].Common locating method for prismatic parts is 3-2-1 method. This method provides the maximum rigidity with the minimum number of fixture elements. A workpiece in 3D may be positively located by means of six points positioned so that they restrict nine degrees of freedom of the workpiece. The other three degrees of freedom are removed by clamp elements. An example layout for 2D workpiece based 3-2-1 locating principle is shown in Fig. 4.Fig. 4. 3-2-1 locating layout for 2D prismatic workpieceThe number of locating faces must not exceed two so as to avoid a redundant location. Based on the 3-2-1 fixturing principle there are two locating planes for accurate location containing two and one locators. Therefore, there are maximum of two side clampings against each locating plane. Clamping forces are always directed towards the locators in order to force the workpiece to contact all locators. The clamping point should be positioned opposite the positioning points to prevent the workpiece from being distorted by the clamping force.Since the machining forces travel along the machining area, it is necessary to ensure that the reaction forces at locators are positive for all the time. Any negative reaction force indicates that the workpiece is free from fixture elements. In other words, loss of contact or the separation between the workpiece and fixture element might happen when the reaction force is negative. Positive reaction forces at the locators ensure that the workpiece maintains contact with all the locators from the beginning of the cut to the end. The clamping forces should be just sufficient to constrain and locate the workpiece without causing distortion or damage to the workpiece. Clamping force optimization is not considered in this paper.4.2. Genetic algorithm based fixture layout optimization approachIn real design problems, the number of design parameters can be very large and their influence on the objective function can be very complicated. The objective function must be smooth and a procedure is needed to compute gradients. Genetic algorithms strongly differ in conception from other search methods, including traditional optimization methods and other stochastic methods [23]. By applying GAs to fixture layout optimization, an optimal or group of sub-optimal solutions can be obtained.In this study, optimum locator and clamp positions are determined using genetic algorithms. They are ideally suited for the fixture layout optimization problem since no direct analytical relationship exists between the machining error and the fixture layout. Since the GA deals with only the design variables and objective function value for a particular fixture layout, no gradient or auxiliary information is needed [19].The flowchart of the proposed approach is given in Fig. 5.Fixture layout optimization is implemented using developed software written in Delphi language named GenFix. Displacement values are calculated in ANSYS software [24]. The execution of ANSYS in GenFix is simply done by WinExec function in Delphi. The interaction between GenFix and ANSYS is implemented in four steps:(1) Locator and clamp positions are extracted from binary string as real parameters.(2) These parameters and ANSYS input batch file (modeling, solution and post processing commands) are sent to ANSYS using WinExec function.(3) Displacement values are written to a text file after solution.(4) GenFix reads this file and computes fitness value for current locator and clamp positions.In order to reduce the computation time, chromosomes and fitness values are stored in a library for further evaluation. GenFix first checks if current chromosome’s fitness value has been calculated before. If not, locator positions are sent to ANSYS, otherwise fitness values are taken from the library. During generating of the initial population, every chromosome is checked whether it is feasible or not. If the constraint is violated, it is eliminated and new chromosome is created. This process creates entirely feasible initial population. This ensures that workpiece is stable under the action of clamping and cutting forces for every chromosome in the initial population.The written GA program was validated using two test cases. The first test case uses Himmelblau function [21]. In the second test case, the GA program was used to optimise the support positions of a beam under uniform loading.5. Fixture layout optimization case studiesThe fixture layout optimization problem is defined as: finding the positions of the locators and clamps, so that workpiece deformation at specific region is minimized. Note that number of locators and clamps are not design parameter, since they are known and fixed for the 3-2-1 locating scheme. Hence, the design parameters areselected as locator and clamp positions. Friction is not considered in this paper. Two case studies are given to illustrate the proposed approach.6. ConclusionIn this paper, an evolutionary optimization technique of fixture layout optimization is presented. ANSYS has been used for FE calculation of fitness values. It is seen that the combined genetic algorithm and FE method approach seems to be a powerful approach for present type problems. GA approach is particularly suited for problems where there does not exist a well-defined mathematical relationship between the objective function and the design variables. The results prove the success of the application of GAs for the fixture layout optimization problems.In this study, the major obstacle for GA application in fixture layout optimization is the high computation cost. Re-meshing of the workpiece is required for every chromosome in the population. But, usages of chromosome library, the number of FE evaluations are decreased from 6000 to 415. This results in a tremendous gain in computational efficiency. The other way to decrease the solution time is to use distributed computation in a local area network.The results of this approach show that the fixture layout optimization problems are multi-modal problems. Optimized designs do not have any apparent similarities although they provide very similar performances. It is shown that fixture layout problems are multi-modal therefore heuristic rules for fixture design should be used in GA to select best design among others.Fig. 5. The flowchart of the proposed methodology and ANSYS interface.采用遗传算法优化加工夹具定位和加紧位置摘要:工件变形的问题可能导致机械加工中的空间问题。