DPMdencens之学解
原神帝利耶悉学院名字
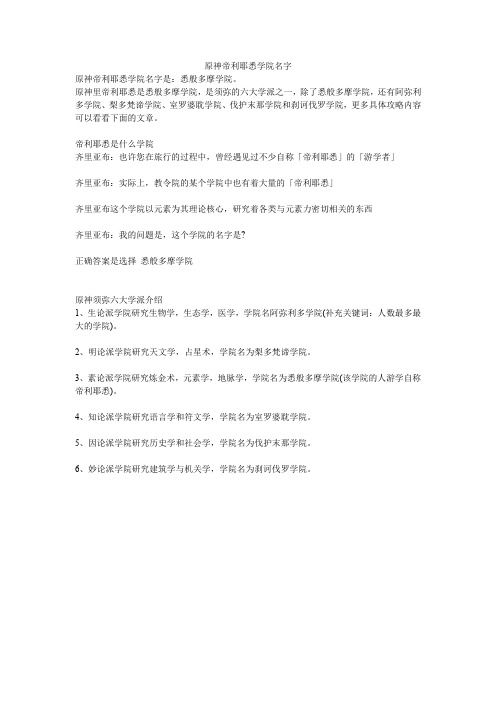
原神帝利耶悉学院名字
原神帝利耶悉学院名字是:悉般多摩学院。
原神里帝利耶悉是悉般多摩学院,是须弥的六大学派之一,除了悉般多摩学院,还有阿弥利多学院、梨多梵谛学院、室罗婆耽学院、伐护末那学院和刹诃伐罗学院,更多具体攻略内容可以看看下面的文章。
帝利耶悉是什么学院
齐里亚布:也许您在旅行的过程中,曾经遇见过不少自称「帝利耶悉」的「游学者」
齐里亚布:实际上,教令院的某个学院中也有着大量的「帝利耶悉」
齐里亚布这个学院以元素为其理论核心,研究着各类与元素力密切相关的东西
齐里亚布:我的问题是,这个学院的名字是?
正确答案是选择悉般多摩学院
原神须弥六大学派介绍
1、生论派学院研究生物学,生态学,医学,学院名阿弥利多学院(补充关键词:人数最多最大的学院)。
2、明论派学院研究天文学,占星术,学院名为梨多梵谛学院。
3、素论派学院研究炼金术,元素学,地脉学,学院名为悉般多摩学院(该学院的人游学自称帝利耶悉)。
4、知论派学院研究语言学和符文学,学院名为室罗婆耽学院。
5、因论派学院研究历史学和社会学,学院名为伐护末那学院。
6、妙论派学院研究建筑学与机关学,学院名为刹诃伐罗学院。
理解入门解译法术笔记

理解入门解译法术笔记
首先,我们可以从法术的基本概念和原理入手,解释该法术的
起源、用途和特点。
这包括法术的分类、属性、元素等方面的解释,以及法术在不同情境下的应用和效果。
其次,我们可以从法术的使用和施法方式来解释。
这包括对于
法术的咒语、手势、材料等要素的解读,以及对于法术能量的引导
和释放的过程进行详细描述。
另外,我们还可以从法术的影响和局限性来进行解释。
这包括
对于法术的强弱、持续时间、影响范围等方面的分析,以及法术在
特定条件下可能出现的副作用或者反噬。
此外,还可以从法术的历史和传承角度进行解释。
这包括对于
该法术在不同文化或者不同历史时期下的应用和传承情况进行探讨,以及法术背后可能存在的传说或者神话故事进行介绍。
最后,我们还可以从玩家或者使用者的角度来解释理解入门解
译法术笔记。
这包括对于玩家如何学习和掌握该法术的技巧和要点
进行讲解,以及在游戏或者小说情节中如何巧妙运用该法术进行战
斗或者解谜等方面的介绍。
总的来说,理解入门解译法术笔记是一个涉及到多个方面的复杂问题,需要我们从不同角度进行全面的解释和回答。
希望以上回答能够满足你的要求。
苍翠秘典——写给德鲁伊们的法术包
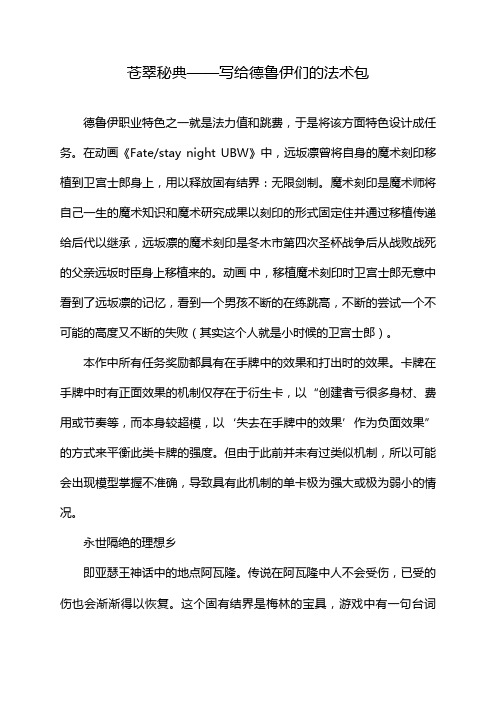
苍翠秘典——写给德鲁伊们的法术包德鲁伊职业特色之一就是法力值和跳费,于是将该方面特色设计成任务。
在动画《Fate/stay night UBW》中,远坂凛曾将自身的魔术刻印移植到卫宫士郎身上,用以释放固有结界:无限剑制。
魔术刻印是魔术师将自己一生的魔术知识和魔术研究成果以刻印的形式固定住并通过移植传递给后代以继承,远坂凛的魔术刻印是冬木市第四次圣杯战争后从战败战死的父亲远坂时臣身上移植来的。
动画中,移植魔术刻印时卫宫士郎无意中看到了远坂凛的记忆,看到一个男孩不断的在练跳高,不断的尝试一个不可能的高度又不断的失败(其实这个人就是小时候的卫宫士郎)。
本作中所有任务奖励都具有在手牌中的效果和打出时的效果。
卡牌在手牌中时有正面效果的机制仅存在于衍生卡,以“创建者亏很多身材、费用或节奏等,而本身较超模,以‘失去在手牌中的效果’作为负面效果”的方式来平衡此类卡牌的强度。
但由于此前并未有过类似机制,所以可能会出现模型掌握不准确,导致具有此机制的单卡极为强大或极为弱小的情况。
永世隔绝的理想乡即亚瑟王神话中的地点阿瓦隆。
传说在阿瓦隆中人不会受伤,已受的伤也会渐渐得以恢复。
这个固有结界是梅林的宝具,游戏中有一句台词“Garden of Avalon”,但是,日本人的英文发音大家都懂,听起来很像“大连有个阿瓦隆”,于是成了梗。
Garden of XXX都有大连有个XXX 的梗,比如虚荣的空中庭园(Hanging Gardens of Babylon)也有大连有个巴比伦的绰号。
吉尔伽美什等人拥有的技能,指获取财富的能力,拥有此技能便被认为随便做点什么就可以赚很多钱。
游戏中效果为充能(宝具释放所消耗的资源,fgo中技能无消耗有CD,而宝具无CD有消耗)获得量提升,本作中效果也为增加法力值(包括空法力水晶、法力水晶和仅在本回合使用的法力水晶)获得数量。
冯契 广义认识论的英文
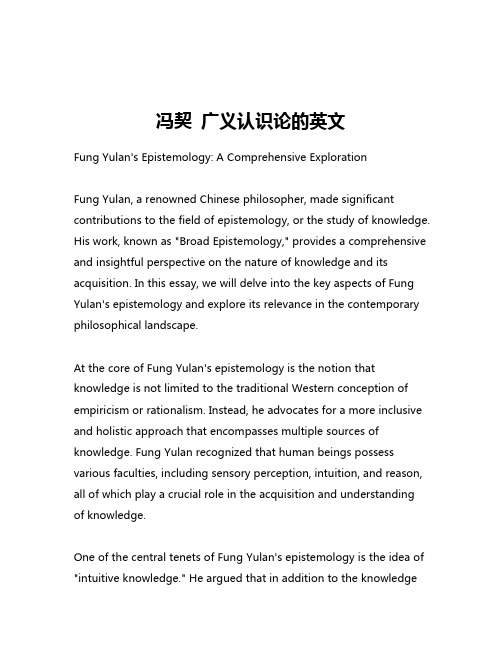
冯契广义认识论的英文Fung Yulan's Epistemology: A Comprehensive ExplorationFung Yulan, a renowned Chinese philosopher, made significant contributions to the field of epistemology, or the study of knowledge. His work, known as "Broad Epistemology," provides a comprehensive and insightful perspective on the nature of knowledge and its acquisition. In this essay, we will delve into the key aspects of Fung Yulan's epistemology and explore its relevance in the contemporary philosophical landscape.At the core of Fung Yulan's epistemology is the notion that knowledge is not limited to the traditional Western conception of empiricism or rationalism. Instead, he advocates for a more inclusive and holistic approach that encompasses multiple sources of knowledge. Fung Yulan recognized that human beings possess various faculties, including sensory perception, intuition, and reason, all of which play a crucial role in the acquisition and understandingof knowledge.One of the central tenets of Fung Yulan's epistemology is the idea of "intuitive knowledge." He argued that in addition to the knowledgederived from sensory experience and logical reasoning, human beings possess an innate capacity for intuitive understanding. This intuitive knowledge, often referred to as "direct apprehension," allows individuals to grasp the essence of things without the need for extensive analysis or external validation. Fung Yulan believed that this form of knowledge is particularly important in the realm of ethics, where moral truths can be directly perceived and experienced.Another key aspect of Fung Yulan's epistemology is the recognition of the role of culture and tradition in shaping our understanding of the world. He emphasized that knowledge is not solely the product of individual cognition but is also influenced by the social, historical, and cultural contexts in which it is situated. Fung Yulan argued that the Chinese philosophical tradition, with its emphasis on holistic thinking and the interconnectedness of all things, offers a unique perspective that complements and enhances the Western epistemological frameworks.Furthermore, Fung Yulan's epistemology challenged the rigid dichotomy between subjectivity and objectivity that often characterizes Western philosophical discourse. He believed that the subjective and objective realms are not mutually exclusive but rather exist in a dynamic interplay. Fung Yulan recognized that our understanding of the world is shaped by both our individual perspectives and the objective realities that exist independently ofour perceptions.One of the key implications of Fung Yulan's epistemology is its potential to bridge the gap between Eastern and Western philosophical traditions. By acknowledging the validity of multiple sources of knowledge, including intuition and cultural traditions, Fung Yulan's work offers a more inclusive and nuanced approach to understanding the nature of knowledge. This perspective can facilitate cross-cultural dialogue and the exchange of ideas, ultimately contributing to a richer and more comprehensive understanding of the human experience.Moreover, Fung Yulan's epistemology has relevance in contemporary discussions surrounding the nature of knowledge and its acquisition. In an era marked by the proliferation of information and the increasing complexity of social and technological systems, the need for a more holistic and flexible approach to knowledge becomes increasingly apparent. Fung Yulan's emphasis on the complementary roles of reason, intuition, and cultural context provides a valuable framework for navigating the challenges of the modern world.In conclusion, Fung Yulan's epistemology, or "Broad Epistemology," offers a comprehensive and insightful perspective on the nature of knowledge and its acquisition. By recognizing the multifaceted nature of human cognition and the influence of cultural traditions,Fung Yulan's work challenges the limitations of traditional Western epistemological frameworks and paves the way for a more inclusive and nuanced understanding of the human experience. As we continue to grapple with the complexities of the modern world, Fung Yulan's contributions to the field of epistemology remain highly relevant and worthy of further exploration and discussion.。
梦魇谱面解析
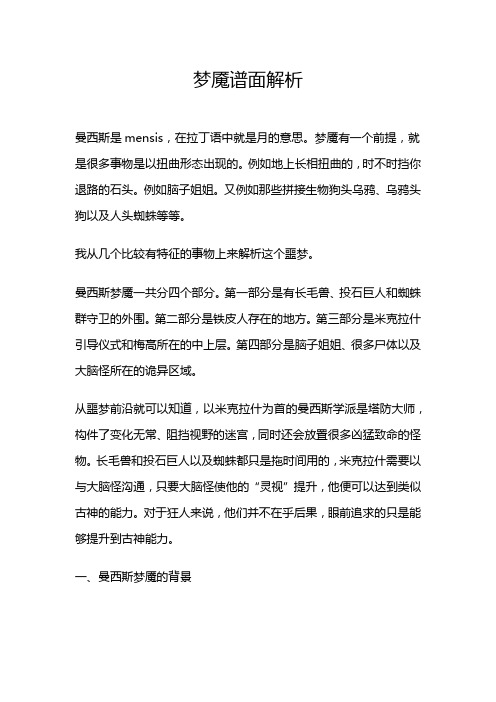
梦魇谱面解析曼西斯是mensis,在拉丁语中就是月的意思。
梦魇有一个前提,就是很多事物是以扭曲形态出现的。
例如地上长相扭曲的,时不时挡你退路的石头。
例如脑子姐姐。
又例如那些拼接生物狗头乌鸦、乌鸦头狗以及人头蜘蛛等等。
我从几个比较有特征的事物上来解析这个噩梦。
曼西斯梦魇一共分四个部分。
第一部分是有长毛兽、投石巨人和蜘蛛群守卫的外围。
第二部分是铁皮人存在的地方。
第三部分是米克拉什引导仪式和梅高所在的中上层。
第四部分是脑子姐姐、很多尸体以及大脑怪所在的诡异区域。
从噩梦前沿就可以知道,以米克拉什为首的曼西斯学派是塔防大师,构件了变化无常、阻挡视野的迷宫,同时还会放置很多凶猛致命的怪物。
长毛兽和投石巨人以及蜘蛛都只是拖时间用的,米克拉什需要以与大脑怪沟通,只要大脑怪使他的“灵视”提升,他便可以达到类似古神的能力。
对于狂人来说,他们并不在乎后果,眼前追求的只是能够提升到古神能力。
一、曼西斯梦魇的背景重生古神的仪式因为罗姆控制了时间而不断重载,曼西斯首脑需要一个梦境空间来使自己不会在亚楠梦境醒来之时忘却所有事物。
同样,月之魔物的梦境也可以使猎人不会在亚楠梦境醒来后忘记自己的任务进行到什么阶段。
因此在被罗姆截取的重生古神降临前的这个片段不断重载的时间里,曼西斯首脑在自己创建的梦境中已经截获了梅高出生时的时空(这或许是通过类似阿米戈达拉传送方式达到的),另外在塔楼底下又截获了某个古墓(拜尔金沃斯或者治愈教会尚未发掘过的)来挖掘未知的古神。
于是他们找到了大脑怪。
可能是因为筑梦者们本身扭曲的思维或是其他客观原因,在梦境里看到的大脑怪有可能已经不是它原本的样貌。
米克拉什并不知道外面的时间重置已经被玩家打破,仪式已经发生。
但按照他的尸体描述为木乃伊可以看出,亚楠梦境重置之前,他们在自己的梦境里也已经度过了相当长的时间。
可以推断,曼西斯首脑在梦境中的进度也极其缓慢,甚至有点显而易见的是,他们的方式方法出了很大问题,也遭遇了很多意想不到的事情。
库恩在科学哲学中首次使用了“范式”(paradigm)术语吗
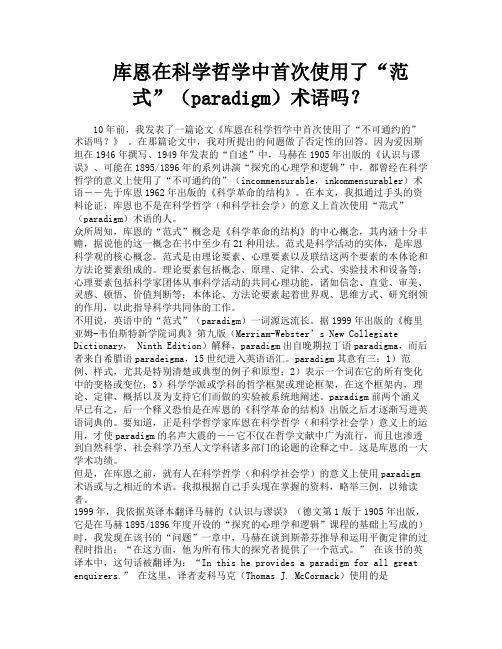
库恩在科学哲学中首次使用了“范式”(paradigm)术语吗?10年前,我发表了一篇论文《库恩在科学哲学中首次使用了“不可通约的”术语吗?》。
在那篇论文中,我对所提出的问题做了否定性的回答。
因为爱因斯坦在1946年撰写、1949年发表的“自述”中,马赫在1905年出版的《认识与谬误》、可能在1895/1896年的系列讲演“探究的心理学和逻辑”中,都曾经在科学哲学的意义上使用了“不可通约的”(incommensurable,inkommensurabler)术语――先于库恩1962年出版的《科学革命的结构》。
在本文,我拟通过手头的资料论证,库恩也不是在科学哲学(和科学社会学)的意义上首次使用“范式”(paradigm)术语的人。
众所周知,库恩的“范式”概念是《科学革命的结构》的中心概念,其内涵十分丰赡,据说他的这一概念在书中至少有21种用法。
范式是科学活动的实体,是库恩科学观的核心概念。
范式是由理论要素、心理要素以及联结这两个要素的本体论和方法论要素组成的。
理论要素包括概念、原理、定律、公式、实验技术和设备等;心理要素包括科学家团体从事科学活动的共同心理功能,诸如信念、直觉、审美、灵感、顿悟、价值判断等;本体论、方法论要素起着世界观、思维方式、研究纲领的作用,以此指导科学共同体的工作。
不用说,英语中的“范式”(paradigm)一词源远流长。
据1999年出版的《梅里亚姆-韦伯斯特新学院词典》第九版(Merriam-Webster’s New Collegiate Dictionary, Ninth Edition)解释,paradigm出自晚期拉丁语paradigma,而后者来自希腊语paradeigma,15世纪进入英语语汇。
paradigm其意有三:1)范例、样式,尤其是特别清楚或典型的例子和原型;2)表示一个词在它的所有变化中的变格或变位;3)科学学派或学科的哲学框架或理论框架,在这个框架内,理论、定律、概括以及为支持它们而做的实验被系统地阐述。
解精微论篇原文译文
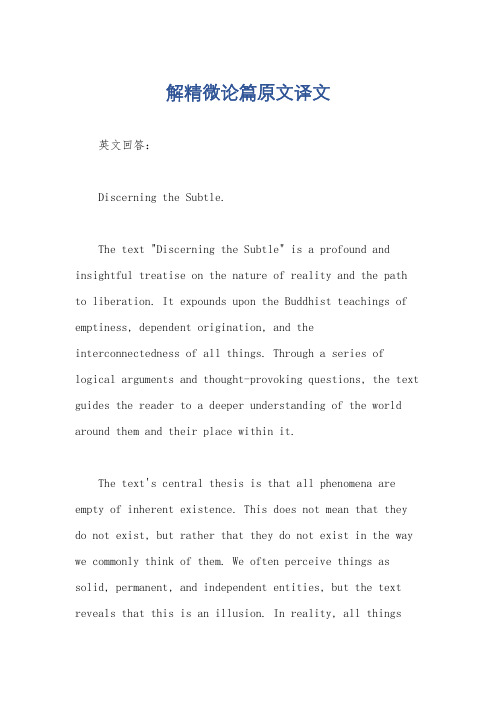
解精微论篇原文译文英文回答:Discerning the Subtle.The text "Discerning the Subtle" is a profound and insightful treatise on the nature of reality and the path to liberation. It expounds upon the Buddhist teachings of emptiness, dependent origination, and the interconnectedness of all things. Through a series of logical arguments and thought-provoking questions, the text guides the reader to a deeper understanding of the world around them and their place within it.The text's central thesis is that all phenomena are empty of inherent existence. This does not mean that they do not exist, but rather that they do not exist in the way we commonly think of them. We often perceive things as solid, permanent, and independent entities, but the text reveals that this is an illusion. In reality, all thingsare constantly changing and interconnected, arising and passing away in a never-ending cycle of existence.This understanding of emptiness has profoundimplications for our understanding of ourselves and the world. If we cling to the belief that things have aninherent existence, we will inevitably experience suffering. We will be attached to things that are constantly changing and impermanent, and we will be disappointed and frustrated when they inevitably fall apart. However, if we can let goof this attachment and see things as they truly are, wewill be free from suffering.The text also emphasizes the importance of dependent origination. This is the idea that all things are interconnected and interdependent. Nothing exists in isolation, but rather is the result of a complex web of causes and conditions. This understanding has implications for our ethical behavior. If we realize that our actions have consequences not only for ourselves but for others, we will be more likely to act in a compassionate and responsible way.Finally, the text discusses the interconnectedness of all things. This is the idea that all beings are ultimately connected to each other. We are all part of a vast web of life, and our actions have an impact on all other beings. This understanding has implications for our sense of compassion and empathy. If we realize that we are all interconnected, we will be more likely to care for others and to work for the common good.The text "Discerning the Subtle" is a challenging and thought-provoking treatise that offers a deep and profound understanding of the nature of reality. It is a text that can be studied and contemplated for a lifetime, and its teachings can help us to live more compassionate, ethical, and enlightened lives.中文回答:解精微论。
密教模拟器蜘蛛之门的谜题

密教模拟器蜘蛛之门的谜题
用通用的办法,把雄鹿之门通晓者放进入梦,然后暂停游戏,往里面丢知识卡,这时把书店里的书都读完了,至少所有知识卡的2阶都应该有了,都丢进去。
如果发现文字有所变化,告诉玩家已经走到一半了,那就说明属性正确,但知识等级不够。
这是可以用杯、灯、启前往蜘蛛之门。
密教模拟器蜘蛛之门解锁攻略
蜘蛛之门
雄鹿之门:通晓者+8阶或更高灯之知识/8阶或更高杯之知识/8阶或更高启之知识=蜘蛛之门
用通用的办法,把雄鹿之门:通晓者放进入梦,然后暂停游戏,往里面丢知识卡。
这时应该把书店里的书都读完了,至少所有知识卡的2阶都应该有了,都丢进去。
如果发现文字有所变化,告诉你已经走到一半了,那就说明属性正确,但知识等级不够。
通过尝试会发现,杯、灯、启都可以用于前往蜘蛛之门。
根据前三道门的规律,这里需要的是8阶知识。
- 1、下载文档前请自行甄别文档内容的完整性,平台不提供额外的编辑、内容补充、找答案等附加服务。
- 2、"仅部分预览"的文档,不可在线预览部分如存在完整性等问题,可反馈申请退款(可完整预览的文档不适用该条件!)。
- 3、如文档侵犯您的权益,请联系客服反馈,我们会尽快为您处理(人工客服工作时间:9:00-18:30)。
DPMdencens {DPpackage} R Documentation Bayesian density estimation for interval-censored data using a DPM ofnormalsDescriptionThis function generates a posterior density sample for a Dirichlet proc ess mixture of normals model for interval-censored data区间删失数据.UsageDPMdencens(left,right,ngrid=100,grid=NULL,prior,mcmc,state,status) Argumentsleft a vector or matrix giving the lower limit for each response variable. Note that the responses are defined on the entire real line and that unknown limitsshould be indicated by NA.right a vector or matrix giving the upper limit for each response variable. Note that the responses are defined on the entire real line and that unknown limitsshould be indicated by NA.ngrid number of grid points where the density estimate is evaluated. The default value is 100.grid matrix of dimension ngrid*nvar of grid points where the density estimate is evaluated. The default value is NULL and the grid is chosen according to therange of the interval limits.prior a list giving the prior information. The list includes the following parameter: a0 and b0 giving the hyperparameters for prior distribution of theprecision parameter of the Dirichlet process prior, alpha giving the value ofthe precision parameter (it must be specified if a0 is missing, see detailsbelow), nu2 and psiinv2 giving the hyperparameters of the inverted Wishartprior distribution for the scale matrix, Psi1, of the inverted Wishart part ofthe baseline distribution, tau1 and tau2 giving the hyperparameters for thegamma prior distribution of the scale parameter k0 of the normal part of thebaseline distribution,m2 and s2 giving the mean and the covariance of thenormal prior for the mean, m1, of the normal component of the baselinedistribution, respectively, nu1 and psiinv1 (it must be specified if nu2 ismissing, see details below) giving the hyperparameters of the invertedWishart part of the baseline distribution and, m1giving the mean of the normal part of the baseline distribution (it must be specified if m2 is missing, seedetails below) and, k0 giving the scale parameter of the normal part of thebaseline distribution (it must be specified if tau1 is missing, see detailsbelow).mcmc a list giving the MCMC parameters. The list must include the following integers: nburn giving the number of burn-in scans, nskip giving the thinning interval,nsave giving the total number of scans to be saved,and ndisplay giving the number of saved scans to be displayed on screen(the function reports on the screen when every ndisplay iterations have beencarried out).state a list giving the current value of the parameters. This list is used if the current analysis is the continuation of a previous analysis.status a logical variable indicating whether this run is new (TRUE) or the continuation of a previous analysis (FALSE). In the latter case the currentvalue of the parameters must be specified in the object state.DetailsThis generic function fits a Dirichlet process mixture of normal model for density estimation (Escobar and West, 1995) based on interval-censored data:yij in [lij,uij),i=1,…,n, j=1,…,m,yi | mui, Sigmai ~ N(mui,Sigmai), i=1,…,n,(mui,Sigmai) | G ~ G,G | alpha, G0 ~ DP(alpha G0),where, yi=(yi1,…,yim), and the baseline distribution is the conjugate normal-inverted-Wishart distribution,G0 = N(mu| m1, (1/k0) Sigma) IW (Sigma | nu1, psi1)To complete the model specification, independent hyperpriors are assumed (optional),alpha | a0, b0 ~ Gamma(a0,b0)m1 | m2, s2 ~ N(m2,s2)k0 | tau1, tau2 ~ Gamma(tau1/2,tau2/2)psi1 | nu2, psi2 ~ IW(nu2,psi2)Note that the inverted-Wishart prior is parametrized such that if A ~ IWq(nu, psi) then E(A)= psiinv/(nu-q-1).To let part of the baseline distribution fixed at a particular value, set the corresponding hyperparameters of the prior distributions to NULL in the hyperprior specification of the model.Although the baseline distribution, G0, is a conjugate prior in this model specification, an algorithm based on auxiliary parameters is adopted. Specifically, the algorithm 8 with m=1 of Neal (2000) is considered inthe DPMdencens function.Finally, note that this function can be used to fit the DPM of normals model for ordinal data proposed by Kottas, Mueller and Quintana (2005). In this case, the arbitrary cut-off points must be specified in left and right. Samples from the predictive distribution contained in the (last columns) of the object randsave (please see below) can be used to obtain an estimate of the cell probabilities.ValueAn object of class DPMdencens representing the DP mixture of normals model fit. Generic functions such as print, summary, and plot have methods to show the results of the fit. The results include the baseline parameters, alpha, and the number of clusters.The function DPrandom can be used to extract the posterior mean of the subject-specific means and covariance matrices.The MCMC samples of the parameters and the errors in the model are stored in the object thetasave and randsave, respectively. Both objects are included in the list save.state and are matrices which can be analyzed directly by functions provided by the coda package.The list state in the output object contains the current value of the parameters necessary to restart the analysis. If you want to specify different starting values to run multiple chains set status=TRUE and create the list state based on this starting values. In this case the list state must include the following objects:ncluster an integer giving the number of clusters.muclus a matrix of dimension (nobservations+100)*(nvariables) giving the means of the clusters (only the first ncluster are considered to start the chain). sigmaclus a matrix of dimension(nobservations+100)*( (nvariables)*((nvariables)+1)/2) giving the lowermatrix of the covariance matrix of the clusters (only the first ncluster areconsidered to start the chain).ss an interger vector defining to which of the ncluster clusters each observation belongs.alpha giving the value of the precision parameter.m1giving the mean of the normal components of the baseline distribution.k0giving the scale parameter of the normal part of the baseline distribution. psi1giving the scale matrix of the inverted-Wishart part of the baseline distribution.y giving the matrix of imputed data points.Author(s)Alejandro Jara <atjara@uc.cl>ReferencesEscobar, M.D. and West, M. (1995) Bayesian Density Estimation and Inference Using Mixtures. Journal of the American Statistical Association, 90: 577-588.Kottas, A., Mueller, P., Quintana, F. (2005). Nonparametric Bayesian modeling for multivariate ordinal data. Journal of Computational and Graphical Statistics, 14: 610-625.Neal, R. M. (2000). Markov Chain sampling methods for Dirichlet process mixture models. Journal of Computational and Graphical Statistics, 9: 249-265.See AlsoDPrandom, DPdensityExamples## Not run:##################################### Bivariate example:# Censored data is artificially# created####################################data(airquality)缺失数据为NAattach(airquality)将数据中变量能直接读取ozone <- Ozone**(1/3)开立方根radiation <- Solar.R重新赋名y <- na.omit(cbind(radiation,ozone))删除带有na的行,并给出行号# create censored-dataxxlim <- seq(0,300,50)yylim <- seq(1.5,5.5,1)生成两个数列left <- matrix(0,nrow=nrow(y),ncol=2)right <- matrix(0,nrow=nrow(y),ncol=2)生成与变量y同行,列为2的全0阵for(i in 1:nrow(y)){left[i,1] <- NAright[i,1] <- NAif(y[i,1] < xxlim[1]) right[i,1] <- xxlim[1]for(j in 1:length(xxlim)){if(y[i,1] >= xxlim[j]) left[i,1] <- xxlim[j]if(y[i,1] >= xxlim[j]) right[i,1] <- xxlim[j+1] }left[i,2] <- NAright[i,2] <- NAif(y[i,2] < yylim[1]) right[i,2] <- yylim[1]for(j in 1:length(yylim)){if(y[i,2] >= yylim[j]) left[i,2] <- yylim[j]if(y[i,2] >= yylim[j]) right[i,2] <- yylim[j+1] }}> left[,1] [,2][1,] 150 2.5[2,] 100 2.5[3,] 100 1.5[4,] 300 2.5[5,] 250 2.5[6,] 50 2.5[7,] 0 1.5[8,] 250 2.5[9,] 250 1.5[10,] 250 1.5[11,] 50 2.5[12,] 300 1.5[13,] 300 2.5[14,] 50 1.5[15,] 300 2.5[16,] 0 1.5[17,] 0 NA[18,] 300 1.5[19,] 0 1.5[20,] 50 2.5[21,] 0 2.5[22,] 250 3.5[23,] 200 4.5[24,] 250 2.5[25,] 100 2.5[26,] 250 3.5[28,] 100 2.5 [29,] 150 2.5 [30,] 250 2.5 [31,] 0 2.5 [32,] 100 1.5 [33,] 100 1.5 [34,] 250 4.5 [35,] 200 3.5 [36,] 200 2.5 [37,] 150 3.5 [38,] 300 2.5 [39,] 250 3.5 [40,] 250 4.5 [41,] 250 4.5 [42,] 150 3.5 [43,] 250 1.5 [44,] 150 2.5 [45,] 0 1.5 [46,] 250 3.5 [47,] 250 2.5 [48,] 250 3.5 [49,] 150 3.5 [50,] 200 3.5 [51,] 0 2.5 [52,] 250 3.5 [53,] 200 4.5 [54,] 50 2.5 [55,] 50 3.5 [56,] 200 3.5 [57,] 250 3.5 [58,] 250 3.5 [59,] 250 3.5 [60,] 50 2.5 [61,] 0 1.5 [62,] 50 2.5 [63,] 250 4.5 [64,] 200 3.5 [65,] 200 4.5 [66,] 150 3.5 [67,] 250 2.5 [68,] 150 3.5 [69,] 50 2.5 [70,] 50 3.5[72,] 200 2.5 [73,] 150 3.5 [74,] 250 2.5 [75,] 0 1.5 [76,] 200 3.5 [77,] 200 5.5 [78,] 200 3.5 [79,] 200 3.5 [80,] 200 4.5 [81,] 200 3.5 [82,] 150 3.5 [83,] 150 4.5 [84,] 150 3.5 [85,] 150 3.5 [86,] 150 3.5 [87,] 50 3.5 [88,] 50 2.5 [89,] 250 2.5 [90,] 200 2.5 [91,] 200 2.5 [92,] 250 2.5 [93,] 200 3.5 [94,] 250 2.5 [95,] 200 2.5 [96,] 0 1.5 [97,] 100 1.5 [98,] 200 3.5 [99,] 200 2.5 [100,] 0 1.5 [101,] 200 2.5 [102,] 200 2.5 [103,] 200 1.5 [104,] 0 2.5 [105,] 100 2.5 [106,] 0 1.5 [107,] 0 1.5 [108,] 150 2.5 [109,] 150 1.5 [110,] 100 2.5 [111,] 200 2.5 > right[,1] [,2] [1,] 200 3.5[3,] 150 2.5 [4,] NA 3.5 [5,] 300 3.5 [6,] 100 3.5 [7,] 50 2.5 [8,] 300 3.5 [9,] 300 2.5 [10,] 300 2.5 [11,] 100 3.5 [12,] NA 2.5 [13,] NA 3.5 [14,] 100 2.5 [15,] NA 3.5 [16,] 50 2.5 [17,] 50 1.5 [18,] NA 2.5 [19,] 50 2.5 [20,] 100 3.5 [21,] 50 3.5 [22,] 300 4.5 [23,] 250 5.5 [24,] 300 3.5 [25,] 150 3.5 [26,] 300 4.5 [27,] NA 3.5 [28,] 150 3.5 [29,] 200 3.5 [30,] 300 3.5 [31,] 50 3.5 [32,] 150 2.5 [33,] 150 2.5 [34,] 300 5.5 [35,] 250 4.5 [36,] 250 3.5 [37,] 200 4.5 [38,] NA 3.5 [39,] 300 4.5 [40,] 300 5.5 [41,] 300 5.5 [42,] 200 4.5 [43,] 300 2.5 [44,] 200 3.5 [45,] 50 2.5[47,] 300 3.5 [48,] 300 4.5 [49,] 200 4.5 [50,] 250 4.5 [51,] 50 3.5 [52,] 300 4.5 [53,] 250 5.5 [54,] 100 3.5 [55,] 100 4.5 [56,] 250 4.5 [57,] 300 4.5 [58,] 300 4.5 [59,] 300 4.5 [60,] 100 3.5 [61,] 50 2.5 [62,] 100 3.5 [63,] 300 5.5 [64,] 250 4.5 [65,] 250 5.5 [66,] 200 4.5 [67,] 300 3.5 [68,] 200 4.5 [69,] 100 3.5 [70,] 100 4.5 [71,] 150 3.5 [72,] 250 3.5 [73,] 200 4.5 [74,] 300 3.5 [75,] 50 2.5 [76,] 250 4.5 [77,] 250 NA [78,] 250 4.5 [79,] 250 4.5 [80,] 250 5.5 [81,] 250 4.5 [82,] 200 4.5 [83,] 200 5.5 [84,] 200 4.5 [85,] 200 4.5 [86,] 200 4.5 [87,] 100 4.5 [88,] 100 3.5 [89,] 300 3.5[90,] 250 3.5[91,] 250 3.5[92,] 300 3.5[93,] 250 4.5[94,] 300 3.5[95,] 250 3.5[96,] 50 2.5[97,] 150 2.5[98,] 250 4.5[99,] 250 3.5[100,] 50 2.5[101,] 250 3.5[102,] 250 3.5[103,] 250 2.5[104,] 50 3.5[105,] 150 3.5[106,] 50 2.5[107,] 50 2.5[108,] 200 3.5[109,] 200 2.5[110,] 150 3.5[111,] 250 3.5# Prior informations2 <- matrix(c(10000,0,0,1),ncol=2)> s2[,1] [,2][1,] 10000 0[2,] 0 1m2 <- c(180,3)psiinv2 <- diag(c(1/10000,1),2)prior <- list(alpha=1,nu1=4,nu2=4,s2=s2,m2=m2,psiinv2=psiinv2,tau1=0.01,tau2=0.01)# Initial statestate <- NULL# MCMC parametersnburn <- 5000nsave <- 5000nskip <- 3ndisplay <- 1000mcmc <- list(nburn=nburn,nsave=nsave,nskip=nskip,ndisplay=ndisplay)# Fitting the modelfit1 <- DPMdencens(left=left,right=right,ngrid=100,prior=prior,mcmc=mcmc,state=state,status=TRUE)fit1DPM model for interval-censored dataCall:DPMdencens.default(left = left, right = right, ngrid = 100, prior = prior, mcmc = mcmc, state = state, status = TRUE)Posterior Inference of Parameters:m1-var1 m1-var2 k0 psi1-var1:var1162.672129 3.184599 0.107712 0.000535psi1-var1:var2 psi1-var2:var2 ncluster alpha-0.009391 1.021222 4.219400 1.000000Number of Observations: 111Number of Variables: 2summary(fit1)DPM model for interval-censored dataCall:DPMdencens.default(left = left, right = right, ngrid = 100, prior = prior, mcmc = mcmc, state = state, status = TRUE)Baseline distribution:Mean Median Std. Dev. Naive Std.Errorm1-var1 1.627e+02 1.609e+02 6.431e+01 9.094e-01m1-var2 3.185e+00 3.190e+00 7.995e-01 1.131e-02k0 1.077e-01 5.657e-02 1.595e-01 2.256e-03psi1-var1:var1 5.350e-04 3.130e-04 6.453e-04 9.126e-06psi1-var1:var2 -9.391e-03 -4.588e-03 1.651e-02 2.334e-04psi1-var2:var2 1.021e+00 8.431e-01 6.868e-01 9.713e-0395%HPD-Low 95%HPD-Uppm1-var1 4.102e+01 2.914e+02m1-var2 1.586e+00 4.738e+00k0 1.785e-04 3.740e-01psi1-var1:var1 2.886e-05 1.664e-03psi1-var1:var2 -4.324e-02 1.130e-02psi1-var2:var2 2.344e-01 2.238e+00Precision parameter:Mean Median Std. Dev. Naive Std.Error 95%HPD-Low ncluster 4.21940 4.00000 1.32711 0.01877 2.00000 95%HPD-Uppncluster 7.00000Number of Observations: 111Number of Variables: 2# Plot the estimated densityplot(fit1)# Extracting the univariate density estimatescbind(fit1$grid[,1],fit1$funi[[1]])cbind(fit1$grid[,2],fit1$funi[[2]])# Extracting the bivariate density estimatesfit1$grid[,1]fit1$grid[,2]fit1$fbiv[[1]]# Plot of the estimated density along with the# true data points and censoring limitscontour(fit1$grid[,1],fit1$grid[,2],fit1$fbiv[[1]])points(y)abline(v=xxlim) abline(h=yylim)## End(Not run)。